ABSTRACT
Individuals fail to elicit protective antibody after hepatitis B vaccination remain at risk for hepatitis B virus infection. Analysis of the transcriptome of peripheral blood mononuclear cells (PBMCs) is essential to elucidate the characteristics of gene expression in non-responders. In this study, we enrolled seven responders who had received three injections and seven non-responders who had six injections of hepatitis B vaccine before. All the participants were then vaccinated with a three-dose boost regimen. Microarray analysis and Luminex assay were applied to examine mRNA expression and Th1/Th2/Th9/Th17/Th22/Treg cytokine and chemokine profiles in non-responders and responders. Differentially expressed genes in PBMCs of non-responders at 5 time points, i.e. pre-vaccination, 3rd, 7th, 28th day post the first dose vaccination and 7th day post the second dose vaccination indicated a dense network trend. Compared with responders, nine coding genes (BPI, DEFA1B, DEFA4, CEACAM8, MMP8, FOLR3, LTF, TCN1 and TKTL1) were significantly up-regulated in non-responders at all 5 time points, which could probably be the characteristic genes in hepatitis B vaccine non-responsiveness. Gene ontology analysis revealed that most of the DEGs were related with immune responses. Validation results of these 9 genes using quantitative real-time polymerase chain reaction were mostly consistent with the results of microarray. Cytokine analysis demonstrated that IL-27 and CXCL12 concentrations in responders were significantly higher than non-responders on the 3rd day after the first dose and 7th day after the second dose of vaccination, respectively. No significant difference was observed in other cytokine and chemokine signatures between the two groups. In conclusion, our results revealed characteristic transcriptome and cytokine changes in hepatitis B vaccine non-responders after boost immunization.
Introduction
As one of the most serious chronic viral infections, hepatitis B caused by hepatitis B virus (HBV) has been a worldwide health problem estimated to lead to over 500,000 deaths every year and is responsible for 50% and 33% of death attributable to liver cancer and cirrhosis, respectively.Citation1,Citation2,Citation3 Vaccination against HBV has been gradually adopted in national immunization programs in many countries. In China, after over 20 years usage of recombinant hepatitis B (HB) vaccine, HBsAg prevalence in Chinese population 1–29 years of age dramatically declined from 10.1% to 2.6% during 1992–2014, especially among children <5 years of age HBsAg prevalence was only 0.3% with a reduction of over 95%.Citation4,Citation5
Although HB vaccine has been proven to be effective and safe in protecting people against HBV infection, following a standard three-dose vaccination regimen, approximately 5–10% of healthy adults fail to elicit protective anti-HBs titer (≥10 mIU/mL) and remain at risk for HBV infection.Citation6 Subjects with anti-HBs titers of <10 mIU/mL 1–6 months after standard immunization schedule, who tested negative for HBsAg and anti-HBc, are defined as non-responders of HB vaccine.Citation7 Various factors may influence the immune response to vaccines such as vaccine type, dosage, and genetic background of vaccinees.Citation8,Citation9,Citation10 Host-related factors such as age, obesity, smoking and chronic infection or immunological tolerance have all been reported attributable to lack of response to HB vaccine.Citation11 Human leukocyte antigens (HLA) which were involved in the antigen processing and presentation, polymorphisms on cytokines, cytokine receptors and Toll-like receptors were reported associated with the status of the HB vaccine-induced protective immune response.Citation12,Citation13,Citation14 Immune deficiency such as dysfunction or insufficient amount of Th cells induced low cytokine secretion or hyporesponsiveness in B cells may also lead to the production of non-response to vaccine.Citation15,Citation16,Citation17
Previous researches on non-response to HB vaccine were based on single-hypothesis approaches, which would probably ignore the interplay in those related factors. Transcriptome microarray provided a multidimensional measure for deep insight into the mechanism of immune response. This approach has been successfully used to identify early gene signature for predicting the post-vaccination antibody of yellow fever vaccine YF-17D and seasonal influenza vaccineCitation18.Citation19 Transcriptomic research offered a novel method for the prediction of post-vaccination immunity and investigation on mechanism of vaccine-induced immune responses, which may provide the basis for improvement of vaccine.
Previous researches showed that 90% of the non-responders revaccinated with a boost regimen of HB vaccine or combined vaccine would elicit protective anti-HBs.Citation20,Citation21 In order to better find out the real mRNA expression characteristics and cytokine changes, non-responders who had received two round standard three-dose vaccination with anti-HBs titers < 1mIU/mL at one month after the last dose of the second regimen were enrolled in this study. Responders with a protective antibody level after a standard three-dose regimen were recruited as a control group. With boosted immunization following a 0-1-2 schedule, all participants were analyzed for the dynamic mRNA expression and cytokine profiling by transcriptome microarray and Luminex assay in this study..
