Abstract
Background and objective: University students report more health complaints and a poorer quality of life than their working peers. The majority of these students do not seek contact with health professionals. Health problems result not only from risk behaviors (e.g. drugs use) but also from various stress-related factors, necessitating the investigation of several factors of students’ lives. The Internet provides a suitable environment for developing an accessible screening and self-regulation instrument for student health promotion. Design and methods: A web questionnaire was created that included reliable measurement scales (e.g. health, health (risk) behavior, quality of life, satisfaction with study), calculation modules with cut-off points and personalized feedback. The instrument included traffic lights, personal scores for comparison with peer scores, advice and referral. The validity, reliability, feasibility and effectiveness of the instrument were demonstrated using three studies (n = 334; n = 267; n = 4009). Results: The hypothesized research model, including the direct and indirect effects of the self-regulation of health via quality of life and health (risk) behaviors was an appropriate fit to the data (Structural Equation Modeling), explaining a substantial amount of variance (67%) and thus demonstrating predictive validity. Students reported intentions to change their behavior, actual behavioral changes and quality of life improvements up to a year after initially participating in a study. Conclusions: This instrument: (1) detects health complaints at an individual level and group level (e.g. study program); (2) encourages students to change their health (risk) behavior via personalized feedback; (3) provides students with a fairly complete and elaborate overview of their health-related quality of life and (4) is the first instrument providing a very high level of tailoring. Self-regulation (both self-assessment and personalized feedback) can fulfill an important role in addressing health problems and risks at an early stage and without the immediate need for professional intervention.
1. Introduction
University students report more physical and mental health complaints, lower health status and a poorer quality of life than their working peers (Boot, Donders, Vonk, & Meijman, Citation2009; Nauta, Meijman, & Meijman, Citation1996; Schmidt & Simons, Citation2013; Stewart-Brown et al., Citation2000; Vaez, Kristenson, & Laflamme, Citation2004). Frequent complaints include shoulder, neck and back problems, fatigue, migraine, depression and stress (Nauta et al., Citation1996; Schmidt & Simons, Citation2013). To date, research results have indicated that these health complaints correlate with students’ problem perceptions of their study situation, health behavior and student life (Boot, Meijman, & Vonk, Citation2010; Nauta et al., Citation1996). In addition, we know from earlier studies that the majority of these students have no contact with health professionals, risking an unnecessary deterioration of their health. (Boot, Vonk, & Meijman, Citation2007; Eisenberg, Golberstein, & Gollust, Citation2007; Raviv, Sills, Raviv, & Wilansky, Citation2000; Timlin-Scalera, Ponterotto, Blumberg, & Jackson, Citation2003; Verouden, Vonk, & Meijman, Citation2010).
Students with health problems are more likely to underachieve academically and experience study delay. These students risk becoming trapped in a vicious cycle between health complaints and poorer academic performance with attrition as a possible outcome (Boot et al., Citation2007, Citation2009; El Ansari & Stock, Citation2010; Nauta et al., Citation1996). Universities in the Netherlands have introduced measures to require students to leave if they fall too far behind in their studies. Needless to say, it is not easy getting accepted again by another good university. In addition to preventing unnecessary student suffering, health funds can be spared if problems are detected at an early stage and targeted help is offered in a timely manner. The number of students enrolled in higher education has been steadily increasing in the Netherlands, with the proportion in higher education doubling from roughly 20% of the population in the 18–25-years-old age group in the 1980s, to 40% in 2010 (Ministerie van Onderwijs, Cultuur, & Wetenschap, Citationn.d.).
National and international studies report increased and persistent student stress levels and/or elevated levels of medication use (De Hoog, & Schilp, Citation2012; Kitzrow, Citation2003; Schmidt & Simons, Citation2013; Schwartz, Citation2006), as well as increased alcohol and drug use (CASA, Citation2007; Karam, Kypri, & Salamoun, Citation2007). The significant stress factors that have been identified are: increased levels of study-related stress, students’ financial situations, family problems, the problems ensuing from combining study and work, and those related to making the transition from home to university (Calvete & Connor-Smith, Citation2006; Cassidy, Citation2004; De Hoog, & Schilp, Citation2012; Hall, Chipperfield, Perry, Ruthig, & Goetz, Citation2006; Hurst, Baranik, & Daniel, Citation2013; Jessop, Herberts, & Solomon, Citation2005; Orr, Gwosc, & Netz, Citation2011; Robotham, Citation2008; Robotham & Julian, Citation2006; Schmidt & Simons, Citation2013).
Stress may also be the result of ‘abrupt changes in many aspects of students’ lives in a relatively short period of time’ (Verouden et al., Citation2010, p. 310). Additionally, in the Netherlands, students experience a lot of pressure to perform at all levels (i.e. study, socially, career) (Philipsen, Citation2014; Recourt, Citation2012; Schmidt & Simons, Citation2013), prioritizing therefore becomes an absolute necessity. Similarly, the research conducted among American freshmen reports rising levels of the drive to achieve and put on a ‘perfect face’ (possibly made worse by the influence of social media) combined with decreasing levels of emotional well-being (Lewin, Citation2011).
1.1. Self-regulation in different areas of student life
Self-regulation is a process which is employed by individuals to attain certain (life) goals, despite experiencing different life events, adversity and stressors; it includes controlling cognitive, emotional and behavioral processes (John & Gross, Citation2004; Leventhal, Leventhal, & Contrada, Citation1998; Maes & Karoly, Citation2005; Schunk, Citation2001; Zimmerman, Citation2000). To achieve the final goal, both collectivistic and individualistic (coping) strategies can be deployed, such as seeking help, engaging in positive and constructive problem-solving, and avoiding escapism (such as alcohol and drug (ab)use). Self-regulation can be practiced in various areas of life, such as health, study, work and relationships, following the three basic human needs of autonomy, relatedness and competence (Deci & Ryan, Citation2000). The clearer a vision of life and health goals a person has, the more he or she will deploy strategies to achieve them (e.g. monitoring goal progress, help-seeking, adopting a healthy lifestyle, etc.) (Visser & Hirsch, Citation2014). Earlier studies reported on the association of personal goal regulation with well-being (Salmelo-Aro, Citation2010) and on the relationship between quality of life and health in student populations (Vaez et al., Citation2004; Vaez, Voss, & LaFlamme, Citation2010). Our hypothesis is that self-regulation in students has a positive effect on health, by using problem-solving behavior, coping strategies (including avoiding health (risk) behavior) and improving quality of life ().
Figure 1. Structural paths and standardized regression coefficients (MLE) and explained variance for the 'self-regulation for the promotion of student health' model. The control variables are not shown for reasons of simplicity.
