ABSTRACT
High uptake of vaccinations is essential in fighting infectious diseases, including severe acute respiratory syndrome coronavirus 2 (SARS-CoV-2) that causes the ongoing coronavirus disease 2019 (COVID-19) pandemic. Social media play a crucial role in propagating misinformation about vaccination, including through conspiracy theories and can negatively impact trust in vaccination. Users typically engage with multiple social media platforms; however, little is known about the role and content of cross-platform use in spreading vaccination-related information. This study examined the content and dynamics of YouTube videos shared in vaccine-related tweets posted to COVID-19 conversations before the COVID-19 vaccine rollout. We screened approximately 144 million tweets posted to COVID-19 conversations and identified 930,539 unique tweets in English that discussed vaccinations posted between 1 February and 23 June 2020. We then identified links to 2,097 unique YouTube videos that were tweeted. Analysis of the video transcripts using Latent Dirichlet Allocation topic modeling and independent coders indicate the dominance of conspiracy theories. Following the World Health Organization’s declaration of the COVID-19 outbreak as a public health emergency of international concern, anti-vaccination frames rapidly transitioned from claiming that vaccines cause autism to pandemic conspiracy theories, often featuring Bill Gates. Content analysis of the 20 most tweeted videos revealed that the majority (n = 15) opposed vaccination and included conspiracy theories. Their spread on Twitter was consistent with spamming and coordinated efforts. These findings show the role of cross-platform sharing of YouTube videos over Twitter as a strategy to propagate primarily anti-vaccination messages. Future policies and interventions should consider how to counteract misinformation spread via such cross-platform activities.
1. Introduction
The spread of vaccine misinformation over social media has been linked to increased global vaccine hesitancy in the past decade.Citation1–6 In a joint statement with other leading international health organizations, the World Health Organization (WHO) noted that, “the Coronavirus disease (COVID-19) is the first pandemic in history in which technology and social media are being used on a massive scale.”Citation7 The statement cautioned against the “infodemic,” which refers to an overabundance of information and misinformation that can cost lives.Citation7 To design effective communication efforts and policies, it is important to understand vaccination-related social media content and its dynamics. However, multiple factors, not the least of which are the quantity and constant changes in content complicate such understanding. Novel computational tools applied by interdisciplinary teams show promise in advancing knowledge of vaccination-related content on social media. In turn, this knowledge sheds light on public sentiments and sense-making.Citation8–11
Twitter has documented importance in mining public discourse about vaccination.Citation10,Citation12–28 Studies revealed that only a minority of tweets about vaccination opposed vaccination,Citation12,Citation17,Citation29 and these are generated by a relatively small number of sources and disseminated in close-knit social networks that create “echo chambers.”Citation5,Citation30 Therefore, vaccine-opposing tweets are often not the reflection of authentic, individual users’ content but are instead spread through bot-like activity.Citation2 Spreaders of this misinformation include certain governments, politicians, celebrities, and other sources.Citation16,Citation26,Citation29 Other social media that were documented as advancing vaccine-opposing messages include Facebook,Citation31–35 Instagram,Citation36–38 Pinterest,Citation39–41 and YouTube.Citation42–46 Use of specific platforms is associated with different levels of trust in vaccination and intentions to get vaccinated,Citation47 and the vaccine-related content on these platforms differs. For example, in contrast to Twitter’s propagation of largely positive or neutral vaccination sentiments and medically substantiated information,Citation12,Citation17,Citation29 YouTube’s platform promotes mostly anti-vaccination videos with narratives that center on conspiracy theories and claims of civil liberty infringement.Citation42–45
A limitation of previous research that examined vaccine-related social media content was the focus on analyzing discrete social media platforms. Social media users typically utilize multiple media sourcesCitation48,Citation49 as part of media affordances.Citation50 Therefore, it is important to explore cross-platform use, which consists of utilizing one social media platform to disseminate information about the content available on other platforms. Social cybersecurity research documented the role of cross-platform use of social media – especially YouTube – in disinformation campaigns.Citation20,Citation51 However, the role of cross-platform use in dissemination of vaccination-related content is largely unknown.
There is also a gap in the literature about content and spread of vaccination-related conspiracy theories over social media during the COVID-19 pandemic. Times of uncertainty and hardship, such as during pandemics, often lead to an increase in conspiracy theories as individuals attempt to make sense of rapidly unfolding events and fears.Citation52–54 Conspiracy theories are narratives that assign a strong potency of causation to evil forces and that have epistemologies that diverge from scientific methods of knowledge.Citation55 Conspiracy theories provide a powerful negative framing of vaccination,Citation32 and believing conspiracy theories is inversely correlated with the intention to get vaccinated for COVID-19.Citation56 Individuals who are exposed to anti-vaccine conspiracy theories are less likely to intent to vaccinate their children, and the effects of such exposure are long lasting.Citation57,Citation58 Furthermore, beliefs in conspiracy theories are difficult to refute,Citation57 as they provide individuals with a sense of coherence and agency in the face of uncertainty.Citation59 Numerous studies documented the dissemination of COVID-19 related conspiracy tweets,Citation9,Citation23,Citation28,Citation60–63 including a recent study that showed that their spread was consistent with bot-like propagation.Citation63 However, to our knowledge, no previous studies focused on vaccine-related conspiracy theories spread across platforms. Given the importance of YouTube in disseminating vaccination-opposing narratives, including conspiracy theories, it is likely that tweets shared during COVID-19-related conversations about vaccination would include links to related videos. Exploring the spread of vaccination-related information, including the content and propagation of conspiracy theories across platforms, is necessary for understanding this new media environment and designing evidence-based interventions and policies.
In this study, we analyzed tweets that were posted from 1 February 2020 (2 days after the WHO declared the outbreak of COVID-19 to be a public health emergency of international concern) through 23 June 2020. This time frame encompasses the early months of the pandemic prior to the rollout of COVID-19 vaccinations. These early conversations reveal vaccine-opposing strategies that were not influenced by the specific characteristics of the COVID-19 vaccines, including claims about their efficacy and safety. Instead, they have the potential to illuminate the discourse of vaccination sense-making early in the pandemic, including the success of public health sources in disseminating their messages on one side, and established vaccine-opposing forces leveraging the pandemic to advance their agenda on the other.
