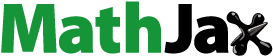
ABSTRACT
Annual seasonal influenza vaccination (SIV) is recommended for people with diabetes, but vaccine coverage remains low. We estimated the probabilities of stopping or starting SIV, their correlates, and the expected time spent in the vaccinated state over 10 seasons for different patient profiles. We set up a retrospective cohort study of patients with diabetes in 2006 (n = 16,026), identified in a representative sample of beneficiaries of the French National Health Insurance Fund. We followed them up over 10 seasons (2005/06–2015/16). We used a Markov model to estimate transition probabilities and a proportional hazards model to study covariates. Between two consecutive seasons, the probabilities of starting (0.17) or stopping (0.09) SIV were lower than those of remaining vaccinated (0.91) or unvaccinated (0.83). Men, older patients, those with type 1 diabetes, treated diabetes or more comorbidities, frequent contacts with doctors, and with any hospital stay for diabetes or influenza during the last year were more likely to start and/or less likely to stop SIV. The mean expected number of seasons with SIV uptake over 10 seasons (range: 2.6–7.9) was lowest for women <65 years with untreated diabetes and highest for men ≥65 years with type 1 diabetes. Contacts with doctors and some clinical events may play a key role in SIV adoption. Healthcare workers have a crucial role in reducing missed opportunities for SIV. The existence of empirical patient profiles with different patterns of SIV uptake should encourage their use of tailored educational approaches about SIV to address patients’ vaccine hesitancy.
Introduction
Because people with diabetes are at increased risk of severe complications linked to seasonal influenza,Citation1 the World Health Organization (WHO) and many national guidelinesCitation2,Citation3 recommend they receive annual seasonal influenza vaccination (SIV). The SIV rate in this population is nonetheless far below WHO’s target of 75% in most Western countries,Citation4 including France (about 30% among those <65 years and 60% among those ≥ 65 years).Citation5,Citation6 Vaccine hesitancy among people with diabetes may contribute significantly to suboptimal vaccination coverage,Citation7 as observed in other population groups, such as parents.Citation8-11 The complexity of the vaccination pathway in France – despite efforts to simplify it – may also contribute to this situation.Citation5
SIV must be repeated annually because immunity from vaccination wanes over time and because of the constant evolution of influenza viruses.Citation2 Each season, the decision to be vaccinated or not is ultimately the patient’s choice. Quantifying behavior changes (starting or stopping SIV) and understanding their determinants are both key to improving public health programs and patient education. Nonetheless, few longitudinal studies have explored the course of SIV behaviors over several consecutive yearsCitation6,Citation12-14 to obtain chronological information about the clinical events preceding and explaining SIV behavior changes.
Multistate Markov (MSM) models, because they allow the analysis of transitions from one health state to another, are particularly appropriate for analyzing sequences of health events. These models make it possible to estimate transition probabilities, namely, the probability of remaining in the same state or of moving to another, and to identify factors affecting these transitions.Citation15 We used this approach to understand the dynamics of SIV behavior changes and identify clinical profiles predictive of this behavior over 10 years.
Our objectives were: 1) to estimate SIV transition probabilities (see above); 2) to quantify associations between these transitions and sociodemographic, clinical, and healthcare utilization characteristics; and 3) to assess the total time spent in the vaccinated state over the 10-season study period according to patients’ characteristics. This third objective seeks to determine easily identifiable demographic-clinical profiles to help clinicians tailor SIV promotion strategies to their patients.
Materials and methods
Study design and data source
We conducted the study in the Permanent Sample of Beneficiaries (Echantillon Généraliste des Bénéficiaires, EGB). The EGB, set up in 2005, is a permanent, representative, and open national random sample of 1/97th of the people affiliated with one of the three major national health insurance funds in France (salaried workers, agricultural workers and farmers, and self-employed workers); these cover nearly 95% of the French population.Citation16 In August 2017 (time of extraction), the database included 804,089 beneficiaries. For this study, we extracted data for salaried workers (including those who are retired) and their covered family members (i.e., children and non-working spouses), who are covered by the French National Health Insurance Fund (NHIF) and account for about 86% of the French population.Citation16 Accessible data were age, sex, long-term illness (LTI) status, and reimbursement claims – for consultations with private healthcare professionals, medical procedures, and drugs purchased in the community (classified by Anatomical Therapeutic Chemical (ATC) codes). At their doctors’ requests, the NHIF grants LTI status to beneficiaries with long-term and costly diseases; this status exempts them from copayments for any medical care associated with that disease, regardless of their income level.Citation17 Data regarding the diagnoses associated with admissions since 2006 to a French public or private hospital, excluding military and psychiatric hospitals, are also available for all individuals in the EGB.