Results
Significant transcriptomic changes in non-responders at 5 time points pre- and post revaccination
Transcriptome microarray analyses were performed to explore the differences in expression profile between the non-responder and responder group. Hierarchical clustering demonstrated significant changes in the transcriptome of PBMCs of the two groups at 5 different time points. In the microarray analysis, 55 up-regulated and 15 down-regulated genes were identified in PBMC in non-responders compared with responders before vaccination (Fold Change (FC) ≥2.0 and p<0.05) (). After the first dose of vaccination, 28, 68 and 45 genes were differentially expressed on the 3rd, 7th and 28th day, respectively, whereas 23 genes were up-regulated and 6 genes were down-regulated on the 7th day post the second dose of vaccination (). Interaction of proteins of differentially expressed coding RNAs pre- and post vaccination exhibited a dense network trend, especially for immune related cytokines, like interleukin-8 (IL-8), CXCL10, CCL4, interferon-γ (IFNG), bactericidal/permeability-increasing protein (BPI), carcinoembryonic antigen related cell adhesion molecule 8 (CEACAM8), defensin alpha 4 (DEFA4), lactotransferrin (LTF), matrix metallopeptidase 8 (MMP8) and ribonuclease A family member 3 (RNASE3) ().
Figure 1. Differential genomic signatures in PBMCs between the non-responder and responder groups at 5 time points. (A-E) PBMCs from 3 participants of each group was conducted tanscriptome analysis at 5 time points, i.e. pre-vaccination, 3rd, 7th, 28th day post the first dose vaccination and 7th day post the second dose vaccination. DEGs exhibited significant differences (Fold Change ≥2.0 and p-value <0.05) between the two groups at all time points. Transcript expressions were transformed to z-score and depicted in green to black to red color scale.
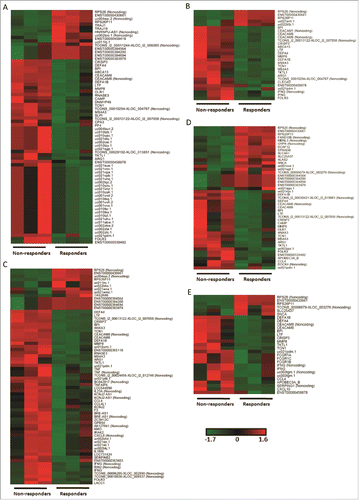
Figure 2. Interaction networks of proteins derived from differentially expressed coding RNAs. STRING database (http://string-db.org/) was used to assess the integration of protein-protein interactions. All DEGs discovered from each time point were analyzed for the interaction of encoded proteins. The numbers of lines represented the closeness of these proteins. Line thickness indicates the strength of data support from the database.
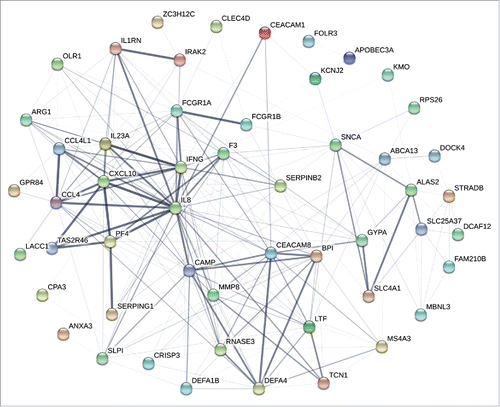
Continuous genetic characteristics revealed in non-responders
Compared with responders, a total of 11 up-regulated genes and 3 down-regulated genes were identified in non-responders at all 5 time points ( and ). Up-regulated genes contained 9 coding RNAs, including BPI, CEACAM8, DEFA1B, DEFA4, folate receptor 3 (FOLR3), LTF, MMP8, transcobalamin 1 (TCN1), transketolase like 1 (TKTL1), and 2 noncoding RNAs, one of which named lnc-CEACAM8, participating in CEACAM8 expression and regulation process (). Down-regulated genes involved 3 non-coding RNAs playing role in protein synthesis. These DEGs which constantly existed in the two groups provided a clue for the existence of fixed genetic differences that were independent of vaccination and might be the transcriptomic characteristics of HB vaccine non-responders.
Figure 3. Comprehensive analyses and dynamic changes of the DEGs identified in non-responder and responder groups at 5 different time points. (A-B) Venn diagrams indicated the numbers of persistent up- or down-regulated expressed genes in non-responders at all time points, compared with responders. (C) Heat map showed the fold change of 11 up-regulated and 3 down-regulated DEGs in non-responders with persistent significant expression at all 5 time points, compared with responders. The change threshold was set to 2-fold.
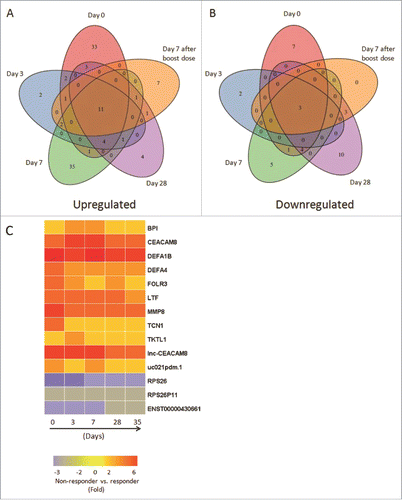
Validation of DEGs expression levels using qRT-PCR
To validate the DEGs exhibited from the microarray, a subset of 9 up-regulated genes with continuous expression at all 5 time points were selected for further qRT-PCR validation and all samples from 28 days after first dose vaccination which were analyzed in microarray were conducted validation. The expression pattern of the selected genes concurred with the microarray data for 8 of 9 of the analyzed probesets (BPI, DEFA1B, DEFA4, FLOR3, LTF, MMP8, TCN1, TKTL1) (). The qRT-PCR results demonstrated that 8 genes were significantly up-regulated with the FC ≥2.0. Down-regulation was detected by PCR in one of the selected probesets (CEACAM8) which was inconsistent with microarray result (0.71-fold vs. 2.96-fold).
