Abstract
The objective of this study was to find common immune mechanism across different kinds of vaccines. A meta-analysis of microarray datasets was performed using publicly available microarray Gene Expression Omnibus (GEO) and Array Express data sets of vaccination records. Seven studies (out of 35) were selected for this meta-analysis. A total of 447 chips (145 pre-vaccination and 302 post-vaccination) were included. Significance analysis of microarrays (SAM) program was used for screening differentially expressed genes (DEGs). Functional pathway enrichment for the DEGs was conducted in DAVID Gene Ontology (GO) database. Twenty DEGs were identified, of which 10 up-regulated genes involved immune response. Six of which were type I interferon (IFN) related genes, including LY6E, MX1, OAS3, IFI44L, IFI6 and IFITM3. Ten down-regulated genes mainly mediated negative regulation of cell proliferation and cell motion. Results of a subgroup analysis showed that although the kinds of genes varied widely between days 3 and 7 post vaccination, the pathways between them are basically the same, such as immune response and response to viruses, etc. For an independent verification of these 6 type I IFN related genes, peripheral blood mononuclear cells (PBMCs) were collected at baseline and day 3 after the vaccination from 8 Enterovirus 71(EV71) vaccinees and were assayed by RT-PCR. Results showed that the 6 DEGs were also upregulated in EV71 vaccinees. In summary, meta-analysis methods were used to explore the immune mechanism of vaccines and results indicated that the type I IFN related genes and corresponding pathways were common in early immune responses for different kinds of vaccines.
Abbreviations:
- GEO, Gene Expression Omnibus
- SAM, significance analysis of microarrays
- DEGs, differentially expressed genes
- GO, gene ontology
- IFN, interferon
- CPE, cytopathogenic effect
- PBMCs, peripheral blood mononuclear cells
- EV71, enterovirus 71
- PRRs, pattern recognition receptors
- TLRs, Toll-like receptors
- DCs, dendritic cells
Introduction
Vaccines have played an important role in controlling infectious diseases, but little is known about the mechanisms by which vaccines induce effective immune responses.Citation1 High throughput technology such as microarray has been applied to explore the immune mechanisms of different kinds of vaccines, such as inactivated vaccines, live attenuated vaccines and virus-like particle vaccines.Citation2-4 While individual microarray study can be informative in identifying genes or significant biological pathways of a single vaccine, it is still difficult to obtain a comprehensive understanding of vaccine immune mechanisms since kinds of vaccines, microarray platforms and analysis methods differed appreciably. Meta analysis can increase the reliability and generalizability of results and obtain a more precise estimate of gene expression through combining information from multiple existing studies.Citation5
Recent advances of the early immune response after vaccination (frequently termed the ‘innate response’) are beginning to reveal the fundamental mechanisms by which the innate immune orchestrates protective immune responses to vaccinations.Citation6,7 The innate immune system is capable of sensing viruses and bacteria through the expression of pattern recognition receptors (PRRs), such as Toll-like receptors (TLRs), C type lectin like receptors and retinoicacid inducible gene I (RIG-I) like receptors, which are expressed by dendritic cells (DCs) and other cells of the innate system.Citation8 Emerging evidence suggests that early immune response such as the nature of the DC subtype, as well as the particular PRR triggered, plays a critical role in regulating the magnitude, quality, and duration of the later adaptive immune responses.Citation9 Specific signatures of innate activation may indicate that the vaccines induced the appropriate quality and sufficient strength of activation to induce protective acquired immunity.Citation1
A better understanding of the early immune mechanisms would be of value in designing new vaccines and screening the biomarkers for predicting the immunogenicity of vaccines.Citation1 The main body of the present study is a meta-analysis of 7 published studies. The goal was to find common immune mechanism across different kinds of vaccines and provide the foundation for exploring the common biomarkers for predicting the immunogenicity of vaccines. Samples from subjects vaccinated with EV71 vaccines were used to validate the result of meta-analysis. To do this, we performed meta-analysis, which combined gene expression data from 7 individual studies on a sample level.Citation10 In different studies, blood samples were collected at a few strategic time points (e.g. 0, 3, 7, 21, 28 and 56 d after vaccination).Citation2-4,11 In this study, we defined within 7 d as early immune response. Although meta-analysis has been applied to identify gene expression signatures in different diseases,Citation12-21 it has not yet, to our knowledge, been applied to vaccine immune mechanisms.
Results
The characteristics of the included studies
Thirty-five potentially relevant articles (1708 datasets) were identified through searching the published literature. Five vaccine-realated microarray expression profile articles from PBMC or whole blood, representing 6 separate vaccines were aggregated for the meta-analysis, according to the procedure described in . A summary of the studies included in the meta-analysis is shown in . Of the 5 included articles (6 kinds of vaccines), 2 from influenza vaccines, 2 from yellow fever vaccines, 1 from the malaria vaccine and 1 from the pneumovax vaccine. Seven studies were included in 5 articles. Among them, 2 microarray testing platforms were used in research from Nakaya HI, et al., which was regarded as 2 studies. Research from Obermoser, et al. included 2 vaccines (pneumovax and influenza), which also were taken as 2 studies. A total of 447 (including 145 pre-vaccination and 302 post-vaccination) microarray chips were included in this study. Raw data from 7 studies were pre-processed for background correction and normalization (Materials and Methods 2), which achieved a standardized assessment on different data.