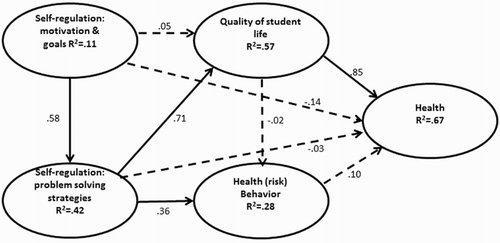
Leventhal et al. (Citation1998)’s perceptual-cognitive model of self-regulation describes the behavior or coping methods (to manage health threats) which result from appraisal at both a personal level (illness representations, representations of health threats and representations of coping resources) and a contextual level (social information and social comparison). Usually, health is not a primary goal for students, but it is still important because other goals (studying, working, having fun, etc.) are difficult to achieve when one is suffering health complaints. Different aspects of illness representations (i.e. illness identity, perceived controllability) have shown to be related to coping strategies, perceived well-being and vitality, among other factors (Hagger & Orbell, Citation2003). Furthermore, coping behavior can be influenced by emotions, such as fear, resulting from certain appraisals (Leventhal et al., Citation1998; McAndrew et al., Citation2008).
Not seeking help can be explained to a certain extent by the ambiguity of the symptoms (e.g. fatigue, headache) which makes them easily attributable to stress (Cameron, Leventhal, & Leventhal, Citation1995). Stress is also perceived by students to be a constant and inevitable condition of student life (Verouden et al., Citation2010). Symptoms of illness can also be regarded as a threat to identity formation and goal setting, which blocks the help-seeking process (Farmer, Farrand, & O'Mahen, Citation2012; Verouden et al., Citation2010). When certain illness symptoms (e.g. depression) and help-seeking are stigmatized by peers, this may inhibit students from seeking treatment (Vidourek, King, Nabors, & Merianos, Citation2014) or confiding in friends/peers.
Interventions that are designed according to self-regulation theory can help students to prevent and manage illness threats (McAndrew et al., Citation2008; Nooijer de, Veling, Ton, Vries, & Vries, Citation2008). These include the self-monitoring of illness symptoms and health (risk) behavior, receiving feedback and tips on action planning and help-seeking. Achieving better results in terms of engagement and bringing about desired behavior change are to be expected with tailoring (Morrison, Moss-Morris, Michie, & Yardly, Citation2013; Nooijer et al., Citation2008). Various success stories are presented regarding smoking, alcohol intake, healthy food consumption, physical activity and stress management (see Bewick, Trusler, Mulhern, Barkham, & Hill, Citation2008; Chiauzzi, Brevard, Thum, Decembrele, & Lord, Citation2008; Cohen, Kamarck, & Mermelstein, Citation1983; Escoffery, McCormick, & Bateman, Citation2004; Franko et al., Citation2008; Larimer & Cronce, Citation2007; Saitz et al., Citation2007), but these are all focused on a restricted area of health (risk) behavior, overlooking the bigger picture of a student's life.
The goal of this research is to develop and validate a self-regulation web-based instrument for university students by conducting three independent studies: Study (I) validates the separate measurement scales that represent the concepts of our research model; Study II validates the hypothesized research model as a whole: that self-regulation has potential to positively influence health via enhancing problem-solving and coping strategies (including avoiding health (risk) behavior) and improving quality of life; Study III develops a valid and reliable tailored self-regulation web-based instrument which provides immediate personalized feedback and asks students to evaluate its effectiveness across a range of different areas.
2. Study I: Content validity
2.1. Procedure
After the systematic literature review of the self-regulation concepts that make a valuable contribution to the health and quality of life of students, we found various valid and reliable measurement scales to measure these variables (). The scales were selected based on their content validity, internal reliability and predictive validity. If necessary, we picked the most appropriate measures for the student context or adapted certain valid and reliable measurement scales to be an exact fit for the student environment. Short scales were preferenced over long scales to promote the practicality of the instrument. Measurement scales of English origin were translated into Dutch and then translated back. If the measurement scales were not available, we developed new ones for our research purposes (Van der Heijde, Vonk, & Meijman, Citation2011). Items were generated for each scale which matched our conceptual framework and then judged by a team of health professionals. Although the profile of a student filling out the questionnaire is obviously only a snapshot in time, several questions were, nevertheless, about their behavior in the last month.
Table 1. Cronbach's alphas for the reliabilities of the included measurement scales and example items.
2.2. Participants
A questionnaire was given to the students of a Dutch university by a GP's assistant whenever they visited the Student Health Service. The questionnaires were completed in the waiting room and ‘posted’ into a cardboard ‘post box’. The data were collected in March–April 2010 and the response rate was 70% (N = 334). Twenty-seven percent of the students were male and 73% female; their mean age was 23.92 years (SD = 2.58). Twelve percent were in their first year, 48% were bachelor students, 34% were master students, 6% were final year medical students and 1% PhD students. The majority of the students (97%) considered themselves to be Dutch. Fifty-five percent reported that they were in a steady relationship and 67% had some kind of job. Only one percent of the students lived with their parents or family, 45% with peers and 54% alone or with a partner.
2.3. Analysis and results
To establish the internal consistency reliability of each scale, a Cronbach's alpha was calculated and a confirmatory factor analysis (orthogonal) was performed. Further details concerning each analysis (SPSS 20.0, IBM) are provided, per theme and measurement scale, in . The questionnaire was improved after statistical analyses and feedback from the first group of participants in Study I (rating scales, answering categories, missing themes, etc.).
The measurement scales included in the instrument covered the topics related to physical and mental health, health (risk) behavior, quality of student life (including satisfaction with study), and self-regulation (e.g. motivation and goals, problem-solving). provides an overview of the measurement scales, their psychometric qualities and sample items. The majority of variables demonstrated acceptable reliabilities, with Cronbach's alpha coefficients of .70 or higher. For the CFA we noted that the loading of an item defining a certain factor was around .40 or larger and then used this as a guideline. The amount of explained variance of the majority of the measurement scales was around 40% or higher. Results led to the elimination or reformulation of poorly loading items and some changes in answering format (for further details, see ).
3. Study II: Predictive validity
3.1. Procedure
We proceeded to investigate our hypothesis that self-regulation in students has a positive effect on health through problem-solving behavior (including avoiding health (risk) behavior) and improving quality of life. We collected data from a second group of participants to test the research model (to demonstrate both content and predictive validity) with the aforementioned valid and reliable health outcome variables (see and ).
3.2. Participants
The second group of participants comprised 267 undergraduate psychology students from a Dutch university. The data were collected in October 2010. All the students received a course credit from the Faculty of Social and Behavioral Sciences, Department of Psychology for taking a battery of digital tests during the test week. Students were debriefed on the specific research purposes and research questions used in the study. Confidentiality and anonymity were guaranteed and participants also had the opportunity to withdraw up to three weeks after the test week; no one withdrew. The student sample included 69 men (26%) and 198 women (74%). Their mean age was 20.61 years (SD = 5.21) and the group consisted largely of freshmen (92%). The majority of the students (247: 93%) had Dutch as their mother tongue, 42% reported that they were in a steady relationship, 36% had some form of employment, over half of the students (53%) reported that they lived with their parents or family, 21% with peers, 20% alone and 5% with a partner.
