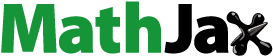
Abstract
With the rapid development of e-commerce, selling fake commodities happens more frequently. It is closely related to consumers' interests, merchants' online word of mouth, and e-commerce platforms' long-term development. To explore and analyse this problem, an evolutionary game model is constructed among a merchant, an e-commerce platform, and a consumer. Some factors are considered in the model, such as online word of mouth of the merchant and punishment mechanism of the platform. Then stable strategies are obtained for all participants and the influence of each factor on the choices of their strategies is analysed. The stability of equilibrium points is discussed through the Jacobian matrix and Lyapunov's indirect method. And evolutionary stable strategy combinations are obtained. The results show that e-commerce platforms' supervision and consumers' evaluations are shown to have important impacts on merchants' behaviours; reducing supervision costs and increasing punishment on merchants can help improve the enthusiasm of platforms' supervision clearly; and merchants' negative online word of mouth brings about potential economic losses. At last, the influence of each factor on the evolution of each participant's strategies is simulated by Matlab 2014a. And potential evolutionary stable strategy combinations of the game system are verified under different conditions.
1. Introduction
Today, online shopping brings convenience to consumers. However, fake commodities are raging online at the same time. In China, the number of complaints about online shopping ranked the first among service complaints according to the Chinese National Consumers Association. It is necessary to strengthen the protection of consumers' rights. Some consumers do not pay attention to or recognize fake commodities. Even if some consumers find fakes, they may give up the right to return or exchange because they do not want to spend too much time. Some merchants sell fake commodities with luck to get more profits, which makes more and more consumers distrust e-commerce platforms and merchants. Not only does it severely harm the interests of consumers but also it has negative impacts on the platforms. And thus merchants' sales will reduce in the long run. We carry out game analysis to investigate what factors affect selling fake commodities of merchants and how to prevent it.
Quite a lot of works have been done on this issue, and evolutionary game models are used frequently. For example, the two-party game models were constructed and analysed in Yang et al. (Citation2017) between the e-commerce and consumers, in Yu and Liu (Citation2016) between the government and the third party, in Li et al. (Citation2018) between merchants and e-commerce platforms, and in Wu et al. (Citation2019) between the government and consumers. Static and dynamic game models are also widely used to analyse e-commerce transactions. To study the influence of the reputation feedback system on the P2P trading platform, a dynamic game model was constructed in Wang et al. (Citation2017). When it comes to B2C e-commerce, Liu et al. (Citation2018) proposed a credit risk game mechanism and logical Petri nets theory to study the credit risk. Nagurney and Li (Citation2015) developed a supply chain network game theory model to study the competition of quality and price among different products. Wu et al. (Citation2018) established a supply chain with a single retailer and manufacturers. The retailer was responsible for the improvement of store-assistance to reduce consumer returns. Zhang et al. (Citation2019) established a game model between two logistics service providers in an e-commerce logistics market. They analysed the influence of order quantity and service level. Signalling game models are also applied to discuss the problem. For example, Xu et al. (Citation2014) constructed a product quality information transmission model between the government and consumers. They concluded that the government's intervention management helped keep the information true.
Confronting the possible punishment, some merchants still choose to sell fake commodities. Except for the temptation of high profits, there are obviously many other reasons. The online market environment is one of the most important factors. Asymmetric information and market uncertainty were considered in Li and Wen (Citation2013) as main causes of selling fake commodities by e-commerce companies. The lack of merchants' behaviour information in e-commerce was thought to be a major reason for selling fakes in Indu et al. (Citation2016). Beqiraj et al. (Citation2020) proved that some behaviours of selling fakes were politically tolerated for positive social returns through an empirical analysis. Altug (Citation2016) pointed out that the grey market price had both a negative demand-cannibalization effect and a positive inventory correction effect on retailers' stocking choices and manufacturer's profits. Chaudhry (Citation2014) discussed the principal factors that encouraged a grey market, and summarized managerial tactics to combat grey markets. The influence of product quality information was discussed in Akdeniz et al. (Citation2013). Carpineto and Romano (Citation2017) studied how to distinguish web sites selling fakes by identifying suitable learning features. For fraud detection among the markets, please refer to Zimmerman and Blythe (Citation2017) for more details.