Table 1. Characteristics of studies included in the meta-analysis
Identifying DEGs in the early immune response of vaccine
Following meta-analysis of the combined data sets, a total of 20 DEGs were identified, of which 10 genes were up-regulated and 10 genes were downregulated. A list of DEGs is presented in and . The prominent finding of this analysis was that most of upregulated genes are type I IFN related genes, including LY6E, MX1, IFI44L, IFI6, OAS3 and IFITM3 (). The up-regulated gene LY6E played a role in the activation and maturation of T and B immune cells.Citation24,25 MX1, OAS3 and IFITM3 were mainly involved in antiviral response.Citation26-28 FI44L and IFI6 mediated apoptosis and cell cycle.Citation29-30 While the down-regulated genes were involved with metabolic enzymes, inflammation, chemokines, cell cycle and receptor related genes ().
Table 2. Differentially expressed up-regulated genes within 7d after vaccination using meta-analysis
Table 3. Differentially expressed down-regulated genes within 7d after vaccination using meta-analysis
Functional pathway enrichment analysis
To identify the biological processes associated with gene expression changes in vaccines, we performed GO analysis with the DEGs. Seven significant enrichments of the DEGs were identified (). Upregulated genes were involved in immune response pathway. Six pathways of downregulated genes mainly mediated negative regulation of cell proliferation and cell motion.
Table 4. The pathway distribution of DEGs within 7d after vaccination
The subgroup analysis based on days 3 and 7 after vaccination
Since the reported studies mainly involved blood samples collected on days 3 and 7 after vaccination, a subgroup analysis was performed for days 3 and 7 post vaccination. The results showed there were 36 up-regulated DEGs 3 d after vaccination. The largest category contained genes with a clear role in immune response, such as C1QA, C1QB, IFITM3 and IFI44L. Other notable categories included genes in the defense response and inflammatory response. Twenty genes were upregulated 7 d after vaccination, 10 of which were immune response genes including IgJ, OAS3, RSAD2 and IFI44L; some genes were involved in response to virus including ISG15 and MX1, and some genes mediated defense responses such as CCL4 and DHX58. A list of the up-regulated DEGs are presented in Table S1 and 2. Four genes were commonly upregulated between days 3 and 7 after vaccination (IFI44L, RSAD2, XAF1 and OAS3) (), among them 2 were type I IFN related genes. In addition, the specific up-regulated genes in 3d mainly involved in complement related genes,such as C1QA and C1QB, while the specific genes in 7d mainly included IgJ and RRM2.
Figure 2. Number of DEGs with upregulation on days 3 and 7 after vaccination. Note:There were 36 upregulated genes in 3 days, including 32 specific genes and there were 20 up-regulated genes in 7 days, including 16 specific genes. Four genes were commonly upregulated between days 3 and 7 after vaccination, among them 2 were type I IFN related genes.
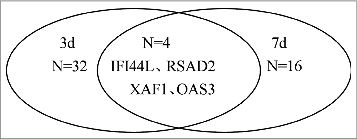
Validation results of type I IFN related genes
Six type I IFN related genes were validated with EV71 vaccinees that did not belong to any part of the meta-analysis. The neutralizing antibody of EV71 vaccinees was tested by cytopathogenic effect (CPE)method. These vaccinees were selected when their neutralizing antibody titer was negative (﹤1:8) before vaccination but positive after vaccination (Table S3). PBMC of 0 and 3 d after vaccination from these EV71 vaccinees were detected by RT-PCR. The results showed that the 6 DEGs of meta-analysis were also upregulated in EV71 vaccinees, as displayed in .
Figure 3. The meta-analysis and EV71 vaccinated group validation results of 6 type I IFN related genes. Note: PBMC for baseline and day 3 after the vaccination from 8 EV71 vaccinees were assayed by RT-PCR. FC of each gene was the arithmetic average of the expression of 3 day post-vaccination/ 0 day pre-vaccination. FC greater than 1.5 is shown in red in the figure.
