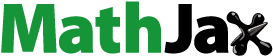
ABSTRACT
VRC01 is being evaluated in the AMP efficacy trials, the first assessment of a passively administered broadly neutralizing monoclonal antibody (bnAb) for HIV-1 prevention. A key analysis will assess serum VRC01-mediated neutralization as a potential correlate of protection. To prepare for this analysis, we conducted a pilot study where we measured longitudinal VRC01 serum concentrations and serum VRC01-mediated neutralization in 47 and 31 HIV-1 uninfected AMP participants, respectively. We applied four different statistical approaches to predict serum VRC01-mediated neutralization titer against Env-pseudotyped viruses, including breakthrough viruses isolated from AMP placebo recipients who became HIV-1 infected during the trial, using VRC01 serum concentration and neutralization potency (IC50 or IC80) of the VRC01 clinical lot against the same virus. Approaches 3 and 4, which utilized pharmacokinetics/pharmacodynamics joint modeling of concentration and neutralization titer, generally performed the best or comparably to Approaches 1 and 2, which, respectively, utilized only measured and model-predicted concentration. For prediction of ID80 titers against breakthrough viruses, Approaches 1 and 2 rendered comparable performance to Approaches 3 and 4, and could be reasonable approaches to adopt in practice as they entail reduced assay cost and less complicated statistical analysis. Our results may be applied to future studies of other bnAbs and bnAb combinations to maximize resource efficiency in serum neutralization titer measurement.
The ongoing phase 2b Antibody Mediated Prevention (AMP) trials (HVTN 704/HPTN 085 and HVTN 703/HPTN 081; ClinicalTrials.gov NCT02716675 and NCT02568215) are the first proof-of-concept studies assessing whether a broadly neutralizing monoclonal antibody (bnAb) – VRC01, which targets the CD4 binding site of the HIV-1 envelope (Env) glycoproteinCitation1,Citation2 – can prevent HIV-1 acquisition in humans. The trials are being conducted in two at-risk cohorts: 1924 women in sub-Saharan Africa (HVTN 703/HPTN 081) and 2699 men and transgender persons who have sex with men (MSM/TG) in the Americas and Switzerland (HVTN 704/HPTN 085). Participants were randomized to receive ten 8-weekly intravenous infusions of 10 mg/kg VRC01, 30 mg/kg VRC01, or placebo; further details of the study design and statistical considerations are given in Gilbert et al.Citation3 The results of the primary efficacy analysis have recently been published.Citation4
Attaining high serum neutralization titers against circulating HIV-1 strains is hypothesized to be important for achieving high prevention efficacy. In support of this hypothesis, day-of-challenge serum neutralization titer of passively immunized bnAbs has been shown to associate strongly with protection of nonhuman primates from simian-human immunodeficiency virus infection.Citation5 A key secondary objective of the AMP trials is to conduct correlates analyses to assess VRC01 markers, including serum neutralization titers at the estimated time of infection against a representative panel of HIV-1 viruses, as predictors of protection against HIV-1 infection via a case–control study. If validated, such a marker could provide an important benchmark that bnAb-inducing vaccines would need to meet in phase 1/2 trials in order to be further considered for advancement into efficacy trials.
In preparation for the AMP correlates analysis, we conducted a pilot study in a subset of VRC01 recipients in the AMP trials who remained HIV-uninfected until the end of the study and were not taking pre-exposure prophylaxis (PrEP) during the study. We measured a total of 1003 VRC01 serum concentrations from n = 47 participants at up to 22 time-points, and a total of 246 50% or 80% inhibitory dose (ID50 or ID80) serum neutralization titers from n = 31 of the 47 participants at up to 8 of the 22 time-points. As it is resource-intensive to longitudinally measure serum neutralization titer, especially against multiple viruses of interest, an important question is whether serum neutralization titer can be predicted using less resource-intensive measurements, e.g. observed serum concentration, or pharmacokinetics model-estimated serum concentration. The latter would allow prediction of serum neutralization titer at the estimated time of infection, with important implications for the correlates analysis. We previously showedCitation6 that, given longitudinally measured serum VRC01 concentrations and the 50% or 80% inhibitory concentration (IC50 or IC80) of the VRC01 clinical lot against a given Env-pseudotyped virus (hereafter, “virus”), we could apply statistical methods to accurately predict serum neutralization titers in HVTN 104,Citation7 a phase 1 trial of VRC01 in healthy HIV-1 uninfected adults. Here we refine the three approaches used in Huang et al.Citation6 and also propose a new approach incorporating instantaneous inhibitory potential (IIP), a measure of antiviral drug activity at a given concentration of the drug that takes into account the slope of the dose–response neutralization curve.Citation8 In addition, we predict VRC01 serum neutralization titer in AMP participant serum samples against breakthrough viruses recovered from AMP participants. This characterization of neutralization titers against contemporaneously transmitted viruses provides valuable data for the interpretation of the primary trial efficacy results.
