ABSTRACT
Background:
Many adults with type 1 (T1D) and type 2 diabetes (T2D) have inadequate sleep increasing their risk of hyperglycemia and developing complications. The objective was to identify psychosocial determinants of healthy sleep habits (HSH) among adults with T1D and T2D.
Methods:
The two HSH were: avoiding screen use in bed and having sleep regularity. Adults (≥18 years) with T1D and T2D were invited to complete an anonymous online survey. The questionnaires were based on the Reasoned Action Approach and formative qualitative research previously conducted in 56 adults with T1D and T2D. Habit was included as an additional variable for screen use in bed.
Results:
In total, 320 adults with diabetes (T1D: 39%; T2D: 61%) completed the questionnaires (screen use in bed: 174; sleep timing: 146). Close to 75% of participants reported screen use in bed and close to 90% reported sleep timing variability in the last month. Perceived behavioral control (PBC) to avoid screen use in bed (β = −0.4486, p < 0.0001), habit of using screens in bed (β = 0.4002; p < 0.0001), and age (β = −0.0202; p = 0.0086) were determinants of screen use in bed, and this model explained 71% of the variance. PBC for sleep regularity (β = −0.2909; p = 0.0004) and being female (β = 0.5057; p = 0.0069) were determinants of sleep timing variability, and this model explained 28% of the variance. The most important beliefs associated with each HSH were identified to obtain information to design targeted interventions.
Conclusions:
Few adults with diabetes have HSH. Screen use in bed was strongly influenced by habit and the results suggest that both HSH are not easy to adopt among adults with diabetes. Younger adults with diabetes should be prioritized for screen use in bed, while females with diabetes should be prioritized for sleep timing variability. Adults with diabetes should have access to behavior change interventions to encourage them to adopt HSH.
Introduction
Adults with T1D and T2D are more at risk for poor sleep quality, shorter sleep duration and insomnia compared to adults without diabetes (Vézina-Im et al., Citation2021). Recent evidence suggests that short and poor sleep quality is associated with hyperglycemia among adults with type 1 (T1D) (Reutrakul et al., Citation2016) and type 2 diabetes (T2D) (Lee et al., Citation2017), increasing their risk of complications. Certain behaviors, such as avoiding screen use in bed as well as caffeine, alcohol, and nicotine before bedtime, and having a regular sleep schedule, are considered healthy sleep habits (HSH). Mobile device-based electronic social media in bed is associated with short sleep duration, and nearly 70% of adults recently mentioned engaging in this unhealthy sleep habit (Bhat et al., Citation2018). Caffeine consumption before bedtime can result in sleep disturbances (Drake et al., Citation2013). Alcohol and nicotine consumption within 4 h of bedtime can affect sleep efficiency, and 45.0%, 19.8%, and 9.2% of adults reported consuming caffeine, alcohol or nicotine, respectively, before bedtime (Spadola et al., Citation2019). Some people have night-to-night sleep variability in their sleep/wake patterns, such as going to bed and waking up later on weekends. Sleep variability is associated with risk of T2D in adults (Rosique-Esteban et al., Citation2018) while sleep regularity is associated with favorable health in adults (Chaput et al., Citation2020).
One of the first steps in developing effective behavior change interventions is to identify factors that can influence behavior (Michie et al., Citation2014). Theories from social psychology aimed at predicting behavior, such as the Reasoned Action Approach (RAA) (Fishbein & Ajzen, Citation2010), can guide the development of behavior change interventions. According to the RAA, the adoption of a health behavior is predicted by intention and perceived behavioral control (PBC) (Fishbein & Ajzen, Citation2010) (see ). Intention represents one's motivation to adopt a given behavior. PBC refers to one's capacity and autonomy over adopting the behavior. Intention is predicted by attitudes, perceived norms and PBC. Each construct of intention is linked to a specific set of beliefs. Attitudes are associated with behavioral beliefs which are the anticipated consequences (cognitive attitude) and emotions (affective attitude) following behavior adoption. Perceived norms are associated with normative beliefs which refer to individuals who are presumed to approve or not behavior adoption (injunctive norm) and the perceived prevalence of behavior adoption (descriptive norm). PBC is associated with control beliefs regarding the factors that can facilitate (facilitating factors) or hinder (barriers) behavior adoption. The RAA is open to the inclusion of additional variables (Fishbein & Ajzen, Citation2010). Habits are automatic responses to specific cues (e.g. context, time of the day, location) and imply adopting a behavior with a degree of automaticity or lack of awareness (Gardner & Rebar, Citation2019). Habits can predict the adoption of health behaviors (Gardner, Citation2015), including adolescents’ screen time (Aulbach et al., Citation2023), and screen use in bed represented an ingrained unhealthy habit in our previous formative qualitative research (Vézina-Im et al., Citation2023). Habit of using screens in bed was thus included as an additional variable in the present study.
Figure 1. Theory used to identify the psychosocial determinants of healthy sleep habits in adults with type 1 and type 2 diabetes. Intention, perceived behavioral control, and habit can predict behavior adoption. Intention is usually predicted by attitudes, perceived norms, and perceived behavioral control. Sociodemographic variables, such as age, biological sex, and type of diabetes, can indirectly affect intention and behavior adoption. Note. PBC: perceived behavioral control, HSH: healthy sleep habits. aHabit was only tested as a determinant of screen use in bed.