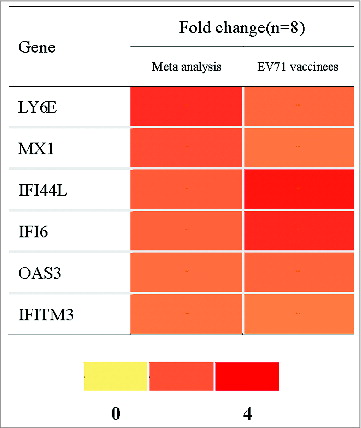
Discussion
The application of high-throughput technology such as microarray provides an opportunity to fully explore the immune mechanisms of different kinds of vaccines.Citation2-4,31 There was a long vaccination history of yellow fever vaccine and influenza vaccine. They have been confirmed to have a good protective effect. Using microarray technology, in yellow fever vaccine, B cell growth factor TNFRS17 predicted the neutralizing antibody response with up to 100% accuracy and in trivalent inactivated influenza vaccine,12 sets of genes containing 2 to 4 genes each predicted the neutralizing antibody response with over 90% accuracy. These studies provided foundation for the evaluation of vaccines. In this study, we focused on the common genes and pathways of early immune response to vaccinations using meta-analysis which allows for an analysis to reveal additional information that would not be apparent in the original studies used. Ten up-regulated genes were obtained from meta-analysis, of which 6 were type I IFN induced genes that were validated by EV71 vaccinees, which suggested that type I IFN played an important role in the early immune response of vaccines. Type I IFN is an important antiviral factor, and its defense response against the virus is primarily through signal transduction and transcription activation of pathways, resulting in a series of interferon-regulated genes expression, generating many enzymes and proteins directly acting on the invasive viruses, thereby protecting the body from infection.Citation32-43 Some viruses obtain antiviral ability of escaping type I IFN in the evolution, by binding to the IFN receptor, affecting type I IFN promoter activity, blocking Jak-STAT signaling pathway, up-regulating the negative regulatory factor of the host cells, and other ways of blocking the killing effect of type I IFN on the viruses.Citation44-48 In addition, type I IFN has multiple immunomodulatory effects, and it is an important cellular signaling molecule which controls the DC cell differentiation and function. In the presence of IFN-α, monocyte-derived DC gradually matures and plays specific functions of stimulating CD4+ and CD8+ T cells.Citation49 Type I IFN can promote T and B cell differentiation and maturation.Citation50,51
In the immune response to vaccines, type I IFN was the necessary signaling molecule producing the antibody after measles vaccination, which showed that type I IFN was directly related to antibody production.Citation52 Research from Jego et al.Citation53 on influenza viruses supported this conclusion. Through secreting type I IFN, pDC induced the activation of CD40 molecule on the surface of B cell, inducing B cell differentiating into plasma cell and producing specific IgG. Type I IFN multiple immunomodulatory effects suggest that type I IFN may be a necessary factor for the success of the vaccines. Together with the results of our meta-analysis, it suggested that type I IFN would play a crucial role in the design of vaccines.
The innate immune system is able to sense microbial or viral stimuli by the expression of PRRs, activating specific signaling pathways to drive immunological responses.Citation1 Among the PRRs, a key role is played by TLRs. Live attenuated yellow fever vaccines have been demonstrated to activate multiple DC subsets via TLRs 2, 7, 8, and 9,Citation3 while inactivated influenza vaccines are likely to activate TLR5.Citation2 Pneumovax vaccines initiate innate immune through activating TLR4, TLR5, TLR6, and TLR8.Citation22 TLRs signaling pathways are the primary cause of producing type I IFN, which may be a proof for the universality of type I IFN in vaccine immune response.
We found that although the kinds of genes varied widely between days 3 and 7 after vaccination, the pathways between them are basically the same in relation to immune response and response to virus. The specific genes of 3d mainly included complement, such as C1QA. It was reported that C1QA played an important role in the innate immune response. C1QA can up-regulate RIG-I mediated activation of IFN-stimulated responsive element (ISRE) and nuclear factorκB reporters and type I IFN transcription, leading to the production of type I IFNs.Citation54 The specific genes of 7d mainly included IgJ. The J chain protein encoded by IgJ links together IgM or IgA monomers to form pentameric IgM or dimeric IgA. J chain expression in B cells is related to gene activation in the B lymphocyte and IgJ played an important role in antibody production. Citation55 It suggested acquired immune response had been initiated by 7 d post vaccination.
Although adjuvant can activate the pattern recognition receptors signal pathway, inducing the expression of innate immune genes, the impact of adjuvants on the result of meta-analysis was negligible in this study. Because 6 out of 7 studies were adjuvant-free, and the amount of subjects inoculated with adjuvant-containing vaccine was less than 10% of total subjects ().
In summary, using meta-analysis, we found the common genes and pathways of early stage after vaccination is type I IFN related genes and pathways. This argument is further validated through the examination of EV71 vaccinees using RT-PCR. Further application of this discovery may be of interest to the design of new vaccines and the screening of predictors of vaccine immunogenicity.
Materials and Methods
Studies included in the meta-analysis
Human studies of vaccine immune mechanisms using microarrays published available on the National Center for Biotechnology Information (NCBI) GEO database (http://www.ncbi.nlm.nih.gov/geo/) and ArrayExpress (http://www.ebi.ac.uk/arrayexpress/) were reviewed for inclusion. We searched the 2 databases using the Medical Subject Heading (MeSH) terms “vaccines,” “Homo sapiens,” “PBMC,” and “whole-blood." In addition, we performed manual searches by scanning the reference lists of the included articles to locate additional papers related to the topic. Data were included in the meta analysis if they met the followed criteria:(1) The selected dataset must be the microarray data of genome-wide expression of mRNA (2) The data was the test results of pre-vaccination (0 days) and post-vaccination (within 7days,including 0.5, 1, 1.5, 3, 6 and 7d in this study) (3) Original data sets or data of known standardization method were considered (4) The selected data set must include more than 3 samples (5) Data sets came from the published literature for years 2008–2013. Furthermore, we excluded samples using raw data from in vitro re-stimulation. Based on these criteria, we included 6 vaccines from 5 studies. Data identification and acquisition are shown in .