Figure 4. Validation of up-regulated expressed genes by qRT-PCR. The expression of 9 up-regulated genes (Fold Change ≥2.0) identified by microarray assay (in black color) at 5 time points was re-evaluated by qRT-PCR (in gray color). Eight genes in qRT-PCR results confirmed the data of the microarray.
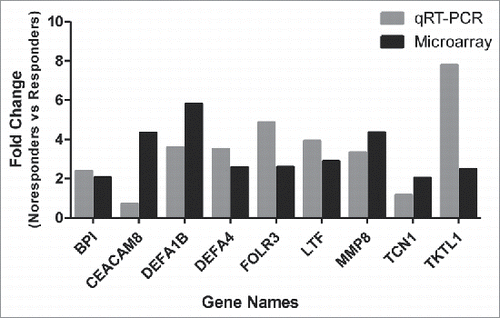
Gene ontology (GO) analysis
In search of the differentially expressed genes in Biological Process between the two groups, the GO enrichment analysis was performed with default settings using the web-based database for annotation, visualization, and integrated discovery (DAVID). At the 5 time points pre- and post-vaccination, 152, 262, 562, 253 and 428 Go items were identified respectively. According to Enrichment Score, these Go items mainly include “defense response to bacterium", “antibacterial and antimicrobial humoral response”, “defense response to other organism”, “immune response”, “immune system process”, etc (). Top 2 Go items in Fold Enrichment were “antibacterial
Figure 5. GO analysis for differentially expressed genes between non-responders and responders at 5 different time points. (A-E)The top 10 biological process of DEGs at the time point of pre-vaccination, 3rd, 7th, 28th day post the first dose vaccination and 7th day post the second dose vaccination, respectively.
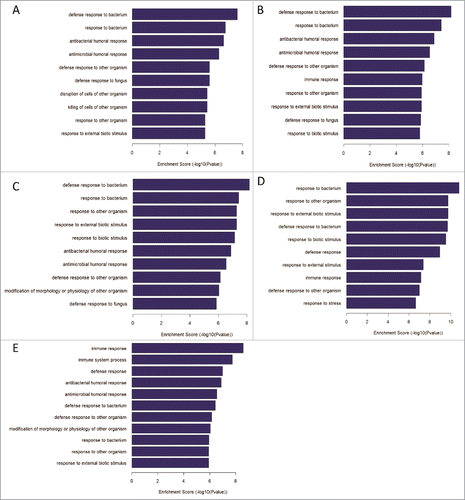
Dynamic cytokine signatures of non-responders and responders
To determine whether non-responders induce detectable cytokine signatures in plasma which were different from responders, cytokine and chemokine panels which contained 27 key mediators (see Materials and Methods) of host immune responses were used to measure the expression of cytokines in plasma on days 3, 7, 28, 35 after first dose of vaccination and 7th day post the second dose of vaccination. Among these, lower concentration of IL-27 was induced in non-responders than responders at all 4 time points, and significant difference was observed on the 3rd day after first dose of immunization. At the time point of 7th day post the second dose, CXCL12 was significantly higher in responders than non-responders (). Other cytokines and chemokines exhibited no significance between the two groups (Data not shown).
Figure 6. Cytokine responses in plasma of HBV vaccine non-responders and responders. Plasmas from all participants were assayed by Luminex. Concentrations of cytokines were expressed as means ± SEM. Statistical analysis was performed using Mann Whitney test for non-responder and responder group at each time point.
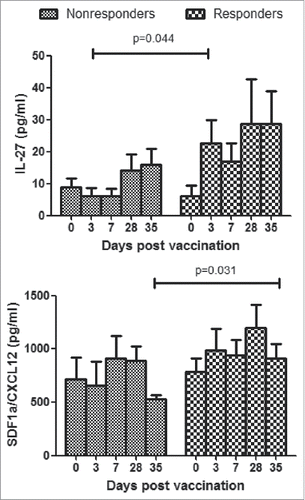
Discussion
Immune response following HBV vaccination could be affected by various factors; nevertheless, the mechanism of the response especially low or non-response to HB vaccine remains to be clearly determined. Specific HLA alleles and polymorphisms in cytokine genes such as IL-2, IL-4 were found to be correlated with variable immune responses to HB vaccine.Citation22,Citation23,Citation24 System vaccinology has emerged as a new approach for the prediction of immunogenicity of vaccines and modules were established to explore the transcriptional signatures of humoral responses to several vaccines.Citation25
We first investigated the expression patterns of genes in HB vaccine non-responders and responders pre- and post-revaccination. All enrolled non-responders were vaccinated with two rounds of three-dose immunization schedule and the titer of anti-HBs was reconfirmed. A total of 5 time points were adopted to compare the dynamic expression change pre- and post- revaccination between non-responders and responders. For the reason that immune response to vaccine was more obvious in short time after immunization, we selected 5 time points before and after the first two doses of inoculation. In responders of HB vaccine, the first two dose of vaccine would elicit high anti-HBs titer and the third dose would enhance the persistence of antibody and in this study we just reconfirmed the anti-HBs status of all participants on the 28th day after the last immunization. Using fold change ≥2.0 as a cutoff, dozens of genes and transcripts were found differentially expressed in non-responders. Analysis results from STRING database exhibited a close interaction among mRNAs selected in DEGs which provided the evidence that differentially expressed mRNAs were closely linked on the functional aspects, rather than exist in isolation.
