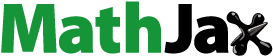
Abstract
This research aims to identify performance indicators and use them to prioritize banks in Iran. Today, the banking industry is severely challenged by decreasing revenues, especially during crisis such as the COVID-19 pandemic. Hence, evaluating banks to find their weaknesses is vital and shows how banks with flaws can be benchmarked from best practice banks. For this work, data is collected from Iranian banks and then evaluated based on the Delphi method. Since the importance of the considered factors is quite diverse, they should be ranked. We use Evaluation by an Area-Based Method of Ranking (EAMR) for this research study. As this method requires factor-specific weights, the Stepwise Weight Assessment Ratio Analysis (SWARA) method is used for determining these weights. This paper looks forward to introducing new hybrid MADM methods in an uncertain environment with high reliability in the results. This new model leads to ensure managers that they can make their decisions accurately. The results reveal the performance of Iranian banks and a respective ranking of them including a model for benchmarking. This empirical research study also provides useful guidance to a better understanding of performance measurement in the banking sector in Iran.
1. Introduction
Nowadays, the service industry has a crucial role in the economic development of most countries. The merit of this industry compared to traditional industries is that it is less based on specific resources and allows for innovative business models. In particular, many countries are looking forward to establishing banking or financial institutes to provide improved services to people of their own country and provide services to foreign companies or people to increase financial indicators such as the gross domestic product (GDP). Central banks, financial regulatory bodies, and government authorities need to achieve financial policy harmonization, especially during times of crisis such as during the current COVID-19 pandemic. Mirzaei et al. (Citation2021) suggested that Islamic banks (IBs) are assumed to be more resilient during crisis times than conventional banks (CBs).
Moreover, government authorities worldwide established intervention measures to mitigate significant financial shocks during the COVID-19 crisis. On the other hand, strict regulations on social distancing, massive pressure on national health systems, severe lockdown measures, as well as the psychological and emotional impact on the population have generated significant consequences on the economy. Indisputably, the banking system is facing a considerable challenge being forced to reconsider its priorities. The current reality requires efficient and urgent measures. Banking customers have high expectations regarding access to new financial products and services based on digital technologies.
Demirguc-Kunt et al. (Citation2020) highlighted specific prudential measures and policy interventions to reduce the stress for the banking system in the context of the COVID-19 pandemic by borrower assistance, liquidity support, and monetary support easing. Mirzaei et al. (Citation2021) investigated the effect of COVID-19 on bank performance. They concluded that Islamic banks reached a higher level of stock returns compared to conventional banks due to their pre-crisis degree of efficiency using a sample period from December 31, 2019 to March 31, 2020. However, Shahabi et al. (Citation2020) suggested that the impact of the COVID-19 pandemic on the banking sector has certain advantages such as a positive and open-minded attitude of customers towards new technologies, a considerable decrease in the number of bank branches and increased importance of Information Technology (IT). Sija (Citation2021) argued that the COVID-19 pandemic significantly influences banking employees’ performance based on certain factors such as compensation and benefits, employee recognition, training, and development. Shahabi et al. (Citation2020) also revealed that new technologies represent instruments against the severe restrictions based on lockdown and social distancing imposed by the COVID-19 pandemic. However, Batool et al. (Citation2021) pointed out that pandemics in general determine economic distress, so it is no great surprise that the COVID-19 pandemic is similar.
Shahabi et al. (Citation2020) defined the term of branchless banking or direct banking as a rather new concept, while considering the implementation of which is a large step in entering industry 4.0. Mehdiabadi et al. (Citation2020) suggested that the emergence of new technologies such as blockchain, robotics, etc., has had a significant impact on the performance of the banking industry.
In our study, we evaluate the performance of banks in Iran under the COVID-19 circumstances. The banking system has a crucial role in the economy of Iran. In 1979, after the revolution in Iran, all banks were in the public sector. After some decades, private banks reemerged. This emerging leads to competition between public and private banks, and hence they were forced to increase their performance. Some public and private banks did not have suitable performance, so it was imperative to be aware of the possibilities for improvement. Various approaches can be used for this purpose. One of them which is applied in this research is finding related performance indicators (PIs), and then based on these PIs, banks are ranked.
For ranking purposes, both mathematical and statistical methods can be used. In this research, mathematically based methods for Multiple Attribute Decision Making (MADM) are used. Let us note that this type of methods is also known as Multicriteria Decision Analysis (MCDA) or Multiple Criteria Decision Making (MCDM) which are, however, more generic terms (Hanne, Citation2012). MADM methods can be categorized into two types. The first category is based on pairwise comparisons such as BWM (Best-Worst Method), AHP (Analytical Hierarchy Process), ANP (Analytical Network Process), MACBETH (Measuring Attractiveness by a Categorical Based Evaluation Technique), and the second is based on decision matrix such as TOPSIS (Technique for Order of Preference by Similarity to Ideal Solution), VIKOR (VIekriterijumsko KOmpromisno Rangiranje), MULTI MOORA (MULTI Multi-Objective Optimization Method by Ratio Analysis), or ELECTRE (ÉLimination et Choix Traduisant la REalité). Each of these methods has some advantages and disadvantages. The reasons for using the Stepwise Weight Assessment Ratio Analysis (SWARA) and Evaluation by an Area-Based Method of Ranking (EAMR) in this paper are that the SWARA method is less complex than pairwise comparison methods. In some research, authors claimed and proved that the EAMR method has more reliability than other decision matrix methods. In this research, besides applying MADM methods, an uncertain environment is assumed. Approaches to dealing with uncertainty such as fuzzy sets have been used whenever linguistic variables are more appropriate than numerical ones. This kind of variables helps decision-makers (DMs) to specify their preferences easily.