Pre-processing raw gene expression data
A CEL data compression package of gene microarray was downloaded in the GEO supplementary file. If arrays did not provide the CEL original packet, then raw data in TXT format of the dataset was downloaded. Arrays from the Affymetrix platform were RMA-preprocessed in R56 and BioConductor release 2.12Citation57 and arrays from Illumina platform were LOESS-normalized with D-chip.Citation58 Extraction of probe level data, background correction, and normalization were performed for each individual experiment. The expression values were on log base 2 scale.
Single sample data consolidation
Probe sets identifiers were converted to gene symbols using probe set annotation data downloaded from the Affymetrix website(http://www.affymetrix.com/support/mas/index.affx)and GEO database. The expression date sheet in which the probe number, gene symbol and gene name corresponding to the sample were established.
Each sample was sequenced by "gene name" key words. Blank genes were removed from the gene pool, and then the "combined calculation" in Excel was applied to the corresponding values of the same genes for the calculation of the average.
Data calculations and statistics
The meta-analysis of public data was performed using consolidation strategy on the sample level. The statistical work was performed by using SAM software. Microarray expression data were organized, managed and calculated using Microsoft Excel. First, identical genes corresponding to 5 samples sets were screened out (10706). Array data (447) were merged to form a single large data table of a matrix of 447 × 10706. Data was imported to D-chip software for re-standardization and parameters were default values. Then SAM software based on Student's t-tests were used for meta-analysis.Citation59 DEGs were screened out, using default settings and selecting genes where fold change (FC) ≥1 .5 and false discovery rate (FDR), q ≤ 0 .05.
Gene Ontology enrichment analysis of DEGs
Functional annotation and GO termed enrichment analysis of the DEGs was performed using the DAVID website(http://david.abcc.ncifcrf.gov/). For GO-term enrichment, the functional annotation chart option was used, with default settings and the Fisher's Exact test, selecting pathways where p ≤ 0 .05. DEGs with up-regulation and down-regulation were analyzed separately.
Subgroup analysis of 3 and 7d post-immunization
The five data sets of 3d post-immunization and 4 datasets of 7d post-immunization were subject to R and DNA-chip pretreatment, as well as DEGs analysis with SAM.
Validation of type I IFN related genes
Samples
Eight blood samples were obtained from EV71 vaccinees (Clinical Trials number NCT01569581): at baseline, and at days 3,28 and 56 post-vaccination (Table S3). PBMC and plasma were isolated from fresh blood(Vacutainer® with EDTA; BD), following the manufacturer's protocol. PBMCs were frozen in DMSO with 90% FBS and stored at −80°C. Plasma samples were stored at −20°C.
CPE assays for the detection of neutralizing antibody against EV71
Classical CPE assays were employed to measure neutralizing antibody against EV71.Citation60 Plasma samples were inactivated at 56°C for 30 minutes, serially diluted fold2- from 1:8 and mixed with equal volumes of TCID50 of a EV71 strain. The mixture was dispensed into a 96-well microplate and incubated at 37°C for 2 hours. RD cells (1–2 ×105 cells/mL) were added to the mixture. The plates were then placed in a CO2 incubator at 35°C for 7 d CPE was observed by microscopy. EV71 national standards were included in each test as a control for the reproducibility of the results.Citation61 Neutralizing antibody titers of EV71 were defined as the dilution rate showing 50% inhibition of the CPE.
Real-time RT-PCR
The gene expression levels were measured by real-time RT-PCR, as previously described.Citation62 The extraction of total RNA from the PBMCs were performed using a RNA extraction kit (Qiagen, GmBH, Germany) according to the manufacturer's instructions. Total RNA was reverse transcribed into cDNA by PrimeScript™ RT reagent Kit with gDNA Eraser(Takara Bio, Japan). RT-PCR was performed according to the manufacturers protocols, using the SYBR® Premix Dimer Eraser™ (Takara Bio, Japan) in a 7500 Fast Real-time RT-PCR system (Applied Biosystems, Foster City, CA, USA). The primers are listed in Table S4, and the dual internal references are GAPDH and β-actin. The fold change for relative quantification of each gene expression of day 3 post-vaccination relative to that of day 0 was calculated by the 2−ΔΔCt method using GAPDH as internal reference.
Disclosure of Potential Conflicts of Interest
No potential conflicts of interest were disclosed.