Previous reports about the responsiveness to HB vaccine mainly focused on a stationary time point, which was generally a long time after last dose of vaccination. In our research, dynamic analysis was adopted to explore the difference on the expression profile of whole transcriptome and secretion of chemokines and cytokines in non-responders, meanwhile, restimulation with HB vaccine would provide a new observation on the differential expression of immune related genes.
At each time point, we found that although DEGs varied in the number, up-regulation of 9 coding RNAs was observed pre- and post-vaccination in non-responders, which indicated that high level expression of these genes was persistent in non-responders, regardless of HB vaccine restimulation or not. DEFA1B, as the most significantly expressed gene, exhibited 7.16-, 6.27-, 7.86-, 5.67-, 6.00-fold up-regulated change in non-responders at 5 time points, respectively (Supplementary Table 1). Fixed and continuous expressed genes in non-responders revealed genetically expressed differences in these HB vaccine non-responders independent of the stimulation of HB vaccine. As a characteristic in HB vaccine non-responders, DEGs may probably affect the emergence of antibody to HB vaccine.
Characteristic DEGs encoded proteins involved in host defense, such as BPI, DEFA1B, DEFA4. As a leukocyte derived protein, BPI coded protein participates in the innate immune response by binding with LPS and neutralizing the pro-inflammatory responses induced by LPS.Citation26 DEFA1B and DEFA4 belong to α-defensins which could be induced by cytokines or TLR activation, and can modulate adaptive immune responses.Citation27 DEFA1 can activate human pDCs through NF-κB and IRF signaling pathways, and as potential antimicrobial molecules that participate in innate and adaptive immunity, α-defensins 1–3 could also modulate the maturation and differentiation of monocyte-derived DCs in vivo.Citation28,Citation29
In this study, the results of CEACAM8 verification in qRT-PCR were inconsistent with microarray analysis. CEACAM8 may activate human eosinophils by releasing of proinflammatory mediators and activated eosinophils exhibited its effector's function by production of cytokine.Citation30,Citation31 After increasing the number of samples of microarray, we will further confirm whether CEACAM8 is the characteristic DEG in non-responders. The expression of MMP8 in non-responders was 4 times higher than that of responders at all 5 time points pre- and post- vaccination (). As playing a dual role in different conditions, MMP8 either participants in the development of an inflammatory response or exerts an anti-inflammatory role during recovery.Citation32
Persistent DEGs also contained 4 genes related to metabolism, namely FOLR3, TCN1, LTF and TKTL1, which facilitate folic acid, vitamin B12, iron ions, glucose transport and metabolic process,Citation33,Citation34,Citation35 and act as critical parts in cell metabolism. Metabolites of vitamin B were reported to exhibit a broad spectrum of mucosal-associated invariant T cell activation which has crucial functions in protective immunity.Citation36 In vitro studies have confirmed that LTF could modulate both cell-mediated and humoral responses by promoting the maturation, the differentiation and the activation of T and B lymphocytes.Citation37 Furthermore, LTF affected the Th1/Th2 immune response balance through the modulation of cytokine expression, and oriented Th responses toward different directions.Citation38
In addition to these up-regulated genes, down-regulated genes consist of two lncRNAs and one pseudogene in non-responders. RPS26 as one of the two lncRNAs significantly expressed with lower level in non-responders, was reported to have relation with p53 stabilization and activation which resulting in cell growth inhibition.Citation39 Although the mechanism of noncoding RNA regulating immune response is not completely clear, it has been identified that lncRNAs can regulate immune cell differentiation and function.Citation40 These up- or down-regulated noncoding RNAs in our study may have a close connection with humoral immune response, which needs further validation in the follow-up study.
In cytokine profiling, of the 27 cytokines and chemokines assayed, IL-27 and CXCL12 concentration exhibited significantly high level in 7 responders on the 3rd day post the first dose of immunization and 7th day post the second dose, respectively. In responders, IL-27 aroused dramatically 3 days after vaccination and maintained at a higher level in the following time points, representing a distinctive feature with non-responders. CXCL12 significantly declined in non-responders after boost immunization compared with 28th day after first vaccination, while in responders it showed no significant change. As IL-27 has both pro- and anti-inflammatory effects through promoting the expansion of naïve CD4+ T cells and negatively regulating Th17 differentiation,Citation41,Citation42 it plays important roles in immune response. CXCL12, also known as pre-B cell growth-stimulating factor (PBSF), which is involved in controlling B cell development and has strong chemotactic capacity of stem cells and lymphocytesCitation43,Citation44 and in our study revaccination in non-responders did not boost CXCL12 secretion hence probably affected the production of humoral immune response. Nevertheless, mRNA expression of IL-27 and CXCL12 in microarray analysis showed no significant change at all time points. Other representative factors of cellular and humoral immune response, such as IFN-γ, IL-2 and IL-4 in our study, rose in concentration post-vaccination in both groups, but no significance were observed between the two groups (data not shown). Lack of antigen specific IL-2 and IFN-γ were reported in non-responders,Citation15 but in the present study the above non-specific cytokine in plasma showed no difference in non- and normal responders. Interestingly, the seroconversion rates of anti-HAV and anti-HEV in non-responders had no difference from responders (data not shown), which implied that Th1 and Th2 cells in non-responders functioned normally. Meanwhile, no significant change was observed in IL-9, IL-17A, IL-21, IL-22, IL-23 and other cytokines which reflected no abnormality of Th9/Th17/Th22/Treg related cell function occurred in the non-responders.