For supporting DMs to make better decisions, using a suitable research method is vital. Fuzzy sets represent one of those tools. Although banking performance is popular and many related research papers have been published, there are very few research frameworks on using hybrid methods in an uncertain environment by using a step-by-step method for finding factors and conducting a reliability test for confirming results. Our research study reveals how to choose the best bank based on the selected factors using a novel methodology. The contribution of this paper is using hybrid MADM methods, which are Stepwise Weight Assessment Ratio Analysis (SWARA) and Evaluation by an Area-based Method of Rating (EAMR) methods with Hesitant Fuzzy Sets (HFS). Although many papers have been published about bank performance both in certainty and uncertain environments, this paper is looking forward to presenting new hybrid MADM methods in uncertain environments with high reliability. Finally, sensitivity analysis is done for investigating the reliability of results.
The remainder of the research paper is organized as follows: Section 2 presents a literature review and points out a research gap. Section 3 describes the used MADM methods. Section 4 points out the research methodology for treating uncertainty, while the obtained results are revealed in Section 5. Finally, in Section 6 we present discussions and conclusions, limitations, and future research directions.
2. Literature review
2.1. Related work
Banking performance represents a popular subject among researchers. There are various relevant research papers on banking performance using different research methods. Karbassi Yazdi and Abdi (Citation2017) used Data Envelopment Analysis (DEA) and the REMBRANDT (Ratio Estimations in Magnitudes and/or deci-Bells to Rate Alternatives which are Non-DominaTed) method for evaluating Iranian banks. They considered 13 banks with profit, deposit, loan, staff, cost and investment factors. They claimed that each factor has a specific weight which is obtained by the REMBRANDT method. Then based on DEA and these factors, all 13 banks are ranked. It is shown which banks are efficient and how inefficient banks can be benchmarked using efficient banks to improve performance. Karbassi Yazdi et al. (Citation2020) provide a ranking of Colombian banks by hybrid MADM methods. First, performance indicators (PIs) related to Balanced Scorecard (BSC) are extracted. These PIs are net profit, ROI, debt, total costs, income, total assets, customer satisfaction, market share, the number of customers, new customers rate, response to time to customer requests, cost of R&D, number of improvement processes, the introduction of new products, staff satisfaction, motivation index, productivity of staff and staff training index. Then for ranking the Colombian banks based on these PIs the WASPAS method is used. Since the WASPAS method is a decision matrix method, it needs weights. For obtaining these weights, the SWARA method is applied. As a result, Colombian banks are prioritized.
Wanke et al. (Citation2016) evaluated ASEAN banks using a combination of the Fuzzy Analytical Hierarchy Process (FAHP), the Technique for Order of Preference by Similarity to Ideal Solution (TOPSIS) and Artificial Neural Networks (ANNs). They used the CAMELS indicators (Capital adequacy, Asset quality, Management, Earnings, Liquidity, and Sensitivity) for evaluating the considered banks. Then for ranking these banks, the TOPSIS method is applied. This method requires criterion-specific weights, which are obtained by using the FAHP. By combining ANN and TOPSIS, the model can predict results more accurately. Wanke et al. (Citation2017) demonstrated how Islamic banks could predict efficiency using TOPSIS, neural networks, and DEA. The factors considered in this research were the number of employees, provisions, personal expenses, banking products, operational results, deposits, and assets. Based on these factors, the ideal and non-ideal factors are computed by TOPSIS. The considered banks are then evaluated using DEA. Using an ANN, the results are evaluated for reliability.
Dinçer and Yüksel (Citation2019) evaluated Turkish service banks by stochastic hybrid MADM and BSC methods. They considered 12 PIs such as profitability, market share, efficiency, demand customization, experience, loyalty, consistency, participation, encouragement, training, technology and information for evaluating services. Then using the Fuzzy Analytical Network Process (FANP), a fuzzy version of TOPSIS (FTOPSIS), a fuzzy version of the MADM method VIKOR (FVIKOR), and Month Carlo simulation techniques, these banks are ranked. Beheshtinia et al. (Citation2017) evaluated 4 Iranian banks by using hybrid MADM and Balanced Score Card (BSC) methods. As PIs for evaluating these banks, they considered the return on investment, net profit ratio, debt ratio, customer satisfaction, minimum wasted time of customers, customer retention, the attraction of new customers, development of sales to the customer, customer loyalty, market share, problem-solving ability, lower cycle times of processes, using new technologies, employee productivity, staff training, knowledge sharing, research and development, employee satisfaction, job security, help during the recession, consideration of stakeholder benefit, enhancement of brand value, environmental care, safer and healthier workplace, and reduction of energy consumption. Then by Modified Digital Logic (MDL), FTOPSIS, FVIKOR and AHP the banks were prioritized. Dinçer and Yüksel (Citation2018) showed how to apply BSC for evaluating Turkish banks based on hybrid MADM and content analysis methods. The parameters of the BSC model for evaluating these banks were profitability, market share, efficiency, demand customization, experience, loyalty, consistency, participation, encouragement, information, training, and technology. Then FAHP, FANP and FVIKOR are used for ranking the banks based on the BSC indicators.