The study analyzed reimbursement data from the National System of Health Data (Système National des Données de Santé), in accordance with the General Conditions of Use of the Portal and the Data (Conditions Générales d’Utilization du Portail et des Données) (version 3.0). Because the study was performed in accordance with Article L1461–1 (paragraph III.6) of the French Public Health Code (Code de la santé publique) for public health purposes with fully anonymized data, there were no further requirements for ethical approval, consent to participate, or data protection agency approval.
Study population
The study population comprised patients identified with diabetes (all types) in 2006, residing in mainland France, and with at least two years of follow-up over the study period. To identify them, we used an NHIF algorithmCitation5 based on LTI status, hospitalization diagnoses and reimbursement claims for antidiabetic drugs or hemoglobin A1c (HbA1c) assays (Supplementary Table 1). Those who died or withdrew from the NHIF during the follow-up period were censored at the start of the year of the event.
Outcome: SIV uptake
For each individual and each SIV season n/n + 1 (temporal statistical units in our analyses), we constructed a binary variable “SIV uptake” (yes, no), based on SIV deliveries (ATC codes J07BB02, J07BB03, J07CA, excluding A/H1N1 pandemic vaccines, Supplementary Table 2) recorded between September 1 of year n and March 31 of year n + 1. Each SIV delivered in a community pharmacy is recorded in the NHIF database. SIV dispensing was considered a proxy for SIV uptake.
Clinical characteristics of cohort members
Cohort members without diabetes in 2005 according to the algorithm mentioned above were considered “patients with newly identified diabetes”. Furthermore, we categorized each patient, each year, according to the characteristics and treatment of her/his diabetes, from data on LTI status and reimbursement claims. Specifically, the reimbursements were those for antidiabetic drugs recorded during the six months before the start of the season under consideration (n/n + 1): type 1 diabetes (LTI status for type 1 diabetes: E10 according to the 10th International Classification of Diseases, ICD-10, and/or treated by insulin at inclusion); other types not treated by antidiabetic drugs or insulin; other types treated by antidiabetic drugs and/or insulin.
To measure comorbidities, we calculated an individual chronic condition score (ICC) for each year of follow-up, based on drug deliveries (excluding antidiabetic drugs). This methodology has previously been published and used in several articles.Citation17,Citation18
We also extracted, for each cohort member, the number of hospital stays each year (between September 1 of year n, and August 31 of year n + 1), for diabetes, diabetes complications, influenza, and influenza complications, and the numbers of visits – separately – with general practitioners (GPs), pulmonologists, endocrinologists, and cardiologists. GPs usually manage most patients with type 2 diabetesCitation6 and refer patients to specialists when they face difficulties or complications. We also extracted information about patients’ changes of GP during follow-up.
Statistical analysis
For objective 1, we used an MSM model and estimated, based on transition intensity (i.e., the instantaneous risks of moving from one state to another), the transition probability : namely, the probability of remaining in the same state r or moving from state r to another state s within a given period of time t (here, a 1-year period: from season n-1/n to season n/n + 1).Citation15 Each season, we considered two states (vaccinated against seasonal influenza, or not), which generates four kinds of transitions (): remaining vaccinated, remaining unvaccinated, starting vaccination, and stopping vaccination. Given the Markov property of our MSM model, the future SIV state is independent of the past state, given the present state and the covariate values. In other words, the probability of SIV the next season depends only on the current state (SIV or no SIV) and the covariate values.
Figure 1. A graphical representation of the two-state Markov model of seasonal influenza vaccination uptake and of the four kind of transitions
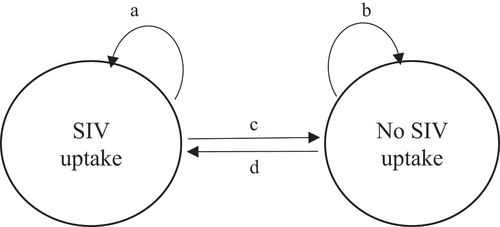
As the A(H1N1) pandemic season (2009/10) had a major impact on SIV coverage rates,Citation5 we could not use a standard MSM model that would assume constant transition intensities over time. We implemented a time-inhomogeneous model with piecewise-constant intensities that makes it possible to take into account specific points in time when an identifiable event (here the 2009 pandemic) may imply a change in intensities of transition for all subjects.Citation19
For objective 2, we used a proportional hazards model to model transition intensities as a function of constant (sex, age at inclusion) and time-varying covariates (type of diabetes, ICC score, hospital stays for diabetes/influenza, and healthcare utilization):
where is the transition intensity from state r to state s;
is the vector of covariates at time t, and
is the vector of regression coefficients, which can be interpreted as a hazard ratio (HR). This model makes it possible to test the effect of covariates on transition intensities, even if some patients have multiple transitions from one state to another.Citation16
For objective 3, we used the transition intensities and covariate coefficient estimates to calculate the total time spent in the “SIV uptake” state over 10 SIV seasons. Given the initial state (SIV/no SIV uptake) and a set of three covariates (age, gender, and type of diabetes and its treatment), we were able to estimate the expected (i.e., predicted by the MSM model) number of seasons with SIV uptake over the next 10 seasonal campaigns for different patient profiles. We selected these three covariates because they were strongly associated with SIV uptake and are easily available to physicians in routine practice.