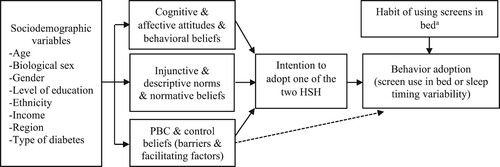
The usefulness of the RAA to predict various health behaviors was confirmed in a meta-analysis (McEachan et al., Citation2016). To our knowledge, only three studies based on the RAA, all conducted among university students, have identified the psychosocial determinants of sleep and intention to adopt HSH (Robbins & Niederdeppe, Citation2015; Tagler et al., Citation2017; Branscum et al., Citation2020). Sleeping 8–9 h/night was predicted by PBC while intention to sleep 8–9 h/night was predicted by attitudes, perceived norms and PBC (Robbins & Niederdeppe, Citation2015). Self-reported (i.e. sleep diary) and objective (i.e. actigraphy) sleep duration were both predicted by intention to sleep at least 8 h/night (Tagler et al., Citation2017). Attitude, perceived norms and PBC predicted intention to adopt HSH (e.g. at least 8 h/night of sleep, consistent bedtimes and wake up times) (Tagler et al., Citation2017). In a last study, intention to sleep 7–9 h/night was predicted by attitudes, perceived norms and PBC (Branscum et al., Citation2020).
The main objective of the present study is to identify psychosocial determinants of HSH among adults with T1D and T2D based on the RAA. The HSH selected were: (1) avoiding screen use in bed, and (2) adopting a regular sleep schedule (i.e. sleep regularity). A secondary objective is to document the HSH of avoiding caffeine, alcohol, and cigarettes before bedtime among adults with diabetes. In our previous pilot study among adults with T1D and T2D, 57.1% of them reported that avoiding screen use in bed and 32.1% that having sleep regularity were HSH they needed to improve, while only 10.7% mentioned this was the case for avoiding caffeine, alcohol, and cigarettes before bedtime (Vézina-Im et al., Citation2023).
Methods
Study population and data collection
The study population consists of French-speaking adults ≥ 18 years of age with T1D or T2D. Pregnant women and adults with a rotating shift were excluded from the study since these factors can influence sleep patterns and ability to have a regular sleep schedule. The project was approved by the Research Ethics Committee of the Centre de recherche du Centre intégré de santé et de services sociaux de Chaudière-Appalaches (number: 2023-965). Informed consent was obtained from all individual participants included in the study. Adults with diabetes were recruited throughout the province of Québec (Canada) from mid-August 2022 to the end of January 2023 using: (1) local diabetes associations’ websites, social media pages and newsletters; (2) the four hospitals from the region of Chaudière-Appalaches (Québec, Canada); and (3) advertisement on a Canadian website promoting healthy sleep (Dormez là-dessus from the Canadian Sleep Society).
Adults with diabetes were invited to complete an anonymous online survey on psychosocial determinants of one of two HSH of their choice (i.e. avoiding screen use in bed or having sleep regularity). Avoiding screen use in bed was defined as avoiding watching television or using a cell phone/smartphone and/or a laptop/tablet in bed, except for electronic devices used to monitor blood glucose levels at night. Sleep regularity was defined as going to bed and waking up with more or less 20–30 min of difference from day to day even on weekends. Avoiding caffeine, alcohol, and cigarettes before bedtime was defined as avoiding beverages that contain caffeine (e.g. coffee, tea, energy drinks, and certain soft drinks), alcohol (e.g. beer, wine or alcoholic beverages), and smoking cigarettes within 4 h of going to bed. Ten CAD$ 100 gift cards at a local bookstore were drawn among participants who completed the entire survey to favor participation.
Screen use in bed as well as sleep timing variability in the last month (not in the last month, less than once a week, 1 or 2 times a week, 3–4 times a week, 5–6 times a week, and every day) were self-reported. The content of both versions of the questionnaire was based on the RAA. The indirect measures (i.e. behavioral beliefs, normative beliefs and control beliefs [barriers and facilitating factors]) were formulated using the modal salient beliefs that were previously identified using a 75% cumulative frequency of mention criterion (Fishbein & Ajzen, Citation2010) in a formative qualitative research conducted among 56 adults with T1D and T2D (Vézina-Im et al., Citation2023) (see Electronic Supplementary Material 1 for the content of both questionnaires). Items were formulated in terms of avoiding screen use in bed and having sleep regularity (i.e. desirable behaviors) as answering a questionnaire on beliefs can lead to behavior change, a phenomenon known as the question-behavior effect (Wilding et al., Citation2016). Items were measured using 5-point, 6-point or 7-point Likert scales (i.e. strongly disagree to strongly agree). Habit of using screens in bed was measured using the short form of the Self-Report Habit Index (Verplanken & Orbell, Citation2003), the most commonly used habit measure (Gardner et al., Citation2011). This short version contains four items (instead of 12) measuring automaticity (i.e. the degree to which a behavior is done automatically) (Gardner et al., Citation2012) as habit is probably more a question of automaticity than frequency of the behavior being performed (Gardner, Citation2012). Questionnaire completion was approximately 15–20 min.