All AMP participants provided written informed consent. VRC01 recipients who remained HIV-1 negative through at least the week 88 study visit, did not permanently discontinue infusions during follow-up, and were inferred to not have used PrEP as described in Huang et al.Citation9 were eligible for sampling into the pilot study, irrespective of the number of infusions received and the timing of infusions. For each sampled participant, serum concentrations measured at five days post-second infusion (Day 61), every four weeks from Week 4 until Week 80, and at Week 88, along with serum neutralization titer measured at Day 61, Weeks 24 (infusion #4 visit), 28, 48 (infusion #8 visit), 52, 72 (infusion #10 visit), 76, and 88 were included in the analyses. In HVTN 704/HPTN 085, n = 12 participants fitting the criteria for non-PrEP users from each VRC01 dose group were randomly sampled. Within each of those non-PrEP user groups of 12 participants, n = 8 were randomly sampled for serum VRC01 neutralization measurements. In HVTN 703/HPTN 081, n = 12 participants from each VRC01 dose group were sampled. One participant in the high-dose group did not fit the criteria for non-PrEP users; serum VRC01 neutralization data were collected from n = 8 and n = 7 participants randomly chosen from the 12 participants in each of the low and high-dose groups, respectively.
VRC01 concentrations in serum samples of study participants were quantified by an anti-idiotype enzyme-linked immunosorbent assay (ELISA).Citation10 Neutralization activity against HIV-1 viruses by VRC01 (using both the clinical lot of VRC01 in vitro and post-administration VRC01 in serum samples) was measured by the TZM-bl target cell neutralization assay.Citation11,Citation12 IC50 and IC80 concentrations of the clinical lot of VRC01, as well as ID50 and ID80 titers in VRC01 serum samples, were assessed against: one negative control virus, SVA-MLV (for QC purposes); one virus highly sensitive to VRC01-mediated neutralization, CH0505TF.gly4; one tier 2 reference virus, PVO.4;Citation13 and four breakthrough viruses, two from each of the two AMP studies, derived from placebo recipients who became infected during the study. The breakthrough viruses (H703_1471_190s, H703_1750_140Es, H704_1535_030sN, and H704_2544_140eN01) were randomly sampled among all placebo breakthrough viruses to be at least moderately sensitive (IC50 < approximately 1 µg/ml) to VRC01-mediated neutralization. Further characterization of these viruses’ sensitivity to bnAb and non-bnAb neutralization is provided in Table S1.