The goodness of fit of the model was assessed by the likelihood-ratio (LR) test and a graphical comparison of the predicted and observed prevalence rates of each state at each seasonal campaign. As most patients were lost during follow-up because of death, we also tested an empty (without covariates) three state model with “death” as an absorbing state to take the probability of dying during follow-up into account. This model yielded to similar results (results available upon request).
Descriptive statistical analyses were performed with SAS, version 9.4 (SAS Institute Inc., Cary, NC, USA). The MSM was fitted with R 3.5.0 software, and the MSM package version 1.6.6 developed by Jackson.Citation19
Results
Study population characteristics ()
Of the 17,259 patients identified with diabetes in 2006, we included in the cohort 16,206 patients (94%) with at least two years of follow-up over the study period. Mean age at inclusion was 65 ± 14 years, and 46% were women. Fewer than 10% were patients with diabetes newly identified at inclusion, and 10% had type 1 diabetes. The average duration of follow-up was 8.6 ± 2.4 years (see details in Supplementary Table 3). Over the follow-up period, 27% of the initial cohort died and 4% were lost to follow-up. SIV uptake rates were estimated at 51% in 2006/2007 and 57% in 2015/2016.
Table 1. Study cohort characteristics during the first and last season n/n + 1 of follow-up (EGB, France, 2006/07–2015/16, n = 16,026)
Objective 1: transition probability estimates
Over the study period, 75% of the patients had stable SIV behaviors (i.e., never vaccinated, always vaccinated, or had only one SIV transition) (). Between two consecutive seasons, the probability of starting SIV (0.17, 95% confidence interval – CI – 0.17, 0.18) was significantly higher than the probability of stopping it (0.09, 95% CI 0.09, 0.10). The probability of remaining vaccinated (0.91, 95% CI 0.90, 0.91) was significantly higher than the probability of remaining nonvaccinated (0.83, 95% CI 0.82, 0.83). The goodness of fit of our model was satisfactory (Supplementary Figure 1).
Figure 2. Proportion of the study population according to the number of SIV uptake transitions over the 10-year follow-up period (EGB, France, 2006/07–2015/16, n = 16,026)
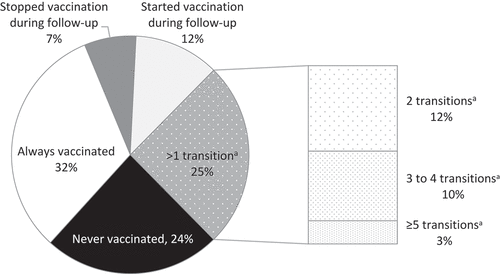
Objective 2: factors associated with starting or stopping SIV ()
Compared with patients who remained nonvaccinated, the probability of starting SIV was higher for men and increased with age. It was also higher for patients with newly identified diabetes in 2006, type 1 diabetes, more comorbidities, or hospitalized for diabetes or influenza since the previous SIV season. Patients with frequent contacts with GPs or at least one contact with a pulmonologist and those who had changed GP since the previous season were also more likely to start SIV.
Table 2. Demographic and clinical characteristics associated with each SIV uptake transition – Hazard ratios and 95% confidence intervals – Two-state Markov model estimates (EGB, France, 2006/07–2015/16, n = 16,026)
Compared with patients who remained vaccinated, the probability of stopping SIV was lower for men and decreased with age. It was also lower for patients with type 1 diabetes or another type of diabetes treated by insulin and/or antidiabetic drugs. Changing GP increased the probability of stopping, as well as starting, SIV, and contacts with endocrinologists and cardiologists reduced the probability of stopping SIV.
Objective 3: expected time spent in the “SIV uptake” state, according to patient profiles
For patients not vaccinated at the beginning of the period, the group with the lowest expected number of seasons with SIV uptake over the 10-season period () (mean = 2.6 seasons) was that of women younger than 65 years with untreated diabetes, and the group with the most vaccinated seasons (mean = 5.7) was that of men aged 65 years or older with type 1 diabetes.