Supplemental Material
Supplemental data for this article can be accessed on the pub-lisher's website
Supplemental_Tables.zip
Download Zip (169.9 KB)Funding
This work was supported by the National 12th Five Major Special Projects Funding Program (No. 2012ZX10004701) and National High Technology Research and Development Program (“863” program, No. 2012AA02A402) from the Ministry of Science and Technology of the People's Republic of China.
References
- Pulendran B, Li S, Nakaya HI. Systems vaccinology. Immunity 2010; 33:516-29; PMID:21029962; http://dx.doi.org/10.1016/j.immuni.2010.10.006
- Nakaya HI, Wrammert J, Lee EK, Racioppi L, Marie-Kunze S, Haining WN, Means AR, Kasturi SP, Khan N, Li GM, et al. Systems biology of vaccination for seasonal influenza in humans. Nat Immunol 2011; 12:786-95; PMID:21743478; http://dx.doi.org/10.1038/ni.2067
- Querec TD, Akondy RS, Lee EK, Cao W, Nakaya HI, Teuwen D, Pirani A, Gernert K, Deng J, Marzolf B, et al. Systems biology approach predicts immunogenicity of the yellow fever vaccine in humans. Nat Immunol 2009; 10:116-25; PMID:19029902; http://dx.doi.org/10.1038/ni.1688
- Gaucher D, Therrien R, Kettaf N, Angermann BR, Boucher G, Filali-Mouhim A, Moser JM, Mehta RS, Drake DR, 3rd, Castro E, et al. Yellow fever vaccine induces integrated multilineage and polyfunctional immune responses. J Exp Med 2008; 205:3119-131; PMID:19047440; http://dx.doi.org/10.1084/jem.20082292
- Ramasamy A, Mondry A, Holmes CC, Altman DG. Key issues in conducting a meta-analysis of gene expression microarray datasets. PLoS Med 2008; 5:e184; PMID:18767902
- Pulendran B, Ahmed R. Translating innate immunity into immunological memory: implications for vaccine development. Cell 2006; 124:849-63; PMID:16497593; http://dx.doi.org/10.1016/j.cell.2006.02.019
- Steinman RM. Dendritic cells in vivo: a key target for a new vaccine science. Immunity 2008, 29:319-24; PMID:18799140; http://dx.doi.org/10.1016/j.immuni.2008.08.001
- Coffman RL, Sher A, Seder RA. Vaccine adjuvants: putting innate immunity to work. Immunity 2010; 33:492-503; PMID:21029960; http://dx.doi.org/10.1016/j.immuni.2010.10.002
- Iwasaki A, Medzhitov R. Regulation of adaptive immunity by the innate immune system. Science 2010; 327:291-5; PMID:20075244; http://dx.doi.org/10.1126/science.1183021
- Campain A, Yang YH. Comparison study of microarray meta-analysis methods. BMC Bioinformatics 2010; 11:408-18; PMID:20678237; http://dx.doi.org/10.1186/1471-2105-11-408
- Garcia-Pineres AJ, Hildesheim A, Dodd L, Kemp TJ, Yang J, Fullmer B, Harro C, Lowy DR, Lempicki RA, Pinto LA. Gene expression patterns induced by HPV-16 L1 virus-like particles in leukocytes from vaccine recipients. J Immunol 2009; 182:1706-29; PMID:19155521; http://dx.doi.org/10.4049/jimmunol.182.3.1706
- Rhodes DR, Barrette TR, Rubin MA, Ghosh D, Chinnaiyan AM. Meta-analysis of microarrays: interstudy validation of gene expression profiles reveals pathway dysregulation in prostate cancer. Cancer Res 2002; 62:4427-33; PMID:12154050
- Lee HK, Hsu AK, Sajdak J, Qin J, Pavlidis P. Coexpression analysis of human genes across many microarray data sets. Genome Res 2004; 14:1085-94; PMID:15173114; http://dx.doi.org/10.1101/gr.1910904
- Pilarsky C, Wenzig M, Specht T, Saeger HD, Grutzmann R. Identification and validation of commonly overexpressed genes in solid tumors by comparison of microarray data. Neoplasia 2004; 6:744-50; PMID:15720800; http://dx.doi.org/10.1593/neo.04277
- Rhodes DR, Yu J, Shanker K, Deshpande N, Varambally R, Ghosh D, Barrette T, Pandey A, Chinnaiyan AM. Large-scale meta-analysis of cancer microarray data identifies common transcriptional profiles of neoplastic transformation and progression. Proc Natl Acad Sci U S A 2004; 101:9309-14; PMID:15184677; http://dx.doi.org/10.1073/pnas.0401994101
- Wang J, Coombes KR, Highsmith WE, Keating MJ, Abruzzo LV. Differences in gene expression between B-cell chronic lymphocytic leukemia and normal B cells: a meta-analysis of three microarray studies. Bioinformatics 2004; 20:3166-78; PMID:15231529; http://dx.doi.org/10.1093/bioinformatics/bth381