According to agenda-setting theory,Citation64,Citation65 the salience of media content and its framing are important in shaping public perceptions.Citation66 In the last decade, social media has disrupted past models in which traditional media set the agenda. Both social and traditional media are now available online and interact in complex ways to shape agendas. Yet, it is largely unknown how social media is used to disseminate other media sources in the context of vaccination. Understanding the frequency in which links to YouTube videos are tweeted as part of vaccination-related tweets in COVID-19 related conversations, the temporal dynamics of these tweeted videos, their content, and the frames employed (including conspiracy theories) can reveal agenda-setting processes in this new media environment.Citation67,Citation68 In addition, examining the propagation strategies of frequently tweeted YouTube videos can help identify potential bots and inauthentic propagation. Such inauthentic spread strategies constitute new processes that might impact agenda setting in digital environments. To understand these issues, we posed the following research questions.
To explore the magnitude of this phenomenon, the first question focused on the overall prevalence of cross-platform sharing of YouTube videos in vaccination-related tweets posted to COVID-19 conversations during the early months of the pandemic.
RQ1: What were the absolute and relative frequencies of YouTube videos tweeted as part of COVID-19 conversations during the early months of the pandemic?
YouTube videos that were highly circulated in our data set demonstrate agenda-setting processes. The content of the most tweeted videos therefore demonstrates both the content that certain users chose to share, the sources that were effective in dissemination over Twitter, and the content that users were exposed to. We chose the 20 most tweeted videos to allow an in-depth analysis, while also providing an insight about diverse videos.
We posed research question two:
RQ 2: What were the content and sources of the 20 most tweeted YouTube videos, including sentiments regarding vaccination and conspiracy theories?
As social media content often represents the efforts of inauthentic users, we also sought to identify the specific strategies of spreading the most tweeted YouTube videos. The third research question focused on these strategies.
RQ 3: What characterized the spread of the 20 most tweeted YouTube videos?
Finally, given the dynamic nature of social media, we sought to gain broader knowledge of the content and temporal distribution of videos tweeted in the data set. Research questions four and five were presented:
RQ 4: What were the topics and frames of YouTube videos that were tweeted as part of COVID-19 conversations during the early months of the pandemic?
RQ 5: What was the temporal distribution of YouTube videos that were tweeted as part of COVID-19 conversations during the early months of the pandemic?
2. Methods
2.1. Data
The analysis reported here is part of a larger study that examined vaccination-related tweets.Citation69 The analyzed data were originally collected in two different studies that examined COVID-19 Twitter conversations in the early months of the pandemic. The first data set was based on a collection of tweet IDs gathered using general COVID-19 related keywords, such as “coronavirus” and “Wuhancoronavirus.”Citation70 We used “hydration,”Footnotea a process of gathering all the pertinent information about each tweet into the JavaScript Object Notation (JSON) format fileCitation71 via the Twitter Search APIFootnoteb on all of the tweet IDs. This process populated data from 140 million tweets that were still available on Twitter in August 2020. The second data set included approximately 4.5 million COVID-19-related tweets collected from 29 January 2020 to 23 June 2020Citation72 using Twitter’s Streaming API.Footnotec To include tweets that referred to vaccination, we filtered the data sets using the sub-strings “vax” and “vaccin,” which resulted in 1.6 million English tweets that discussed vaccinations. Both data sets were then filtered to include only the dates of overlap (1 February to 23 June 2020) and to remove any duplicate tweets across the data sets. The final data set included 930,539 unique tweets. To identify tweets that contained URLs linking to YouTube videos, all URLs from the Tweet’s JSON “entities” object of each tweet were extracted.Footnoted shows a graphical flowchart for the data selection and exclusion process.
2.2. YouTube video topic modeling
All transcriptsFootnotee that were available for the YouTube videos were downloaded directly from YouTube.com; 61% (n = 1,280) of the YouTube videos in the data set had a transcript. Examples of videos lacking transcripts include those taken down by YouTube.com, videos without text (e.g., the opening scene of the 1995 film Outbreak), and videos in languages other than English that had the transcript feature disabled. To preprocess the transcripts, we removed common English stop words, and some commonly used phrases were mapped to their representative concept. Each of the terms was then lemmatized, a process that involves mapping each of the words to their base words, which facilitates their analysis as a single concept. Following this pre-processing, we used Latent Dirichlet Allocation (LDA),Citation73 a natural language processing, unsupervised machine learning technique to identify topics in the YouTube transcripts. Footnotef LDA Topic modeling is based on commonly co-occurring words that are likely to belong to a topic. We created a number of different LDA models with varying hyperparameters and used a coherence metricCitation74 to select the final model and number of topics. Each transcript received a probability score that reflected the likelihood that the transcript is related to each topic. We then created word clouds for each topic to visually illustrate keyword frequencies in the transcripts.Citation75 We also analyzed the temporal breakdown of each topic. In addition, we examined the word counts of “Gates,” “autism,” and “5 G” as proxies of known vaccination/COVID-19 conspiracy theoriesCitation2 in each topic.
2.3. Content analysis
In addition to computational methods, content analysis methodsCitation76 were applied in analyzing the 20 most tweeted YouTube videos and the topics generated by the LDA analysis of YouTube transcripts. The coders were pre-medical and medical students who were trained by the first author. The second author (IC) independently coded the content as a verification. First, the 20 most tweeted YouTube videos were coded to identify their source, number of comments, overall sentiment, presence of conspiracy theories, and source. We considered conspiracy theories as explanations to events that involve secretive and malevolent plotting by powerful and evil groups when more plausible explanations are available.Citation77 Intercoder reliability was 100%, and no conflicts needed to be resolved. In analyzing the YouTube topics, two independent coders watched and read 10 transcripts from each topic and independently assigned a descriptive label to each topic. These labels were compared and discussed with the first author, and a shared understanding was achieved.
2.4. Twitter spread analysis
In order to understand the particular Twitter spread strategies used to propagate the most tweeted YouTube videos, we applied social cybersecurity methods to identify coordinated link sharing and coordinated inauthentic behavior between different social media accounts.Citation78,Citation79 To analyze all the tweets that shared one of the top 20 most tweeted YouTube videos, we first excluded retweets and removed from the remaining original tweet mentions, URLs, trailing whitespace, and formatting characters (i.e. ‘\n’). Then, we recorded the following: the numbers of tweets that included a URL to each of the videos, the unique users that tweeted the URL, the unique texts across all of the tweets that contained the URL, the tweets that contained a URL that were tweeted within an hour of the first tweet of the video, and non-reply mentions. Finally, we documented the range of days between the first and last tweets that included these URLs.