Zhao et al. (Citation2019) demonstrated how to improve the financial services of Chinese banks by hybrid MADM methods. Customer-centricity, service efficiency enhancement, reduced credit risks, development of finance scenarios, improved communication channels with customers, active identification of customer needs, provision of customized services, alliance with FinTech, alliance with offline merchants, alliance with financial peers, multi-faceted business strategies, professional business strategies, organizational structure reform, employee training and learning, organizational innovation culture, development of big data analysis, development of intelligent investment management, blockchain application, new payment methods, and digital marketing enhancements are the considered factors. For ranking the considered banks for using ANP, first the Decision Making Trial and Evaluation Laboratory (DEMATEL) method was applied to show relationships among the criteria. A modified VIKOR method is used for ranking the banks, which used weights obtained by the ANP. The result of the modified VIKOR shows the ranks of banks.
Dahooie et al. (Citation2018) used the methods SWARA (Step-Wise Weight Assessment Ratio Analysis) and ARAS (Additive Ratio Assessment) for the evaluation corporate finance performance of banks in Iran. Wanke et al. (Citation2018) showed how fuzzy TOPSIS and regression could be used to find the efficiency of banking in the BRICS countries.
Ghosh and Saima (Citation2021) studied how the bank of Bangladesh analysed resilience during the COVID-19 pandemic based on MCDM methods. They considered 18 banks and evaluated these banks based on several factors. The result of the research showed that these banks could be categorized into three groups based on resilience regarding COVID-19.
Table shows the summary of related work.
Table 1. A brief survey of most recent related literature.
2.2. Research gap
In the following, we will highlight the research gap. Our study aims to find factors and then prioritize Iranian banks. As this is one of the most popular topics, many related research studies have been published. However, the main research gap of our research compared with similar studies is the new hybrid MADM approach based on SWARA and EAMR in an uncertain environment. Previous papers focused on some methods such as DEA, REMBRANT, WASPAS and SWARA, FTOPSIS, FVIKOR, FAHP and other MCDM methods. The combination of these methods with HFS leads to highly reliable results. Besides, by using the Delphi method for screening factors, decision-makers ensure that the most relevant factors are gathered and customized.
Another contribution in this research is that we do not just use well-known models such as BSC, CAMEL and considered comprehensive factors for evaluating banks as used in previous papers. Using hybrid MADM methods under uncertainty, we attempt to ensure that the results are accurate and reliable.
3. Methods
In this section, we first describe two MADM methods used to consider multi-criteria selection problems and then an approach to dealing with uncertainty in the issues considered.
3.1. Step-wise Weight Assessment Ratio Analysis (SWARA) method
In methods based on pairwise comparisons, there are drawbacks, especially when the number of alternatives or criteria are relatively high: The number of pairwise comparisons for finding the weights is high and the resulting inconsistency rates are usually not acceptable. Although some methods such as BWM eliminate this drawback, these methods are rather complicated. The Step-wise Weight Assessment Ratio Analysis (SWARA) method is one of the MADM methods for calculating weights for criteria. This method needs fewer computations compared to similar methods (Karbassi Yazdi et al., Citation2020; Stanujkic et al., Citation2015).
An expert specifies priorities for all items in this method. Based on the priorities, items are sorted in descending order (Keršuliene et al., Citation2010). The following steps are conducted by the method:
Step 1. Sort items from the highest importance to lowest importance
Step 2. Then, allocate preferences to the second criterion and continue with this for all criteria. Comparative importance average values denoted as are the ratios of this comparison.
Step 3. The coefficient is computed by Equation (1)
(1)
(1)
Step 4. An importance indicator is calculated by Equation (2)
(2)
(2)
Step 5. The final weights are calculated by where
is weight of criterion j.
3.2. Evaluation by an Area-Based Method of Ranking (EAMR) method
EAMR is one of the MADM methods based on a decision matrix. Keshavarz Ghorabaee et al. (Citation2016) have introduced this model as EAMRT-2F. Karbassi Yazdi et al. (Citation2018) used the method with crisp data. This problem is based on beneficial and non-beneficial criteria. The methodological approach for EAMR is described as follows.
Step 1. The decision matrix is created as follows
(3)
(3) k is the number of decision-makers, d is the index of a decision maker, and
are the criterion scores of alternative i for criterion j of a decision maker. n is the number of alternatives, and m the number of criteria.
Step 2. For calculating the average of the decision matrix, Equations (4) and (5) are used:
(4)
(4)
(5)
(5) where
is the mean value of performance (criterion value) of alternative i and criterion j and
is the mean decision matrix, with
.