Statistical analyses
Descriptive statistics, including means, standard deviations, and percentages, were computed to describe participants and their HSH. Only one participant identified as non-gendered or non-binary. All the other participants reported similar answers to biological sex and gender (being male and identifying as a man; being female and identifying as a woman). Therefore, biological sex instead of gender was used in all the analyses. Significant differences between the sample of participants who completed the questionnaire on screen use in bed and the one who answered the questionnaire on sleep timing were identified using independent t-test, chi-squared, and Fisher's exact test analyses. The psychometric qualities of the questionnaire were verified by computing Cronbach alpha coefficients (α) (Cronbach, Citation1951) when there was at least three items, and the internal consistency of constructs was classified as adequate using the criteria of Nunnally (Citation1978) (α > 0.70). Spearman correlation coefficients (rs) were computed for variables that had only two items.
A calculation was performed to verify if the sample size was sufficient to perform multivariate linear regression analyses for each HSH. According to the recommendation for the ratio of number of cases to independent variables for multiple regression analyses (Tabachnick & Fidell, Citation2013), a minimum of 50 + 8 (number of predictors) was needed for testing a regression model and a minimum of 104 + number of predictors was needed for testing individual predictors. The present study had five predictors for screen use in bed (intention, PBC, habit, age, and type of diabetes) and 4 predictors for sleep timing (intention, PBC, age, and biological sex). Using the previous formulas, this gives: 50 + 8 (5) = 90 and 104 + 5 = 109 for screen use in bed, and 50 + 8 (4) = 82 and 104 + 4 = 108 for sleep timing. Our final samples of 174 for screen use in bed and 146 for sleep timing were thus sufficient to perform multivariate linear regression analyses.
Hierarchical multivariate linear regression analyses were performed and the models’ percentage of variance explained (R2) was computed for each HSH. The variables entered in the equation for each HSH were based on the RAA recommendations (Fishbein & Ajzen, Citation2010). In a first model for screen use in bed, the variables of the RAA (intention and PBC to avoid screen use in bed) were entered. In a second model, the variable added to the RAA (habit of using screens in bed) was entered. In a third model, sociodemographic variables that were significantly associated with screen use in bed in univariate analyses were entered. In a first model for sleep timing, the variables of the RAA (intention and PBC to have sleep regularity) were entered. In a second model, sociodemographic variables that were significantly associated with having sleep timing variability in univariate analyses were entered. Finally, the significant psychosocial determinants of each HSH were replaced by their underlying beliefs or items to identify the most important beliefs associated with each HSH and obtain information to design targeted interventions (von Haeften et al., Citation2001). The alpha level was set at p < 0.05, and all analyses were performed in SAS version 9.4 (SAS Institute, Cary, NC, USA).
Results
Characteristics of participants and psychometric qualities of questionnaires
A total of 320 participants (174 for screen use in bed and 146 for sleep timing) completed the section on psychosocial determinants of HSH and among those 317 (99.1%) also completed the section on demographic information (172 for screen use in bed and 145 for sleep timing). Participants who answered the questionnaire on screen use in bed were significantly younger, a higher proportion were between 18 and 40 years, more were females and identified as women, and more reported having T1D compared to participants who answered the questionnaire on sleep timing. A very high percentage of adults with T2D reported taking medication to control their diabetes (e.g. metformin, gliclazide). Complete characteristics of participants are presented in .
Table 1. Characteristics of Participants (n = 317).
All constructs of the questionnaires had adequate internal consistency (α = 0.74–0.88) according to the criteria of Nunnally (Citation1978) (α > 0.70) or were significantly correlated if they only had two items (rs = 0.40-0.72; p < 0.0001). The short form of the Self-Report Habit Index (Verplanken & Orbell, Citation2003) (α = 0.85) in the screen use sample also had adequate internal consistency according to the criteria of Nunnally (Citation1978). The mean scores for intention, attitudes (cognitive and affective), perceived norms (injunctive and descriptive norms), and PBC for both HSH were all lower than 4.5 on 7-point scales while the score for descriptive norms was lower than 2.7 on a 5-point scale (0%–100%). This indicates that for both HSH several adults with diabetes had low intention to adopt them; perceived many negative consequences (cognitive attitude) and emotions (affective attitude) if they were to adopt them; perceived low approval if they were to adopt them (injunctive norm) and that few people adopt them (descriptive norm); and had low confidence in their ability to adopt them. Complete psychometric qualities of both questionnaires and mean scores for each construct are presented in Electronic Supplementary Material 1.