Four approaches (summarized in ) were investigated for predicting serum ID50 titers against each virus, as well as the geometric mean serum ID50 titer against the four breakthrough viruses. Approaches 1 and 2 do not require observed serum neutralization titers, whereas Approaches 3 and 4 do. Specifically, Approach 1 predicted serum ID50 titers as the measured VRC01 serum concentration divided by the IC50 of the VRC01 clinical lot against each virus, where concentrations below the lower limit of quantification (LLoQ) were replaced by half of the LLoQ. Approaches 2 and 3 are similar to what was described before,Citation6 except that serum concentration (for both approaches) and neutralization titers (for Approach 3 only) below the respective assay LLoQs were not excluded, but contributed to the estimation of parameters in the population pharmacokinetics (popPK) model for Approach 2 and in the joint pharmacokinetics/pharmacodynamics (PK/PD) model for Approach 3 via computing the maximum likelihood estimate of the population parameters based on all the information provided by data both above and below the LLoQ. For the calculation of the likelihood, this method for imputation of data below the LLoQ is equivalent to the M3 method in NONMEM.Citation14 For Approach 2, the popPK model-predictedCitation9,Citation15 VRC01 serum concentration was divided by the IC50 of the VRC01 clinical lot against each virus as the predicted neutralization titer, where unlike Approach 1 predicted concentrations below the LLoQ were taken as is without any truncation. For Approach 3, the relationship between the popPK model-predicted concentration and the observed neutralization titers (i.e. PD outcome) over multiple time-points was modeled using a linear mixed effects PD model with a random intercept and a random slope to account for variabilities across individuals. Approach 4 expanded upon Approach 3 by using longitudinal IIP values instead of serum concentration as the predictor in the PD modeling; IIP was calculated as IIP = -log10(1- cm/(km+ cm), where c = popPK model-predicted VRC01 serum concentration, k = IC50 and m = log10(4)/[log10(IC80) – log10(IC50)]. For both Approach 3 and Approach 4, serum concentration data from all 47 participants were used in the construction of the popPK model, whereas a leave-one-out cross-validation procedure on the PD portion of the joint modeling was employed for an unbiased assessment of the prediction performance based on “left-out” test data not used to train and optimize the PD model. Specifically, within each iteration data from one participant were “left out” as test data and data from the remaining 30 participants were used as training data to construct the PD model; prediction of neutralization titers were obtained from the model for the “'left-out” participant. This procedure was repeated 31 times until every participant was “left out” once and the performance of approaches was evaluated based on the prediction from all 31 iterations. The same four approaches were used for predicting serum ID80 titers, except that IC80 and ID80 values were used in the analysis. All PK/PD modeling was performed in MonolixCitation16 and other analyses in R version 3.5.1.Citation17
Table 1. Summary table of the four approaches for predicting serum neutralization titer of VRC01. For concreteness, serum ID50 titers are considered but all descriptions apply to the prediction of either ID50 or ID80 titers
These four approaches were applied to the analyses of all available serum concentration (Figure S1) and serum neutralization titers (Figures S2-S5) in the pilot study. Of note, not all samples had detectable serum VRC01 concentration (defined as serum concentration > 1.0 µg/mL, the LLoQ of the ELISA assay) and not all samples had detectable VRC01 serum neutralization titers (defined as serum neutralization titer >10, the LLoQ of the TZM-bl target cell neutralization assay). Because the precision of the measurements is expected to impact the performance of the prediction approaches, we evaluated the four approaches separately in the following four sample categories:
No detectable concentration AND no detectable neutralization titer (-, -; “double-negative”);
Detectable concentration BUT no detectable neutralization titer (+, -);
No detectable concentration BUT detectable neutralization titer (-, +); and
Detectable concentration AND detectable neutralization titer (+, +; “double-positive”).
The prediction performance of each of the four approaches was evaluated as follows. As none of the samples in category (1) or category (2) had their observed neutralization titer above the LLoQ of the assay, we reported the classification accuracy in terms of the percent of samples out of total in each category with titers that were correctly predicted to be ≤LLoQ. As all of the samples in category (3) had their observed neutralization titer >LLoQ, we report the classification accuracy in terms of the percent of samples out of the total in this category with titers that were correctly predicted to be >LLoQ. For samples in category (4) because both the concentration and titers were above the LLoQs of the respective assays, we report how well the predicted neutralization titer agrees with the observed neutralization titer, where the extent of agreement is quantified according to the same metrics as in Huang et al.Citation6 [fold difference (FD): the ratio of the observed titer over the predicted titer; relative fold difference (RFD): the difference of the loge-transformed observed and predicted titers divided by the loge-transformed observed titer; relative mean squared error (RMSE): the average of the squared difference between the observed and predicted loge-transformed titers of each approach relative to Approach 1; and concordance correlation coefficients for assessing agreement of repeated measurements (CCCrm)Citation18,Citation19 of the longitudinal observed vs. predicted loge-transformed titers]. Using these metrics, a given prediction is more accurate the closer its FD is to 1, the closer its RFD is to 0, the smaller its RMSE value is, and the closer its CCCrm is to 1.