Step 3. The weighting matrix (weighting vector) is specified as follow
(6)
(6) In this equation, p is the index of the pth decision maker and the respective weight of criterion
is specified as j,
.
Step 4. The mean weighting matrix (weighting vector) is computed as follows
(7)
(7)
(8)
(8)
Step 5. The normalized average decision matrix from , denoted as nij is computed as follows:
(9)
(9)
(10)
(10)
(11)
(11) where 1
.
Step 6. The normalized weights of the decision matrix are as follows:
(12)
(12)
(13)
(13)
Step 7. The normalized scores for beneficial criteria () and non-beneficial criteria (
) are calculated as follows:
(14)
(14)
(15)
(15) In this research,
and
shows normalized weighted values for beneficial and non-beneficial criteria, respectively.
Step 8. The rank of value (RV) is calculated by summation of the scores and
(
). DMs have ranked alternatives based on normalized weights for both beneficial and cost criteria.
Step 9. The appraisal score () based on the rank values is computed as follows:
(16)
(16) where
shows the alternative which has the highest score.
EAMR is one of the decision matrix methods (Hanne, Citation2012), with few papers being published about it. One of the most significant merits of using this method is its high reliability and robustness compared with other similar methods as shown in some papers (Karbassi Yazdi et al., Citation2018; Karbassi Yazdi et al., Citation2020; Keshavarz Ghorabaee et al., Citation2016). Other possible MADM methods based on pairwise comparison such as AHP, ANP, or MACBETH easily lead to high inconsistencies of results. Based on Olson (Citation2008) the inconsistency rate will be too high when the number of criteria or alternatives is more than 9. As the number of criteria in our study is 15, we cannot use a pairwise comparison method.
3.3. Hesitant fuzzy sets
Over the past decades, fuzzy sets were suggested initially and further popularized by Zadeh (Citation1965). Fuzzy sets are used in situations of incomplete knowledge. In particular, fuzzy sets are based on the idea that it is not fully clear whether an element belongs to a set or not. Thus, such features are characterized by partial membership of a set. A membership function indicates gradual values between non-membership and complete membership of a set (Liao et al., Citation2015; Rodríguez et al., Citation2016; Wei et al., Citation2017). An intuitionistic fuzzy set (IFS) represents a generalization of fuzzy sets. It incorporates the degree of hesitation called hesitation margin, defined as 1 minus the sum of membership and non-membership degrees, respectively (Ejegwa et al., Citation2014). An intuitionistic fuzzy set is based on two values: a degree of membership and a degree of non-membership. The intuitionistic fuzzy set was introduced by Atanassov (Citation1986) and further detailed e.g. in Atanassov (Citation2012), and is used currently mainly for solving multiple criteria decision-making problems (MCDM) and group decision-making problems (Dymova & Sevastjanov, Citation2011). Various research studies have been published about using MADM methods and HFS in diverse industries. For instance, Narayanamoorthy et al. (Citation2019) pointed out how a robot companies can be selected using intuitionistic hesitant fuzzy sets together with VIKOR and fuzzy entropy. Since HFSV (Hesitant Fuzzy Set Vikor) method needs weights for ranking robot companies, fuzzy entropy is used for determining them. Mishra et al. (Citation2019) used HFSWASPAS (Hesitant Fuzzy Set Weighted Aggregated Sum Product ASsessment) for selecting a green supplier. They applied the entropy method for finding weights and then based on these weights HFSWASPAS is used for ranking green suppliers. Sałabun et al. (Citation2018) applied HFSCOMET (Hesitant Fuzzy Sets Characteristic Objects Method) for selecting electric buses. The result demonstrated that DMs could make a decision more reliably when faced with an uncertain environment. Different researchers related to fuzzy sets suggested various terminology, explanations, and delineations, some of them are as follows:
Definition 3.1:
Let us consider X a nonempty set (reference set) For this set, a membership function : X [0,1] is defined for a regular fuzzy set. In addition, we consider another function
: X
[0,1] interpreted as a non-membership function.
(17)
(17)
(18)
(18)
Definition 3.2:
Consider X as a reference set, a hesitant fuzzy set of X is defined by a function h which maps each element of X to a subset of [0,1]. We usually assume that h(x) is a finite set.
Definition 3.3:
If X is a reference set, the specific hesitant fuzzy set can be defined as follows:
(19)
(19)
(20)
(20)
(21)
(21)
(22)
(22)
and
points out empty and full sets which are neither nonsense nor complete ignorance.
Based on these equations, DMs can evaluate alternatives and allocate their preferences to them. M is used for showing preferences or options and indicates the set of opinion experts (Torra & Narukawa, Citation2009).
In MADM methods, DMs frequently specify their preferences by scores. These scores are numbers that can be used whenever the environment is stable. As mentioned above, if the environment is changeable or uncertain, DMs cannot specify their preferences by exact number. Therefore, fuzzy numbers with linguistics variables as a useful means to support DMs in specifying preferences. In this paper, we apply the HFS type of fuzzy numbers.