Transcriptome analysis provides a convenient alternative for large-scale screening and gene expression identification, with which we explored the transcriptome characteristics of non-responders with boost immunization of HB vaccine. No matter whether received revaccination or not, constant expression of some DEGs exist which may prompt the clue of innate correlation between these genes and vaccine responsiveness. In multiplex cytokine assay, IL-27 and CXCL12 may probably act as the characteristic cytokine marker for responders.
Some limitations exist in our study. First, there may be false-positive association of immune response in non- and normal responders in transcriptome analysis. Second, due to the limited sample availability, our detections were conducted in 7 non-responders for cytokine determination and 3 non-responders for microarray analysis with the same amount of responders. We would enroll more non-responder subjects to verify the gene expression profiles and the dynamic cytokine changes in further investigation. Third, responders enrolled in the study had received three doses of hepatitis B vaccine before, and in this study all of them were revaccinated with three additional doses, so the immune response status of responders may reflected the characteristics of booster vaccinations rather than naïve baselines. As this was an inadequate control for non-responder group, the results could not clarify the genetic differences between non-responders and responders, or predict the occurrence of non-responders.
In summary, our results appear to demonstrate that multiple factors may participate in the immune response of HB vaccine non-responders after boost immunization, such as genes related with immune response, metabolism and immune regulation. Further investigation of the characteristic genes and cytokines in non-responders screened from naïve vaccines may unveil the mechanism of non-response to HB vaccine or other vaccines, hence facilitating development of individualized vaccines for non-responders.
Materials and methods
Study design and conduct
A total of 14 subjects were included in the study, including 7 non-responders and 7 responders. Enrolled subjects were excluded based on current or previous infection with HBV, HCV and other acute or chronic infectious diseases, and with history of diabetes, dialysis and smoking. Included subjects were of age <50 and BMI<28. In the screening stage, all participants were vaccinated with a 0-1-6 standard regimen, and anti-HBs levels were determined at one month after the last dose. Non-responders with anti-HBs tiers < 1mIU/mL received a second round standard regimen. One month after the last dose of the second regimen, participants with anti-HBs titers < 1mIU/mL were enrolled as non-responders in the study. Responders had received three-dose standard regimen and elicited protective anti-HBs. Participants were vaccinated with three booster doses of recombinant hepatitis B vaccine (20 μg HBsAg/mL per dose, Shenzhen Kangtai Biological Products Co., Ltd.) according to the schedule of 0, 4, 8-week since 12 months after the last vaccination.
Anticoagulated blood samples were obtained pre-vaccination, 3, 7, 28 d after the first dose, 7 d after the second dose and 28 d after the last dose. Inoculation, blood sampling and test procedure were shown in . The study was approved by the Ethics Committee of Shandong Center for Disease Control and Prevention. Before the commencement of the study, written informed consents were obtained from all participants.
Isolation of PBMCs and plasma
PBMCs were separated from anticoagulated peripheral blood by density gradient centrifugation with Lymphoprep™ (Axis-Shield, Olso, Norway) and frozen in FBS (Gibco, NY, USA) with 10% DMSO (Sigma-Aldrich, MO, USA) in liquid nitrogen freezers. Plasma samples were stored at −80°C. Trizol (Invitrogen, CA, USA) was used to lyse PBMCs (1 mL of Trizol to 2.0 × 106 cells) and to protect RNA from degradation. Trizol samples were stored at −80°C for microarray.
RNA preparation and microarray hybridization
After extraction from PBMCs, RNAs were quantified using a NanoDrop 2000 (Thermo Scientific, MA, USA), and integrity was assessed by agarose gel electrophoresis. Sample preparation for microarray processing was carried out according to the GeneChip® WT PLUS Reagent Kit (Affymetrix, CA, USA). After fragmentation of 2nd-cycle single-stranded cDNA, samples were labeled with biotin by terminal deoxynucleotidyl transferase. Then, samples were hybridized to the Affymetrix Human Transcriptome Array 2.0 (Affymetrix) for 16–18 h at 45°C. Following hybridization, the microarrays were washed and stained. Microarrays were scanned by using Affymetrix® GeneChip Command Console which installed in GeneChip® Scanner 3000 7G (Affymetrix).
Microarray data anaylsis
The data were analyzed with Robust Multichip Analysis (RMA) algorithm using default analysis settings and global scaling as normalization method by Partek® Genomics Suite 6.6. Values presented are log2 RMA signal intensity. Normalized data were further analyzed using one-way ANOVA to screen out the differentially expressed genes. To identify DEGs with statistical significance FC ≥2.0 (p < 0.05) was used as a cutoff. Then, DAVID (http://david.abcc.ncifcrf.gov/home.jsp) was used to determine pathways and processes of major biological significance and importance based on the GO annotation function. STRING database (http://string-db.org/) was used to provide an assessment and integration of protein-protein interactions.
Real-time quantitative (qRT) PCR detection
Following RNA extraction, 1μg RNA of each sample was reverse transcribed into cDNA using PrimeScript™ RT reagent Kit with gDNA Eraser (Takara, Tokyo, Japan) according to manufacturer's protocols. Real-time PCR was performed using SYBR® Premix Ex Taq™ II (Tli RNaseH Plus) ROX plus (Takara) on a ABI 7500 Real-Time PCR System (Applied Biosystems, CA, USA). The gene encoding 18S rRNA was chosen as an endogenous reference. qRT-PCR measurements were performed with samples collected on 28 days after first dose vaccination.