We first discuss the results for predicting ID50 titer. Approach 3 generally showed the best accuracy in predicting the detectability of neutralization titer against all viruses for samples in categories (1)–(3), and the best performance in predicting the magnitude of neutralization titer for samples in category (4), though with overlapping 95% confidence intervals (CIs) across all four approaches in most cases (); Approaches 1–4 had a similar performance in terms of the binary classification accuracy when all four sample categories were combined (Table S2A). Specifically, for samples in category (1), perfect (100%) classification accuracy was achieved in nearly all of the predictions, with the lowest accuracy still excellent at 86% (Approach 2, H704_1535_030sN) (). For samples that were deemed negative or positive by only one of the two assays [i.e., categories (2) and (3)], the prediction performance of all approaches was generally dampened. Approach 3 was nearly always the best-performing method, with classification accuracies ranging from 69% to 74% across viruses, with the exception of CH0505TF.gly4 where Approach 3 showed lower, albeit still highly accurate, prediction (96% vs 100% by the other three approaches) (). For samples in category (4), the agreement between predicted vs. observed ID50 titers was high (CCCrm ranging from 0.74 to 0.89 across viruses) by Approaches 3 and 4, and intermediate (CCCrm ranging from 0.57 to 0.80 across viruses) by Approaches 1 and 2, with generally slightly improved results for Approach 3 compared to Approach 4 across viruses (). Approaches 3 and 4 also tended to render more consistent prediction performance, exhibited by smaller FD and RFD ranges, and smaller RMSEs compared to those obtained by Approaches 1 and 2, with perhaps slightly better performance by Approach 3 compared to Approach 4 (). These findings suggest that the popPK modeling – and especially the joint PK/PD modeling – best accommodates the entire data range of concentration and neutralization titers, smoothing out the few extreme predictions by Approach 1.
Table 2. Performance of approaches 1–4 for predicting ID50 (Panel A) and ID80 (Panel B) neutralization titers broken down by the status (detectable vs. undetectable, + vs. -) of measured serum concentration and serum neutralization titer
Figure 1. Plots of observed vs. predicted serum neutralization titer for each approach among double positives [category (4) samples]. (a) ID50, (b) ID80. Values in the lower right-hand corner of each plot correspond to CCCrm agreement values, with 95% confidence intervals shown in parentheses. Double positive = participant with detectable (>LLoQ) serum concentration of VRC01 and detectable (>LLoQ) serum VRC01-mediated neutralization. Breakthrough virus geometric mean: predict the geometric mean titer based on the geometric mean of the observed titers across the 4 breakthrough viruses, and the geometric mean of the IC50 values (Panel A) or of the IC80 values (Panel B) of the 4 breakthrough viruses (as if the geometric mean corresponded to a single virus).
![Figure 1. Plots of observed vs. predicted serum neutralization titer for each approach among double positives [category (4) samples]. (a) ID50, (b) ID80. Values in the lower right-hand corner of each plot correspond to CCCrm agreement values, with 95% confidence intervals shown in parentheses. Double positive = participant with detectable (>LLoQ) serum concentration of VRC01 and detectable (>LLoQ) serum VRC01-mediated neutralization. Breakthrough virus geometric mean: predict the geometric mean titer based on the geometric mean of the observed titers across the 4 breakthrough viruses, and the geometric mean of the IC50 values (Panel A) or of the IC80 values (Panel B) of the 4 breakthrough viruses (as if the geometric mean corresponded to a single virus).](/cms/asset/3297dccb-222d-4844-a25a-34867d23a3b0/khvi_a_1908030_f0001_b.gif)
Figure 2. Plots of fold difference (Fold diff.), relative fold difference (Rel. fold diff.), and relative mean squared error (Rel. MSE) for each approach predicting (a) serum ID50 neutralization titers and (b) serum ID80 neutralization titers. Serum neutralization titers were predicted among double positives [category (4) samples] for each of the six tested viruses and for the breakthrough virus geometric mean. Double positive = participant with detectable (>LLoQ) serum concentration of VRC01 and detectable (>LLoQ) neutralization of VRC01. Breakthrough virus geometric mean: predict the geometric mean titer based on the geometric mean of the observed titers across the 4 breakthrough viruses, and the geometric mean of the IC50 values (Panel A) or of the IC80 values (Panel B) of the 4 breakthrough viruses (as if the geometric mean corresponded to a single virus).