Healthy sleep habits and determinants of healthy sleep habits
In terms of screen use in bed, close to 75% of participants reported screen use in bed in the last month and among those who reported screen use in bed, the vast majority mentioned doing this every day. Significantly more participants who answered the questionnaire on screen use in bed reported adopting this unhealthy sleep habit in the last month compared to those who answered the questionnaire on sleep timing. In terms of sleep timing, close to 90% of participants reported sleep timing variability in the last month. Most participants mentioned this happened once or twice a week, regardless of which questionnaire they answered. In terms of caffeine and alcohol consumption, and smoking cigarettes before bedtime, most participants reported not consuming caffeine, alcohol, and smoking cigarettes within 4 h of bedtime, regardless of which questionnaire they answered. Smoking cigarettes before bedtime was by far the least prevalent unhealthy sleep habit, followed by caffeine consumption, and alcohol consumption before bedtime. Detailed adoption of HSH is presented in .
Table 2. Adoption of healthy sleep habits (n = 317).
Screen Use in Bed. In a first model of linear regression (see Model 1 in the first part of ), intention and PBC to avoid screen use in bed were tested as determinants of screen use in bed. Only PBC to avoid screen use in bed was significantly and negatively associated with screen use in bed (p < 0.0001), and this model explained 60% of the variance. In a second model (see Model 2 in the first part of ), habit of using screens in bed was added to the equation. PBC to avoid screen use in bed (p < 0.0001) and habit of using screens in bed (p < 0.0001) were significantly associated with screen use in bed, and this model explained 69% of the variance. In a third model (see Model 3 in the first part of ), age and type of diabetes were added to the equation as they were significantly associated with screen use in bed in univariate analyses. PBC to avoid screen use in bed (p < 0.0001), habit of using screens in bed (p < 0.0001), and age (p = 0.0086) were significantly associated with screen use in bed, and this model explained 71% of the variance. Age was negatively associated with screen use in bed, indicating that younger adults reported more screen use in bed.
Table 3. Psychosocial determinants of each healthy sleep habit.
Sleep Timing. In a first model (see Model 1 in the second part of ), intention and PBC to have sleep regularity were tested as determinants of sleep timing variability. Only PBC to have sleep regularity was significantly and negatively associated with sleep timing variability (p = 0.0004), and this model explained 23% of the variance. In a second model (see Model 2 in the second part of ), age and biological sex were added to the equation as they were significantly associated with sleep timing variability in univariate analyses. PBC to have sleep regularity (p = 0.0004) and biological sex (p = 0.0069) were significantly associated with sleep timing variability, and this model explained 28% of the variance. Being female was positively associated with having sleep timing variability, indicating that females reported more sleep timing variability.
Most important beliefs associated with each healthy sleep habit
Screen Use in Bed. Given that PBC to avoid screen use in bed and habit of using screens in bed were significant psychosocial determinants of screen use in bed, their underlying beliefs and items, respectively, were tested to identify the most important beliefs associated with screen use in bed. The underlying beliefs for PBC are control beliefs which include barriers and facilitating factors. Univariate analyses indicated one barrier and one facilitating factor for PBC to avoid screen use in bed, and three items for habit of using screens in bed, which were all tested for their association with screen use in bed in a first model (see Model 1 in the first part of ). Only one facilitating factor (p = 0.0080) and two items of habit (p < 0.0001 and p = 0.0240) were significantly associated with screen use in bed, and this model explained 59% of the variance. In a second model (see Model 2 in the first part of ), only the significant facilitating factor and the two habit items were entered in the equation, and this model explained 58% of the variance. The significant facilitating factor was: ‘It would be easier to avoid screen use in bed in the next month if I removed alerts on my cell phone/smartphone and/or tablet in the evening’ (p = 0.0068). The two significant habit items were: ‘I use screens in bed automatically’ (p < 0.0001) and ‘I use screens in bed without having to consciously remember’ (p = 0.0027).
Table 4. Most important beliefs associated with each healthy sleep habit.
Sleep Timing. Given that PBC to have sleep regularity was the only significant psychosocial determinant of sleep timing variability, its underlying control beliefs (barriers and facilitating factors) were tested to identify the most important beliefs associated with sleep timing variability. Univariate analyses indicated one barrier and one facilitating factor for PBC to have sleep regularity, which were tested for their association with sleep timing variability in a first model (see Model 1 in the second part of ). Only one barrier (p = 0.0022) was significantly associated with sleep timing variability, and this model explained 6% of the variance. In a second model (see Model 2 in the second part of ), only the significant barrier was entered in the equation, and this model explained 5% of the variance. The significant barrier was: ‘I feel capable of having a regular sleep schedule in the next month even if I have many things to do in the evening (e.g. work, studies, taking care of children)’ (p = 0.0030).
Discussion
The results of the present study suggest that few adults with diabetes adopt HSH. Close to 75% of adults with diabetes mentioned having used screens in bed in the last month, which is similar to another study in which nearly 70% of adults reported mobile device-based electronic social media in bed (Bhat et al., Citation2018). Close to 90% of adults with diabetes indicated that their sleep timing varied by more than 20–30 min in the past month. Sleep variability is associated with risk of T2D in adults (Rosique-Esteban et al., Citation2018), suggesting it might have an impact on glucose levels in adults with diabetes. As anticipated based on the results of our previous formative qualitative research (Vézina-Im et al., Citation2023), fewer adults with diabetes reported caffeine and alcohol consumption, and smoking cigarettes before bedtime. Similar numbers of adults with diabetes reported consuming caffeine (46.1%) and smoking cigarettes (8.6%) before bedtime compared with a study in which 45.0% and 9.2% of adults reported caffeine and nicotine consumption, respectively, before bedtime (Spadola et al., Citation2019). However, more adults with diabetes mentioned consuming alcohol before bedtime (53.4%) compared to adults in that previous study (19.8%) (Spadola et al., Citation2019). A future study may want to investigate if alcohol is used as a sleep aid in adults with diabetes as it is sometime the case in university students (Goodhines et al., Citation2019).