We find that researches about merchants selling fake commodities mainly focus on the analysis of e-commerce platforms and merchants. There are few works about the analysis of returning commodities by consumers, online word of mouth of merchants, and punishment mechanism of platforms. In fact, consumers' negligence in complaints is also an important reason. So we add consumers to the game model to study the problem more deeply. Under the assumptions of asymmetric information and bounded rationality, evolutionary games can effectively describe the evolution of participants' strategies (for the knowledge of the evolutionary game, please refer to Hofbauer et al. (Citation1979) and Weibull (Citation1995). Our work is organized as follows.
In Section 2, we build some assumptions and establish an evolutionary game model with a merchant, an e-commerce platform, and a consumer as the three participants.
In Section 3, we analyse the evolutionary stability of all the participants' strategies. And we study the influence of each factor on the choices of their strategies in detail. Then, the stability of equilibrium points is discussed through the Jacobian matrix and Lyapunov's indirect method (Lyapunov, Citation1992). And evolutionary stable strategy combinations are obtained under different conditions.
In Section 4, we simulate the influence of each factor on the evolution of each participant's strategies and verify the stability of the game system.
In Section 5, we draw a conclusion and have a look into the future.
2. Model assumption and construction
We establish a three-party evolutionary game model among a merchant, an e-commerce platform, and a consumer in this section. Before that we need to build several assumptions and show the meaning of the symbols used in the sequel.
2.1. Assumptions and parameters of the model
Considering the real situation in the markets online, we build the following assumptions. They will also help analyse the model.
Assumption 2.1
The merchant is participant 1, the e-commerce platform is participant 2, and the consumer is participant 3, all of whom are of bounded rationality. Their choices of strategies gradually evolve with time and are stable to the optimal strategies.
Assumption 2.2
The merchant's strategic space is (selling genuine commodities, selling fake commodities). He chooses
with the probability x, and chooses
with the probability
, where
. The strategic space of the e-commerce platform is
(strict supervision, slack supervision). It chooses
with the probability y, and chooses
with the probability
, where
. Strategic space of the consumer is
(accepting, returning). He chooses
with the probability z, and chooses
with the probability
, where
. Note that the probabilities x, y, and z are measures, respectively, for average decisions made by the merchant, the e-commerce platform, and the consumer. The consumer is often in a state of serious information asymmetry of commodities, so he does not know if commodities are genuine. We suppose that the consumer chooses to return the commodity only when he thinks it is fake.
Assumption 2.3
The price of a genuine commodity sold by the merchant is P. Considering that fake commodities are usually sold at a reduced price in practice, we set the price of a fake commodity as . The cost of purchasing a genuine commodity by the merchant is
and of a fake one is
. If the consumer returns a commodity, the merchant will bear the time cost and depreciation loss B.
Assumption 2.4
If the merchant sells a commodity and the consumer accepts it, the e-commerce platform will gain revenue . The strict supervision cost of the e-commerce platform is
. If the merchant sells a fake commodity and the e-commerce platform supervises strictly, the e-commerce platform will impose an economic penalty F on the merchant. Furthermore, the merchant must resend a genuine commodity and be supervised again to ensure that every commodity received by the consumer after supervision is genuine. If the e-commerce platform supervises slackly and the commodity is returned, the e-commerce platform will inspect it and spend supervision cost
. And if the commodity is fake, the merchant will be imposed the penalty F, and have to pay economic compensation I to the consumer.
Assumption 2.5
According to Assumption 2.4, the consumer receives a fake commodity only when the merchant sells a fake commodity and the e-commerce platform supervises slackly. If the consumer receives a genuine commodity and accepts it, he will gain the utility . It represents the average level of consumers' utility, because the utility of commodities is heterogeneous over different consumers in practice. A fake commodity is usually different from a genuine one for consumers. However, a fake commodity usually has a lower price, so it is still attractive. So we assume that the consumer selects returning at an average possibility. If the consumer receives a fake commodity and accepts it, he will gain the utility
, which is lower than the utility brought by a genuine commodity. Considering that the fake commodity brings a bad experience to the consumer, he always issues negative comments. They damage the online word of mouth of the merchant. We assume the potential loss of revenues brought by negative online word of mouth as
. It will influence the merchant obviously in the future. If the consumer chooses to return the commodity, the cost (including time cost, freight, communication cost with the merchant, etc.) will be
.