4. Research methodology
4.1. Procedure of research
The research procedure conducted for this study is as follows:
Step 1: Identify factors from experts and previous research
Step 2: Customize these factors by the Delphi method (i.e. select only the relevant ones)
Step 3: Find weights for the relevant factors by using the SWARA method
Step 4: Rank the considered governmental banks by the EAMR method
The research questions of this research are as follows:
RQ1: What factors are relevant for evaluating bank performance, especially during the COVID-19 pandemic?
RQ2: Which banks have the best performance among the considered banks?
The following Figure shows the research methodology of this paper based on the main phases.
4.2. Customized factors and DMs attributes
There are 17 factors selected for evaluating the banks. Table points out these factors which are selected from previous research studies.
Table 2. Survey of experts.
The Delphi method is a method for the prediction or customizing of factors. First, all possible factors are listed. Then a questionnaire based on these factors and a Likert scale for evaluation is designed. The Likert scale can comprise 5, 7 or 9 points. We considered a 5-point Likert scale as sufficient for our study.
The number of experts that should be answering the Delphi questionnaires is debatable. Some researchers believe that more than 100 people should be answered while others believe that 10–15 experts are sufficient (Dunham, Citation1998; Linstone & Turoff, Citation1975; Powell, Citation2003). This number of experts involved in our study is ten, which are considered for answering the Delphi questionnaires. Table shows information about these people. These factors to be evaluated are indicating the performance of the banks. As the study is conducted during the COVID-19, this context is considered regarding the impact of factors on bank performance. Table shows further details of the DMs.
Then the Delphi method is applied for customizing the factors. If the average scores of DMs is equal to 4 or higher, this factor is accepted for further consideration in our study. If the average score is less than 4 it is rejected and not considered for next step. The result shows that among these 17 factors, the size of the bank and technical factors are eliminated. Table reveals the results for the factors.
Table 3. Experts’ opinions.
The results show that among 17 factors, two factors (size of the bank and technical factors) are eliminated, and 15 factors are accepted.
Then based on these factors, seven governmental banks of Iran are evaluated using EAMR. A respective questionnaire is designed using a 9-point Likert scale. Again, the above mentioned 10 experts are selected to answer these questions. For the calculations of SWARA and EAMR, EXCEL V.2017 are applied.
5. Results and Sensitivity analysis
5.1. Results
First of all, weights are obtained by the SWARA method. Table demonstrates the calculation of this method. As the EAMR method assumes given weights, the SWARA method is applied to finding these weights. First, according to Equation (1), is obtained. Then based on Equation (2),
is extracted. Finally, the weights are obtained according to the final equation.
Table 4. Results of the SWARA method.
In the second step, the decision matrix is created, as shown in Table . This table shows the preferences of experts according to a 9-point Likert scale. The legend suggests that 1 is the least important, and 9 is the highest importance.
Table 5. Hesitant fuzzy numbers.
In Table , the first decision matrix based on crisp data is created according to Equation (3). This data is based on DMs preferences regarding each company according to the selected factors.
Table 6. Decision matrix of the EAMR method.
These scores are DMs’ preferences that are allocated based on a 9-point Likert scale.
Then these data are transferred to hesitant fuzzy data based on Table . The results of the transformation according to Equations (9)–(11) are shown in Table .
Table 7. Decision-making matrix of the EAMR method based on HFS data.
Table points out the decision matrix of EAMR according to HFS data.
In Table , the scores are calculated as averages according to Equations (4)–(5).
Table 8. Average matrix of the EAMR method.
Then normalized the decision matrix is created in step three (see Table ). This work leads to dimensionless data, and all data will be homogenous (Equations (9)–(11)).
Table 9. The normalized average decision matrix.
In this step first, the maximum number of each row is determined. Then, each entry is divided on the maximum number of the respective row. Step four reveals the weighted data in the decision matrix. This work is done to obtain a dimensionless and standardized score. Table shows the weighted matrix (Equations (12)–(13)).
Table 10. Weighted matrix of the EAMR method.
In step five, the normalized score of both beneficial and non-beneficial criteria is included as shown in Table (Equation (14)).
Table 11. Normalized scores of the EAMR method.
Benefit and cost criteria are obtained from the dimensionless scores according to related formula.
The rank values (RV) are calculated in step six, as shown in Table (Equation (15)).
Table 12. Rank values of the EAMR method.
Table shows the rank value for both benefit and cost criteria.
Step seven leads to the final score () and rankings as shown in Table (Equation (16)).
Table 13. Appraisal score of EAMR method.
In this table, based on the appraisal score, the companies are ranked.
5.2. Sensitivity analysis
In this section, the results of our study are compared with other MADM methods based on a decision matrix and using the same data. It is not unusual that different methods lead to different results due to various mathematical models and assumptions. Therefore, decision-makers prefer to understand which methods lead to similar results. Although some research reveals that the EAMR method has better accuracy than other methods (Keshavarz Ghorabaee et al., Citation2016), the sensitivity analysis is useful to confirm this assumption (Karbassi Yazdi et al., Citation2018). For sensitivity analysis, results of the methods TOPSIS, VIKOR, and WASPAS are compared with the results of this research using the Spearman coefficient. The Spearman coefficient shows the similarity of the two scores. The ranking results of the considered methods are shown in Table .
