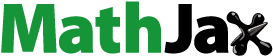
ABSTRACT
Vaccination has become the primary means for citizens to prevent severe morbidity and mortality during the pandemic. However, vaccine hesitancy poses a major threat to global public health security. Based on the data from Chinese General Social Survey in 2021, this study aims to explore the socio-political aspects of COVID-19 vaccine hesitancy, focusing on the relationship between COVID-19 risk perceptions, institutional trust and vaccine hesitancy. Among the samples, 39.8% of the respondents exhibited COVID-19 vaccine hesitancy, 48.9% had a high awareness of the risk of COVID-19, and 74.6% presented a high level of trust in institutions. The results showed that higher risk perception and institutional trust are negatively correlated with vaccine hesitancy (p < .001). Institutional trust had no statistically significant moderating effect on the association between risk perception and vaccine hesitancy, but the role of institutional trust in influencing vaccine hesitancy is more significant at a lower level of perceptions of COVID-19 risk. Furthermore, regional variations in the factors contributing to vaccine hesitancy were demonstrated in China. These findings have important implications for developing strategies to address vaccine hesitancy.
Introduction
Vaccination is widely recognized as a critical measure for preventing pandemic infectious diseases. However, vaccine hesitancy (VH), defined by the WHO as the “delay in acceptance or refusal of vaccines despite availability of vaccine services,”Citation1,Citation2 poses one of the most significant threats to global public health security.Citation3 Previous research has demonstrated that VH is a complex and dynamic issue that is influenced by a range of individual-level factors, as well as contextual, social, cultural and political factors.Citation4,Citation5 Recently, the COVID-19 pandemic has brought even greater attention to this issue across the world.Citation6–10 Similar to the United States, the United Kingdom and some other Western countries, China has witnessed a substantial body of research on COVID-19 VH.Citation11–13 However, the majority of existing research is based on specific populations (such as people with underlying diseases,Citation14 healthcare worker,Citation15 college students,Citation16 parents of minorsCitation17 and individuals from one or several provinces,Citation18 with very few nationally representative samples. Furthermore, few studies have focused on the socio-political aspects of VH, particularly the relationship between risk perception (RP), institutional trust (IT) and VH, which represents significant issues in VH research. There are basically no studies exploring regional differences of VH in China. Therefore, this study aimed to fill these gaps.
Method
Hypotheses
As mentioned above, available evidence indicates that COVID-19 VH may be explained by various factors, including not only medical factors but also socio-political ones. Thus, our main focus is on the relationship between RP, IT and VH.
RP refers to an individual’s estimation of their future likelihood of contracting a particular disease, considering behavioral and temporal factors.Citation19,Citation20 People’s catching and even fear of COVID-19 can have a serious adverse impact on their physical and mental health.Citation21 Thus, people who perceive high risks of catching the COVID-19 may be more likely to have a positive attitude toward vaccination, as vaccines can be effective in reducing fear and preventing severe or even fatal disease.Citation22 So we propose our first hypothesis, H1a: RP is negatively associated with the degree of VH.
Trust has been given a multitude of definitions and dimensions within the socio-medical literature.Citation23 IT means that citizens have trust in institutions such as government, judicial system, medical institutions and scientific institutions to behave in a predictable, fair and transparent way, and to act in a manner that is in the interests of citizens.Citation24 It functions to reduce uncertainty and complexity when dealing with novel crisis such as COVID-19, and increase people’s compliance with government campaigns.Citation25,Citation26 Therefore, people who trust their institutions may have a more positive attitude toward getting the COVID-19 vaccine.Citation27 Conversely, people who distrust institutions may refuse vaccination because they question the safety and efficacy of vaccines.Citation28,Citation29 Thus, we derive the following hypothesis, H1b: IT is negatively associated with the degree of VH.
It is noteworthy that the link between risk perception and VH may not necessarily be straightforward. Some extant studies have shown that despite individuals having some perceptions of risk, they seldom take appropriate preparations. The relationship may be influenced by other intervening factors, an important one of which is trust. When facing an extremely uncertain and complex crisis like the COVID-19, individuals may not be able to handle with all the threats, which make them employ trust in authorities, experts and other external systems to manage personal risk.Citation25 Consequently, we hypothesize that H2: IT positively moderated the relationship between RP and VH.