Based on the above assumptions and explanations of the model, the main parameters of the model and their implications are summarized as shown in Table .
Table 1. Main parameters' symbols and implications of the three-party evolutionary game model.
2.2. Model construction
According to the assumptions and parameters, we build a mixed strategy game model with the merchant, e-commerce platform, and consumer as participants. Obviously, there are eight game strategy combinations. Their payoffs under eight different situations are shown in Table .
Table 2. Payoff matrix of the mixed strategy game model among the merchant, e-commerce platform, and consumer.
3. Analysis of model
3.1. Stability analysis of the merchant's strategies
The merchant's expect revenues are denoted by and
for selling a genuine commodity and a fake one, respectively. Then his overall expected revenue is denoted by
. According to Table , they are obtained as follows:
(1)
(1) In the evolutionary game theory, replication dynamics are an important mechanism. According to Xie (Citation2017), replication dynamics describe the dynamic strategy adjustment of bounded rational players who have the ability to simply imitate dominant strategies. The basic principle is that strategies with better results than the average level will be gradually adopted by more players. Therefore, the proportion of players who adopt various strategies in the group will change. The change of the number of strategies can be solved by dynamic differential equations. On the basis of Malthusian dynamical equations (see Weibull (Citation1995)), the growth rate of the number of strategies of selling genuine commodities by the merchant is equal to
minus
. Then the replication dynamic equation for the merchant is shown below,
(2)
(2) where t is the time and
(3)
(3) Then we have
(4)
(4) According to the stability theorem of first-order differential equations, the probability x of the merchant selling genuine commodities is in a stable state when
and
. When
, then
,
, and
, so all levels of x are in evolutionary stable states. The merchant's strategy will not change with time under this condition. If
, then
. And then x = 1 is the only evolutionary stable strategy (ESS) since
and
. If
, then
, and
will be a decreasing function with respect to z. When
, we have
; then
; and then x = 0 is the only ESS. When
, we have
; and then x = 1 is the only ESS. The phase diagram of the merchant's strategy evolution is shown in Figure . The curved surface
exists in the cubic only under the condition
.
Figure 1. Phase diagram of the merchant's strategy evolution. It shows the relationship between the merchant's ESS and the probability z. Arrows represent the merchant's strategy choice.
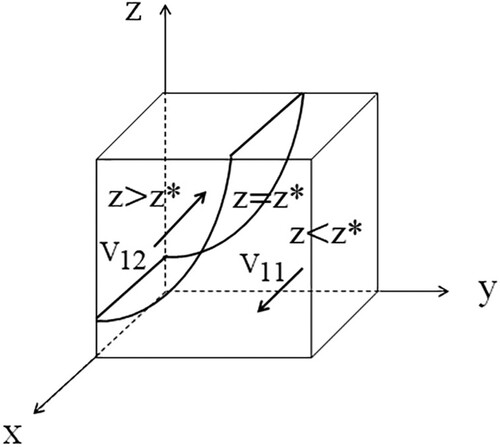
According to Figure , when the initial state of the merchant's strategy is in , that is
, x = 1 is an equilibrium point. And selling genuine commodities is the only ESS. When z becomes less, the ESS of the merchant is to sell genuine commodities. When the initial state of the merchant's strategy is in
, that is
, x = 0 is an equilibrium point. And selling fake commodities is the only ESS for the merchant. When z becomes larger, the ESS for the merchant is to sell fake commodities.
Proposition 3.1
When , the probability x increases to 1 with the increase of
, and the decrease of
, and vice versa.
Proof.
We take as the function of
and α. Then we have
and
. Therefore, the value of
becomes larger with the increase of
, and the decrease of
. Then the volume of
increases. And then the probability x for ESS of the merchant will increase to 1.