3. Results
The results demonstrated the magnitude, temporal distribution, and content of YouTube videos shared within vaccine-related tweets as part of early COVID-19 Twitter conversations. Specifically, this study explored the content and spread of the 20 most tweeted YouTube videos, and the content and frames of all tweeted YouTube videos, including the presence of conspiracy theories.
The first research question examined the absolute and relative frequencies of YouTube videos tweeted as part of COVID-19 Twitter conversations during the early months of the pandemic. A total of 2,097 unique YouTube video URLs were identified. Two percent (n = 3,722) of all tweets that shared a URL had a URL of a YouTube video. YouTube.com was the most persistently tweeted domain. On most days (134 of 143 days, or 93.7%) in our sample, at least one tweet with at least one YouTube URL was tweeted. YouTube was the third most tweeted domain across all of the domains with 4,106 tweets featuring a YouTube URL. Almost 90% of the tweeted videos were only tweeted within a 1-week period, and 75% of videos were only tweeted once.
The second research question focused on the content and sources of the 20 most tweeted YouTube videos, including their sentiments regarding vaccination and conspiracy theories. These results are presented in , with videos ranked-order according to the number of tweets that included their URL.
Table 1. Content and sources of the 20 most tweeted YouTube videos
As shows, one video (ranked 17th) was removed by the time of the analysis. The video transcript was available and analyzed by the team. It indicated that it was produced by Peggy Hall, who has a vlog named “The Healthy American.” The video focused on her objection to mask mandates and mentioned vaccines as unhealthy. Additional information on this video is unavailable. Of the remaining 19 videos, 15 were classified as opposing vaccination, and 15 presented conspiracy theories. Most videos that were classified as opposing vaccination were also classified as featuring a conspiracy theory. The exception included one video that did not focus on vaccines but rather on debunking psychiatry as a conspiracy theory (“Psychiatry: Science or Fraud” ranked 9th). Two other videos were classified as opposing vaccination, as they included messages that doubted the need for vaccination with no conspiracy theory. These consisted of a video (ranked 6th) that featured President Trump saying: “I feel about vaccines like I feel about tests, this is all going to go away without the need for a vaccine,” and a CNBC video (ranked 11th) reporting that the vaccine is unlikely to be produced or helpful in combating the pandemic. Moreover, 4 of these videos (ranked 9th, 13th, 14th and 19th) were originally produced and circulated years before the pandemic. In addition, the most tweeted video and the third most tweeted video did not relate to the pandemic, for a total of 7 videos that contained vaccine-opposed content or other conspiracy theories unrelated to the current pandemic.
The sources of the videos were diverse. Traditional media presented only three of the most tweeted YouTube videos. These included two CNBC videos (ranked 11th and 16th) and one by the Guardian (ranked 6th). The most tweeted video was produced by RT (Russian TV) America, a media outlet funded and produced in English by the Russian government. This video presented Robert F. Kennedy, Jr., the chairman of an anti-vaccine advocacy group. In it, he alleged corruption in the Centers for Disease Control and Prevention (CDC), including that the CDC has allegedly engaged in silencing whistleblowers who proved that vaccines caused autism and other epidemics, along with additional conspiratorial claims. The vlog “The Corbett Report” created 5 of the 20 most tweeted videos (ranked 5th,7 th,8 th, and 10th). In his vlogs, Mr. Corbett presented himself as a Canadian English teacher living in Japan. Other sources included vlogs based in different countries. All 15 videos that presented conspiracy theories were posted by non-official sources, such as vlogs and YouTube channels, with RT as the only source overtly created by a government.
The analysis further revealed that the conspiracies depicted in these videos were explicitly stated. They included narratives about Bill Gates planning the pandemic and benefiting from vaccinations, and the CDC as an organization aiming to hurt the public by knowingly administering harmful vaccinations. RT, the Corbett Report, and the Informed Consent Action Network presented a news-like set up that resembled mainstream newsrooms or scientific broadcasts. Other videos documented real or manufactured communication between officials. These included a video titled “Funvax,” an old hoax claiming to show a lecture to the Pentagon about a vaccine that would treat religious extremists by targeting their brain.Citation80 Another was an edited video of the CDC deliberation and subsequent approval of a hepatitis B vaccine that increased the risk for acute myocardial infarction. One video’s title claimed it was in Hindi, but in fact had content in Urdu (as verified by two Hindi/Urdu speakers) following 5 minutes of English content.
The third research question centered on the spread of the 20 most tweeted YouTube videos in the data set, as evident in the tweets sharing these URLs. The results are presented in .
Table 2. Tweets spreading the 20 most tweeted YouTube videos
As the table reveals, three videos (#17: [Removed], #19 “Pentagon briefing on removing the God Gene -Funvax,” and #12 “Agenda 21: Global Depopulation, Global Enslavement, One World Totalitarian Government (Hindi)”) were propagated over tweets that originated from one account and were typically tweeted within less than 1 hour. For each video, the texts of these tweets were identical. The only variations included the number of mentions and users referred to in those mentions. For example, two of the tweets that shared the second-most tweeted video (“Coronavirus, the potential for a vaccine, and the cytokine storm”) are detailed below:
@davidicke Remember this Video?
“Unexpected” results were: Disabling other Free-will Brain-functions to create Slaves (Closed Minded, Obeying, Limiting Imagination, etc).
Why some can’t Awaken to Reality.
Why “They” desire to push COVID vaccination ASAP …
(Not Bill)
@Ujalacygnus @shuchinbajaj Remember this Video?
“Unexpected” results were: Disabling other Free-will Brain-functions to create Slaves (Closed Minded, Obeying, Limiting Imagination, etc).
Why some can’t Awaken to Reality.
Why “They” desire to push COVID vaccination ASAP …
(Not Bill)
This spread strategy indicates that individual accounts were spreading the videos by tweeting the same message over multiple tweets and using mentions to draw other users’ attention to their tweets. This is consistent with spamming strategies (also referred to as flooding), which is a common tactic employed by Twitter bots and in spreading disinformation.Citation81,Citation82 Moreover, the second and tenth most tweeted videos had more unique users tweeting the videos than unique texts of tweets that had videos, indicating likely coordination between different accounts.