3.3. Analysis and results
We analyzed the self-regulation for the promotion of student health model with structural equation modeling (SEM) techniques, using the AMOS 18 software package (Arbuckle, Citation2003). We analyzed the covariance matrix using the maximum likelihood method of estimation. Besides the chi-square statistic, the analysis assessed the incremental fit index (IFI), the Tucker–Lewis index (TLI), the comparative fit index (CFI) and the root-mean-square error of approximation (RMSEA). Specifically, each control variable was included in our model as a manifest variable and allowed to correlate with all model variables. We tested whether quality of life and health (risk) behaviors were mediators in the relationship between self-regulation and health and compared a model with both direct and indirect paths (partial mediation) to a model with only indirect paths (full mediation).
Age, gender and peer pressure lifestyle self-efficacy were used as control variables in the model. The means, standard deviations, internal consistencies (Cronbach's alphas), and correlations between the study variables are displayed in . Model 1, including both direct and indirect effects had a reasonable fit to the data (). Results showed that motivation and goals were positively related to problem-solving (β = .58, p < .001; see also ) but not to quality of student life (β = .05, p = .77). Problem-solving, in turn, was a significant predictor of quality of student life (β = .71, p < .01) and of health (risk) behaviors (β = .36, p < .05). Furthermore, quality of student life was significantly related to health (β = .58, p < .01). Direct relationships between motivation and goals and health (β = −.14, p = .20) and between problem-solving and health (β = −.03, p = .92) were not significant. Similarly, health (risk) behaviors, in turn, were not significantly related to health (β = .10, p = .24). The proportion of variance in the variable health that was explained by the aforementioned variables in this model was 67%.
Table 2. Means, standard deviations, reliability coefficients (Cronbach's alphas on the diagonal), and correlations between the model variables (Sample 2; N = 267).
Table 3. Goodness-of-fit indices for proposed models.
After evaluating the proposed model, a subsequent model (2) was fit, with non-significant paths trimmed, including the direct ones (). Constraining these paths to zero did not result in a model which provided a much better fit to the data (). Furthermore it resulted in less explained variance in the dependent variable health (R2 = .60), leading us to believe that all the direct paths together and the indirect effects also contributed. The amount of variance explained in the first model is also a test of the predictive validity of our chosen or developed measures. Combining all this led us to confirm our hypothesis that self-regulation in students has a positive effect on health, through problem-solving behavior (including avoiding health (risk) behavior) and improving quality of life.
4. Study III: Development of a web-based instrument and effectiveness evaluation
4.1. Procedure
We used NETQ software (NetQ, Citation2011) to build the self-regulation web-based instrument for promoting student health, including traffic lights, personalized feedback, relevant information and referral. At the same time a website, http://www.studenthealthcheck.nl/, that was connected to the instrument was developed in cooperation with a web builder and web designer; the website also included information about the project and its history.
The instrument consisted of a general section of questions that all the students had to complete and several other sections that were designed for specific subsets of students. Some questions were not applicable to all students, such as frequency of smoking, drinking or using drugs. The self-regulation section was an additional section that only students with at least two amber traffic lights or one red traffic light (indicative of problems) had to complete. The instrument also included an evaluation of effectiveness (based on an identification of the problems and an assessment of behavioral intentions at two points in time: immediately after filling in the questionnaire and three weeks after reading the feedback). A ‘dropout’ questionnaire, asking for reasons of non-completion, was also added to the instrument. We tested the Internet self-regulation instrument for promoting student health and assessed its feasibility (process evaluation) (Van der Heijde et al., Citation2011). A year later, students were contacted by email and asked to complete an effectiveness evaluation (examining behavioral changes and any quality of life improvement which they ascribed to filling in the questionnaire and reading the personalized feedback).
4.1.1. Feedback
The personalized feedback from the instrument includes traffic lights that serve as a warning (red, amber, green), some relevant information and referral options. We chose the traffic light system because its meaning is clear, fast and unambiguous. It has also been found that combining high fear messages with messages facilitating the development of an action plan was most effective in changing health behavior attitudes (Diefenbach & Leventhal, Citation1996). A number of public health interventions (e.g. nutrition, obesity; e.g. Balcombe, Fraser, & Di Falco, Citation2010; Sacks, Veerman, Moodie, & Swinburn, Citation2011) have also confirmed this combination's potential effectiveness. Every completed measurement scale in the instrument was followed by a text message, the respondent's score, peers’ average scores (following the ‘social norms approach’, Berkowitz, Citation2004; Perkins, Meilman, Leichliter, Cashin, & Presley, Citation1999) and, where necessary, included referrals (e.g. to the student health service, student psychologist or particular web facilities).
We wrote several versions of the feedback for the students, correlated to specific answers and score ranges in the various sections of the instrument. If the student's score was in the problematic range, the student received a message that the situation was undesirable and was given tips on how to change the situation. If the student's score was in the unproblematic score range, the student received a text message stating that the situation was okay. All the texts were formulated in a neutral fashion. For referrals to certain websites, the HON code (http://www.hon.ch/) was taken into account, as there are no official rules or regulations in the Netherlands about the quality and reliability of health and medical information on the Internet (Van de Laar & Meijman, Citation2011) ().
4.1.2. Determining the cut-off scores and traffic lights
Calculation of cut-off scores was needed to be able to provide the students with personalized feedback using the image of a green, amber or red traffic light. To determine the cut-off scores we chose the participants from our first study which took place in the waiting room of a student health facility. This sample had a wider range of students than the other, both in terms of enrolled study programs as well as various study phases. Despite the fact that these students were potential patients, many students visited the practice for contraceptives or vaccinations and not because they were ill. An exception was made for the measurement scales that were administered solely in Study II (Internet addiction, buying addiction) and scales that were transformed and improved in Study II.
Creating traffic light responses for every section (measurement scale) of the questionnaire meant that a large number of traffic lights were produced, so it was agreed that the personalized feedback should be centered on the following themes: health, health (risk) behavior, satisfaction with study, quality of student life and self-regulation (). If the result of one measurement scale, relating to a certain theme, was in the 15% most extreme and disadvantageous score range, an amber traffic light response was generated; if two or more measurement scales produced results in the 15% most extreme and disadvantageous score range, then a red traffic light was generated. For traffic lights that were based on the outcome of only one measurement scale, a result which was in the 10–20% most extreme and disadvantageous score range produced an amber traffic light and a result in the 10% most extreme and disadvantageous score range produced a red traffic light. Cut-off scores were set in line with recommendations of Ingraham and Aiken (Citation1996).
Table 4. Cut-off scores based on Samples 1 and 2 and traffic lights for the web-based instrument.
4.2. Participants
In 2011, the third group participated in the first round of the web-based traffic-light instrument with automatized and personalized feedback. The students in this group were invited from a limited number of study programs at a Dutch university. This gave us an opportunity to improve the instrument before all the study programs started using it. Advisory services, executive boards, educational and research directors, and student committees, were all informed about the project, its goals and the project results (newsletters). The total number of students that eventually took part was 3881. Students from an adequately varied group of study programs participated to ensure that the data collated could be generalized to a larger spectrum of university students (Science, Medicine, Dentistry, Pedagogics, Educational Sciences, Philosophy, Art, Religious and Cultural Sciences, Communication Science, Economics and Health Sciences). The average response rate from these study programs was 9%.