A total of 9 probesets were selected for confirmation. All selected genes had an average fold change of 2.0 or higher by microarray. Relative expression was calculated using the comparative Ct method.Citation45
Multiplex analysis
Human Th1/Th2 & Chemokine Panel (20 plex, CCL11 [Eotaxin], GM-CSF, CXCL1 [GROα], IFN-γ, IL-1β, IL-2, IL-4, IL-5, IL-6, CXCL8 [IL-8], IL-12p70, IL-13, IL-18, CXCL10 [IP-10], CCL2 [MCP-1], CCL3 [MIP-1α]/, CCL4 [MIP-1β], CCL5 [Rantes], CXCL12 [SDF1α], TNFα) and Human Th9/Th17/Th22/Treg Cytokine Panel (7 plex, IL-9, IL-10, IL-17A, IL-21, IL-22, IL-23, IL-27) were used to measure 70 plasma samples of all participants. Samples were assayed to determine the concentration of secreted proteins including cytokines, chemokines and other proteins according to ProcartaPlex™ immunoassays protocols (eBioscience, CA, USA). The data were tested for significance by Mann Whitney test (p < 0.05).
Abbreviations
PBMC | = | Peripheral blood mononuclear cell |
HB | = | Hepatitis B |
HLA | = | Human leukocyte antigens |
HBsAg | = | Hepatitis B surface antigen |
GO | = | Gene Ontology |
IL | = | Interleukin |
IFNG | = | Interferon-γ |
BPI | = | Bactericidal/ permeability-increasing protein |
CEACAM8 | = | Carcinoembryonic antigen related cell adhesion molecule 8 |
DEFA4 | = | Defensin alpha 4 |
LTF | = | Lactotransferrin |
MMP8 | = | Matrix metallopeptidase 8 |
RNASE3 | = | Ribonuclease A family member 3 |
FOLR3 | = | Folate receptor 3 |
TCN1 | = | Transcobalamin 1 |
TKTL1 | = | Transketolase like 1 |
RMA | = | Robust multichip analysis |
qRT-PCR | = | Real-time quantitative polymerase chain reaction |
Disclosure potential conflicts of interest
The authors declare no conflict of interest.
KHVI_A_1450122_Supplemental.xlsx
Download MS Excel (33.3 KB)Acknowledgments
We thank relevant personnel from Zhangqiu Center for Disease Control and Prevention of Shandong province for their contribution in blood sample collection.
Additional information
Funding
References
- Lavanchy D. Hepatitis B virus epidemiology, disease burden, treatment, and current and emerging prevention and control measures. Journal of viral hepatitis. 2004;11(2):97–107. doi:10.1046/j.1365-2893.2003.00487.x. PMID:14996343
- Lozano R, Naghavi M, Foreman K, Lim S, Shibuya K, Aboyans V, et al. Global and regional mortality from 235 causes of death for 20 age groups in 1990 and 2010: a systematic analysis for the Global Burden of Disease Study 2010. Lancet. 2012;380(9859):2095–128. doi:10.1016/S0140-6736(12)61728-0. PMID:23245604
- Shepard CW, Simard EP, Finelli L, Fiore AE, Bell BP. Hepatitis B virus infection: epidemiology and vaccination. Epidemiologic reviews. 2006;28:112–25. doi:10.1093/epirev/mxj009. PMID:16754644
- Wang FZ, Zhang GM, Shen LP, Zheng H, Wang F, Miao N, et al. Comparative analyze on hepatitis B seroepidemiological surveys among population aged 1–29 years in different epidemic regions of China in 1992 and 2014. Zhonghua yu fang yi xue za zhi [Chinese journal of preventive medicine]. 2017;51(6):462–8. PMID:28592086
- Cui F, Shen L, Li L, Wang H, Wang F, Bi S, et al. Prevention of Chronic Hepatitis B after 3 Decades of Escalating Vaccination Policy, China. Emerging infectious diseases. 2017;23(5):765–72. doi:10.3201/eid2305.161477. PMID:28418296
- Zuckerman JN. Protective efficacy, immunotherapeutic potential, and safety of hepatitis B vaccines. Journal of medical virology. 2006;78(2):169–77. doi:10.1002/jmv.20524. PMID:16372285
- Kubba AK, Taylor P, Graneek B, Strobel S. Non-responders to hepatitis B vaccination: A review. Communicable disease and public health. 2003;6(2):106–12. PMID:12889288
- Newport MJ, Goetghebuer T, Weiss HA, Whittle H, Siegrist CA, Marchant A, et al. Genetic regulation of immune responses to vaccines in early life. Genes and immunity. 2004;5(2):122–9. doi:10.1038/sj.gene.6364051. PMID:14737096
- Hohler T, Reuss E, Evers N, Dietrich E, Rittner C, Freitag CM, et al. Differential genetic determination of immune responsiveness to hepatitis B surface antigen and to hepatitis A virus: a vaccination study in twins. Lancet. 2002;360(9338):991–5. doi:10.1016/S0140-6736(02)11083-X. PMID:12383669