The fourth research question examined the topics and frames of YouTube videos that were tweeted in the data set, including their frequency over time. The topic modeling analysis of the transcripts provided a final LDA model with 12 topics and had a coherence of 0.40. The Appendix displays the twenty most common words for each of the topics.
presents the summary of the content analysis and the number of YouTube videos included in each topic in descending order based on the number of videos in each topic. As the table shows, only topics 3 and 4 were consistently positive or neutral in depicting vaccination. The other topics consisted of videos that were either opposed to vaccinations (topics 1, 5, 8, and 12) or included a mixture of videos with different sentiments toward vaccination. Some topics included both pro- and anti-vaccination videos (topics 2, 6, 7, and 9). The three topics with the lowest number of videos were largely opposed to vaccination but also included some videos that supported vaccinations (topics 10 and 12) or that were neutral (topic 11).
Table 3. Topics of YouTube transcripts by order of magnitude
The analysis also documented the frequency across the topics of “Gates,” “Autism,” and “5 G” as proxies of conspiracy theories. The qualitative analysis demonstrated that these topics were consistent with conspiracy theories depicting Bill Gates as planning the pandemic in order to control the population and benefit from the vaccine, rehashing the myth that vaccinations cause autism spectrum disorder, and claims that fifth generation (5 G) cellphone towers caused the pandemicCitation2,Citation62 (presented in ). The quantitative analysis depicted in the table shows that one out of six transcripts referred to Bill Gates (15.6%, n = 200), whereas “5 G” and “autism” were mentioned in 90 (7%) transcripts each. These references dominated topic 8 and were also prevalent in topic 1.
Word clouds with the top words in the data set and in 5 sample topics are presented in .
Figure 2. Word clouds of the most common terms from the YouTube video transcript topics. A word’s relative size indicates its relative usage for a given topic of transcripts (larger = more usage).
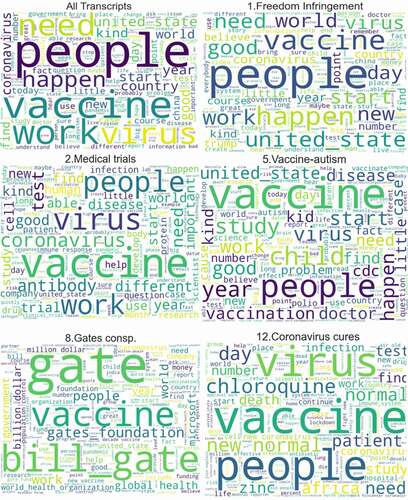
Finally, the fifth research question examined the temporal distribution of the videos. The temporal dynamics of the above topics are displayed in , which shows the proportion of each topic per each week in the data set.
Figure 3. Temporal heatmap of the YouTube transcript topics used in each week of the data. A darker cell indicates a greater proportion of YouTube videos from that topic being used that week.
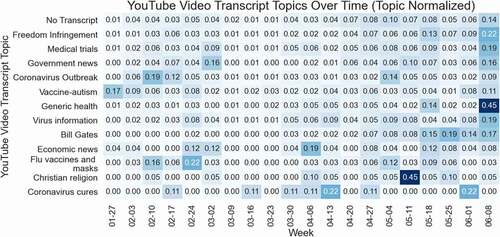
shows the temporal dynamics by weeks of the different topics, indicating notable changes in the relative frequencies of certain topics over time. Specifically, videos that advanced the claim that vaccines cause autism dominated the first week but were quickly replaced as a dominant frame. While this frame decreased in prevalence over the following weeks, it did not disappear, and was the fifth most common frame overall.
The most tweeted topic over time framed vaccinations as conspiracies that aimed to infringe on individual freedom and civil rights. This topic was detected from the first week and increased rather steadily with a spike in the last week of April and in the first three weeks of June. Medical trials, the second-most common framing was also tweeted throughout the data set but spiked in March and April 2020. Similarly, the third- and fourth-most tweeted topics of government news and news about the outbreak were prominent in the sample until mid-May 2020. In May to June 2020, videos featuring Bill Gates and infringement on freedom – two topics that advanced conspiracy theories and resistance of public health messages – became the most tweeted topics.
4. Discussion
This study examined the prevalence, propagation, dynamics, and content of YouTube videos shared in vaccination-related tweets that were posted in early COVID-19 conversations. The findings indicate that sharing links to YouTube videos was a common practice in the months following the declaration of the pandemic and that these videos typically opposed vaccination. The findings also captured a “bursty” pattern of sharing these videos, that is, links to a rather small number of YouTube videos were tweeted for short durations of time. This cross-platform sharing revealed both the enduring importance of legacy media and the emergence of non-traditional media outlets in the production of vaccine-related content. It therefore exemplifies the disruption brought by digital media and online publics. Whereas mass media dictated the public agenda in the past,Citation64 today social media both “rehash” mass media content and allow for new topics and non-elite, fringe voices to emerge.Citation68 These processes were previously documented in other contexts,Citation67,Citation68 and this study extends understanding of agenda setting over Twitter to a public health context. Whereas political communication scientists hailed the potential of social media to democratize civic engagement,Citation68 the picture that emerged from this analysis is concerning from a public health perspective. Anti-vaccination sentiments and conspiracy theories were evident in the YouTube videos shared in the early months of the COVID-19 pandemic. This finding supports and extends previous studies that reported a strong anti-vaccination sentiment expressed on this platform.Citation42–45
These results reveal that the anti-vaccination movement is using a hybrid strategy of tweeting links of YouTube videos to advance their messages and engage users. It is possible that tweeting vaccine-opposing YouTube videos is a tactic to overcome changes to the YouTube recommendation algorithms that attempt to decrease the propagation of vaccine-opposing messages.Citation45 Bot-like activities were previously documented in tweeting vaccine-opposing messagesCitation16,Citation17 and more recently in COVID-19-related tweets.Citation63 This study contributes to current research by showing that anti-vaccination advocates are using various tweeting strategies to boost the spread of these videos, including spamming and use of mentions. These strategies were recently identified in global disinformation campaigns,Citation51 and they could indicate the influence of global forces in disseminating anti-vaccine disinformation.Citation26,Citation83 The findings also point to the salience of international vaccine-opposing content in videos spread over tweets in English. The prominence of RT reveals the unabashed intervention of the Russian government in promoting anti-vaccination misinformation and conspiracy theories.Citation16,Citation26
These findings are important in underscoring the relentless efforts and nimble strategies of the anti-vaccination movement. All of the tweets included in this study were parts of COVID-19 conversations posted many months before any concrete information about COVID-19 vaccines was available to the public. Our data clearly show that vaccine-opposing entities utilized COVID-19 conversations to promote their messages from the first week when the pandemic was announced. Therefore, they expose efforts of anti-vaccination advocates to “hijack” conversations about the pandemic to advance their agenda rather than to raise sincere concerns. These efforts were carried out as a tragic global crisis was unfolding and lives were lost.