The study program with the highest response rate (20%) used several channels (e.g. intranet messages, newsletters, flyers) to inform their students about the project, including three emails. Not all study programs, however, followed this procedure, so we anticipated that we would have lower response rates as a consequence. When students were approached by their study programs, instead of some central body of the university, they demonstrated very high percentages (95%) of reach (almost no dropouts in filling out the complete questionnaire) (Steckler & Linnan, Citation2002).
The website was also open to other Dutch students from other universities, who could participate spontaneously, thereby enlarging the potential of the instrument to make a contribution to the health of the student population. This also brought the total number of respondents up to 4009 and increased the generalizability of the data. Thirty percent of the students were male and 70% female. Their mean age was 22.80 years (SD = 4.33). Twenty-eight percent were first-year students, 58% were bachelor students, 13% were master students and 1% were final-year medical students. The majority of the students (96%) considered themselves to be Dutch, 50% reported themselves as being in a steady relationship and 77% had some form of employment. Forty-five percent of the students reported that they lived with their parents or family, 22% with peers, 18% alone and 14% with a partner.
On average the rate of completed questionnaires was 62%, with the majority of respondents rating the usability of the questionnaire as reasonable to good (Very good quality 8%, Good quality 51%, Reasonable quality 33%, Bad quality, 6% and Very bad quality 2%). Of those who completed the questionnaire, 74 percent also agreed to take part in the future. The most important reasons for not completing given by a sample of 237 dropouts were: too many questions (36%), some technical error (12%) or no time left (11%).
The percentage of students who received and read their personalized feedback was 38%, while the percentage who completed the additional section of the instrument on the effects of the intervention (intentions and behavioral change with regard to self-regulation, health, health (risk) behavior and quality of life) some three weeks later was 16%. The percentage of students who completed the questionnaire a year later was 10%. The level of non-response was explained, to some extent, by outdated email addresses (caused, in part, by students graduating). Since logging onto the system with anonymous email addresses was encouraged, this non-response level was an accepted risk.
4.3. Analysis and results
4.3.1. Analysis
We examined the frequencies of the identification of health- or student-related problems, and behavioral intentions reported directly after filling out the questionnaire, and, from reading the feedback reported after three weeks. We also examined the frequencies of the behavioral changes caused by the intervention, quality of life improvement stimulated by filling out the questionnaire and quality of life improvement from reading the feedback reported after a year. We used the Pearson chi-square test and Fisher's two-tailed exact test to assess differences by gender, study phase and living situation (SPSS 20.0, IBM). Generally, a p-value <.05 in the data analyses was considered statistically significant.
4.3.2. Results in general
Directly after completing the questionnaire, 14.6% of students reported that they had identified health- or student-related problems and 16.9% claimed to have different behavioral intentions from filling out the questionnaire. Four percent did not report any such effects, although they reported to have problems.
After three weeks, of the students who filled out the questionnaire and read their feedback, 30.1% reported that they had identified health- or student-related problems, 28.1% reported behavioral intentions and 22.8% behavioral changes after reading the feedback. Nine point one percent reported quality of life improvement from filling out the questionnaire and 8.4% reported quality of life improvement from reading the feedback.
Even after a year, of the students who filled out the questionnaire and read their feedback, 9.7% reported behavioral change from the intervention, 9.1% reported quality of life improvement from filling out the questionnaire and 5.2% reported quality of life improvement from reading the feedback.
4.3.3. Results by gender, study phase and living situation
Our chi-square test revealed that significantly more female students (15.6%) than male students (12.3%) identified health- or student-related problems, χ2(1, N = 2473) = 4.56, p = .03 and significantly more female students (18.1%) than male students (14.0%) reported that they had different behavioral intentions after filling in the questionnaire χ2(1, N = 2462) = 6.13, p = .01. After three weeks we found no significant differences between female and male students (reported identification of health- or student-related problems, behavioral intentions and behavioral change after reading the feedback). Interestingly, we found inversed significant differences between female and male students after a year. Significantly more male students (21.4%) than female students (6.5%) reported behavioral change resulting from the intervention χ2(1, N = 300) = 13.25, p = .00 and significantly more male students (17.6%) than female students (8.4%) reported quality of life improvement as a result of filling out the questionnaire χ2(1, N = 293) = 4.67, p = .03. Possibly female students anticipated the benefits of the Student Health Check at an earlier stage than male students.
As regards study phase, we found differences regarding identifying health problems from completing the questionnaire and reading the personalized feedback. Significantly more bachelors (including first-year students) (15.6%) than master students (9.5%) identified health- or student-related problems, χ2(1, N = 2402) = 8.04, p = .01 directly after filling out the questionnaire. We also found that significantly more bachelor students (32.6%) than master students (17.3%) identified health- or student-related problems, χ2(1, N = 640) = 9.73, p = .00 from reading their personalized feedback. It seems as though the Student Health Check is even more helpful for students who are in their bachelor phase than it is for those in their master phase.
As regards living situation, we found some significant differences between more social living situations and living alone. Significantly more students living alone (39.6%) than students living with peers (28.3%) reported changed behavioral intentions χ2(1, N = 263) = 3.73, p = .05 and significantly more students living alone (36.0%) than students living with peers (18.4%) reported behavioral change χ2(1, N = 247) = 9.74, p = .00 after three weeks, from reading their personalized feedback. A possible interpretation is that students living alone profit more from the personalized feedback than students living with peers or a partner, because they already received feedback regarding their health situation from their living partners. The same pattern is found between students living alone and students living with a partner. Significantly more students living alone (39.6%) than students living with a partner (18.8%) reported behavioral intentions χ2(1, N = 180) = 8.51, p = .00 and significantly more students living alone (36.0%) than students living with a partner (14.1%) reported behavioral change χ2(1, N = 164) = 9.43, p = .00 after three weeks, from reading their personalized feedback. We also demonstrated a trend between these groups as regards quality of life improvement from the personalized feedback (Fisher's exact test, N = 157, p = .071). More students living alone (14.4%) than students living with a partner (5.0%) reported quality of life improvement from reading their personalized feedback. No similar differences were shown immediately after filling out the Student Health Check or after a year.
5. Discussion
To the best of our knowledge, we have developed the first valid and reliable preventative web-based self-regulation instrument for the promotion of student health which considers the totality of student life (health, health (risk) behavior, quality of life, and satisfaction with study) rather than simply study-related factors (Vaez et al., Citation2010; Verouden et al., Citation2010) or health (risk) behavioral factors (Nooijer et al., Citation2008). The advantage of our instrument is that it is quite complete and contains many themes relevant to students. Apart from giving a snapshot of the student's life status quo, the instrument points them to blind spots that they may have been unaware of. Students are often hesitant about seeking help for their physical and/or mental health problems and by doing so they risk their health status deteriorating (with repercussions on academic performance). The strength of this instrument is that it enables health (-related) problems to be detected by the student at an early stage and enabling them to take action themselves, without the immediate personal intervention of a professional.