Figure 3. Box-plot diagrams1 for the expected number of seasons with SIV uptake over 10 SIV seasons according to demographic-clinical profiles2 among patients with no SIV uptake at the beginning of the period (Figure 3a) and among patients with SIV uptake at the beginning of the period (Figure 3b) – Two-state Markov model estimates3 (EGB, France, 2006/07–2015/16, n = 16,026).
Abbreviations: F, female; Other noT, other types of diabetes without any treatment; Other T, other type of diabetes treated by insulin and/or antidiabetic drugs; M, men; SIV, seasonal influenza vaccination; T1, type 1 diabetes.
1 The box extends from the 25th percentile to the 75th percentile. The line in the middle is the median (i.e., the 50th percentile). The diamond inside the box is the mean. The whiskers, those two lines at either end, extend from the box as far as the minimum and maximum values, up to 1.5 times the interquartile range (i.e., the distance from the 25th percentile to the 50th).
2 Profiles are ranked in ascending order of the expected (i.e., predicted by the MSM model) number of seasons with SIV uptake for patients not vaccinated at the beginning of the period.
3 For each profile, all remaining covariates (individual chronic condition score, hospital stays for diabetes/influenza, healthcare use) are set to their mean values.
Reading Figure 3a: for a woman not vaccinated at the beginning of the period and younger than 65 years with untreated diabetes, the expected number of seasons with SIV uptake over the next 10 SIV seasons was estimated at 2.6.
Reading Figure 3b: for a woman vaccinated at the beginning of the period and younger than 65 years with untreated diabetes, the expected number of seasons with SIV uptake over the next 10 SIV seasons was estimated at 3.6
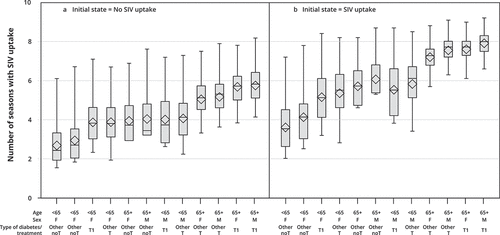
For patients vaccinated at the beginning of the period, the expected number of seasons with SIV uptake was higher for all profiles, but their ranking remained similar ().
Discussion
Among this cohort of patients with diabetes followed up over 10 seasons, the probability of remaining vaccinated or nonvaccinated was far higher than the probability of moving from one state to the other. The probability that a nonvaccinated patient would start SIV during the next season was higher (0.17) than the probability of a vaccinated patient stopping (0.09). Men, older patients, those with type 1 diabetes, treated diabetes or more comorbidities, frequent contacts with GPs or pulmonologists, or with any hospital stay for diabetes or influenza during the previous year were all more likely to start and/or less likely to stop SIV. Changing GP was associated with starting SIV among those non-vaccinated, and stopping it among those who had previously been vaccinated. The mean expected number of seasons with SIV uptake depended on initial SIV state and patients’ demographic and clinical profiles.
Our results demonstrate, consistently with previous findings, that people with diabetes have mainly stable SIV behaviors.Citation6,Citation7,Citation14 The finding that the probability of remaining vaccinated (0.91) was significantly higher than that of remaining unvaccinated (0.83) is somewhat encouraging, even though the order of magnitude of these probabilities did not differ notably. Nonetheless, the probabilities of behavioral shifts were non-negligible in each group and higher for starting to vaccinate than for stopping it. Our results () confirm that both modifiable (medical care) and nonmodifiable factors (aging and clinical deterioration) were associated with increased SIV uptake rates.Citation6,Citation12,Citation14,Citation20 This finding is both intuitive and fortunate and helps to lighten, in part, the dark picture of low SIV coverage in patients with diabetes: low coverage involves, above all, patients with the least serious forms of disease. Our results suggest that any clinical encounter with patients with diabetes, both vaccinated and nonvaccinated, should be taken as a new opportunity to make them aware of the importance of SIV, especially when their diabetes is becoming more severe. Our results also suggest that this is already the case, to some extent, as we found a higher probability of starting SIV among patients hospitalized for either influenza (+35%) or diabetes (+12%).
These results and their clinical implications are important for doctors. First, the probability of starting SIV rose with contacts with GPs and especially with pulmonologists in the last year. These findings confirm the key role of doctors in promoting SIV among at-risk patients.Citation6,Citation12,Citation21 Previous studies have shown that patients trust their physicians stronglyCitation7 and that these professionals can play an important role in improving patients’ knowledge and decision-making process about SIV.Citation22,Citation23 The absence of any similar effect for endocrinologists and cardiologists, however, suggests that these specialists might be missing some opportunities to promote SIV.Citation24 In particular, it is surprising that medical contacts with endocrinologists are not associated with an increase in SIV uptake, as endocrinologists work with patients on behavioral changes in their daily practice, particularly concerning diet or physical activity. Endocrinologists and cardiologists might have a lower perception of the severity of influenza than pulmonologists because the association between influenza-induced complications of diabetes or cardiovascular diseases might appear less frequent, direct or severe than in patients with chronic respiratory diseases.Citation24 These specialists may also have the perception that patients will receive SIV elsewhere, a common explanation for missed vaccination among some specialists.Citation24 Increasing awareness among endocrinologists and cardiologists of the benefits of SIV for people with diabetes (e.g., through guidelines and initial and continuing medical education) is an important goal.