Screen use in bed among adults with diabetes was significantly associated with PBC to avoid screen use in bed. This suggests that the adoption of this HSH is not easy as adults with diabetes are faced with many barriers to overcome, which was also reflected in the low mean score for PBC to avoid screen use in bed. In our previous pilot study, adults with diabetes reported more barriers than facilitating factors (5 vs 2) compared to adults without diabetes (2 vs 3) for avoiding screen use in bed (Vézina-Im et al., Citation2023). Habit of using screens in bed was another significant psychosocial determinant of screen use in bed. This was also echoed in the most important beliefs associated with screen use in bed; adults with diabetes reported using screens in bed automatically and without having to consciously remember. Similarly, in our previous pilot study, adults with diabetes reported that screen use in bed was a ‘habit too ingrained’ (Vézina-Im et al., Citation2023). In the present study, most adults with diabetes who reported screen use in bed mentioned doing this every day. While habit is likely more a question of automaticity than frequency of the behavior being performed (Gardner, Citation2012), the fact that many adults with diabetes mentioned using screens in bed every day might have contributed to making this a habit by strengthening the association between their bed and screen use. Interestingly, in one of our previous studies based on the RAA, habit was not a significant psychosocial determinant of recreational screen use in adolescents (Roussel-Ouellet et al., Citation2022), suggesting that location (in bed) might be a more important trigger than context (leisure time). More research is needed to identify which cues are the most important to develop healthy habits (e.g. HSH) or break undesirable habits (e.g. excessive recreational screen use) (Gardner et al., Citation2023). Similarly to a previous study among adults (Bhat et al., Citation2018), age was negatively associated with screen use in bed among adults with diabetes.
PBC to have a regular sleep schedule was the only significant psychosocial determinant of sleep timing variability in adults with diabetes. The low mean score for PBC to have a regular sleep schedule suggests that adopting this HSH is perceived as difficult. In our previous pilot study, adults with diabetes mentioned more barriers than facilitating factors (5 vs 2) compared to adults without diabetes (4 vs 8) for adopting a regular sleep schedule (Vézina-Im et al., Citation2023). Biological sex was also significantly associated with sleep timing variability. More females with diabetes reported having sleep timing variability compared to males with diabetes. This result is in line with previous studies reporting that females are usually more at risk for short (Grandner et al., Citation2015) and poor sleep quality (Mehta et al., Citation2015; Nugent & Black, Citation2016) compared to males because their sleep can be influenced by biological factors, such as menstrual cycle, pregnancy, and menopause (Mehta et al., Citation2015), and family responsibilities (Maume et al., Citation2010). Feeling capable of overcoming the barrier of having many things to do in the evening – including taking care of children – was the most important belief associated with having sleep timing variability. This suggests that family responsibilities may be a reason why females with diabetes reported having sleep timing variability. The final model on sleep timing variability explained considerably less variance than the model for screen use in bed, perhaps suggesting that extrinsic factors (e.g. work schedule) are important for ability to have sleep regularity.
Intention was not a significant psychosocial determinant of behavior for both HSH. According to the RAA, the adoption of a health behavior should be predicted by intention and PBC when the context is volitional (i.e. individuals have control over behavior adoption) (Fishbein & Ajzen, Citation2010). Previous studies based on the RAA conducted among university students have found mixed results. In one study, sleeping 8–9 h/night was predicted by PBC (Robbins & Niederdeppe, Citation2015) while in another study, sleep duration was predicted by intention to sleep at least 8 h/night (Tagler et al., Citation2017). In the present study, the psychosocial determinants of HSH were identified, since people have control over whether they adopt HSH, but not over when they will fall asleep, how long and how well they will sleep. Our results however suggest that adults with diabetes perceived they have little control over the adoption of both HSH. It seems that adopting HSH was perceived as too challenging, which sapped intention to adopt them. Adults with diabetes reported that lack of motivation or willpower to avoid screen use in bed was a barrier to adopting this HSH. Another potential explanation is that intention might not be a psychosocial determinant of screen use in bed because it was strongly influenced by habit in the present study. Habits are automatic responses to specific cues (Gardner & Rebar, Citation2019) and technically, an individual cannot intend to perform an action done automatically (i.e. without awareness), although there is evidence that intention might predict habitual health behaviors in certain cases (Gardner et al., Citation2015). Changing a behavior that is strongly habitual is most likely difficult and maybe more than developing new healthy habits (Gardner et al., Citation2023).