We demonstrated (Study II) that self-regulation in students has a positive effect on health, through problem-solving behavior (including avoiding health (risk) behavior) and improving quality of life. Furthermore, a substantial number of students (Study III) reported effects from completing the self-regulation instrument. Apart from the early recognition of health(-related) problems in a student's life, this surveillance instrument could also motivate students to change their health-related behavior based on their personalized feedback. Part of its effect possibly comes from its high-end tailoring, combining a personalized questionnaire with personalized feedback, based on the provision of personalized messages and on measurements tailored to a university students’ population.
Another strength of the instrument is the signaling function on a group level, which enables faculties or study programs to get insight into their student populations’ health status, health (risk) behavior and quality of life. Faculties can monitor the intensity of their study programs and the adequacy of their student support services so that they can safeguard healthy studying.
5.1. Internet as a medium for self-regulation
Research in the past has demonstrated that it is quite difficult to reach and help students with health (-related) problems because they avoid seeking help, so an intervention which uses the Internet is a promising choice. A preventative web-based self-regulation instrument satisfies the students’ desire to try and help themselves before seeking out professional support. Bandura (Citation2005, p. 250) points to the fact that ‘people at risk for certain health problems typically ignore preventive or remedial health services’, but interactive technological advances now have potential to overcome this attitude and interventions using the Internet have potential to improve health-related knowledge, attitudes, intentions and behavior (Portnoy, Scott-Sheldon, Johnson, & Carey, Citation2008).
As the Internet is anonymous (compared to face-to face, post and telephone surveys), it is a suitable medium for collecting information about risky health behavior that is normally influenced by social desirability (Pealer, Weiler, Pigg, Miller, & Dorman, Citation2001). From previous studies, it appears that students are quite willing to complete questionnaires about their health status (Bewick et al., Citation2008; Boot et al., Citation2007).
5.2. Study limitations
There are several limitations to the project. First, its representativeness may be endangered if preventative web-based self-regulation instruments for promoting student health prove more attractive to students with health (-related) problems. This may cause an overestimation of the number of problems in the overall student population to be made. On the other hand, there is also a chance that students who have major health (-related) problems do not participate or dropout early because of their perception of it as a threatening experience. The fact that approximately three out of the four students responding were female is a well-known phenomenon with regard to health issues. Currently, in the Netherlands, the distribution of the sexes in higher education is about 50/50 (Ministerie van Onderwijs, Cultuur & Wetenschap, n.d.). Further tailoring of the self-regulation instrument to manage this gender bias should be done, wherever necessary.
Second, as we do not have any data from clinical and non-clinical student populations with which to make comparisons, specificity and sensitivity cannot be calculated at this stage. We do not pretend to provide students with a diagnosis or make statements about abnormality though. In their feedback, students are told their exact scores, what the average student scores are and in which range their scores are (e.g. 10% upper or 10–20% upper). In the near future a study could be made in which the Student Health Check outcomes of normal and clinical student populations are compared and cut-off scores adapted accordingly.
Third, the research model needs to be tested longitudinally, so that the plausibility of the existence of the causal relationships between self-regulation, quality of life and health outcomes can be examined at more depth. Some students filled out the instrument more than once, sometimes a month or a couple of months apart, suggesting that a number of students are motivated to monitor their health, health (risk) behavior and quality of life on a regular basis.
Fourth, although the instrument follows recommended guidelines about its accessibility, usability and recruitment of participants, (Hallett, Maycock, Kypri, Howat, & Mcmanus, Citation2009; Morrison et al., Citation2013) it is rather long. For the group of students who had a major health (-related) problem, the length of the instrument may have proved too much of a burden. However, if being able to provide personalized, tailored and extensive feedback is a principal goal, then a lengthy questionnaire that collects all the necessary personal information to create this feedback is inevitable.
During the whole process of self-regulation (filling out the questionnaire, reading the extensive feedback and reporting on effects) too many students give up. Several initiatives to encourage students to complete the entire self-regulation process include: having more extensive information up front about what the goal is (preferably from tutors, study advisors, counselors and professors), being clear about the questionnaire's length, offering advice in case of technical errors, and delivering inspiring messages and information about the respondent's progress during the questionnaire's completion. Another solution would be to rotate the topics over several years so that there was only a limited number each year.
Fifth, we did not opt for a randomized controlled trial during this stage of the project. Our first aim was to develop a valid instrument with personalized feedback, capture the attention of university students and personnel and test its usability and practicality. The next step might be to conduct a randomized controlled trial to demonstrate the effectiveness of the instrument, a trial which includes tracking down students’ routes to health professionals via the links provided in the feedback.
5.3. Research implications
The group of students who completed the effectiveness evaluation after three weeks and then again after a year, indicated that students benefited from the self-regulation process in that it enabled them to: (1) identify health- and other student life-related complaints and from the personalized feedback (2) stay motivated to change their health-related behavior. More extensive analyses are necessary to identify the relationship between parts of the self-regulation process and specific improvements in health- and student-related problems.
Further study is also necessary to determine whether the personalized feedback is being delivered with visual and reading materials that are effective. Thus, future research may be helpful to optimize the personalized feedback messages. For example, various effects have been demonstrated on students’ health (risk) behavior using texts formulated in terms of gains or losses (e.g. regarding physical exercise, using sunscreen) and positive or negative images (e.g. a healthy person, a sick person) (Detweiler, Bedell, Salovey, Rothman, & Pronin, Citation1999; Rothman, Kelly, Hertel, & Salovey, Citation2003; Werch, Citation2007). Extra attention also has to be given to the online presentation and promotional activities to better capture and hold the attention of the target group, in this case, students in higher education (Mesters et al., Citation2010).
5.4. Practical implications
This instrument provides students with a fairly complete overview of their health-related quality of life. Student participation can be made a student facility/service that contributes to students’ health and quality of life. In addition to the high accessibility and speed of an e-instrument and the confidentiality it offers, an Internet-based intervention becomes financially attractive after the initial financial investments have been made. This is an important factor given the increased stress levels that students face and the lower budgets for guidance and counseling services that universities currently have (Chiauzzi et al., Citation2008). The fact that self-assessment and motivational feedback can be used with other services (such as guidance and counseling) (Chiauzzi et al., Citation2008), and that the information can be easily updated with new materials and developments, make the instrument even more advantageous. Students often start seeking help for emotional problems on the Internet during high school and then combine this with other sources of help (Gould, Munfakh, Lubell, Kleinman, & Parker, Citation2002).
The instrument could focus even more upon bachelor students and students living alone, as these groups appear to benefit proportionately more from its use. Finally, this instrument serves as an example of a possible intervention for various patient groups who do not seek help easily, thereby supporting consumer empowerment. These groups could include patients with mental health or other problems concerning sensitive subjects (e.g. sexual problems, reproductive problems, suicidal thoughts). Reaching out to these groups could be effected through various communication channels such as GP's websites, health-care facilities and social media.