![Figure 2. Plots of fold difference (Fold diff.), relative fold difference (Rel. fold diff.), and relative mean squared error (Rel. MSE) for each approach predicting (a) serum ID50 neutralization titers and (b) serum ID80 neutralization titers. Serum neutralization titers were predicted among double positives [category (4) samples] for each of the six tested viruses and for the breakthrough virus geometric mean. Double positive = participant with detectable (>LLoQ) serum concentration of VRC01 and detectable (>LLoQ) neutralization of VRC01. Breakthrough virus geometric mean: predict the geometric mean titer based on the geometric mean of the observed titers across the 4 breakthrough viruses, and the geometric mean of the IC50 values (Panel A) or of the IC80 values (Panel B) of the 4 breakthrough viruses (as if the geometric mean corresponded to a single virus).](/cms/asset/704d228c-261a-49b3-bb28-32c079be1d29/khvi_a_1908030_f0002_b.gif)
We next discuss the results for predicting ID80 titer, which showed a few differences from the ID50 results. For samples in category (1), perfect (100%) classification accuracy was achieved in all predictions (). Samples in category (3) only existed for one virus, CH0505TF.gly4, and classification accuracy was again comparable across the four approaches (100% accuracy for all approaches except for Approach 3 at 96%). However, for samples in category (2) or (4), the performance of Approaches 1 and 2 tended to be more comparable to that of Approaches 3 and 4, and the relative prediction performance of the four approaches appeared to depend somewhat on the neutralization sensitivity of the viruses: for viruses that are less resistant to VRC01 (IC80 < ~1.0 μg/mL), Approach 3 generally showed the best accuracy in predicting the detectability of serum neutralization and the magnitude of serum neutralization titer, followed by nearly indistinguishable differences in performance among Approaches 1, 2 and 4; for viruses that are more resistant against VRC01 (IC80 > ~1.0 μg/mL), Approach 4 generally showed comparable or better performance than the other approaches, with Approaches 1 and 2 being slightly better or comparable to Approach 3 except for PVO.4 in category (4) (). For example, for samples in category (4), similar results were seen: CCCrm for Approaches 1, 2, 3 and 4 against the three viruses with IC80 < ~1 μg/mL was 85%, 82%, 89% and 87%, 81%, 78%, 87% and 84%, and 84%, 87%, 89% and 86%; against the three viruses with IC80 > ~1.0 μg/mL, CCCrm for Approaches 1, 2, 3 and 4 was 80%, 83%, 73% and 81%, 83%, 86%, 78% and 86%, and, 68%, 71%, 88% and 90%. For the breakthrough virus geometric mean, CCCrm was again comparable between Approaches 3 and 4 (83% vs 85%, respectively), followed by Approaches 1 and 2 (70% and 63%, respectively) (). Lastly, compared to the prediction of ID50 titers, the relative merit of Approaches 3 and 4 to Approaches 1 and 2 in terms of smaller FD and RFD ranges, and smaller RMSEs was less obvious for the prediction of ID80 titers except for the breakthrough virus geometric mean (). Similarly, approaches 1–4 had comparable performance in classification accuracy when all four sample categories were combined (Table S2B).