Additionally, VH represents “a complex behavioral phenomenon specific to vaccines, contexts, time and place.”Citation30 In this sense, we expect that geographical locations and regional factors may exhibit an impact on COVID-19 VH. Some existing studies suggest that COVID-19 acceptance rates and the causal factors of VH differ both between and within countries.Citation6,Citation26 Hence, we propose that H3: RP and IT have different impacts on VH in different regions (such as the east, central and west in China).
Data and samples
The data for this study were collected from the 2021 Chinese General Social Survey (CGSS2021), which is a national, comprehensive and ongoing academic survey project in China. The survey is mainly conducted by Renmin University of China, with focus on adults and families across mainland China. The CGSS has a strong scientific foundation and has been widely used in scientific research, teaching and government decision-making. Due to the outbreak of COVID-19, a special topic on vaccination and VH was incorporated into the survey conducted in 2021. The samples were located in various provinces and cities, including Beijing, Hebei, Liaoning, Jiangsu, Zhejiang, Fujian, Shandong and Guangxi in the east; Shanxi, Inner Mongolia, Anhui, Jiangxi, Henan, Hubei and Hunan in the central region; and Chongqing, Shaanxi, Gansu and Ningxia in western China. These provinces and cities are representative of their respective regions. A total of 8148 respondents were interviewed in CGSS2021. After applying the inclusion criteria – being an adult (age ≥18 years) and living, working, and/or receiving healthcare in China, 2717 samples were finally eligible for analysis. Missing values for the education level variable were imputed using the mean difference imputation method. Other missing values were generally eliminated. Among the samples, 45.1% were males and 54.9% were females. The age of the respondents ranged from 18 to 99 years, with a mean age of 52.0 ± 17.6.
Measurement
COVID-19 VH was assessed using the question “Which of the following statements best describes your situation?” Respondents were provided with four response options: (a) Initially reluctant, but eventually accept vaccination; (b) Initially hesitant, but ultimately decided to get vaccinated; (c) Unwilling to provide an answer; and (d) Initially intended to get vaccinated. To identify VH, responses were dichotomized, with options (a), (b), and (c) coded as positive (1), and option (d) coded as negative (0).
RP of COVID-19 infection was measured by asking respondents, “How worried are you about getting COVID-19?” Respondents could choose from the options “Very worried,” “Some worry,” “Not too worried,” and “Not worried at all.” The degree of RP was graded using a four-point Likert scale,Citation31 with these options coded from 4 to 1 respectively. “Very worried’ indicated the highest level of risk perception, while “Not worried at all” indicated lowest level of risk perception. The scale of 1–4 represented an increasing level of risk perception. Further, the data was analyzed using the method of entropy weight calculation of composite scores. Scores ranging from 1 to 2 were categorized as low risk perception (1), while scores ranging from 3–4 were categorized as high risk perception (2).
IT was measured across three dimensions: the perception of social trust, confidence in the health care system, and confidence in the government. For ease of measurement and explanation, each dimension was evaluated using a five-point Likert scale.Citation32 Social trust was assessed by the question, “In general, would you agree that most people in society can be trusted?” Respondents were allowed to specify their levels of agreement on a five-point Likert scale, namely “Strongly disagree”(1), “Disagree”(2), “Neither agree nor disagree” or “Don’t know” or “Refusing to answer”(3), “Agree”(4) and “Strongly agree”(5). Higher scores indicated greater trust. Trust in the healthcare system was measured by asking, “How have the measures the healthcare system in our country has taken to address the COVID-19 pandemic changed your confidence?” Trust in the government was measured by asking, “How have the measures the government has taken to deal with the COVID-19 pandemic changed your confidence?” For both questions, respondents could choose from the following options: “Increased significantly,” “Increased slightly,” “Remain basically unchanged,” “Unable to choose,” “Refused to answer,” “Decreased slightly,” and “decreased significantly,” which were coded as 5, 4, 3, 3, 3, 2, and 1, respectively. Higher scores indicated higher levels of trust. Following Cheng, et al.Citation33 the weight was calculated using the entropy method, which enabled the computation of the composite index for IT.