Acknowledgements
We would like to thank all the students, advisory services and other employees of the University of Amsterdam/Amsterdam University of Applied Sciences who made a contribution to this study, and supported the development of the web-based health questionnaire, including the feedback tool. We would also like to thank the three anonymous reviewers and the associate editor of Health Psychology and Behavioral Medicine for the valuable feedback they provided which enabled us to improve our manuscript. Ethics approval: The Ethics Committee at the University of Amsterdam granted ethics approval for this project.
Disclosure statement
No potential conflict of interest was reported by the authors.
ORCID
Claudia Maria Van der Heijde http://orcid.org/0000-0002-5256-0785
Peter Vonk http://orcid.org/0000-0001-9011-1908
Frans J. Meijman http://orcid.org/0000-0001-5032-540X
References
- Aaronson, N. K., Muller, M., Cohen, P. D., Essink-Bot, M. L., Fekkes, M., Sanderman, R., … Verrips, E. (1998). Translation, validation and norming of the Dutch language version of the SF-36 health survey in community and chronic disease populations. Journal of Clinical Epidemiology, 51, 1055–1068. doi:10.1016/S0895-4356(98)00097-3
- Arbuckle, J. (2003). Amos 5.0 update to the Amos user's guide. Chicago: SmallWaters.
- Balcombe, K., Fraser, I., & Di Falco, S. (2010). Traffic lights and food choice: A choice experiment examining the relationship between nutritional food labels and price. Food Policy, 35, 211–220. doi:10.1016/j.foodpol.2009.12.005
- Bandura, A. (2005). The primacy of self-regulation in health promotion. Applied Psychology, 54, 245–254. doi:10.1111/j.1464-0597.2005.00208.x
- Berkowitz, A. D. (2004). An overview of the social norms approach. In L. Lederman, L. Stewart, F. Goodhart & L. Laitman (Eds.), Changing the culture of college drinking: A socially situated prevention campaign (pp. 187–208). Creskill, NJ: Hampton Press.
- Bewick, B. M., Trusler, K., Mulhern, B., Barkham, M., & Hill, A. J. (2008). The feasibility and effectiveness of a web-based personalised feedback and social norms alcohol intervention in UK university students: A randomised control trial. Addictive Behaviors, 33, 1192–1198. doi:10.1016/j.addbeh.2008.05.002
- Boot, C. R. L., Donders, N. C. G. M., Vonk, P., & Meijman, F. J. (2009). Development of a student health questionnaire: The necessity of a symbiosis of science and practice. Global Health Promotion, 16, 35–44. doi:10.1177/1757975909339763
- Boot, C. R. L., Meijman, F. J., & Vonk, P. (2010). Problem perception in Dutch university students using tobacco, alcohol and drugs. Communication and Medicine, 7, 33–42. doi:10.1558/cam.v7i1.33
- Boot, C. R. L., Vonk, P., & Meijman, F. J. (2007). Health-related profiles of study delay in university students in the Netherlands. International Journal of Adolescent Medicine and Health, 19, 413–423. doi:10.1515/IJAMH.2007.19.4.413
- Calvete, E., & Connor-Smith, J. K. (2006). Perceived social support, coping, and symptoms of distress in American and Spanish students. Anxiety, Stress, and Coping, 19, 47–65. doi:10.1080/10615800500472963
- Cameron, L., Leventhal, E. A., & Leventhal, H. (1995). Seeking medical care in response to symptoms and life stress. Psychosomatic Medicine, 57, 37–47. doi: 10.1097/00006842-199501000-00006
- Cassidy, T. (2004). Mapping variables related to social identity, distress and perceived health in an undergraduate student population. Social Psychology of Education, 7, 339–352. doi:10.1023/B:SPOE.0000037504.24380.b3
- Chiauzzi, E., Brevard, J., Thum, C., Decembrele, S., & Lord, S. (2008). My StudentBody Stress: An online stress management intervention for college students. Journal of Health Communication, 13, 555–527. doi:10.1080/10810730802281668
- Cohen, S., Kamarck, T., & Mermelstein, R. (1983). A global measure of perceived stress. Journal of Health and Social Behavior, 24, 385–396. doi: 10.2307/2136404
- Deci, E. L., & Ryan, R. M. (2000). The ‘what’ and ‘why’ of goal pursuits: Human needs and the internal motivation of behavior. Psychological Inquiry, 11, 227–268. doi:10.1207/S15327965PLI1104_01
- De Hoog, D., & Schilp, M. (2012, March 8). De grote studiedruk enquête [The big academic pressure survey]. Ad Valvas, Dossier, 6–8.
- Detweiler, J. B., Bedell, B. T., Salovey, P., Rothman, A. J., & Pronin, E. (1999). Message framing and sunscreen use: Gain-framed messages motivate beach-goers. Health Psychology, 18, 189–196. doi:10.1037/0278-6133.18.2.189
- Diefenbach, M. A., & Leventhal, H. (1996). The common-sense model of illness representations: Theoretical and practical considerations. Journal of Social Distress & the Homeless, 5, 11–38. doi:10.1007/BF02090456
- Donker, T., Comijs, H. C., Cuijpers, P., Terluin, B., Nolen, W. A., Zitman, F. G., & Penninx, B. W. (2010). The validity of the Dutch K10 and extended K10 screening scales for depressive and anxiety disorders. Psychiatry Research, 176, 45–50. doi:10.1016/j.psychres.2009.01.012
- Eisenberg, D., Golberstein, E., & Gollust, S. E. (2007). Help-seeking and access to mental health care in a university student population. Medical Care, 45, 594–601. doi:10.1097/MLR.0b013e31803bb4c1
- El Ansari, W., & Stock, C. (2010). Is the health and wellbeing of university students associated with their academic performance? Cross sectional findings from the United Kingdom. International Journal of Environmental Research and Public Health, 7, 509–527. doi:10.3390/ijerph7020509
- Escoffery, C., McCormick, L., & Bateman, K. (2004). Development and process evaluation of a web-based smoking cessation program for college smokers: Innovative tool for education. Patient Education and Counselling, 53, 217–225. doi:10.1016/S0738-3991(03)00163
- Etter, J. F., LeHouezec, J., & Perneger, T. V. (2003). A self-administered questionnaire to measure addiction to cigarettes: The cigarette dependence scale. Neuropsychopharmacology, 28, 359–370. doi:10.1038/sj.npp.1300030
- Faber, R. J., & O'Guinn, T. C. (1992). A clinical screener for compulsive buying. Journal of Consumer Research, 19, 459–469. doi: 10.1086/209315
- Farmer, C. S., Farrand, P., & O'Mahen, H. (2012). ‘I am not a depressed person’: How identity conflict affects help-seeking rates for major depressive disorder. BMC Psychiatry, 12, 164. doi:10.1186/1471-244X-12-164
- Franko, D. L., Cousineau, T. M., Trant, M., Green, T. C., Rancourt, D., Thompson, D., … Ciccazzo, M. (2008). Motivation, self-efficacy, physical activity and nutrition in college students: Randomized controlled trial of an internet-based education program. Preventive Medicine, 47, 369–377. doi:10.1016/j.ypmed.2008.06.013
- Gould, M. S., Munfakh, J. L. H., Lubell, K., Kleinman, M., & Parker, S. (2002). Seeking help from the Internet during adolescence. Journal of American Academy of Child and Adolescent Psychiatry, 41, 1182–1188. doi:10.1097/00004583-200210000-00007