In summary, our results show that incorporating longitudinal measurements of serum concentration and neutralization titers of VRC01 considerably improves post-administration prediction of both the detectability of serum neutralization, and the magnitude of serum neutralization titer. This finding holds true for predicting neutralization against not only reference viruses but also contemporaneously circulating strains of HIV-1. We also found that for samples with detectable concentration and neutralization titer, joint PK/PD modeling using neutralization titers and IIP (i.e., Approach 4) did not show improvement in predicting ID50 titers compared to joint modeling using neutralization titers and serum concentration (i.e., Approach 3), and only incremental improvement in predicting ID80 titers against more resistant viruses. Even though IIP has been proposed to more accurately quantify antiviral activity (compared to IC50 or IC80 alone), our findings suggest that the additional information in IIP is likely only more valuable for viruses that are harder to be neutralized by VRC01 or that serum neutralization titers and IIP may exhibit a relationship that is not best modeled linearly with the number of paired data available in our pilot study. As it is resource-intensive to longitudinally measure serum neutralization titers, it is likely that the latter implication applies to most clinical bnAb studies. In addition, because Approaches 1 and 2 generally also rendered reasonable prediction accuracy, and the merit of Approaches 3 and 4 relative to Approaches 1 and 2 was less obvious for the prediction of ID80 titers against both reference viruses and circulating strains of HIV-1, our analyses suggest that Approaches 1 and 2 could be reasonable approaches in practice as they do not require running neutralization titer assays on serum samples and the statistical analyses are simpler. Lastly, although neither Approach 1 nor Approach 2 obviously outperformed the other (likely due to the relatively small sample size) and both approaches are empirical-based, Approach 2 (which utilizes model-predicted concentration) is generally preferred over Approach 1 when there are sufficient serum concentration data to build a robust PK model. These observations provide valuable support in the choice of approaches for the prediction of serum neutralization titers at the estimated time of infection in case-control studies.
One constraint of our previous studyCitation6 was that the comparison of Approach 3 vs. Approaches 1 and 2 was based on a single tier 2 virus. To increase the scope of the study, here we also predicted serum neutralization titer against four contemporaneously circulating HIV-1 viruses, strengthening the relevance of our conclusion regarding the advantage of Approach 3. Additionally, building on our previous work where we predicted post-administration serum neutralization titer in adults in the US at low risk for HIV infection,Citation6 here we used data from the first efficacy trials of an HIV bnAb (VRC01), which are being conducted in at-risk cohorts in sub-Saharan Africa and the Americas and Switzerland, with important demographic differences from the US cohort. Lastly, our previous study was unable to predict neutralization titers for samples that were not detectable by both the concentration and neutralization titer assays due to its restricted way in handling readouts below the LLoQ of the assays. Here we accommodated the modeling of all samples regardless of whether their readouts were above or below the LLoQs, and the proposed approaches all achieved satisfactory prediction accuracy in classifying the detectability of VRC01 via neutralization activity.
We next discuss some limitations of our study. First, it is unknown whether our prediction methods will be applicable to other bnAbs besides VRC01, or perhaps more importantly, combinations of bnAbs. The latter question is particularly important to address, given the possibilities of synergistic or antagonistic neutralization interactions among multiple bnAbs infused in combination. These possibilities should be thoroughly investigated, for each considered bnAb combination, in both in vitro and animal models prior to clinical consideration. In vitro studies have already identified a number of doubleCitation20 - and tripleCitation21 -bnAb combinations with synergistic neutralization potency; these findings await validation in vivo in passive immunization/SHIV challenge studies. When data become available from ongoing and future trials of other single bnAb or combination bnAb infusions,Citation22 similar approaches can be adapted to analyze data from such studies. Another potential consideration is that resource barriers associated with testing both serum concentrations and serum neutralization titers of multiple bnAbs infused simultaneously may arise, which could lead to a more resource-sparing approach (Approach 1 or 2) outweighing the demonstrated advantages (in terms of prediction accuracy) of the resource-intensive approach (Approach 3 or 4). Second, it is unknown how well our approaches would predict serum neutralization titers in people living with HIV. Given that our approaches are developed using data collected prior to HIV infection, further research is needed to understand the relationship between serum concentration and serum neutralization post acquisition of HIV infection. Lastly, our proposed approaches could be readily applicable to the prediction of neutralization titers in mucosal samples; however, further research is needed to understand whether comparable predictive accuracy is achieved across different biological samples and whether the incorporation of serum data could be used to improve prediction when such data become available.