- Grutzmann R, Boriss H, Ammerpohl O, Luttges J, Kalthoff H, Schackert HK, Kloppel G, Saeger HD, Pilarsky C. Meta-analysis of microarray data on pancreatic cancer defines a set of commonly dysregulated genes. Oncogene 2005; 24:5079-88; PMID:15897887; http://dx.doi.org/10.1038/sj.onc.1208696
- Mehra R, Varambally S, Ding L, Shen R, Sabel MS, Ghosh D, Chinnaiyan AM, Kleer CG. Identification of GATA3 as a breast cancer prognostic marker by global gene expression meta-analysis. Cancer Res 2005; 65:11259-64; PMID:16357129; http://dx.doi.org/10.1158/0008-5472.CAN-05-2495
- Bianchi F, Nuciforo P, Vecchi M, Bernard L, Tizzoni L, Marchetti A, Buttitta F, Felicioni L, Nicassio F, Di Fiore PP. Survival prediction of stage I lung adenocarcinomas by expression of 10 genes. J Clin Invest 2007; 117:3436-44; PMID:17948124; http://dx.doi.org/10.1172/JCI32007
- Kim SY, Kim JH, Lee HS, Noh SM, Song KS, Cho JS, Jeong HY, Kim WH, Yeom YI, Kim NS et al. Meta- and gene set analysis of stomach cancer gene expression data. Mol Cells 2007; 24:200-9; PMID:17978572
- Silva GL, Junta CM, Mello SS, Garcia PS, Rassi DM, Sakamoto-Hojo ET, Donadi EA, Passos GA. Profiling meta-analysis reveals primarily gene coexpression concordance between systemic lupus erythematosus and rheumatoid arthritis. Ann N Y Acad Sci 2007; 1110:33-46; PMID:17911418; http://dx.doi.org/10.1196/annals.1423.005
- Obermoser G, Presnell S, Domico K, Xu H, Wang Y, Anguiano E, Thompson-Snipes L, Ranganathan R, Zeitner B, Bjork A, et al. Systems scale interactive exploration reveals quantitative and qualitative differences in response to influenza and pneumococcal vaccines. Immunity 2013; 38:831-44; PMID:23601689; http://dx.doi.org/10.1016/j.immuni.2012.12.008
- Vahey MT, Wang Z, Kester KE, Cummings J, Heppner DG, Jr., Nau ME, Ofori-Anyinam O, Cohen J, Coche T, Ballou WR, et al. Expression of genes associated with immunoproteasome processing of major histocompatibility complex peptides is indicative of protection with adjuvanted RTS,S malaria vaccine. J Infect Dis 2010; 201:580-9; PMID:20078211; http://dx.doi.org/10.1086/650310
- Palfree RG, LeClair KP, Bothwell A, Hammerling U. cDNA characterization of an Ly-6.2 gene expressed in BW5147 tumor cells. Immunogenetics 1987; 26:389-91; PMID:2822573; http://dx.doi.org/10.1007/BF00343712
- Codias EK, Malek TR. Regulation of B lymphocyte responses to IL-4 and IFN-gamma by activation through Ly-6A/E molecules. J Immunol 1990; 144:2197-204; PMID:2107254
- Henrik Gad H, Paulous S, Belarbi E, Diancourt L, Drosten C, Kummerer BM, Plate AE, Caro V, Despres P. The E2-E166K substitution restores Chikungunya virus growth in OAS3 expressing cells by acting on viral entry. Virology 2012; 434:27-37; PMID:22889614
- Schoggins JW, Wilson SJ, Panis M, Murphy MY, Jones CT, Bieniasz P, Rice CM. A diverse range of gene products are effectors of the type I interferon antiviral response. Nature 2011; 472:481-5; PMID:21478870; http://dx.doi.org/10.1038/nature09907
- Xiao H, Killip MJ, Staeheli P, Randall RE, Jackson D. The human interferon-induced MxA protein inhibits early stages of influenza A virus infection by retaining the incoming viral genome in the cytoplasm. J Virol 2013; 87:13053-8; PMID:24049170; http://dx.doi.org/10.1128/JVI.02220-13
- Bloch DB, Li P, Bloch EG, Berenson DF, Galdos RL, Arora P, Malhotra R, Wu C, Yang W. LMKB/MARF1 localizes to mRNA processing bodies, interacts with Ge-1, and regulates IFI44L gene expression. PLoS One 2014; 9:e94784; PMID:24755989; http://dx.doi.org/10.1371/journal.pone.0094784
- Park GH, Kim KY, Cho SW, Cheong JY, Yu GI, Shin DH, Kwack KB. Association between Interferon-Inducible Protein 6 (IFI6) Polymorphisms and Hepatitis B Virus Clearance. Genomics Inform 2013; 11:15-23; PMID:23613678; http://dx.doi.org/10.5808/GI.2013.11.1.15
- Garcia-Pineres AJ, Hildesheim A, Dodd L, Kemp TJ, Yang J, Fullmer B, Harro C, Lowy DR, Lempicki RA, Pinto LA. Gene expression patterns induced by HPV-16 L1 virus-like particles in leukocytes from vaccine recipients. J Immunol 2009; 182:1706-29; PMID:19155521; http://dx.doi.org/10.4049/jimmunol.182.3.1706