Second, doctors must be aware that some specific events (e.g., intensification of treatment and hospitalization for diabetes or influenza) may present favorable opportunities for encouraging SIV uptake. These events may increase patients’ perception of their own vulnerability toward influenza or of the severity of the disease – both strong facilitators of the adoption of SIV.Citation7 Moreover, they provide a good practical opportunity to meet caregivers involved in therapeutic education programs, provided that these include education about SIV.
Third, our results also suggest that the first visits of new patients may be a critical period for doctors to review their prevention needs (as shown for medicationsCitation25) and promote SIV. We also found, however, that changing GPs may be correlated with stopping SIV as well. Lack of additional data on GPs’ characteristics makes this finding difficult to interpret. Changing GPs might reflect a change in a patient’s commitment to health or attitudes toward healthcare, and doctors should be particularly attentive to these new patients.
Fourth, our results highlight the existence of profiles of patients with contrasting propensities for regular vaccination. For example, we estimated that a woman with diabetes younger than 65 years and with no pharmacological treatment for this disease will be vaccinated only about 3 times over the next 10 seasons (vs around 7 times for a woman 65 years or older with type 1 diabetes). While clinicians should systematically assess whether or not their patients have been vaccinated against the flu, they should also bear these profiles in mind to help them to identify those at high risk of irregular or no SIV uptake. This would help them to anticipate the need to spend more effort and time to focus on them, to understand their motives for potential hesitancy/reluctance, and to propose tailored approaches to address these motives and encourage SIV, as recommended for addressing vaccine hesitancy.Citation26
These results have also several implications for policymakers. According to the Community Guide’s categories of interventions to increase appropriate vaccination,Citation27 strategies to improve SIV rates in target populations should rely on: increasing community demand for SIV (e.g., media campaigns, patient reminders); enhancing access to SIV (e.g., in France, free vaccine, vaccination by nurses since 2008, and by community pharmacists – nationwide implementation starting in 2019); and using provider- or system-based interventions (e.g., pay-for-performance for GPs, where performances includes SIV indicators). Applying these recommendations at the national level has remained insufficient in many countries, and SIV rates have remained far lower than the targets of both WHO and individual nations.Citation5 Further efforts are required. One method, implied by our results, may involve training to enhance the educational skills of physicians and other healthcare workers’ educational skills; it should also provide them with guidance and tools for adapting SIV educational efforts to different patient profiles. No “one size fits all” intervention is likely to exist, and tailored interventions adapted to patients’ characteristics, attitudes, and perceptions may be more effective in fostering SIV.Citation26 For example, providing communication training to healthcare workers, together with tailored information sheets and patient education websites, should be evaluated for increasing SIV uptake, as it has been shown to be an effective strategy for increasing uptake for HPV vaccine.Citation28 As healthcare workers are also prone to vaccine hesitancy,Citation29,Citation30 and SIV coverage remains suboptimal among them in many countries,Citation29–32 health authorities should also promote and evaluate multicomponent interventions to promote SIV among healthcare professionals.