In conclusion, it is encouraging to confirm that neutralization titers in serum against both reference viruses and breakthrough viruses could be reasonably well predicted. The performance of the proposed approaches achieved satisfactory levels based on serum concentration data from 47 participants, but neutralization titer data from only 31 participants in two different dose groups and two different study cohorts. These findings further solidified assay planning for the AMP marker studies, which will include measurements of serum concentration at all available time-point and neutralization titers at a subset of time-points to enable Approaches 3 and 4 to be used. These results also provide important guidance for the planning of other marker studies in future bnAb and combination bnAb trials.
Disclosure of potential conflicts of interest
The authors have no conflicts of interest to disclose.
Supplemental Material
Download MS Word (23.2 MB)Acknowledgments
The authors thank the HVTN 704/HPTN 085 and HVTN 703/HPTN 081 study participants and study teams for their dedication and contributions.
Supplementary material
Supplemental data for this article can be accessed on the publisher’s website at https://doi.org/10.1080/21645515.2021.1908030.
Additional information
Funding
References
- Wu X, Yang Z-Y, Li Y, Hogerkorp C-M, Schief WR, Seaman MS, Zhou T, Schmidt SD, Wu L, Xu L, et al. Rational design of envelope identifies broadly neutralizing human monoclonal antibodies to HIV-1. Science. 2010;329(5993):856–10. doi:10.1126/science.1187659.
- Zhou T, Georgiev I, Wu X, Yang ZY, Dai K, Finzi A, Do Kwon Y, Scheid JF, Shi W, Xu L, et al. Structural basis for broad and potent neutralization of HIV-1 by antibody VRC01. Science. 2010;329(5993):811–17. doi:10.1126/science.1192819.
- Gilbert PB, Juraska M, deCamp AC, Karuna S, Edupuganti S, Mgodi N, Donnell DJ, Bentley C, Sista N, Andrew P, et al. Basis and statistical design of the passive HIV-1 Antibody Mediated Prevention (AMP) test-of-concept efficacy trials. Stat Commun Infect Dis. 2017;9. doi:10.1515/scid-2016-0001.
- Corey L, Gilbert PB, Juraska M, Montefiori DC, Morris L, Karuna ST, Edupuganti S, Mgodi NM, deCamp AC, Rudnicki E, et al. Two Randomized Trials of Neutralizing Antibodies to Prevent HIV-1 Acquisition. N Engl J Med. 2021 Mar 18;384(11):1003–1014. doi:10.1056/NEJMoa2031738.PMID:33730454.
- Pegu A, Borate B, Huang Y, Pauthner MG, Hessell AJ, Julg B, Doria-Rose NA, Schmidt SD, Carpp LN, Cully MD, et al. A meta-analysis of passive immunization studies shows that serum-neutralizing antibody titer associates with protection against SHIV challenge. Cell Host Microbe. 2019;26:336–46 e3. doi:10.1016/j.chom.2019.08.014.
- Huang Y, Zhang Y, Bailer R, Grunenberg N, Carpp LN, Seaton K, Mayer KH, Ledgerwood J, Corey L, Mascola J, et al. Brief report: prediction of serum HIV-1 neutralization titers after passive administration of VRC01. J Acquir Immune Defic Syndr. 2020;83:434–39. doi:10.1097/QAI.0000000000002272.
- Mayer KH, Seaton KE, Huang Y, Grunenberg N, Isaacs A, Allen M, Ledgerwood JE, Frank I, Sobieszczyk ME, Baden LR, et al. Safety, pharmacokinetics, and immunological activities of multiple intravenous or subcutaneous doses of an anti-HIV monoclonal antibody, VRC01, administered to HIV-uninfected adults: results of a phase 1 randomized trial. PLoS Med. 2017;14:e1002435. doi:10.1371/journal.pmed.1002435.
- Shen L, Peterson S, Sedaghat AR, McMahon MA, Callender M, Zhang H, Zhou Y, Pitt E, Anderson KS, Acosta EP, et al. Dose-response curve slope sets class-specific limits on inhibitory potential of anti-HIV drugs. Nat Med. 2008;14:762–66. doi:10.1038/nm1777.