Our study is also important for identifying specific frames and their persistence over time. Whereas the long-standing hoax of framing vaccinations as causing autismCitation84 was the first main frame of these videos, they quickly switched to framing vaccinations as infringing on individual freedom and the creation of new frames featuring Bill Gates as the major villain behind the pandemic. While another study examined depiction of vaccination on YouTube,Citation85 this is the first that examined framing in a large number of YouTube videos over time during the pandemic.
Unfortunately, health organizations were not the leading information sources driving the agenda in our data. While they were depicted in news that were shared over tweets, they did not directly communicate with the public via cross-platform sharing of YouTube videos over Twitter. The absence of evidence-based information communicated by public health figures is alarming, especially in view of the prominence of videos produced by alternative media sources that oppose vaccination and spread misinformation and conspiracy theories.
The strengths of this study stem from its analysis of a large data set that was collected during a historically important period. Moreover, we employed cutting-edge, interdisciplinary computational methods and human coding to investigate a previously unexplored communication strategy within vaccine discourse on Twitter. However, this work is not without limitations. First, our methods allow for exploring online content, and do not establish relationship between this content and online publics’ vaccine hesitancy. Moreover, tweets were collected by searching common vaccine-related keywords and hashtags. While these keywords and hashtags were identified following an extensive literature review and analysis of tweets by multiple research teams, it is possible that some emerging keywords and hashtags were not included. Additional limitations are grounded in our focus on tweets in English during a specific time frame. Future studies should expand research to include additional languages and time frames, particularly during and following the rollout of the COVID-19 vaccines. In addition, many vaccine-related tweets and videos – particularly those advancing vaccine-opposing messages – were deleted by Twitter and YouTube by the time of analysis. Hence, the actual number of anti-vaccination tweets and videos shared is likely higher than what we were able to report, and their content might be different from what was collected. Similarly, we might have under-reported the number of unique texts across all tweets, as we used computational methods to search for exact text matches. Notably, the time lapses between these tweets were significantly longer than reported for other coordinated inauthentic behaviors of link sharing.Citation78 While there is some evidence of coordination in sharing the videos between user accounts, we cannot determine the strength and degree of inauthenticity of this strategy in spreading the videos.
Finally, we focused on vaccine-related tweets that were part of COVID-19 Twitter conversations. Although our data set is unique in including all related tweets rather than a sample, our findings do not apply to vaccine-related discourse on Twitter that was not part of the pandemic discourse.
5. Conclusions
Although YouTube claimed to have reduced the availability of misinformation including anti-vaccination videos on its platform years before the data collection period,Citation43 this study documented that it continued to feature vaccine-opposing content, misinformation, and conspiracy theories early in the pandemic. It is therefore paramount that more attention be given to censoring anti-vaccination content, as well as to creating and propagating scientific evidence and clear messages about vaccine efficacy and safety. Such messages should include narratives and information that accurately contextualize the risks and benefits of vaccinations. The absence of such messages and policies early in the pandemic likely helped conspiracy theories to flourish. Accurate and transparent information that refutes claims made in vaccination-opposing videos are necessary in preventing individuals who are vaccine hesitant from joining those who actively oppose vaccinations.
The findings of this work pave the way for future studies that would answer additional questions. First, researchers should expand the scope of this study by examining URLs shared after June 2020, especially as new COVID-19 vaccines were approved and disseminated. Given the global importance of the pandemic and vaccinations, studies should also increase the scope of analysis to include languages other than English. Research should also examine relationships between video visuals, content, and dissemination. Finally, we call for hypothesis-driven communication interventions to measure how and why anti-vaccination messages propagate over social media and that attempt to prevent propagation and instead advance scientifically accurate content. Such future interventions should not focus on one social media platform; rather, they should consider and integrate cross-platform use for message sharing.
Abbreviations
Supplemental Material
Download MS Word (24.5 KB)Acknowledgments
We would like to thank Melanie Catron, Zohar Griffith, Kenneth Hafer, and Paulina Majewska for their help in coding the data.
Supplementary material
Supplemental data for this article can be accessed on the publisher’s website at https://doi.org/10.1080/21645515.2021.2003647.
Disclosure statement
No potential conflict of interest was reported by the author(s).
Additional information
Funding
Notes
References
- Hernandez RG, Hagen L, Walker K, O’Leary H, Lengacher C. The COVID-19 vaccine social media infodemic: healthcare providers’ missed dose in addressing misinformation and vaccine hesitancy. Hum Vaccin Immunother. 2021;17(9):2962–2964. doi:10.1080/21645515.2021.1912551.
- Puri N, Coomes EA, Haghbayan H, Gunaratne K. Social media and vaccine hesitancy: new updates for the era of COVID-19 and globalized infectious diseases. Hum Vaccin Immunother. 2020;16(11):2586–93. doi:10.1080/21645515.2020.1780846.
- Wiysonge CS, Ndwandwe D, Ryan J, Jaca A, Batouré O, Anya BPM, Cooper S. Vaccine hesitancy in the era of COVID-19: could lessons from the past help in divining the future? Hum Vaccin Immunother. 2021;1–3. doi:10.1080/21645515.2021.1893062.
- Blume S. A nti-vaccination movements and their interpretations. Soc Sci Med. 2006;62(3):628–42. doi:10.1016/j.socscimed.2005.06.020.
- Dredze M, Broniatowski DA, Smith M, Hilyard KM. Understanding vaccine refusal: why we need social media now. Am J Prev Med. 2016;50(4):550. doi:10.1016/j.amepre.2015.10.002.
- Ahmed N, Quinn SC, Hancock GR, Freimuth VS, Jamison A. Social media use and influenza vaccine uptake among White and African American adults. Vaccine. 2018;36(49):7556–61. doi:10.1016/j.vaccine.2018.10.049.
- Joint statement by WHO U U, UNDP, UNESCO, UNAIDS, ITU, UN Global Pulse, and IFRC. Managing the COVID-19 infodemic: Promoting healthy behaviours and mitigating the harm from misinformation and disinformation; 2020.
- Sinnenberg L, Buttenheim AM, Padrez K, Mancheno C, Ungar L, Merchant RM. Twitter as a tool for health research: a systematic review. Am J Public Health. 2017;107(1):e1–e8. doi:10.2105/AJPH.2016.303512.