- Hagger, M. S., & Orbell, S. (2003). A meta-analytic review of the common-sense model of illness representations. Psychology and Health, 18, 141–184. doi:10.1080/088704403100081321
- Hall, N. C., Chipperfield, J. G., Perry, R. P., Ruthig, J. C., & Goetz, T. (2006). Primary and secondary control in academic development: Gender-specific implications for stress and health in college students. Anxiety, Stress, and Coping, 19, 189–210. doi:10.1080/10615800600581168
- Hallett, J., Maycock, B., Kypri, K., Howat, P., & Mcmanus, A. (2009). Development of a web-based alcohol intervention for university students: Processes and challenges. Drug and Alcohol Review, 28, 31–39. doi:10.1111/j.1465-3362.2008.00008.x
- Hurst, C. S., Baranik, L. E., & Daniel, F. (2013). College student stressors: A review of the qualitative research. Stress and Health, 29, 275–285. doi:10.1002/smi.2465
- Ingraham, L. J., & Aiken, C. B. (1996). An empirical approach to determining criteria for abnormality in test batteries with multiple measures. Neuropsychology, 10, 120–124. doi: 10.1037/0894-4105.10.1.120
- Jessop, D. C., Herberts, C., & Solomon, L. (2005). The impact of financial circumstances on student health. British Journal of Health Psychology, 10, 421–439. doi:10.1348/135910705X25480
- John, O. P. & Gross, J. J. (2004). Healthy and unhealthy emotion regulation: Personality processes, individual differences and lifespan development. Journal of Personality, 72, 1301–1334. doi:10.1111/j.1467-6494.2004.00298.x
- Karam, E., Kypri, K., & Salamoun, M. (2007). Alcohol use among college students: An international perspective. Current Opinion in Psychiatry, 20, 213–221. doi:10.1097/YCO.0b013e3280fa836c
- Kessler, R. C., Andrews, G., Colpe, L. J., Hiripi, E., Mroczek, D. K., Normand, S.-L.T., … Zaslavsky, A. M. (2002). Short screening scales to monitor population prevalences and trends in nonspecific psychological distress. Psychological Medicine, 32, 959–976. doi:10.1017/S0033291702006074
- Kitzrow, M. A. (2003). The mental health needs of today's college students: Challenges and recommendations. NASPA Journal, 41, 167–181. doi: 10.2202/0027-6014.1310
- Kuo, B. C. H., Roysircar, G., & Newby-Clark, I. R. (2006). Development of the cross-cultural coping scale: Collective, avoidance, and engagement strategies. Measurement and Evaluation in Counseling and Development, 39, 161–181.
- Larimer, M. E., & Cronce, J. M. (2007). Identification, prevention, and treatment revisited: Individual-focused college drinking prevention strategies 1999–2006. Addictive Behaviors, 32, 2439–2468. doi:10.1016/j.addbeh.2007.05.006
- Leventhal, H., Leventhal, E. A., & Contrada, R. J. (1998). Selfregulation, health, and behavior: A perceptual-cognitive approach. Psychology & Health, 13, 717–733. doi:10.1080/08870449808407425
- Lewin, T. (2011, January 26). Record level of stress found in college freshmen. The New York Times. Retrieved from http://www.nytimes.com/
- Maes, S., & Karoly, P. (2005). Self-regulation assessment and intervention in physical health and illness: A review. Applied Psychology: An International Review, 54, 267–299. doi:10.1111/j.1464-0597.2005.00210.x
- McAndrew, L. M., Burns, E., Musumeci-Sbazo, T., Mora, P., Halm, E., Vileikyte, L., … Leventhal, H. (2008). Using the common sense model to design interventions for the prevention and management of chronic illness threats: From description to process. British Journal of Health Psychology, 13, 195–204. doi:10.1348/135910708X295604
- Mesters, I., Van der Velden, N., Drenthen, T., Van Dale, D., Kok, G., Brug, H., … Van der Velde, M. (2010). Standaard web-based leefstijlinterventies [Norm web-based lifestyle interventions]. Maastricht: Maastricht University.
- Ministerie van Onderwijs, Cultuur & Wetenschap. (n.d.). Monitor Trends in Beeld: Aantal studenten in het hoger onderwijs als percentage van de bevolking van 18–25 jaar [Number of students in higher education as a percentage of the population from 18–25 years old]. Retrieved from http://www.trendsinbeeld.minocw.nl/grafieken/3_1_1_3.php
- Morrison, L., Moss-Morris, R., Michie, S., & Yardly, L. (2013). Optimizing engagement with Internet-based health behaviour change interventions: Comparison of self-assessment with and without tailored feedback using a mixed methods approach. British Journal of Health Psychology, 1–71. doi:10.1111/bjhp.12083
- Nauta, M. C. E., Meijman, F. J., & Meijman, T. F. (1996). Perceived health and perceived study situation of university students in Amsterdam [Dutch: De subjectief ervaren gezondheid en studiebeleving van studenten van de Universiteit van Amsterdam]. Tijdschrift voor Sociale Gezondheidszorg, 74, 391–396.
- NETQ Internet Surveys 6.7. (2011). Software for creating and assessment of internet surveys. Utrecht: NetQuestionnaires Nederland BV. Retrieved from http://www.netq.nl
- de Nooijer, J., Veling, M. L., Ton, A., de Vries, H., & de Vries, N. K. (2008). Electronic monitoring and health promotion: An evaluation of the E-MOVO Web site by adolescents. Health Education Research, 23, 382–391. doi:10.1093/her/cym086
- Orr, D., Gwosc, C., & Netz, N. (2011). Social and economic conditions of student life in Europe. Synopsis of indicators (Final report. Eurostudent IV 2008–2011). Bielefeld: W. Bertelsmann Verlag.
- Pealer, L. N., Weiler, R. M., Pigg, R. M., Miller, D., & Dorman, S. M. (2001). The feasibility of a web-based surveillance system to collect health risk behavior data from college students. Health Education and Behavior: The Official Publication of the Society for Public Health Education, 28, 547–559. doi:10.1177/109019810102800503
- Perkins, H. W., Meilman, P. W., Leichliter, J. S., Cashin, J. R., & Presley, C. A. (1999). Misperceptions of the norms for the frequency of alcohol and other drug use on college campuses. Journal of American College Health, 47, 253–258. doi:10.1080/07448489909595656
- Philipsen, R. (2014, June 1). Generatie Y is niet lui, maar moe van de excellentiedruk [Generation Y is not lazy, but tired from the pressure to perform], Trouw. Retrieved from http://www.trouw.nl/tr/nl/6704/Sociale-Vraagstukken/article/detail/3665032/2014/06/01/Generatie-Y-is-niet-lui-maar-moe-van-de-excellentiedruk.dhtml
- Portnoy, D. B., Scott-Sheldon, L. A. J., Johnson, B. T., & Carey, M. P. (2008). Computer-delivered interventions for health promotion and behavioral risk reduction: A meta-analysis of 75 randomized controlled trials, 1988–2007. Preventive Medicine, 47, 3–16. doi:.org/10.1016/j.ypmed.2008.02.014 doi: 10.1016/j.ypmed.2008.02.014
- Raviv, A., Sills, R., Raviv, A., & Wilansky, P. (2000). Adolescents’ help-seeking behaviour: The difference between self- and other-referral. Journal of Adolescence, 23, 721–740. doi.org/10.1006/jado.2000.0355 doi: 10.1006/jado.2000.0355
- Recourt, A. (2012, March 21). Beter afremmen dan opbranden [Better slow down instead of burn out]. Folia Magazine, 25, 32–34.