- Huang Y, Naidoo L, Zhang L, Carpp LN, Rudnicki E, Randhawa A, Gonzales P, McDermott A, Ledgerwood J, Lorenzo MMG, et al. Pharmacokinetics and predicted neutralisation coverage of VRC01 in HIV-uninfected participants of the Antibody Mediated Prevention (AMP) trials. EBioMedicine. 2021;64:103203. doi:10.1016/j.ebiom.2020.103203.
- Ledgerwood JE, Coates EE, Yamshchikov G, Saunders JG, Holman L, Enama ME, DeZure A, Lynch RM, Gordon I, Plummer S, et al. Safety, pharmacokinetics and neutralization of the broadly neutralizing HIV-1 human monoclonal antibody VRC01 in healthy adults. Clin Exp Immunol. 2015;182(3):289–301. doi:10.1111/cei.12692.
- Sarzotti-Kelsoe M, Bailer RT, Turk E, Lin CL, Bilska M, Greene KM, Gao H, Todd CA, Ozaki DA, Seaman MS, et al. Optimization and validation of the TZM-bl assay for standardized assessments of neutralizing antibodies against HIV-1. J Immunol Methods. 2014;409:131–46. doi:10.1016/j.jim.2013.11.022.
- Montefiori DC. Measuring HIV neutralization in a luciferase reporter gene assay. Methods Mol Biol. 2009;485:395–405.
- deCamp A, Hraber P, Bailer RT, Seaman MS, Ochsenbauer C, Kappes J, Gottardo R, Edlefsen P, Self S, Tang H, et al. Global panel of HIV-1 Env reference strains for standardized assessments of vaccine-elicited neutralizing antibodies. J Virol. 2014;88(5):2489–507. doi:10.1128/JVI.02853-13.
- Beal SL. Ways to fit a PK model with some data below the quantification limit. J Pharmacokinet Pharm. 2001;28(5):481–504. doi:10.1023/A:1012299115260.
- Huang Y, Zhang L, Ledgerwood J, Grunenberg N, Bailer R, Isaacs A, Seaton K, Mayer KH, Capparelli E, Corey L, et al. Population pharmacokinetics analysis of VRC01, an HIV-1 broadly neutralizing monoclonal antibody, in healthy adults. MAbs. 2017;9(5):792–800. doi:10.1080/19420862.2017.1311435.
- Monolix version 2019R1. Antony (France): Lixoft SAS; 2019 [accessed 2020 Nov]. http://lixoft.com/products/monolix/.
- R Core Team. R: a language and environment for statistical computing. Vienna (Austria): R Foundation for Statistical Computing; 2017 [accessed 2020 Nov]. https://www.R-project.org/.
- Carrasco JL, King TS, Chinchilli VM. The concordance correlation coefficient for repeated measures estimated by variance components. J Biopharm Stat. 2009;19(1):90–105. doi:10.1080/10543400802527890.
- Carrasco JL, Phillips BR, Puig-Martinez J, King TS, Chinchilli VM. Estimation of the concordance correlation coefficient for repeated measures using SAS and R. Comput Methods Programs Biomed. 2013;109(3):293–304. doi:10.1016/j.cmpb.2012.09.002.
- Miglietta R, Pastori C, Venuti A, Ochsenbauer C, Lopalco L. Synergy in monoclonal antibody neutralization of HIV-1 pseudoviruses and infectious molecular clones. J Transl Med. 2014;12(1):346. doi:10.1186/s12967-014-0346-3.
- Berendam SJ, Styles TM, Morgan-Asiedu PK, Tenney D, Kumar A, Obregon-Perko V, Bar KJ, Saunders KO, Santra S, De Paris K, et al. Systematic assessment of antiviral potency, breadth, and synergy of triple broadly neutralizing antibody combinations against Simian-human immunodeficiency viruses. J Virol. 2020;95(3). doi:10.1128/JVI.01667-20.
- Karuna ST, Corey L. Broadly neutralizing antibodies for HIV prevention. Annu Rev Med. 2020;71(1):329–46. doi:10.1146/annurev-med-110118-045506.