The most important beliefs associated with each HSH identified in the present study can be used to design targeted interventions (von Haeften et al., Citation2001). Adults with diabetes mentioned that removing alerts on their cell phone/smartphone and/or tablet in the evening might make it easier to avoid screen use in bed. It is most likely that those alerts trigger screen use in bed and that removing those cues might help break the cycle of automaticity, but more research is needed on which behavior change techniques are the most effective at disrupting unhealthy habits (Gardner et al., Citation2023). Replacing screen use in bed by other activities conducive to sleep, such as reading, yoga, and meditation, was recently found to promote HSH in adults (Mohideen et al., Citation2023). Adults with diabetes also mentioned reading a good book before going to sleep as an alternative to screen use in bed in our previous formative qualitative research (Vézina-Im et al., Citation2023). Adults with diabetes who reported feeling capable of overcoming the barrier of having many things to do in the evening were more likely to have sleep regularity. In a previous study based on the RAA, university students mentioned ‘managing my time effectively’ as a facilitating factor for sleeping 8–9 h/night (Robbins & Niederdeppe, Citation2015). This suggests that time management might be useful to encourage adults with diabetes to have sleep regularity. Time management has successfully been used to encourage adults to adopt other health behaviors, such as physical activity and healthy eating (Michie et al., Citation2011). The results of the present study also indicate that younger adults with diabetes should be prioritized for screen use in bed as they reported more using screens in bed, while females with diabetes should be prioritized for sleep timing variability as they reported more having a sleep schedule that varies than their male counterparts.
Strengths and limitations
The present study has a few strengths and limitations. Strengths include: (1) recruiting participants with T1D and T2D as adults with both types of diabetes are at risk for poor sleep quality, shorter sleep duration and insomnia compared to adults without diabetes (Vézina-Im et al., Citation2021); (2) being based on the RAA, a theory whose usefulness to predict various health behaviors was confirmed in a meta-analysis (McEachan et al., Citation2016); (3) conducting formative qualitative research in adults with diabetes (Vézina-Im et al., Citation2023) to develop the content of the questionnaire and verifying the internal consistency of the items according to the criteria of Nunnally (Citation1978); (4) including habit of using screens in bed as an additional variable based on previous results (Aulbach et al., Citation2023; Vézina-Im et al., Citation2023); and (5) identifying the most important beliefs associated with each HSH to obtain information to design targeted interventions (von Haeften et al., Citation2001). Adding habit of using screens in bed led to close to 10% increase in the percentage of variance explained in screen use in bed and the identification of two items of habit that were the most strongly associated with this HSH. The most important limitation of the present study is that it is cross-sectional. A longitudinal design is preferable to identify determinants of behavior. A cross-sectional study was conducted to facilitate recruitment of participants – especially as adults with diabetes are a specific population who might be harder to recruit than university students – and allow participants’ answers to be completely anonymous. Behavior was self-reported, so the presence of a social bias is possible, even though answers were anonymous. Future studies might want to use a combination of self-reported and objective measures of screen use in bed and sleep timing because they can have low concordance (Mahalingham et al., Citation2023) and to overcome limitations associated with both types of measures (Sadeh, Citation2011). Finally, letting adults with diabetes select the HSH on which they wanted to answer questions may have introduced a selection bias. This was to ensure that participants answered a questionnaire on a HSH that is deemed relevant to them, and it allowed us to have data on which HSH adults with diabetes think they should prioritize.
Conclusion
To our knowledge, this is the first study based on the RAA to identify the psychosocial determinants of HSH in adults with diabetes and include habit of using screens in bed as an additional variable. Few adults with diabetes have HSH and the results suggest that they are difficult to adopt. Screen use in bed was strongly influenced by habit. Adults with diabetes, especially younger ones, can remove alerts on their cell phone/smartphone and/or tablet in the evening to break the cycle of automaticity of using screens in bed. Adults with diabetes, especially females, will likely benefit from receiving guidance on effective time management, such as sharing family responsibilities with other family members, to allow them to have a regular sleep schedule. Finally, adults with diabetes should have access to behavior change interventions to encourage them to adopt HSH and prevent complications associated with hyperglycemia resulting from inadequate sleep.
Acknowledgements
The authors want to acknowledge Diabète Québec, Diavie, Marie-Claude Garneau, Liliane Bernier, Marie-Hélène Bélanger, Mathieu Pelletier, Annick Bureau and Monique St-Hilaire of the CISSS de Chaudière-Appalaches, and the people of Dormez là-dessus who helped with recruiting adults with diabetes. We also want to acknowledge the contribution of Dominic Simard of the Université du Québec à Rimouski (UQAR) for his assistance with the online questionnaire. Finally, we want to thank the people who participated in the present study.
Data deposition
The Research Ethics Committee of the Centre de recherche du Centre intégré de santé et de services sociaux (CISSS) de Chaudière-Appalaches did not approve public sharing of our data.
Geolocation information
The study was conducted in the province of Québec (Canada).
Supplemental Material
Download MS Word (23.8 KB)Additional information
Funding
References
- Aulbach, M. B., Konttinen, H., Gardner, B., Kujala, E., Araujo-Soares, V., Sniehotta, F. F., … Hankonen, N. (2023). A dual process model to predict adolescents’ screen time and physical activity. Psychology & Health, 38(7), 827–846.