3.2. Stability analysis of the e-commerce platform's strategies
The e-commerce platform's expected revenues are denoted by and
for strict supervision and slack supervision, respectively. Its overall expected revenue is denoted by
. According to Table , they are obtained as follows:
(5)
(5) On the basis of Malthusian dynamical equation, the replication dynamic equation for the e-commerce platform is shown below,
(6)
(6) where
(7)
(7) Then we have
(8)
(8) According to the stability theorem of differential equations, the probability y of the e-commerce platform choosing strict supervision is in a stable state when
and
. When
, then
,
, and
, so all levels of y are in evolutionary stable states. The e-commerce platform's strategy will not change with time under this condition. If
, then
, and
will be a decreasing function with respect to x. When
, we have
; then
; and then y = 0 is the only ESS. When
, we have
; and then y = 1 is the only ESS. If
, then
, and
will be an increasing function with respect to x. Because
is always larger than 1 under this condition, then
. We have
; then
; and then y = 0 is the only ESS. The phase diagram of the e-commerce platform's strategy evolution is shown in Figure . The curved surface
exists in the cubic only under the condition
.
Figure 2. Phase diagram of the e-commerce platform's strategy evolution. It shows the relationship between the e-commerce platform's ESS and the probability x. Arrows represent the e-commerce platform's strategy choice.
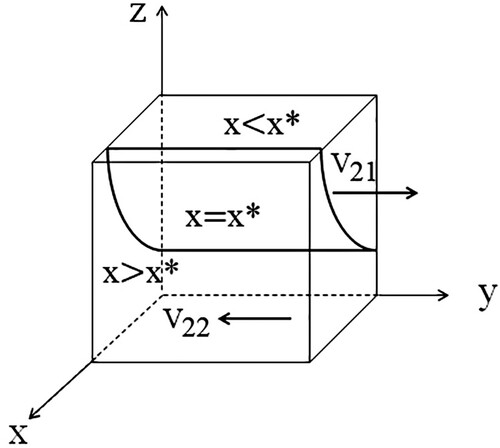
According to Figure , when the initial state of the e-commerce platform's strategy is in , that is
, y = 1 is an equilibrium point, and the e-commerce platform's strict supervision is the only ESS. When x becomes less, the ESS of the e-commerce platforms is strict supervision. When the initial state of the e-commerce platform's strategy is in
, that is
, y = 0 is an equilibrium point, and slack supervision is the only ESS for the e-commerce platform. When x becomes larger, the ESS for the e-commerce platform is slack supervision.
By a similar argument with as that in the proof of Proposition 3.1, we can obtain the following proposition.
Proposition 3.2
When , the probability y decreases to 0, with the decrease of z, F, and the increase of
, and vice versa.
3.3. Stability analysis of the consumer's strategies
The consumer's expected revenues are denoted by and
for accepting and returning commodities, respectively. His overall expected revenue is denoted by
. According to Table , they are obtained as follows:
(9)
(9) The replication dynamic equation for the consumer is shown below,
(10)
(10) where
(11)
(11) Then we have
(12)
(12) The probability z of the consumer accepting commodities is in a stable state when
and
. When
, then
,
, and
, so all levels of z are in evolutionary stable states. The consumer's strategy will not change with time under this condition. Obviously,
is an increasing function with respect to y. When
, we have
; then
; and then z = 1 is the only ESS. When
, we have
; then
, and then z = 0 is the only ESS. The phase diagram of the consumer's strategy evolution is shown in Figure .
Figure 3. Phase diagram of the consumer's strategy evolution. It shows the relationship between the consumer's ESS and the probability y. Arrows represent the consumer's strategy choice.