- Rains SA, Leroy G, Warner EL, Harber P. Psycholinguistic markers of COVID-19 conspiracy tweets and predictors of tweet dissemination. Health Commun. 2021;1–10. doi:10.1080/10410236.2021.1929691.
- Criss S, Nguyen TT, Norton S, Virani I, Titherington E, Tillmanns EL, Kinnane C, Maiolo G, Kirby AB, Gee GC, et al. Advocacy, hesitancy, and equity: exploring U.S. Race-related discussions of the COVID-19 vaccine on Twitter. Int J Environ Res Public Health. 2021;18(11):5693. doi:10.3390/ijerph18115693.
- To QG, To KG, Huynh VAN, Nguyen NTQ, Ngo DTN, Alley SJ, Tran ANQ, Tran ANP, Pham NTT, Bui TX, et al. Applying machine learning to identify anti-vaccination Tweets during the COVID-19 pandemic. Int J Environ Res Public Health. 2021;18(8):4069. doi:10.3390/ijerph18084069.
- Love B, Himelboim I, Holton A, Stewart K. Twitter as a source of vaccination information: content drivers and what they are saying. Am J Infect Control. 2013;41(6):568–70. doi:10.1016/j.ajic.2012.10.016.
- Massey PM, Leader A, Yom-Tov E, Budenz A, Fisher K, Klassen AC. Applying multiple data collection tools to quantify human papillomavirus vaccine communication on Twitter. J Med Internet Res. 2016;18(12):e318. doi:10.2196/jmir.6670.
- Tomeny TS, Vargo CJ, El-Toukhy S. Geographic and demographic correlates of autism-related anti-vaccine beliefs on Twitter, 2009-15. Soc Sci Med. 2017;191:168–75. doi:10.1016/j.socscimed.2017.08.041.
- Blankenship EB, Goff ME, Yin J, Tse, ZT, Fu, KW, Liang, H, Saroha, N, Fung, IC. Sentiment, contents, and retweets: a study of two vaccine-related Twitter datasets. Perm J. 2018;22:17–138.
- Broniatowski DA, Jamison AM, Qi S, AlKulaib L, Chen T, Benton A, Quinn SC, Dredze M. Weaponized health communication: Twitter bots and Russian trolls amplify the vaccine debate. Am J Public Health. 2018;108(10):1378–84. doi:10.2105/AJPH.2018.304567.
- Yuan X, Schuchard RJ, Crooks AT. Examining emergent communities and social bots within the polarized online vaccination debate in Twitter. Social Media+ Soc. 2019;5:2056305119865465.
- Featherstone JD, Ruiz JB, Barnett GA, Millam BJ. Exploring childhood vaccination themes and public opinions on Twitter: a semantic network analysis. Telematics Inf. 2020;54:101474. doi:10.1016/j.tele.2020.101474.
- Himelboim I, Xiao X, Lee DKL, Wang MY, Borah P. A social networks approach to understanding vaccine conversations on Twitter: network clusters, sentiment, and certainty in HPV social networks. Health Commun. 2020;35(5):607–15. doi:10.1080/10410236.2019.1573446.
- Horawalavithana S, Ng KW, Iamnitchi A. Twitter is the megaphone of cross-platform messaging on the white helmets. International Conference on Social Computing, Behavioral-Cultural Modeling and Prediction and Behavior Representation in Modeling and Simulation; 2020. Springer. p. 235–44.
- Jamison A, Broniatowski DA, Smith MC, Parikh KS, Malik A, Dredze M, Quinn SC. Adapting and extending a typology to identify vaccine misinformation on Twitter. Am J Public Health. 2020;110(S3):S331–S9. doi:10.2105/AJPH.2020.305940.
- Milani E, Weitkamp E, Webb P. The visual vaccine debate on Twitter: a social network analysis. Media Commun. 2020;8(2):364–75. doi:10.17645/mac.v8i2.2847.
- Nuzhath T, Tasnim S, Sanjwal RK, Trisha, NF, Rahman, M, Mahmud, SMF, Arman, A, Chakraborty, S, Hossain, MM. COVID-19 vaccination hesitancy, misinformation and conspiracy theories on social media: a content analysis of Twitter data; SocArXiv 2020.
- Righetti N. Health politicization and misinformation on Twitter. A study of the Italian Twittersphere from before, during and after the law on mandatory vaccinations; 2020.
- Tavoschi L, Quattrone F, D’Andrea E, Ducange P, Vabanesi M, Marcelloni F, Lopalco PL. Twitter as a sentinel tool to monitor public opinion on vaccination: an opinion mining analysis from September 2016 to August 2017 in Italy. Hum Vaccin Immunother. 2020;16(5):1062–69. doi:10.1080/21645515.2020.1714311.
- Walter D, Ophir Y, Jamieson KH. Russian Twitter accounts and the partisan polarization of vaccine discourse, 2015–2017. Am J Public Health. 2020;110(5):718–24. doi:10.2105/AJPH.2019.305564.
- Prieto Santamaria L, Tuñas JM, Fernandez Peces-Barba D, Jaramillo A, Cotarelo M, Menasalvas E, Conejo Fernández A, Arce A, Gil de Miguel A, Rodríguez González A, et al. Influenza and measles-MMR: two case study of the trend and impact of vaccine-related Twitter posts in Spanish during 2015-2018. Hum Vaccin Immunother. 2021:1–15. doi:10.1080/21645515.2021.1877597
- Thelwall M, Kousha K, Thelwall S. Covid-19 vaccine hesitancy on English-language Twitter. Profesional de la información (EPI). 2021;30(2): e300212. doi:10.3145/epi.2021.mar.12.
- Bonnevie E, Gallegos-Jeffrey A, Goldbarg J, Byrd B, Smyser J. Quantifying the rise of vaccine opposition on Twitter during the COVID-19 pandemic. J Commun Healthc. 2020;1–8. doi:10.1080/17538068.2020.1822726.
- Cossard A, Morales GDF, Kalimeri K, Mejova Y, Paolotti D, Starnini M. Falling into the echo chamber: the Italian vaccination debate on Twitter. Proceedings of the International AAAI conference on web and social media; 2020. p. 130–40.