Table 14. Sensitivity analysis.
In the above table, the rank of each method with the original method (EAMR) is compared by the Spearman coefficient, and when the sig of result is less than 0.05, there is a statistically significant relationship between these two methods. As all sig values are above 0.05, there is no significant relationship between these methods and the original method (Karbassi Yazdi et al., Citation2018). The results indicated that the ranking of EAMR is different from other methods. As mentioned before, the ranking of the EAMR method can be considered more accurate than other methods (Karbassi Yazdi et al., Citation2018; Keshavarz Ghorabaee et al., Citation2016).
6. Discussion and conclusions
Unlike the case of the global financial crisis of 2008, the banking system is not the source of the global economic crisis since 2020. In this case, the crisis triggered by the COVID-19 pandemic which has generated an unprecedented health, economic and social crisis for the current generation and implicitly affected the dynamics of the banking system. The world economy has been severely affected by the COVID-19 pandemic, so it has contracted, while the banking system has been shaken by the impact of restrictive measures imposed by government authorities although strong countermeasures were taken in various countries. The impact of the COVID-19 pandemic on Iran's banking system must be understood in connection with the particularly difficult political moment that overlapped with domestic economic and financial pressure, influenced by US economic sanctions (Iranian authorities have been under pressure since January 2020 due to strained relations with the United States). Such sanctions against the Iranian banking system have also led to a range of restrictions and limitations in other areas such as trade, insurance, and business, which in turn affected the access to medical and health services. In other words, since the beginning of the COVID-19 pandemic, the Iranian government has been facing insufficient economic resources to combat the spread of the virus and its severe implications. Consequently, the selection of Iran for this case study was based on a highly disrupted financial and economic environment due to COVID-19 pandemic so that the empirical results reach an increased utility in the implementation of possible measures and sustainable strategies regarding the banking system in Iran.
The banking environment in Iran has witnessed significant developments in the last decade, although it is still a relatively young industry. Currently, the banking sector transformation is focused on increasing profitability, effectiveness, and competitiveness. Thus, evaluation and performance measurement has become increasingly important. Our empirical research study provides valuable guidance to better understand performance measurement in the banking sector in Iran. Factors differ between various sectors of activity and are not constant as they change over time. The empirical results suggest certain conclusions about factors of performance measurement of the banking sector in Iran.
The aim of this paper is first to find customized factors for evaluation performance banks. We find very little data in the existing literature on this particular research topic. However, we selected 17 factors based on existing literature and interviews with experts to provide a detailed insight into the Iranian banking system. The Delphi method applied to the considered 17 factors rejects two factors, staff satisfaction and the motivation index.
The second aim of this research is prioritizing banks according to the selected 15 factors based on hybrid MADM methods in an uncertain environment. In the first step, relevant factors of seven governmental banks of Iran are assessed. EAMR is then used as a MADM method for prioritizing the considered banks. EAMR is a method based on a decision matrix and needs weights for the considered criteria. For obtaining these weights, the SWARA method is implemented.
As a result of ranking these factors based on SWARA, profit appears as the most important factor. It means that this factor has a strong effect on the performance measurement of public banks in Iran. The rate of new customers has the lowest priority, which means that the absorbing resources of money and loyalty of customers is more important than attracting new customers. The evaluation results of the considered banks reveal that the oldest bank with high profit has the highest rank. It means that the most senior bank can create the highest profit and is more resilient regarding issues such as COVID-19. The highest profit helps them create new ways to provide services during the pandemic, keep their staff, can extend deadlines of loan instalment, and prevent bankruptcy. The prioritizing of the considered factors indicates which bank has a suitable performance and which one must focus on improving its performance.
The comparison of results of the EAMR method with those of other MADM methods such as WASPAS, TOPSIS and VIKOR confirms the claim that EAMR appears more accurate and shows no significant relationship with the results of the other methods.
A limitation of this research is the access to information because there is a very competitive environment among Iranian banks. They think publishing this information leads to possible damage to their reputation. Although we committed to not revealing their names, access to information was hard. Further banks could be included in the evaluation study for future research if access to respective information becomes possible. This would lead to a more comprehensive comparison of banks. Besides, researchers may use D-numbers, Z-numbers, Pythagorean numbers, or fuzzy picture numbers instead of HFS. Also, this research focused on the performance of Iranian banks during the COVID-19 pandemic. For future research studies, we will investigate the impact of the COVID-19 pandemic on the banking system in other countries or regions. In connection with that, it would also be useful to compare the Islamic banking system with traditional banking. Moreover, a comparative analysis is indicated between the effects of several global financial crises in the recent past and the effect generated by the COVID-19 pandemic on selected economies.
Disclosure statement
No potential conflict of interest was reported by the author(s).
Data availability statement
The authors confirm that the data supporting the findings of this study are available within the article. Due to confidentiality agreements, further details on the collected data such as the names of the involved institutions are not available.
References
- Atanassov, K. T. (1986). Intuitionistic fuzzy sets. Fuzzy Sets and Systems, 20(1), 87–96. https://doi.org/10.1016/S0165-0114(86)80034-3
- Atanassov, K. T. (2012). On intuitionistic fuzzy sets theory (Vol. 283). Springer.