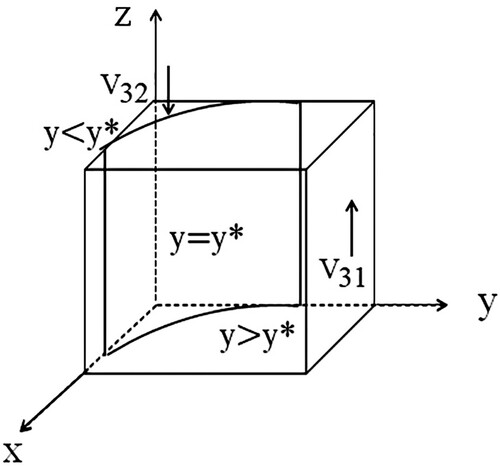
According to Figure , when the initial state of the consumer's strategy is in , that is
, z = 1 is an equilibrium point and accepting commodities is the only ESS for the consumer. When the probability y under strict supervision by the e-commerce platform becomes larger, the ESS for the consumer is accepting commodities. When the initial state of the consumer's strategy is in
, that is
, z = 0 is an equilibrium point and returning commodities is the only ESS for the consumer. When the probability y under strict supervision by the e-commerce platform becomes less, the ESS of the consumer is returning commodities.
We now obtain the following proposition as we have done with and
.
Proposition 3.3
The probability z increases to 1 with the increase of , and the decrease of I, and vice versa.
3.4. Stability analysis of systemic equilibrium points of the three-party evolutionary game
By the constraints ,
, and
, we obtain systemic equilibrium points for the evolutionary game system consisting of Equations (Equation2
(2)
(2) ), (Equation6
(6)
(6) ), and (Equation10
(10)
(10) ). These are
,
,
,
,
,
,
, and
.
The Jacobian matrix of the three-party evolutionary game system is
(13)
(13) Lyapunov's stability theory is the most powerful theoretical tool to analyse the stability of a system. According to Lyapunov's indirect method (Lyapunov, Citation1992), we can judge whether the equilibrium point is stable without solving differential equations. Its basic conclusions are as follows: if all eigenvalues of the Jacobian matrix have negative real parts, the equilibrium point will be asymptotically stable; if at least one eigenvalue of the matrix has a positive real part, the equilibrium point will be unstable; if other eigenvalues of the matrix have negative real parts except for the eigenvalues whose real parts are zero, the equilibrium point will be in a critical state, and the stability would not be determined by symbols of eigenvalues. And eigenvalues of the Jacobian matrix are calculated by
where
(14)
(14) Obviously, it has three eigenvalues
and
. Then we analyse the stability of each equilibrium point based on symbols of the eigenvalues. Table shows
and
under
, and symbols of their real parts.
Table 3. Stability analysis of equilibrium points.
We see from Table that , and
are unstable, because they all have at least one eigenvalue of Jacobian matrices with positive real parts. And the points
and
may be ESS combinations.
When , the evolutionary game system has an ESS combination
, and
is unstable. When
,
is unstable. When
,
, and
,
is an ESS combination.
Therefore, if the values of and F are too small, no matter whether the merchant sells a genuine commodity or not, the consumer will accept it unconditionally and blindly. Just as bad money drives out good, so do fake commodities drive out the genuine ones. In order to avoid this situation, the influence of word of mouth should be improved and penalty implied on the merchant due to selling fakes should be strengthened. And if the value of
is too high, the e-commerce platform will supervise slackly.
4. Simulation analysis
To verify the validity of evolutionary stability analysis, we assign the model with numerical values according to actual situations. Then we carry out the numerical simulation by Matlab 2014a.
We assign , and
, which satisfy the condition of
. We denote the assignment by Array 1. Then we analyse the influence of F,
,
, and I on the process and results of the evolutionary game. Note that we fix
in the evolutionary process, and the process starts from t = 0.
Firstly, to analyse the influence of the change of economic penalty F on three participants, we assign F with three different values, 100, 150, and 200. The simulation results of the system are shown in Figure .
Figure 4. Influence of economic penalty F's changes on x, y, and z. (a) Influence of F's changes on x, y, and z and (b) influence of F's changes on x and y.
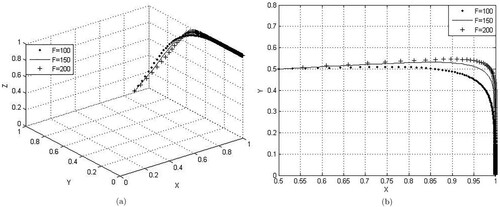
The system can arrive at the ESS combination after 100 times' evolutions. We see from Figure that the probability x of the merchant selling genuine commodities increases and the probability 1−y of the e-commerce platform slack supervision decreases with the increase of the economic penalty imposed on merchants F.