The strengths of this study include its large sample size, and the use of a combination of different criteria to identify patients with diabetes with various clinical features (Supplementary Table 1). Another strength of our study is its 10-year follow-up period, which is, with our previous article based on the same cohort,Citation14 the longest of the studies of SIV behavior over several consecutive seasons. From a statistical standpoint, our study is the first to use an MSM model to estimate transition probabilities for SIV behaviors. Moreover, although several previous studies have examined the factors associated with being vaccinated (or not),Citation6,Citation12,Citation14,Citation20 our approach is the first one to explore associations between shifts in SIV behaviors and several demographic and clinical characteristics. This is essential for identifying opportunities (critical periods and events) to promote SIV. Our model provided a good prediction of SIV rates over the 10-year follow-up period (Supplementary Figure 1), confirming that the Markovian approach is well adapted to modeling SIV state transitions. Moreover, our time-inhomogeneous model with piecewise-constant intensities allowed us to adjust for some variations of the probabilities of SIV uptake during the follow-up period, especially those, now well documented, induced by the 2009 A(H1N1) campaign in France.Citation5
We also acknowledge some limitations. First, vaccinations that took place during occupational medicine visits or at vaccination centers or some nursing homes that buy vaccines for their residents (fewer than 20% of all nursing homesCitation33) are not recorded in the French NHIF databases. However, these limitations are unlikely to affect our results substantially as the vast majority of vaccinations in France are administered by private healthcare workers and are thus recorded in these databases.Citation34 Second, as SIV behavior may vary by social characteristics,Citation35 our results cannot be extrapolated to population categories (e.g., farmers, tradespeople, self-employed individuals) that could not be included in this study because they were first included in the EGB only in 2011. Nonetheless, salaried workers (including those who are retired, and their covered spouses and children) account for 86% of the French population. Third, our study is observational and retrospective and thus cannot draw definitive conclusions about causality. Notwithstanding this limitation, our statistical approach is respectful of the chronological succession of events and therefore more robust because it also limits confounding by reverse causality. That is, SIV may be associated with a significant decrease in risk for hospital admission due to stroke, heart failure, and influenza or pneumonia,Citation36 and an approach that fails to take the order of events into account might easily confound the association between hospitalization for diabetes or its complications and the probability of SIV uptake. Finally, several socioeconomic characteristics (e.g., educational level) and cognitive factors (e.g., beliefs about SIV efficacy and safety) may be associated with changes in SIV behaviors but could not be included in the analysis because they are not recorded in the NHIF databases.
While several trials have assessed the effectiveness of interventions to increase SIV rates (especially among older people), further research is needed to determine how best to target these interventions to specific populations, depending on patient characteristics.Citation37 Further research is also needed to assess the effectiveness of interventions on SIV vaccination that have showed promising results for other vaccines and population groups (e.g., motivational interviewing and web-based tailored educational toolsCitation25,Citation38,Citation39).
Disclosure of potential conflicts of interest
The authors declare that they have no competing interests.
Supplemental Material
Download MS Word (55.5 KB)Acknowledgments
We thank Florence Garry (National Health Insurance Fund, Cnam-TS) and Professor Jean-Luc Pasquié (CHU Montpellier) for their help in defining the algorithms to identify the study population, Daniel Levy-Bruhl (French Public Health Agency) for his contribution to the study design, and Jo Ann Cahn for her help in editing the manuscript.
Supplementary material
Supplemental data for this article can be accessed on the publisher’s website.
Additional information
Funding
References
- Mertz D, Kim TH, Johnstone J, Lam -P-P, Science M, Kuster SP, Fadel SA, Tran D, Fernandez E, Bhatnagar N, et al. Populations at risk for severe or complicated influenza illness: systematic review and meta-analysis. BMJ. 2013;347:f5061. doi:10.1136/bmj.f5061.
- World Health Organization. Fact sheet on influenza (Seasonal); 2018 [accessed 2020 Feb 21]. http://www.who.int/en/news-room/fact-sheets/detail/influenza-(seasonal).
- Rizzo C, Rezza G, Ricciardi W. Strategies in recommending influenza vaccination in Europe and US. Hum Vaccines Immunother. 2018;14:693–98. doi:10.1080/21645515.2017.1367463.
- Loerbroks A, Stock C, Bosch JA, Litaker DG, Apfelbacher CJ. Influenza vaccination coverage among high-risk groups in 11 European countries. Eur J Public Health. 2012;22:562–68. doi:10.1093/eurpub/ckr094.
- Verger P, Fressard L, Cortaredona S, Lévy-Bruhl D, Loulergue P, Galtier F, Bocquier A. Trends in seasonal influenza vaccine coverage of target groups in France, 2006/07 to 2015/16: impact of recommendations and 2009 influenza A(H1N1) pandemic. Euro Surveill. 2018;23. doi:10.2807/1560-7917.ES.2018.23.48.1700801.
- Verger P, Cortaredona S, Pulcini C, Casanova L, Peretti-Watel P, Launay O. Characteristics of patients and physicians correlated with regular influenza vaccination in patients treated for type 2 diabetes: a follow-up study from 2008 to 2011 in southeastern France. Clin Microbiol Infect. 2015;21:930.e1-9. doi:10.1016/j.cmi.2015.06.017.
- Verger P, Bocquier A, Vergélys C, Ward J, Peretti-Watel P. Flu vaccination among patients with diabetes: motives, perceptions, trust, and risk culture - a qualitative survey. BMC Public Health. 2018;18:569. doi:10.1186/s12889-018-5441-6.
- Bianco A, Mascaro V, Zucco R, Pavia M. Parent perspectives on childhood vaccination: how to deal with vaccine hesitancy and refusal? Vaccine. 2019;37:984–90. doi:10.1016/j.vaccine.2018.12.062.