- Batool, M., Ghulam, H., Hayat, M. A., Naeem, M. Z., Ejaz, A., Imran, Z. A., Spulbar, C., Birau, R., & Gorun, T. H. (2021). How COVID-19 has shaken the sharing economy? An analysis using Google trends data. Economic Research-EkonomskaIstraživanja, 34(1), 2374–2386. https://doi.org/10.1080/1331677X.2020.1863830.
- Beheshtinia, M. A., & Omidi, S. (2017). A hybrid MCDM approach for performance evaluation in the banking industry. Kybernetes, 46(8), 1386–1407. https://doi.org/10.1108/K-03-2017-0105
- Dahooie, J. H., Zavadskas, E. K., Abolhasani, M., Vanaki, A., & Turskis, Z. (2018). A novel approach for evaluation of projects using an interval–valued fuzzy additive ratio assessment (ARAS) method: A case study of oil and gas well drilling projects. Symmetry, 10(2), 45. https://doi.org/10.3390/sym10020045
- Demirguc-Kunt, A., Pedraza, A., & Ruiz-Ortega, C. (2020). Banking sector performance during the COVID-19 crisis. Policy Research Working Paper; No. 9363. Washington, DC: World Bank. © World Bank. License: CC BY 3.0 IGO. https://openknowledge.worldbank.org/handle/10986/34369
- Dinçer, H., & Yüksel, S. (2018). Comparative evaluation of BSC-based new service development competencies in Turkish banking sector with the integrated fuzzy hybrid MCDM using content analysis. International Journal of Fuzzy Systems, 20(8), 2497–2516. https://doi.org/10.1007/s40815-018-0519-y
- Dinçer, H., & Yüksel, S. (2019). An integrated stochastic fuzzy MCDM approach to the balanced scorecard-based service evaluation. Mathematics and Computers in Simulation, 166, 93–112. https://doi.org/10.1016/j.matcom.2019.04.008
- Dunham, R. B. (1998). The DELPHI technique. Organizational Behavior, 50(2).
- Dymova, L., & Sevastjanov, P. (2011). Operations on intuitionistic fuzzy values in multiple criteria decision making. Scientific Research of the Institute of Mathematics and Computer Science, 1(10), 41–48. http://amcm.pcz.pl/get.php?article=2011_1/art_05.pdf
- Ejegwa, P. A., Akowe, S. O., Otene, P. M., & Ikyule, J. M. (2014). An overview on intuitionistic fuzzy sets. International Journal of Scientific & Technology Research, 3(3), 142–145. https://www.ijstr.org/final-print/mar2014/An-Overview-On-Intuitionistic-Fuzzy-Sets.pdf
- Ghosh, R., & Saima, F. N. (2021). Resilience of commercial banks of Bangladesh to the shocks caused by COVID-19 pandemic: An application of MCDM-based approaches. Asian Journal of Accounting Research, 6(3), 281–295. https://doi.org/10.1108/AJAR-10-2020-0102
- Hanne, T. (2012). Intelligent strategies for meta multiple criteria decision making (Vol. 33). Springer Science & Business Media.
- Karbassi Yazdi, A., & Abdi, F. (2017). Designing robust model for banks benchmarking based on Rembrandt method and DEA. Benchmarking: An International Journal, 24(2), 431–444. https://doi.org/10.1108/BIJ-01-2015-0001
- Karbassi Yazdi, A., Hanne, T., & Osorio Gómez, J. C. (2020). Evaluating the performance of Colombian banks by hybrid multicriteria decision making methods. Journal of Business Economics and Management, 21(6), 1707–1730. https://doi.org/10.3846/jbem.2020.11758
- Karbassi Yazdi, A., Hanne, T., Osorio Gómez, J. C., & García Alcaraz, J. L. (2018). Finding the best third-party logistics in the automobile industry: A hybrid approach. Mathematical Problems in Engineering. Article ID 5251261. https://doi.org/10.1155/2018/5251261
- Keršuliene, V., Zavadskas, E. K., & Turskis, Z. (2010). Selection of rational dispute resolution method by applying new step-wise weight assessment ratio analysis (SWARA). Journal of Business Economics and Management, 11(2), 243–258. https://doi.org/10.3846/jbem.2010.12
- Keshavarz Ghorabaee, M., Zavadskas, E. K., Amiri, M., & Antucheviciene, J. (2016). A new method of assessment based on fuzzy ranking and aggregated weights (AFRAW) for MCDM problems under type-2 fuzzy environment. Economic Computation and Economic Cybernetics Studies and Research, 50(1), 39–68. https://ssrn.com/abstract=2939724
- Liao, H., Xu, Z., Zeng, X. J., & Merigó, J. M. (2015). Qualitative decision making with correlation coefficients of hesitant fuzzy linguistic term sets. Knowledge-Based Systems, 76, 127–138. https://doi.org/10.1016/j.knosys.2014.12.009
- Linstone, H. A., & Turoff, M. (1975). The Delphi method: Techniques and applications (Vol. 29). Addison-Wesley.