Secondly, to analyse the influence of the change of supervision cost on three participants, we assign
with 20, 30, and 40, respectively, and the simulation results are shown in Figure .
Figure 5. Influence of supervision cost 's changes on probabilities x, y, and z. (a) Influence of
's changes on x, y and z and (b) influence of
's changes on y with time t.
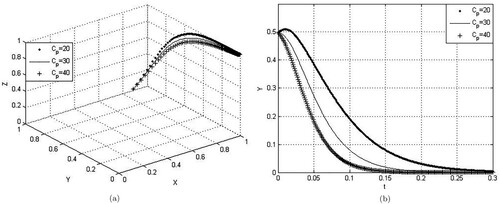
The system can arrive at after 300 times' evolutions. We see from Figure that the higher the supervision cost
is, the lower the probability y under strict supervision is. And
accelerates the evolution speed of the e-commerce platform's slack supervision.
Thirdly, to analyse the influence of the change of losses brought by negative online word of mouth , we assign
with 50, 100, and 200, and the simulation results are shown in Figure .
Figure 6. Influence of economic losses 's changes brought by negative online word of mouth on x, y, and z. (a) Influence of
's changes on x, y, and z and (b) influence of
's changes on x with time t.
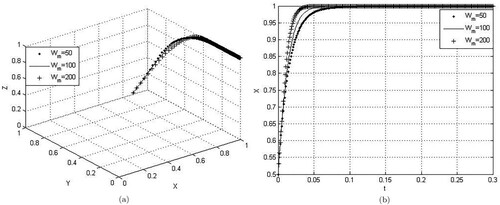
The system can arrive at after 300 times' evolutions. We see from Figure that the increase of
accelerates the evolution speed of the merchant selling genuine commodities. The higher the potential loss of the merchant brought by negative online word of mouth
is, the higher the probability x of the merchant selling genuine commodities is. Therefore, the e-commerce platform should broaden the consumer's voice channels and encourage him to issue comments on commodities after purchasing.
Fourthly, to analyse the influence of the change of economic compensation I, we assign I with 10, 50, and 100, and the simulation results are shown in Figure .
Figure 7. Influence of economic compensation I's changes on probabilities x, y, z. (a) Influence of I's changes on x, y, and z and (b) influence of I's changes on x and z.
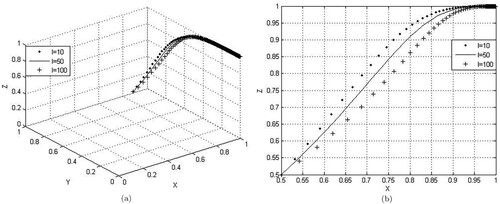
The system can arrive at after 300 times' evolutions. We see from Figure that the increase of I accelerates the evolution speed of the merchant selling genuine commodities. Moreover, the probability of x selling genuine commodities of the merchant and the probability z of the consumer accepting commodities increase with the increase of I.
We then assign and
, which satisfy the conditions of
,
, and
. We denote the assignment by Array 2. We analyse the influence of F,
, and
on the process and results of the evolutionary game as we have done for Array 1. Similarly, we fix
in the evolutionary process, and the process starts from t = 0. The results are shown in Figures − .
Figure 8. Influence of economic penalty F's changes on probabilities x and y with time t. (a) Influence of F's changes on x with time and (b) influence of F's changes on y with time.
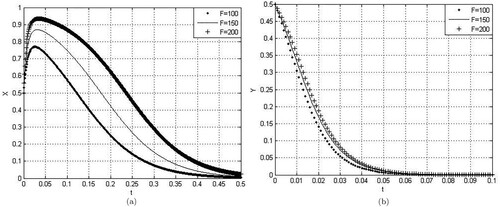
Figure 10. Influence of loss 's changes brought by negative online word of mouth on the probability x with time t.