- Bhat, S., Pinto-Zipp, G., Upadhyay, H., & Polos, P. G. (2018). To sleep, perchance to tweet: In-bed electronic social media use and its associations with insomnia, daytime sleepiness, mood, and sleep duration in adults. Sleep Health, 4(2), 166–173. doi:10.1016/j.sleh.2017.12.004
- Branscum, P., Fay, K. Q., & Senkowski, V. (2020). Do different factors predict the adoption and maintenance of healthy sleep behaviors? A reasoned action approach. Translational Behavioral Medicine, 10(1), 78–86.
- Chaput, J. P., Dutil, C., Featherstone, R., Ross, R., Giangregorio, L., Saunders, T. J., Janssen, I., Poitras, V. J., Kho, M. E., Ross-White, A., Zankar, S., & Carrier, J. (2020). Sleep timing, sleep consistency, and health in adults: A systematic review. Applied Physiology, Nutrition, and Metabolism, 45(10), S232–SS47. doi:10.1139/apnm-2020-0032
- Cronbach, L. J. (1951). Coefficient alpha and the internal structure of tests. Psychometrika, 16(3), 297–334. doi:10.1007/BF02310555
- Drake, C., Roehrs, T., Shambroom, J., & Roth, T. (2013). Caffeine effects on sleep taken 0, 3, or 6 hours before going to bed. Journal of Clinical Sleep Medicine JCSM: Official Publication of the American Academy of Sleep Medicine, 9(11), 1195–1200.
- Fishbein, M., & Ajzen, I. (2010). Predicting and changing behavior: The reasoned action approach. Routledge.
- Gardner, B. (2012). Habit as automaticity, not frequency. The European Health Psychologist, 14(2), 32–36.
- Gardner, B. (2015). A review and analysis of the use of ‘habit’ in understanding, predicting and influencing health-related behaviour. Health Psychology Review, 9(3), 277–295. doi:10.1080/17437199.2013.876238
- Gardner, B., Abraham, C., Lally, P., & de Bruijn, G. J. (2012). Towards parsimony in habit measurement: Testing the convergent and predictive validity of an automaticity subscale of the self-report habit index. The International Journal of Behavioral Nutrition and Physical Activity, 9(102).
- Gardner, B., Arden, M. A., Brown, D., Eves, F. F., Green, J., Hamilton, K., Hankonen, N., Inauen, J., Keller, J., Kwasnicka, D., Labudek, S., Marien, H., Masaryk, R., McCleary, N., Mullan, B. A., Neter, E., Orbell, S., Potthoff, S., & Lally, P. (2023). Developing habit-based health behaviour change interventions: Twenty-one questions to guide future research. Psychology & Health, 38(4), 518–540. doi:10.1080/08870446.2021.2003362
- Gardner, B., Corbridge, S., & McGowan, L. (2015). Do habits always override intentions? Pitting unhealthy snacking habits against snack-avoidance intentions. BMC Psychology, 3(1), 8. doi:10.1186/s40359-015-0065-4
- Gardner, B., de Bruijn, G. J., & Lally, P. (2011). A systematic review and meta-analysis of applications of the self-report habit index to nutrition and physical activity behaviours. Annals of Behavioral Medicine, 42(2), 174–187. doi:10.1007/s12160-011-9282-0
- Gardner, B., & Rebar, A. L. (2019). Habit formation and behavior change. Oxford University Press.
- Goodhines, P. A., Gellis, L. A., Kim, J., Fucito, L. M., & Park, A. (2019). Self-medication for sleep in college students: Concurrent and prospective associations with sleep and alcohol behavior. Behavioral Sleep Medicine, 17(3), 327–341. doi:10.1080/15402002.2017.1357119
- Grandner, M. A., Jackson, N. J., Izci-Balserak, B., Gallagher, R. A., Murray-Bachmann, R., Williams, N. J., Patel, N. P., & Jean-Louis, G. (2015). Social and behavioral determinants of perceived insufficient sleep. Frontiers in Neurology, 6(112).
- Lee, S. W. H., Ng, K. Y., & Chin, W. K. (2017). The impact of sleep amount and sleep quality on glycemic control in type 2 diabetes: A systematic review and meta-analysis. Sleep Medicine Reviews, 31, 91–101. doi:10.1016/j.smrv.2016.02.001
- Mahalingham, T., McEvoy, P. M., & Clarke, P. J. F. (2023). Assessing the validity of self-report social media use: Evidence of no relationship with objective smartphone use. Computers in Human Behavior, 140, 107567.
- Maume, D. J., Sebastian, R. A., & Bardo, A. R. (2010). Gender, work-family responsibilities, and sleep. Gender & Society, 24(6), 746–768. doi:10.1177/0891243210386949
- McEachan, R., Taylor, N., Harrison, R., Lawton, R., Gardner, P., & Conner, M. (2016). Meta-analysis of the reasoned action approach (RAA) to understanding health behaviors. Annals of Behavioral Medicine, 50(4), 592–612. doi:10.1007/s12160-016-9798-4
- Mehta, N., Shafi, F., & Bhat, A. (2015). Unique aspects of sleep in women. Missouri Medicine, 112(6), 430–434.