- Dubé È, Farrands A, Lemaitre T, Boulianne N, Sauvageau C, Boucher FD, Tapiero B, Quach C, Ouakki M, Gosselin V, et al. Overview of knowledge, attitudes, beliefs, vaccine hesitancy and vaccine acceptance among mothers of infants in Quebec, Canada. Hum Vaccines Immunother. 2019;15:113–20. doi:10.1080/21645515.2018.1509647.
- Napolitano F, D’Alessandro A, Angelillo IF. Investigating Italian parents’ vaccine hesitancy: A cross-sectional survey. Hum Vaccines Immunother. 2018;14:1558–65. doi:10.1080/21645515.2018.1463943.
- Bocquier A, Fressard L, Cortaredona S, Zaytseva A, Ward J, Gautier A, Peretti-Watel P, Verger P. Social differentiation of vaccine hesitancy among French parents and the mediating role of trust and commitment to health: A nationwide cross-sectional study. Vaccine. 2018;36:7666–73. doi:10.1016/j.vaccine.2018.10.085.
- Jiménez-Garcia R, Lopez-de-Andres A, Hernandez-Barrera V, Gómez-Campelo P, San Andrés-Rebollo FJ, de Burgos-lunar C, Cárdenas-Valladolid J, Abánades-Herranz JC, Salinero-Fort MA. Influenza vaccination in people with type 2 diabetes, coverage, predictors of uptake, and perceptions. Result of the MADIABETES cohort a 7years follow up study. Vaccine. 2017;35:101–08. doi:10.1016/j.vaccine.2016.11.039.
- Caille-Brillet AL, Raude J, Lapidus N, De Lamballerie X, Carrat F, Setbon M. Trends in influenza vaccination behaviours–results from the CoPanFlu cohort, France, 2006 to 2011. Euro Surveill. 2013;18:20628. doi:10.2807/1560-7917.ES2013.18.45.20628.
- Bocquier A, Cortaredona S, Fressard L, Loulergue P, Raude J, Sultan A, Galtier F, Verger P. Trajectories of seasonal influenza vaccine uptake among French people with diabetes: a nationwide retrospective cohort study, 2006–2015. BMC Public Health. 2019;19:918. doi:10.1186/s12889-019-7209-z.
- Kalbfleisch JD, Lawless JF. The analysis of panel data under a Markov Assumption. J Am Stat Assoc. 1985;80:863. doi:10.1080/01621459.1985.10478195.
- Tuppin P, de Roquefeuil L, Weill A, Ricordeau P, Merlière Y. French national health insurance information system and the permanent beneficiaries sample. Rev Epidemiol Sante Publique. 2010;58:286–90. doi:10.1016/j.respe.2010.04.005.
- Cortaredona S, Pambrun E, Verdoux H, Verger P. Comparison of pharmacy-based and diagnosis-based comorbidity measures from medical administrative data. Pharmacoepidemiol Drug Saf. 2017;26:402–11. doi:10.1002/pds.v26.4.
- Huber CA, Szucs TD, Rapold R, Reich O. Identifying patients with chronic conditions using pharmacy data in Switzerland: an updated mapping approach to the classification of medications. BMC Public Health. 2013;13:1030. doi:10.1186/1471-2458-13-1030.
- Jackson CH. Multi-state models for panel data: the MSM package for R. J Stat Software. 2011:38. doi:10.18637/jss.v038.i08.
- Achtymichuk KA, Johnson JA, Al Sayah F, Eurich DT. Characteristics and health behaviors of diabetic patients receiving influenza vaccination. Vaccine. 2015;33:3549–55. doi:10.1016/j.vaccine.2015.05.047.
- Nagata JM, Hernández-Ramos I, Kurup AS, Albrecht D, Vivas-Torrealba C, Franco-Paredes C. Social determinants of health and seasonal influenza vaccination in adults ≥65 years: a systematic review of qualitative and quantitative data. BMC Public Health. 2013;13:388. doi:10.1186/1471-2458-13-388.
- Napolitano F, Della Polla G, Angelillo IF. Knowledge, attitudes, and behaviors of parents towards recommended adult vaccinations: an explanatory survey in the geographic area of Naples, Italy. Int J Environ Res Public Health. 2019;16:2070. doi:10.3390/ijerph16122070.
- Teo LM, Smith HE, Lwin MO, Tang WE. Attitudes and perception of influenza vaccines among older people in Singapore: A qualitative study. Vaccine. 2019;37:6665–72. doi:10.1016/j.vaccine.2019.09.037.