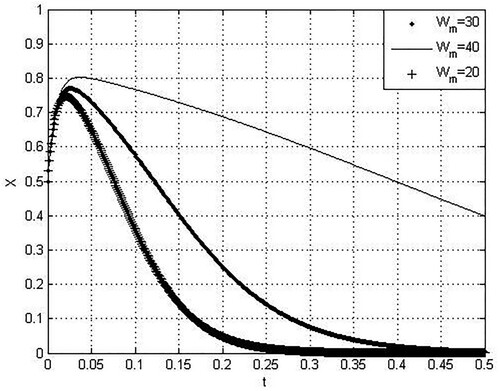
The system can arrive at the ESS combination after 500 times' evolutions. We see from Figure that the increase of economic penalty imposed on the merchant decelerates the evolution speed of the merchant selling fake commodities and slack supervision by the e-commerce platform. The probability 1−x of selling fake commodities of the merchant and the probability 1−y under slack supervision by the e-commerce platform decrease with the increase of F.
The system can arrive at after 100 times' evolutions. We see from Figure shows that the increase of
accelerates the evolution speed of slack supervision by the e-commerce platform. The higher the supervision cost
is, the lower the probability y under strict supervision is.
The system can arrive at after 500 times' evolutions. We see from Figure that the increase of
decelerates the evolution speed of the merchant selling fake commodities. The higher the potential loss of the merchant brought by negative online word of mouth
is, the higher the probability x of selling genuine commodities is.
5. Conclusion
From the perspective of merchants selling fake commodities, we have established an evolutionary game model among a merchant, an e-commerce platform, and a consumer. We have introduced parameters such as potential losses brought by negative online word of mouth and economic penalties to make the model more explanatory.
We have discussed the stability of equilibrium points of the game system and obtained evolutionary stable strategy combinations. Obviously, selling genuine commodities by merchants, slack supervision by the e-commerce platform, and accepting commodities by consumers are the optimal and most efficient strategies. And we have further analysed corresponding conditions. The main results are as follows. Firstly, e-commerce platforms' supervision and consumers' evaluations have important impacts on the behaviours of merchants. Secondly, reducing supervision costs and increasing punishment strength on merchants help improve the enthusiasm for supervision by platforms so as to decrease merchants' selling fake commodities. Thirdly, online word of mouth formed by consumers' evaluations can cause potential economic losses to merchants and put pressure on them so that merchants reduce the possibility of selling fake commodities.
In the future, we hope to do deep research based on repeated dynamic game models to track the bad effects of selling fakes repeatedly. Furthermore, we will set the penalty and reputation loss as functions of the times, and analyse the behaviours of merchants and e-commerce platforms.
Disclosure statement
No potential conflict of interest was reported by the author(s).
Additional information
Funding
References
- Akdeniz, B., Calantone, R. J., & Voorhees, C. M. (2013). Effectiveness of marketing cues on consumer perceptions of quality: The moderating roles of brand reputation and third-party information. Psychology and Marketing, 30(1), 76–89. https://doi.org/https://doi.org/10.1002/mar.20590
- Altug, M. S. (2016). The dynamics of domestic gray markets and its impact on supply chains. Production and Operations Management, 26(3), 525–541. https://doi.org/https://doi.org/10.1111/poms.12645
- Beqiraj, E., Fedeli, S., & Giuriato, L. (2020). Policy tolerance of economic crime? An empirical analysis of the effect of counterfeiting on Italian trade. European Journal of Political Economy, 65, 101933. https://doi.org/https://doi.org/10.1016/j.ejpoleco.2020.101933.
- Carpineto, C., & Romano, G.. (2017). Learning to detect and measure fake ecommerce websites in search-engine results. Proceedings of the international conference on web intelligence. ACM Press the International Conference, 17, 403–410. https://doi.org/http://doi.org/10.1145/3106426.3106441, Leipzig, Germany, 2017.08.23-2017.08.26.