- Sadler AJ, Williams BR. Interferon-inducible antiviral effectors. Nat Rev Immunol 2008; 8:559-68; PMID:18575461; http://dx.doi.org/10.1038/nri2314
- Kochs G, Haller O. Interferon-induced human MxA GTPase blocks nuclear import of Thogoto virus nucleocapsids. Proc Natl Acad Sci U S A 1999; 96:2082-6; PMID:10051598; http://dx.doi.org/10.1073/pnas.96.5.2082
- Accola MA, Huang B, Al Masri A, McNiven MA. The antiviral dynamin family member, MxA, tubulates lipids and localizes to the smooth endoplasmic reticulum. J Biol Chem 2002; 277:21829-35; PMID:11916975; http://dx.doi.org/10.1074/jbc.M201641200
- Kerr IM, Brown RE, Hovanessian AG. Nature of inhibitor of cell-free protein synthesis formed in response to interferon and double-stranded RNA. Nature 1977; 268:540-2; PMID:196217; http://dx.doi.org/10.1038/268540a0
- Rebouillat D, Hovanessian AG. The human 2',5'-oligoadenylate synthetase family: interferon-induced proteins with unique enzymatic properties. J Interferon Cytokine Res 1999; 19:295-308; PMID:10334380; http://dx.doi.org/10.1089/107999099313992
- Clemens MJ, Vaquero CM. Inhibition of protein synthesis by double-stranded RNA in reticulocyte lysates: evidence for activation of an endoribonuclease. Biochem Biophys Res Commun 1978; 83:59-68; PMID:212051; http://dx.doi.org/10.1016/0006-291X(78)90397-2
- Roberts WK, Hovanessian A, Brown RE, Clemens MJ, Kerr IM. Interferon-mediated protein kinase and low-molecular-weight inhibitor of protein synthesis. Nature 1976; 264:477-80; PMID:1004583; http://dx.doi.org/10.1038/264477a0
- Yuan W, Krug RM. Influenza B virus NS1 protein inhibits conjugation of the interferon (IFN)-induced ubiquitin-like ISG15 protein. EMBO J 2001; 20:362-71; PMID:11157743; http://dx.doi.org/10.1093/emboj/20.3.362
- Takeuchi T, Iwahara S, Saeki Y, Sasajima H, Yokosawa H. Link between the ubiquitin conjugation system and the ISG15 conjugation system: ISG15 conjugation to the UbcH6 ubiquitin E2 enzyme. J Biochem 2005; 138:711-9; PMID:16428300; http://dx.doi.org/10.1093/jb/mvi172
- Zhao C, Beaudenon SL, Kelley ML, Waddell MB, Yuan W, Schulman BA, Huibregtse JM, Krug RM. The UbcH8 ubiquitin E2 enzyme is also the E2 enzyme for ISG15, an IFN-alpha/beta-induced ubiquitin-like protein. Proc Natl Acad Sci U S A 2004; 101:7578-82; PMID:15131269; http://dx.doi.org/10.1073/pnas.0402528101
- Lenschow DJ, Giannakopoulos NV, Gunn LJ, Johnston C, O'Guin AK, Schmidt RE, Levine B, Virgin HW, 4th. Identification of interferon-stimulated gene 15 as an antiviral molecule during Sindbis virus infection in vivo. J Virol 2005; 79:13974-83; PMID:16254333; http://dx.doi.org/10.1128/JVI.79.22.13974-13983.2005
- Osiak A, Utermohlen O, Niendorf S, Horak I, Knobeloch KP. ISG15, an interferon-stimulated ubiquitin-like protein, is not essential for STAT1 signaling and responses against vesicular stomatitis and lymphocytic choriomeningitis virus. Mol Cell Biol 2005; 25:6338-45; PMID:16024773; http://dx.doi.org/10.1128/MCB.25.15.6338-6345.2005
- Best SM, Morris KL, Shannon JG, Robertson SJ, Mitzel DN, Park GS, Boer E, Wolfinbarger JB, Bloom ME. Inhibition of interferon-stimulated JAK-STAT signaling by a tick-borne flavivirus and identification of NS5 as an interferon antagonist. J Virol 2005; 79:12828-39; PMID:16188985; http://dx.doi.org/10.1128/JVI.79.20.12828-12839.2005
- Yu S, Chen J, Wu M, Chen H, Kato N, Yuan Z. Hepatitis B virus polymerase inhibits RIG-I- and Toll-like receptor 3-mediated beta interferon induction in human hepatocytes through interference with interferon regulatory factor 3 activation and dampening of the interaction between TBK1/IKKepsilon and DDX3. J Gen Virol 2010; 91:2080-90; PMID:20375222; http://dx.doi.org/10.1099/vir.0.020552-0
- Munoz-Jordan JL, Sanchez-Burgos GG, Laurent-Rolle M, Garcia-Sastre A. Inhibition of interferon signaling by dengue virus. Proc Natl Acad Sci U S A 2003; 100:14333-8; PMID:14612562; http://dx.doi.org/10.1073/pnas.2335168100