- Davis MM, Wortley PM, Ndiaye SM, Cowan AE, Osta AD, Clark SJ. Influenza vaccine for high-risk non-elderly adults: a national survey of subspecialists. Hum Vaccine. 2008;4:229–33. doi:10.4161/hv.4.3.5516.
- Schuling J, Gebben H, Veehof LJG, Haaijer-Ruskamp FM. Deprescribing medication in very elderly patients with multimorbidity: the view of Dutch GPs. A qualitative study. BMC Fam Pract. 2012;13:56. doi:10.1186/1471-2296-13-56.
- Dubé E, Gagnon D, MacDonald NE. SAGE working group on vaccine hesitancy. Strategies intended to address vaccine hesitancy: review of published reviews. Vaccine. 2015;33:4191–203. doi:10.1016/j.vaccine.2015.04.041.
- The Community Guide. What works. Increasing appropriate vaccination. Evidence-base intervention for your community; 2017 [accessed 2020 Feb 21]. https://www.thecommunityguide.org/sites/default/files/assets/What-Works-Factsheet-Vaccination.pdf.
- Dempsey AF, Pyrznawoski J, Lockhart S, Barnard J, Campagna EJ, Garrett K, Fisher A, Dickinson LM, O’Leary ST. Effect of a health care professional communication training intervention on adolescent human papillomavirus vaccination: a cluster randomized clinical trial. JAMA Pediatr. 2018;e180016. doi:10.1001/jamapediatrics.2018.0016.
- Verger P, Collange F, Fressard L, Bocquier A, Gautier A, Pulcini C, Raude J, Peretti-Watel P. Prevalence and correlates of vaccine hesitancy among general practitioners: a cross-sectional telephone survey in France, April to July 2014. Euro Surveill. 2016;21:30406. doi:10.2807/1560-7917.ES.2016.21.47.30406.
- Wilson R, Zaytseva A, Bocquier A, Nokri A, Fressard L, Chamboredon P, Carbonaro C, Bernardi S, Dubé E, Verger P. Vaccine hesitancy and self-vaccination behaviors among nurses in southeastern France. Vaccine. 2020;38:1144–1151. doi:10.1016/j.vaccine.2019.11.0118.
- Boey L, Bral C, Roelants M, De Schryver A, Godderis L, Hoppenbrouwers K, Vandermeulen C. Attitudes, believes, determinants and organisational barriers behind the low seasonal influenza vaccination uptake in healthcare workers – A cross-sectional survey. Vaccine. 2018;36:3351–58. doi:10.1016/j.vaccine.2018.04.044.
- Napolitano F, Bianco A, D’Alessandro A, Papadopoli R, Angelillo IF. Healthcare workers’ knowledge, beliefs, and coverage regarding vaccinations in critical care units in Italy. Vaccine. 2019;37:6900–06. doi:10.1016/j.vaccine.2019.09.053.
- Agence technique de l’information sur l’hospitalisation. Les coûts en établissement d’hébergement pour personnes âgées dépendantes. Lyon (France): ATIH; 2013.
- Institut de veille sanitaire. Mesure de la couverture vaccinale en France. Bilan des outils et méthodes en l’an 2000. Saint-Maurice, France: Institut de veille sanitaire; 2001.
- Rey D, Fressard L, Cortaredona S, Bocquier A, Gautier A, Peretti-Watel P, Verger P. Vaccine hesitancy in the French population in 2016, and its association with vaccine uptake and perceived vaccine risk–benefit balance. Euro Surveill. 2018;23. doi:10.2807/1560-7917.ES.2018.23.17.17-00816.
- Goeijenbier M, van Sloten TT, Slobbe L, Mathieu C, van Genderen P, Beyer WEP, Osterhaus ADME. Benefits of flu vaccination for persons with diabetes mellitus: a review. Vaccine. 2017;35:5095–101. doi:10.1016/j.vaccine.2017.07.095.
- Thomas RE, Lorenzetti DL. Interventions to increase influenza vaccination rates of those 60 years and older in the community. Cochrane Database Syst Rev. 2018. doi:10.1002/14651858.CD005188.pub4.
- Lemaitre T, Carrier N, Farrands A, Gosselin V, Petit G, Gagneur A. Impact of a vaccination promotion intervention using motivational interview techniques on long-term vaccine coverage: the PromoVac strategy. Hum Vaccines Immunother. 2019;15:732–39. doi:10.1080/21645515.2018.1549451.
- Pot M, Paulussen TG, Ruiter RA, Eekhout I, de Melker HE, Spoelstra ME, van Keulen HM. Effectiveness of a web-based tailored intervention with virtual assistants promoting the acceptability of HPV vaccination among mothers of invited girls: randomized controlled trial. J Med Internet Res. 2017;19:e312. doi:10.2196/jmir.7449.