- Mehdiabadi, A., Tabatabeinasab, M., Spulbar, C., Karbassi Yazdi, A., & Birau, R. (2020). Are we ready for the challenge of banks 4.0? Designing a roadmap for banking systems in industry 4.0. International Journal of Financial Studies, 8(2), 1–28. https://doi.org/10.3390/ijfs8020032
- Mirzaei, A., Saad, M., & Emrouznejad, A. (2021). Bank stock performance during the COVID-19 crisis: Does efficiency explain why Islamic banks fared relatively better? https://ssrn.com/abstract=3702116 or https://doi.org/10.2139/ssrn.3702116
- Mishra, A. R., Rani, P., Pardasani, K. R., & Mardani, A. (2019). A novel hesitant fuzzy WASPAS method for assessment of green supplier problem based on exponential information measures. Journal of Cleaner Production, 238, 117901. https://doi.org/10.1016/j.jclepro.2019.117901
- Narayanamoorthy, S., Geetha, S., Rakkiyappan, R., & Joo, Y. H. (2019). Interval-valued intuitionistic hesitant fuzzy entropy based VIKOR method for industrial robots selection. Expert Systems with Applications, 121, 28–37. https://doi.org/10.1016/j.eswa.2018.12.015
- Olson, D. L. (2008). Multi-criteria decision support. In F. Burstein & C. W. Holsapple (Eds.), Handbook on decision support systems (Vol. 1, pp. 299–314). Springer.
- Powell, C. (2003). The Delphi technique: Myths and realities. Journal of Advanced Nursing, 41(4), 376–382. https://doi.org/10.1046/j.1365-2648.2003.02537.x
- Rodríguez, R. M., Bedregal, B., Bustince, H., Dong, Y. C., Farhadinia, B., Kahraman, C., Martínez, L., Torra, V., Xu, Y.J., Xu, Z.S., & Herrera, F. (2016). A position and perspective analysis of hesitant fuzzy sets on information fusion in decision making. Towards high quality progress. Information Fusion, 29, 89–97. https://doi.org/10.1016/j.inffus.2015.11.004
- Sałabun, W., Karczmarczyk, A., & Wątróbski, J. (2018). Decision-making using the hesitant fuzzy sets COMET method: An empirical study of the electric city buses selection. In 2018 IEEE Symposium Series on Computational Intelligence (SSCI) (pp. 1485–1492).
- Shahabi, V., Azar, A., Razi, F. F., & Shams, M. F. F. (2020). Simulation of the effect of COVID-19 outbreak on the development of branchless banking in Iran: Case study of Resalat Qard–al-Hasan Bank. Review of Behavioral Finance, 13(1), 85–108. https://doi.org/10.1108/RBF-06-2020-0123
- Sija, A. (2021). The key factors influencing bank employees’ performance during pandemic COVID-19: An investigation on selected banks in Malaysia. European Journal of Human Resource Management Studies, 5(1), https://oapub.org/soc/index.php/EJHRMS/article/view/1010 https://doi.org/10.46827/ejhrms.v5i1.1010
- Stanujkic, D., Karabasevic, D., & Zavadskas, E. K. (2015). A framework for the selection of a packaging design based on the SWARA method. Inzinerine Ekonomika-Engineering Economics, 26(2), 181–187. https://doi.org/10.5755/j01.ee.26.2.8820
- Torra, V., & Narukawa, Y. (2009). On hesitant fuzzy sets and decision. In 2009 IEEE International Conference on Fuzzy Systems (pp. 1378–1382).
- Wanke, P., Azad, A. K., & Emrouznejad, A. (2018). Efficiency in BRICS banking under data vagueness: A two-stage fuzzy approach. Global Finance Journal, 35, 58–71. https://doi.org/10.1016/j.gfj.2017.05.001
- Wanke, P., Hassan, M. K., & Gavião, L. O. (2017). Islamic banking and performance in the ASEAN banking industry: A TOPSIS approach with probabilistic weights. International Journal of Business & Society, 18, 129–150. http://www.ijbs.unimas.my/images/repository/pdf/Vol18-S1-paper8.pdf
- Wanke, P., Kalam Azad, M. A., Barros, C. P., & Hadi-Vencheh, A. (2016). Predicting performance in ASEAN banks: An integrated fuzzy MCDM–neural network approach. Expert Systems, 33(3), 213–229. https://doi.org/10.1111/exsy.12144
- Wei, G., Alsaadi, F. E., Hayat, T., & Alsaedi, A. (2017). A linear assignment method for multiple criteria decision analysis with hesitant fuzzy sets based on fuzzy measure. International Journal of Fuzzy Systems, 19(3), 607–614. https://doi.org/10.1007/s40815-016-0177-x
- Zadeh, L. A. (1965). Fuzzy sets. Information and Control, 8(3), 338–353. https://doi.org/10.1016/S0019-9958(65)90241-X
- Zhao, Q., Tsai, P.-H., & Wang, J.-L. (2019). Improving financial service innovation strategies for enhancing China’s banking industry competitive advantage during the fintech revolution: A hybrid MCDM model. Sustainability, 11(5), 1419. https://doi.org/10.3390/su11051419