- Yang S, Tian G, Cui Y, Ding C, Deng M, Yu C, et al. Factors influencing immunologic response to hepatitis B vaccine in adults. Scientific reports. 2016;6:27251. doi:10.1038/srep27251. PMID:27324884
- Boland GJ, van Bommel T, Rulos-van den Berg A, van den Berg JP, van Loon AM, van Hattum J. The efficacy of a two-dose hepatitis B vaccination scheme. Advances in experimental medicine and biology. 2003;531:185–90. doi:10.1007/978-1-4615-0059-9_14. PMID:12916790
- Godkin A, Davenport M, Hill AV. Molecular analysis of HLA class II associations with hepatitis B virus clearance and vaccine nonresponsiveness. Hepatology. 2005;41(6):1383–90. doi:10.1002/hep.20716. PMID:15915462
- Wang Y, Xu P, Zhu D, Zhang S, Bi Y, Hu Y, et al. Association of polymorphisms of cytokine and TLR-2 genes with long-term immunity to hepatitis B in children vaccinated early in life. Vaccine. 2012;30(39):5708–13. doi:10.1016/j.vaccine.2012.07.010. PMID:22824342
- Pan L, Zhang W, Liang Z, Wu X, Zhu X, Li J, et al. Association between polymorphisms of the cytokine and cytokine receptor genes and immune response to hepatitis B vaccination in a Chinese Han population. Journal of medical virology. 2012;84(1):26–33. doi:10.1002/jmv.22251. PMID:22052597
- Kardar GA, Jeddi-Tehrani M, Shokri F. Diminished Th1 and Th2 cytokine production in healthy adult nonresponders to recombinant hepatitis B vaccine. Scandinavian journal of immunology. 2002;55(3):311–4. doi:10.1046/j.1365-3083.2002.01057.x. PMID:11940238
- Valats JC, Tuaillon E, Funakoshi N, Hoa D, Brabet MC, Bollore K, et al. Investigation of memory B cell responses to hepatitis B surface antigen in health care workers considered as non-responders to vaccination. Vaccine. 2010;28(39):6411–6. doi:10.1016/j.vaccine.2010.07.058. PMID:20682363
- Goncalves L, Albarran B, Salmen S, Borges L, Fields H, Montes H, et al. The nonresponse to hepatitis B vaccination is associated with impaired lymphocyte activation. Virology. 2004;326(1):20–28. doi:10.1016/j.virol.2004.04.042. PMID:15262491
- Querec TD, Akondy RS, Lee EK, Cao W, Nakaya HI, Teuwen D, et al. Systems biology approach predicts immunogenicity of the yellow fever vaccine in humans. Nature immunology. 2009;10(1):116–25. doi:10.1038/ni.1688. PMID:19029902
- Nakaya HI, Wrammert J, Lee EK, Racioppi L, Marie-Kunze S, Haining WN, et al. Systems biology of vaccination for seasonal influenza in humans. Nature immunology. 2011;12(8):786–95. doi:10.1038/ni.2067. PMID:21743478
- Pan HX, Zeng Y, Song XF, Zhang YJ, Xu K, Liang ZL, et al. Immune response to hepatitis B vaccine with high antigen content in non-responders after standard primary vaccination in Chinese adults. Vaccine. 2014;32(29):3706–12. doi:10.1016/j.vaccine.2014.02.094. PMID:24681228
- Cardell K, Akerlind B, Sallberg M, Fryden A. Excellent response rate to a double dose of the combined hepatitis A and B vaccine in previous nonresponders to hepatitis B vaccine. The Journal of infectious diseases. 2008;198(3):299–304. doi:10.1086/589722. PMID:18544037
- Wang C, Tang J, Song W, Lobashevsky E, Wilson CM, Kaslow RA. HLA and cytokine gene polymorphisms are independently associated with responses to hepatitis B vaccination. Hepatology 2004;39(4):978–88. doi:10.1002/hep.20142. PMID:15057902
- Lin YJ, Lan YC, Huang YC, Lin TH, Huang SM, Lai CC, et al. Effects of cytokine and cytokine receptor gene variation on high anti-HB titers: following up on Taiwan's neonatal hepatitis B immunization program. Clinica chimica acta; international journal of clinical chemistry. 2012;413(15-16):1194–8. doi:10.1016/j.cca.2012.03.004. PMID:22484276
- Yoon JH, Shin S, In J, Chang JY, Song EY, Roh EY. Association of HLA alleles with the responsiveness to hepatitis B virus vaccination in Korean infants. Vaccine. 2014;32(43):5638–44. doi:10.1016/j.vaccine.2014.08.007. PMID:25148772
- Li S, Rouphael N, Duraisingham S, Romero-Steiner S, Presnell S, Davis C, et al. Molecular signatures of antibody responses derived from a systems biology study of five human vaccines. Nature immunology. 2014;15(2):195–204. doi:10.1038/ni.2789. PMID:24336226
- Marra MN, Wilde CG, Collins MS, Snable JL, Thornton MB, Scott RW. The role of bactericidal/permeability-increasing protein as a natural inhibitor of bacterial endotoxin. Journal of immunology. 1992;148(2):532–7.