- Hoffman BL, Felter EM, Chu K-H, Shensa A, Hermann C, Wolynn T, Williams D, Primack BA. It’s not all about autism: the emerging landscape of anti-vaccination sentiment on Facebook. Vaccine. 2019;37(16):2216–23. doi:10.1016/j.vaccine.2019.03.003.
- Klimiuk K, Czoska A, Biernacka K, Balwicki Ł. Vaccine misinformation on social media–topic-based content and sentiment analysis of Polish vaccine-deniers’ comments on Facebook. Hum Vaccin Immunother. 2021;17(7):2026–35. doi:10.1080/21645515.2020.1850072.
- Tustin JL, Crowcroft NS, Gesink D, Johnson I, Keelan J, Lachapelle B. User-driven comments on a Facebook advertisement recruiting Canadian parents in a study on immunization: content analysis. JMIR Public Health Surveillance. 2018;4(3):e10090. doi:10.2196/10090.
- Orr D, Baram-Tsabari A, Landsman K. Social media as a platform for health-related public debates and discussions: the Polio vaccine on Facebook. Isr J Health Policy Res. 2016;5(1):1–11. doi:10.1186/s13584-016-0093-4.
- Buller DB, Walkosz BJ, Berteletti J, Pagoto SL, Bibeau J, Baker K, Hillhouse J, Henry KL. Insights on HPV vaccination in the United States from mothers’ comments on Facebook posts in a randomized trial. Hum Vaccin Immunother. 2019;15(7–8):1479–87. doi:10.1080/21645515.2019.1581555.
- Kearney MD, Selvan P, Hauer MK, Leader AE, Massey PM. Characterizing HPV vaccine sentiments and content on Instagram. Health Educ Behav. 2019;46(2_suppl):37S–48S. doi:10.1177/1090198119859412.
- Basch CH, MacLean SA. A content analysis of HPV related posts on Instagram. Hum Vaccin Immunother. 2019;15(7–8):1476–78. doi:10.1080/21645515.2018.1560774.
- Massey PM, Kearney MD, Hauer MK, Selvan P, Koku E, Leader AE. Dimensions of misinformation about the HPV vaccine on Instagram: content and network analysis of social media characteristics. J Med Internet Res. 2020;22(12):e21451. doi:10.2196/21451.
- Guidry JP, Carlyle K, Messner M, Jin Y. On pins and needles: how vaccines are portrayed on Pinterest. Vaccine. 2015;33(39):5051–56. doi:10.1016/j.vaccine.2015.08.064.
- Guidry J, Messner M. Social media crisis commun. In: Austin, L, and Jin, Y, editors. Social media and crisis communication. (New York, NY). 2017. p. 267–279.
- Guidry JP, Vraga EK, Laestadius LI, Miller CA, Occa A, Nan X, Ming HM, Qin Y, Fuemmeler BF, Carlyle KE, et al. HPV vaccine searches on Pinterest: before and after Pinterest’s actions to moderate content. Am J Public Health. 2020;110(S3):S305–S11. doi:10.2105/AJPH.2020.305827.
- Ache KA, Wallace LS. Human papillomavirus vaccination coverage on YouTube. Am J Prev Med. 2008;35(4):389–92. doi:10.1016/j.amepre.2008.06.029.
- Briones R, Nan X, Madden K, Waks L. When vaccines go viral: an analysis of HPV vaccine coverage on YouTube. Health Commun. 2012;27(5):478–85. doi:10.1080/10410236.2011.610258.
- Song MY-J, Gruzd A. Examining sentiments and popularity of pro-and anti-vaccination videos on YouTube. Proceedings of the 8th International Conference on Social Media & Society. 2017; Toronto, Canada. p. 1–8.
- Donzelli G, Palomba G, Federigi I, Aquino F, Cioni L, Verani M, Carducci A, Lopalco P. Misinformation on vaccination: a quantitative analysis of YouTube videos. Hum Vaccin Immunother. 2018;14(7):1654–59. doi:10.1080/21645515.2018.1454572.
- Żuk P, Żuk P. Right-wing populism in Poland and anti-vaccine myths on YouTube: political and cultural threats to public health. Glob Public Health. 2020;15(6):790–804. doi:10.1080/17441692.2020.1718733.
- Jennings W, Stoker G, Bunting H, Valgarðsson VO, Gaskell J, Devine D, McKay L, Mills MC. Lack of trust, conspiracy beliefs, and social media use predict COVID-19 vaccine hesitancy. Vaccines. 2021;9(6):593. doi:10.3390/vaccines9060593.
- Kulshrestha J, Zafar M, Noboa L, Gummadi K, Ghosh S. Characterizing information diets of social media users. Proceedings of the International AAAI Conference on Web and Social Media; 2015; University of Oxford, Oxford, UK.
- Tandoc EC Jr, Lou C, Min VLH. Platform-swinging in a poly-social-media context: how and why users navigate multiple social media platforms. J Comp-Mediated Commun. 2019;24(1):21–35. doi:10.1093/jcmc/zmy022.
- Madianou M, Miller D. Polymedia: towards a new theory of digital media in interpersonal communication. Int J Cult Stud. 2013;16(2):169–87. doi:10.1177/1367877912452486.
- Wilson T, Starbird K. Cross-platform disinformation campaigns: lessons learned and next steps. Harvard Kennedy Sch Misinf Rev. 2020;1. doi:10.37016/mr-2020-38.
- Douglas KM, Uscinski JE, Sutton RM, Cichocka A, Nefes T, Ang CS, Deravi F. Understanding conspiracy theories. Polit Psychol. 2019;40(S1):3–35. doi:10.1111/pops.12568.
- Van Prooijen J-W, Jostmann NB. Belief in conspiracy theories: the influence of uncertainty and perceived morality. Eur J Soc Psychol. 2013;43(1):109–15. doi:10.1002/ejsp.1922.
- Van Prooijen J-W, Douglas KM. Conspiracy theories as part of history: the role of societal crisis situations. Mem Stud. 2017;10(3):323–33. doi:10.1177/1750698017701615.
- Baden C, Sharon T. BLINDED BY THE LIES? Toward an integrated definition of conspiracy theories. Commun Theory. 2021;31(1):82–106. doi:10.1093/ct/qtaa023.
- Romer D, Jamieson KH. Conspiracy theories as barriers to controlling the spread of COVID-19 in the U.S. Soc Sci Med. 2020;263:113356. doi:10.1016/j.socscimed.2020.113356.
- Jolley D, Douglas KM. The effects of anti-vaccine conspiracy theories on vaccination intentions. PloS One. 2014;9(2):e89177. doi:10.1371/journal.pone.0089177.