- Chaudhry, P. E. (2014). Confronting the gray market problem. Business Economics, 49(4), 263–270. https://doi.org/https://doi.org/10.1057/be.2014.29
- Hofbauer, J., Schuster, P., & Sigmund, K. (1979). A note on evolutionary stable strategies and game dynamics. Journal of Theoretical Biology, 81(3), 609–612. https://doi.org/https://doi.org/10.1016/0022-5193(79)90058-4
- Indu, T. R., Joseph, A., Divya, V. R., & Mathew, L. S. (2016). Fake product detection and reputation system for e-commerce. International Journal of Scientific Research in Science, Engineering and Technology, 2(3), 300–304. https://doi.org/http://doi.org/10.32628/IJSRSET162375
- Li, B., & Wen, D. (2013). A review on the mechanism of commodity quality problems in online shopping and its supervision. Finance and Trade Research, 24(2), 20–28. https://doi.org/https://doi.org/10.3969/j.issn.1001-6260.2013.02.003
- Li, J., Zhang, R., & Xu, Y. (2018). The regulation of e-commerce platform and the evolution game of merchants selling fake products. Journal of Systems Engineering, 33(5), 75–87. https://doi.org/https://doi.org/10.13383/j.cnki.jse.2018.05.008
- Liu, W., Feng, X., Zhang, F., Du, Y., & Yan, C. (2018). Analytic of B2C e-commerce credit mechanism mixed strategy risk behavior based on logical game petri nets. IEEE Access, 6, 29109–29131. https://doi.org/https://doi.org/10.1109/ACCESS.2018.2838765
- Lyapunov, A. M. (1992). The general problem of the stability of motion. International Journal of Control, 55(3), 531–534. https://doi.org/https://doi.org/10.1080/00207179208934253
- Nagurney, A., & Li, D. (2015). A supply chain network game theory model with product differentiation, outsourcing of production and distribution, and quality and price competition. Annals of Operations Research, 226(1), 479–503. https://doi.org/https://doi.org/10.1007/s10479-014-1692-5
- Wang, Y., Yang, J., & Qi, L. (2017). A game-theoretic model for the role of reputation feedback systems in peer-to-peer commerce. International Journal of Production Economics, 191, 178–193. https://doi.org/https://doi.org/10.1016/j.ijpe.2017.06.012
- Weibull, J. W. (1995). Evolutionary game theory. MIT Press.
- Wu, Z., Chen, D., & Feng, L. (2018). Design of optimal control strategies for a supply chain with competing manufacturers under consignment contract. Systems Science and Control Engineering, 6(1), 171–179. https://doi.org/https://doi.org/10.1080/21642583.2018.1474144
- Wu, B., Cheng, J., & Qi, Y. (2019). Tripartite evolutionary game analysis for “Deceive acquaintances” behavior of e-commerce platforms in cooperative supervision. Physica A: Statistical Mechanics and Its Applications, 550, 123892. https://doi.org/https://doi.org/10.1016/j.physa.2019.123892
- Xie, S. (2017). Economic game theory (4th ed.). Fudan University Press.
- Xu, Y., Gao, X., & Ying, Y.. (2014). A new information transmission model of product quality and safety based on government intervention. The 36th Annual International Conference of the IEEE Engineering in Medicine & Biology Society, 2014.11.01.
- Yang, F., Wang, A., Wu, J., & Tang, L. (2017). Research on e-commerce credit supervision mechanism based on C2B2C model of game theory. Systems Engineering Theory and Practice, 37(8), 2102–2110. https://doi.org/https://doi.org/10.12011/1000-6788(2017)08-2102-09
- Yu, T., & Liu, C. (2016). Game analysis and simulation study on the evolution of government and third party in product quality supervision. Chinese Management Science, 24(6), 90–96. https://doi.org/https://doi.org/10.16381/j.cnki.issn1003-207x.2016.06.011
- Zhang, M., Fu, Y., Zhao, Z., Pratap, S., & Huang, G. Q. (2019). Game theoretic analysis of horizontal carrier coordination with revenue sharing in e-commerce logistics. International Journal of Production Research, 57(5), 1524–1551. https://doi.org/https://doi.org/10.1080/00207543.2018.1492754
- Zimmerman, A., & Blythe, J. (2017). Business to business marketing management: a global perspective (3rd ed.). Routledge.