- Michie, S., Ashford, S., Sniehotta, F. F., Dombrowski, S. U., Bishop, A., & French, D. P. (2011). A refined taxonomy of behaviour change techniques to help people change their physical activity and healthy eating behaviours: The CALO-RE taxonomy. Psychology & Health, 26(11), 1479–1498. doi:10.1080/08870446.2010.540664
- Michie, S., Atkins, L., & West, R. (2014). The behaviour change wheel: A guide to designing interventions. Silverback Publishing.
- Mohideen, A., Bouvin, C., Judah, G., Picariello, F., & Gardner, B. (2023). Feasibility and acceptability of a personalised script-elicitation method for improving evening sleep hygiene habits. Health Psychology and Behavioral Medicine, 11(1), 2162904. doi:10.1080/21642850.2022.2162904
- Nugent, C. N., & Black, L. I. (2016). Sleep duration, quality of sleep, and use of sleep medication, by sex and family type, 2013–2014. NCHS Data Brief, 230, 1–8.
- Nunnally, J. C. (1978). Psychometric theory (2nd ed). McGraw-Hill.
- Reutrakul, S., Thakkinstian, A., Anothaisintawee, T., Chontong, S., Borel, A. L., Perfect, M. M., Silva Janovsky, C. C. P., Kessler, R., Schultes, B., Harsch, I. A., van Dijk, M., Bouhassira, D., Matejko, B., Lipton, R. B., Suwannalai, P., Chirakalwasan, N., Schober, A. K., & Knutson, K. L. (2016). Sleep characteristics in type 1 diabetes and associations with glycemic control: Systematic review and meta-analysis. Sleep Medicine, 23, 26–45. doi:10.1016/j.sleep.2016.03.019
- Robbins, R., & Niederdeppe, J. (2015). Using the integrative model of behavioral prediction to identify promising message strategies to promote healthy sleep behavior among college students. Health Communication, 30(1), 26–38. doi:10.1080/10410236.2013.835215
- Rosique-Esteban, N., Papandreou, C., Romaguera, D., Warnberg, J., Corella, D., Martínez-González, M., … Salas-Salvadó, J. (2018). Cross-sectional associations of objectively-measured sleep characteristics with obesity and type 2 diabetes in the PREDIMED-plus trial. Sleep, 41(12), 1–10. doi:10.1093/sleep/zsy190
- Roussel-Ouellet, J., Beaulieu, D., Vézina-Im, L. A., Turcotte, S., Labbé, V., & Bouchard, D. (2022). Psychosocial correlates of recreational screen time among adolescents. International Journal of Environmental Research and Public Health, 19(24). doi:10.3390/ijerph192416719
- Sadeh, A. (2011). The role and validity of actigraphy in sleep medicine: An update. Sleep Medicine Reviews, 15(4), 259–267. doi:10.1016/j.smrv.2010.10.001
- Spadola, C. E., Guo, N., Johnson, D. A., Sofer, T., Bertisch, S. M., Jackson, C. L., … Redline, S. (2019). Evening intake of alcohol, caffeine, and nicotine: Night-to-night associations with sleep duration and continuity among African Americans in the jackson heart sleep study. Sleep, 42(1), zsz136.
- Tabachnick, B. G., & Fidell, L. S. (2013). Using multivariate statistics (6th ed). Pearson Education.
- Tagler, M. J., Stanko, K. A., & Forbey, J. D. (2017). Predicting sleep hygiene: A reasoned action approach. Journal of Applied Social Psychology, 47(1), 3–12. doi:10.1111/jasp.12411
- Verplanken, B., & Orbell, S. (2003). Reflections on past behavior: A self-report index of habit strength. Journal of Applied Social Psychology, 33(6), 1313–1330. doi:10.1111/j.1559-1816.2003.tb01951.x
- Vézina-Im, L. A., Morin, C. M., & Desroches, S. (2021). Diet and physical activity among adults living with type 1 and type 2 diabetes. Canadian Journal of Diabetes, 45(7), 659–665. doi:10.1016/j.jcjd.2021.01.013
- Vézina-Im, L. A., Morin, C. M., Lapointe, A., & Desroches, S. (2023). Beliefs about healthy sleep habits in adults with diabetes compared to adults without diabetes: A reasoned action approach elicitation study. Sleep Science, 15(1), 14–28.
- von Haeften, I., Fishbein, M., Kasprzyk, D., & Montano, D. (2001). Analyzing data to obtain information to design targeted interventions. Psychology, Health & Medicine, 6(2), 151–164. doi:10.1080/13548500125076
- Wilding, S., Conner, M., Sandberg, T., Prestwich, A., Lawton, R., Wood, C., Miles, E., Godin, G., & Sheeran, P. (2016). The question-behaviour effect: A theoretical and methodological review and meta-analysis. European Review of Social Psychology, 27(1), 196–230. doi:10.1080/10463283.2016.1245940