- Jolley D, Douglas KM. Prevention is better than cure: addressing anti‐vaccine conspiracy theories. J Appl Soc Psychol. 2017;47(8):459–69. doi:10.1111/jasp.12453.
- van Prooijen J-W, Van Vugt M. Conspiracy theories: evolved functions and psychological mechanisms. Perspect Psychol Sci. 2018;13(6):770–88. doi:10.1177/1745691618774270.
- Gruzd A, Mai P. Going viral: how a single tweet spawned a COVID-19 conspiracy theory on Twitter. Big Data Soc. 2020;7(2):2053951720938405. doi:10.1177/2053951720938405.
- Gerts D, Shelley CD, Parikh N, Pitts T, Watson Ross C, Fairchild G, Vaquera Chavez NY, Daughton AR. “Thought I’d share first” and other conspiracy theory Tweets from the COVID-19 infodemic: exploratory study. JMIR Public Health Surveillance. 2021;7(4):e26527. doi:10.2196/26527.
- Ahmed W, Vidal-Alaball J, Downing J, Seguí FL. COVID-19 and the 5G conspiracy theory: social network analysis of Twitter data. J Med Internet Res. 2020;22(5):e19458. doi:10.2196/19458.
- Ferrara E. # covid-19 on twitter: bots, conspiracies, and social media activism. arXiv Preprint arXiv: 200409531; 2020.
- McCombs M, Shaw D. The agenda setting function of mass media. Public Opin Q. 1972;36(2):176–87. doi:10.1086/267990.
- Scheufele DA, Tewksbury D. Framing, agenda setting, and priming: the evolution of three media effects models. J commun. 2007;57:9–20.
- Ophir Y, Walter D, Arnon D, Lokmanoglu, A, Tizzoni, M, Carota, J, D'Antiga, LO, Nicastro, E. The framing of COVID-19 in Italian media and its relationship with community mobility: a mixed-method approach. J Health Commun. 2021;26(3):161–173. doi:10.1080/10810730.2021.1899344.
- Valenzuela S, Puente S, Flores PM. Comparing disaster news on Twitter and television: an intermedia agenda setting perspective. J Broadcast Electron Media. 2017;61(4):615–37. doi:10.1080/08838151.2017.1344673.
- Rogstad I. Is Twitter just rehashing? Intermedia agenda setting between Twitter and mainstream media. J IT Polit. 2016;13(2):142–58. doi:10.1080/19331681.2016.1160263.
- Cruishank I, Ginossar T, Zheleva E, Berger-Wolf TY. Content and dynamics of websites shared over vaccine-related Tweets in COVID-19 conversations: a computational analysis. J Med Internet Res. 2021;23(11): e29127. doi:10.2196/29127.
- Chen E, Lerman K, Ferrara E. Tracking social media discourse about the covid-19 pandemic: development of a public coronavirus twitter data set. JMIR Public Health Surveillance. 2020;6(2):e19273. doi:10.2196/19273.
- Pezoa F, Reutter JL, Suarez F, Ugarte M, Vrgoč D. Foundations of JSON schema. Proceedings of the 25th International Conference on World Wide Web; 2016; Montreal, Canada. p. 263–273.
- Huang B. Learning user latent attributes on social media [PhD thesis]. Carnegie Mellon University; 2021.
- Blei DM, Ng AY, Jordan MI. Latent Dirichlet allocation. J Mach Learn Res. 2003;3:993–1022.
- Röder M, Both A, Hinneburg A. Exploring the space of topic coherence measures. Proceedings of the eighth ACM international conference on Web search and data mining; 2015; Shanghai, China. p. 399–408.
- Boon-Itt S, Skunkan Y. Public perception of the COVID-19 pandemic on Twitter: sentiment analysis and topic modeling study. JMIR Public Health Surveillance. 2020;6(4):e21978. doi:10.2196/21978.
- Downe‐Wamboldt B. Content analysis: method, applications, and issues. Health Care Women Int. 1992;13(3):313–21. doi:10.1080/07399339209516006.
- Moulding R, Nix-Carnell S, Schnabel A, Nedeljkovic M, Burnside EE, Lentini AF, Mehzabin N. Better the devil you know than a world you don’t? Intolerance of uncertainty and worldview explanations for belief in conspiracy theories. Pers Individ Dif. 2016;98:345–54. doi:10.1016/j.paid.2016.04.060.
- Giglietto F, Righetti N, Rossi L, Marino G. Coordinated link sharing behavior as a signal to surface sources of problematic information on Facebook. International Conference on Social Media and Society; 2020. p. 85–91.
- Pacheco D, Hui P-M, Torres-Lugo C, Truong BT, Flammini A, Menczer F. Uncovering coordinated networks on social media: methods and case studies. arXiv preprint. 2020;arXiv:200105658.
- False claim: Video shows Bill Gates presenting vaccine for religious fundamentalists to Pentagon; 2020. https://www.reuters.com/article/uk-factcheck-gates-fundamentalists-penta/false-claim-video-shows-bill-gates-presenting-vaccine-for-religious-fundamentalists-to-pentagon-idUSKBN22P35M. Accessed3 June 2021.
- Menczer F. 4 Reasons why social media make us vulnerable to manipulation. Fourteenth ACM Conference on Recommender Systems; 2020. p. 1.
- Yang K-C, Hui P-M, Menczer F. Bot electioneering volume: visualizing social bot activity during elections. Companion Proceedings of The 2019 World Wide Web Conference, WWW’19, ACM; 2019; New York, NY, USA. p. 214–217. doi:10.1145/3308560.3316499.
- Jamison AM, Broniatowski DA, Quinn SC. Malicious actors on Twitter: a guide for public health researchers. Am J Public Health. 2019;109(5):688–92. doi:10.2105/AJPH.2019.304969.
- Lahouati M, De Coucy A, Sarlangue J, Cazanave C. Spread of vaccine hesitancy in France: what about YouTube™? Vaccine. 2020;38(36):5779–82. doi:10.1016/j.vaccine.2020.07.002.
- Basch CH, Hillyer GC, Zagnit EA, Basch CE. YouTube coverage of COVID-19 vaccine development: implications for awareness and uptake. Hum Vaccin Immunother. 2020;16(11):2582–85. doi:10.1080/21645515.2020.1790280.
Appendix
Twenty most probable words for the twelve topics of the best LDA model on the YouTube transcripts.