- Caignard G, Bourai M, Jacob Y, Tangy F, Vidalain PO. Inhibition of IFN-alpha/beta signaling by two discrete peptides within measles virus V protein that specifically bind STAT1 and STAT2. Virology 2009; 383:112-20; PMID:19007958; http://dx.doi.org/10.1016/j.virol.2008.10.014
- Schlender J, Hornung V, Finke S, Gunthner-Biller M, Marozin S, Brzozka K, Moghim S, Endres S, Hartmann G, Conzelmann KK. Inhibition of toll-like receptor 7- and 9-mediated alpha/beta interferon production in human plasmacytoid dendritic cells by respiratory syncytial virus and measles virus. J Virol 2005; 79:5507-15; PMID:15827165; http://dx.doi.org/10.1128/JVI.79.9.5507-5515.2005
- Parlato S, Bruni R, Fragapane P, Salerno D, Marcantonio C, Borghi P, Tataseo P, Ciccaglione AR, Presutti C, Romagnoli G et al. IFN-alpha regulates Blimp-1 expression via miR-23a and miR-125b in both monocytes-derived DC and pDC. PLoS One 2013; 8:e72833; PMID: 23977359
- Coro ES, Chang WL, Baumgarth N. Type I IFN receptor signals directly stimulate local B cells early following influenza virus infection. J Immunol 2006; 176:4343-51; PMID:16547272; http://dx.doi.org/10.4049/jimmunol.176.7.4343
- Le Bon A, Thompson C, Kamphuis E, Durand V, Rossmann C, Kalinke U, Tough DF. Cutting edge:enhancement of antibody responses through direct stimulation of B and T cells by type I IFN. J Immunol 2006; 176:2074-8; PMID:16455962; http://dx.doi.org/10.4049/jimmunol.176.4.2074
- Kim D, Martinez-Sobrido L, Choi C, Petroff N, Garcia-Sastre A, Niewiesk S, Carsillo T. Induction of type I interferon secretion through recombinant Newcastle disease virus expressing measles virus hemagglutinin stimulates antibody secretion in the presence of maternal antibodies. J Virol 2011; 85:200-7; PMID:20962092; http://dx.doi.org/10.1128/JVI.01624-10
- Jego G, Palucka AK, Blanck JP, Chalouni C, Pascual V, Banchereau J. Plasmacytoid dendritic cells induce plasma cell differentiation through type I interferon and interleukin 6. Immunity 2003; 19:225-34; PMID:12932356; http://dx.doi.org/10.1016/S1074-7613(03)00208-5
- Wang Y, Tong X, Zhang J, Ye X. The complement C1qA enhances retinoic acid-inducible gene-I-mediated immune signalling. Immunology 2012; 136:78-85; PMID:22260551; http://dx.doi.org/10.1111/j.1365-2567.2012.03561.x
- Max EE, Korsmeyer SJ. Human J chain gene. Structure and expression in B lymphoid cells. J Exp Med 1985; 161:832-49; PMID:2984306
- R Development Core Team 2006 R: A language and enviroment for statistical computing. Vienna, Austria:R Foundation for Statistical Computing.
- Gentleman RC, Carey VJ, Bates DM, Bolstad B, Dettling M, Dudoit S, Ellis B, Gautier L, Ge Y, Gentry J, et al. Bioconductor: open software development for computational biology and bioinformatics. Genome Biol 2004; 5:R80; PMID:15461798
- Li C, Hung Wong W. Model-based analysis of oligonucleotide arrays: model validation, design issues and standard error application. Genome Biol 2001; 2:RESEARCH0032; PMID:11532216
- Tusher VG, Tibshirani R, Chu G. Significance analysis of microarrays applied to the ionizing radiation response. Proc Natl Acad Sci U S A 2001; 98:5116-21; PMID:11309499; http://dx.doi.org/10.1073/pnas.091062498
- Mao Q, Cheng T, Zhu F, Li J, Wang Y, Li Y, Gao F, Yang L, Yao X, Shao J, et al. The cross-neutralizing activity of enterovirus 71 subgenotype c4 vaccines in healthy chinese infants and children. PLoS One 2013; 8:e79599; PMID:24260259
- Liang Z, Mao Q, Gao Q, Li X, Dong C, Yu X, Yao X, Li F, Yin W, Li Q, et al. Establishing China's national standards of antigen content and neutralizing antibody responses for evaluation of enterovirus 71 (EV71) vaccines. Vaccine 2011; 29:9668-74; PMID:22015395; http://dx.doi.org/10.1016/j.vaccine.2011.10.018
- Liu L, Zhang Y, Wang J, Zhao H, Jiang L, Che Y, Shi H, Li R, Mo Z, Huang T, et al. Study of the integrated immune response induced by an inactivated EV71 vaccine. PLoS One 2013; 8:e54451; PMID:23372725; http://dx.doi.org/10.1371/journal.pone.0054451