- Klotman ME, Chang TL. Defensins in innate antiviral immunity. Nature reviews Immunology. 2006;6(6):447–56. doi:10.1038/nri1860. PMID:16724099
- Wang F, Qiao L, Lv X, Trivett A, Yang R, Oppenheim JJ, et al. Alarmin human alpha defensin HNP1 activates plasmacytoid dendritic cells by triggering NF-kappaB and IRF1 signaling pathways. Cytokine. 2016;83:53–60. doi:10.1016/j.cyto.2016.03.015. PMID:27031443
- Rodriguez-Garcia M, Oliva H, Climent N, Escribese MM, Garcia F, Moran TM, et al. Impact of alpha-defensins1-3 on the maturation and differentiation of human monocyte-derived DCs. Concentration-dependent opposite dual effects. Clinical immunology. 2009;131(3):374–84. doi:10.1016/j.clim.2009.01.012. PMID:19237318
- Trulson A, Bystrom J, Engstrom A, Larsson R, Venge P. The functional heterogeneity of eosinophil cationic protein is determined by a gene polymorphism and post-translational modifications. Clinical and experimental allergy: journal of the British Society for Allergy and Clinical Immunology. 2007;37(2):208–18. doi:10.1111/j.1365-2222.2007.02644.x. PMID:17250693
- Rothenberg ME, Hogan SP. The eosinophil. Annual review of immunology. 2006;24:147–74. doi:10.1146/annurev.immunol.24.021605.090720. PMID:16551246
- Dejonckheere E, Vandenbroucke RE, Libert C. Matrix metalloproteinase8 has a central role in inflammatory disorders and cancer progression. Cytokine & growth factor reviews. 2011;22(2):73–81. doi:10.1016/j.cytogfr.2011.02.002.
- Shen F, Wu M, Ross JF, Miller D, Ratnam M. Folate receptor type gamma is primarily a secretory protein due to lack of an efficient signal for glycosylphosphatidylinositol modification: protein characterization and cell type specificity. Biochemistry. 1995;34(16):5660–5. doi:10.1021/bi00016a042. PMID:7727426
- Hazra A, Kraft P, Lazarus R, Chen C, Chanock SJ, Jacques P, et al. Genome-wide significant predictors of metabolites in the one-carbon metabolism pathway. Human molecular genetics. 2009;18(23):4677–87. doi:10.1093/hmg/ddp428. PMID:19744961
- Grimm M, Schmitt S, Teriete P, Biegner T, Stenzl A, Hennenlotter J, et al. A biomarker based detection and characterization of carcinomas exploiting two fundamental biophysical mechanisms in mammalian cells. BMC cancer. 2013;13:569. doi:10.1186/1471-2407-13-569. PMID:24304513
- Kjer-Nielsen L, Patel O, Corbett AJ, Le Nours J, Meehan B, Liu L, et al. MR1 presents microbial vitamin B metabolites to MAIT cells. Nature. 2012;491(7426):717–23. PMID:23051753
- Legrand D. Lactoferrin, a key molecule in immune and inflammatory processes. Biochemistry and cell biology = Biochimie et biologie cellulaire. 2012;90(3):252–68. doi:10.1139/o11-056. PMID:22136726
- Fischer R, Debbabi H, Dubarry M, Boyaka P, Tome D. Regulation of physiological and pathological Th1 and Th2 responses by lactoferrin. Biochemistry and cell biology = Biochimie et biologie cellulaire. 2006;84(3):303–11. doi:10.1139/o06-058. PMID:16936801
- Cui D, Li L, Lou H, Sun H, Ngai SM, Shao G, et al. The ribosomal protein S26 regulates p53 activity in response to DNA damage. Oncogene. 2014;33(17):2225–35. doi:10.1038/onc.2013.170. PMID:23728348
- Wang P, Xue Y, Han Y, Lin L, Wu C, Xu S, et al. The STAT3-binding long noncoding RNA lnc-DC controls human dendritic cell differentiation. Science. 2014;344(6181):310–3. doi:10.1126/science.1251456. PMID:24744378
- Lucas S, Ghilardi N, Li J, de Sauvage FJ. IL-27 regulates IL-12 responsiveness of naive CD4+ T cells through Stat1-dependent and -independent mechanisms. Proceedings of the National Academy of Sciences of the United States of America. 2003;100(25):15047–52. doi:10.1073/pnas.2536517100. PMID:14657353
- Diveu C, McGeachy MJ, Boniface K, Stumhofer JS, Sathe M, Joyce-Shaikh B, et al. IL-27 blocks RORc expression to inhibit lineage commitment of Th17 cells. Journal of immunology. 2009;182(9):5748–56. doi:10.4049/jimmunol.0801162.
- Egawa T, Kawabata K, Kawamoto H, Amada K, Okamoto R, Fujii N, et al. The earliest stages of B cell development require a chemokine stromal cell-derived factor/pre-B cell growth-stimulating factor. Immunity. 2001;15(2):323–34. doi:10.1016/S1074-7613(01)00185-6. PMID:11520466
- Ferrari D, Gulinelli S, Salvestrini V, Lucchetti G, Zini R, Manfredini R, et al. Purinergic stimulation of human mesenchymal stem cells potentiates their chemotactic response to CXCL12 and increases the homing capacity and production of proinflammatory cytokines. Experimental hematology. 2011;39(3):360–374, 374 e361–365. doi:10.1016/j.exphem.2010.12.001.
- Livak KJ, Schmittgen TD. Analysis of relative gene expression data using real-time quantitative PCR and the 2(-Delta Delta C(T)) Method. Methods. 2001;25(4):402–s8. doi:10.1006/meth.2001.1262. PMID:11846609