- Robotham, D. (2008). Stress among higher education students: Towards a research agenda. Higher Education, 56, 735–746. doi:10.1007/s10734-008-9137-1
- Robotham, D., & Julian, C. (2006). Stress and the higher education student: A critical review of the literature. Journal of Further and Higher Education, 30, 107–117. doi:10.1080/03098770600617513
- Rothman, A. J., Kelly, K. M., Hertel, A., & Salovey, P. (2003). Message frames and illness representations: Implications for interventions to promote and sustain healthy behavior. In L. D. Cameron & H. Leventhal (Eds.), The self-regulation of health and illness behavior (pp. 278–296). New York, NY: Routledge.
- Sacks, G., Veerman, J. L., Moodie, M., & Swinburn, B. (2011). ‘Traffic-light’ nutrition labelling and ‘junk-food’ tax: A modelled comparison of cost-effectiveness for obesity prevention. International Journal of Obesity, 35, 1001–1009. doi:10.1038/ijo.2010.228
- Saitz, R., Palfai, T. P., Freedner, N., Winter, M. R., Macdonald, A., Lu, J., … Dejong, W. (2007). Screening and brief intervention online for college students: The ihealth study. Alcohol and Alcoholism, 42, 28–36. doi:10.1093/alcalc/agl092
- Salmelo-Aro, K. (2010). Personal goals and well-being: How do young people navigate their lives? New Directions for Child and Adolescent Development, 130, 13–26. doi:10.1002/cd.278
- Saunders, J. B., Aasland, O. G., Babor, T. F., de la Fuente, J. R., & Grant, M. (1993). Development of the Alcohol Use Disorders Identification Test (AUDIT): WHO collaborative project on early detection of persons with harmful alcohol consumption: II. Addiction, 88, 791–804. doi:10.1111/j.1360-0443.1993.tb02093.x
- Schmidt, E., & Simons, M. (2013). Psychische klachten onder studenten [Students and mental health complaints]. Utrecht: LSVB.
- Schunk, D. H. (2001). Self-regulation through goal setting (ERIC/CASS Digest: ED462671). Washington, DC: ERIC/CASS.
- Schwartz, A. J. (2006). Are college students more disturbed today? Stability in the acuity and qualitative character of psychopathology of college counseling center clients: 1992–1993 through 2001–2002. Journal of American College Health, 54, 327–337. doi:10.3200/JACH.54.6.327-337
- Skinner, H. (1982). The drug abuse screening test. Addictive Behaviors, 7, 363–371. doi:10.1016/0306-4603(82)90005-3
- Steckler, A., & Linnan, L. (2002). Process evaluation for public health interventions and research. San Francisco: Jossey Bass.
- Stewart–Brown, S., Evans, J., Patterson, J., Peterson, S., Doll, H., Balding, J., & Regis, D. (2000). The health of students in institutes of higher education: An important and neglected public health problem? Journal of Public Health Medicine, 22, 492–499. doi:10.1093/pubmed/22.4.492
- The National Center on Addiction and Substance Abuse (CASA) at Columbia University. (2007). Wasting the best and the brightest: Substance abuse at America's colleges and universities. New York: Author.
- Timlin-Scalera, R. M., Ponterotto, J. G., Blumberg, F. C., & Jackson, M. A. (2003). A grounded theory study of help-seeking behaviors among white male high school students. Journal of Counseling Psychology, 50, 339–350. doi:10.1037/0022–0167.50.3.339
- Vaez, M., Kristenson, M., & Laflamme, L. (2004). Perceived quality of life and self-rated health among first-year university students – a comparison with their working peers. Social Indicators Research, 68, 221–234. doi:10.1023/B:SOCI.0000025594.76886.56
- Vaez, M., Voss, M., & LaFlamme, L. (2010). Health-related quality of life among university students. In V. R. Preedy & R. R. Watson (Eds.), Handbook of disease burdens and quality of life measures (pp. 2555–2577). New York, NY: Springer New York.
- Van de Laar, F., & Meijman, F. J. (2011). Zoekers op internet de goede kant op helpen [Leading surfers into the right direction]. Medisch Contact, 66, 876–877.
- Van den Eijnden, R. J. J. M., Meerkerk, G. J., Vermulst, A. A., Spijkerman, R., & Engels, R. C. M. E. (2008). Online communication, compulsive internet use, and psychosocial well-being among adolescents: A longitudinal study. Developmental Psychology, 44, 655–665. doi:10.1037/0012-1649.44.3.655
- Van der Heijde, C. M., Vonk, P., & Meijman, F. J. (2011). Developing a valid and reliable preventative web-based self-regulation instrument for promoting student health: Stop lights. Internal Student Health Service Report, unpublished.
- Verouden, N. W., Vonk, P., & Meijman, F. J. (2010). Context guides illness-identity: A qualitative analysis of Dutch university students’ non-help-seeking behavior. International Journal of Adolescent Health and Medicine, 22, 307–320. doi:10.1515/IJAMH.2010.22.2.307
- Vidourek, R. A., King, K. A., Nabors, L. A., Lynch, A. N., & Merianos, A. L. (2014). College students’ perceived confidence in mental health help-seeking. International Journal of Mental Health Promotion, 16, 83–90. doi:10.1080/14623730.2014.892257
- Visser, P. L., & Hirsch, J. K. (2014). Health behaviors among college students: The influence of future time perspective and basic psychological need satisfaction. Health Psychology and Behavioral Medicine: An Open Access Journal, 2, 88–99. doi:10.1080/21642850.2013.872992
- Werch, C. (2007). The behavior-image model: A paradigm for integrating prevention and health promotion in brief interventions. Health Education Research, 22, 677–690. doi:10.1093/her/cyl146
- Yudko, E., Lozhkina, O., and Fouts, A. (2007). A comprehensive review of the psychometric properties of the drug abuse screening test. Journal of Substance Abuse Treatment, 32, 189–198. doi:10.1016/j.jsat.2006.08.002
- Zimmerman, B. J. (2000). Attaining self-regulation: A social cognitive perspective. In M. Boekaerts, P. R. Pintrich, & M. Zeidner, (Eds.), Handbook of self-regulation (pp. 13–39). San Diego: Academic Press.