VH may exhibit regional differences.Citation34 To test whether the influencing factors of VH are different across China, provinces are divided into the eastern, the central, and the western region.Citation35
The study also incorporated several control variables including sex, age-group, ethnicity, income, education and residency registration. Sex was categorized as nominal, with men assigned as 1 and female as 0. Respondents were categorized into two groups according to their age: age-group was given a value of 1 if a respondent was 70 years old or above and a value of 0 otherwise.Citation36 Ethnicity was classified as Han, Mongolian, Manchu, Hui, Tibetan, Zhuang, Uygur, and others. The Han ethnicity was assigned a value of 1, while other ethnicities were assigned a value of 0. Income is divided into five ranking categories (in yuan): less than 4000, 4000 to less than 10,000, 10000 to less than 20,000, 20000 to less than 50,000, and 50,000 or more. Education was categorized as primary school and below, junior high school, high school, and college degree or above. Residency registration is classified into agricultural and non-agricultural registrations, with the former recorded as 1, while the latter recorded as 2.
Statistical analysis
SPSS20.0 was used for data processing and analysis. In a first step, hierarchical regression analysis was employed to examine the association between RP, IT, and VH. In a second step, the moderating effect of IT on the correlation between RP and VH was analyzed by incorporating an interaction term. In a third step, the whole samples were divided into two sub-groups (high RP and low RP), and group regression analysis was carried out to explore the effects of IT on VH. Finally, the entire samples were divided into three groups based on the eastern, central and western regions of China, and comparisons were made to reveal the regional differences of the factors associated with VH. What we conducted are two-sided statistical tests. A two-sided p-value of less than .1 indicates a statistically significant difference.
Results
Descriptive statistics
presents descriptive statistics for the sample. 60.2% of the respondents reported that they were likely or extremely likely to receive the COVID-19 vaccine if it were accessible to them; however, 39.8% reported that they were unlikely or very unlikely to do so. 48.9% of the respondents had a high perception of risk, while 51.1% had a low perception of risk. 74.6% of the respondents expressed a high level of trust in institutions, whereas 25.4% remained distrustful ().
Figure 1. Proportions of vaccine hesitancy, risk perception and institutional trust. VH: vaccine hesitancy; RP: risk perception; IT: institutional trust. Abscissa: Categories;Ordinate: proportion.

Table 1. Descriptive statistics.
Hierarchical regression analysis
The results of hierarchical regression analysis were presented in , revealing the associations between RP, IT, other individual-level factors and VH. The results of Model 1 showed that age-group (p = .000), ethnicity (p = .014), and residency registration (p = .000) exhibited a significantly positive correlation with VH, while education (p < .1) had a negative correlation with VH. Furthermore, the coefficients of sex and income were not found to be statistically significant.
Table 2. Hierarchical regression analysis (dependent variable: VH).
Based on Model 1, Model 2 added RP, which resulted in significant changes in F (p = .008), indicating that the inclusion of RP had explanatory significance for the model. The coefficient of RP was −0.023, which was statistically negatively significant (p = .000), indicating that RP had a significantly negative effect on VH, after controlling for such factors as age-group, sex, ethnicity, income, education, and residency registration.
Furthermore, based on Model 2, Model 3 included IT and demonstrated significant changes in F (p = .000), suggesting that the incorporation of IT has explanatory value for the model. Specifically, the coefficient of IT was −0.060, which was statistically negatively significant (p = .002), indicating that IT had a significantly negative effect on VH, after controlling for RP as well as other individual-level variables.
Moderation analysis
In order to further explore the moderation effects of IT on the relationship between RP and VH, we incorporated into Model 3 an interaction term of RP and IT. Thus, we constructed a new regression model as follows:
In this model, CV represents other control variables, while the coefficient β3 is the primary focus of our attention. Specifically, β3 indicates the strength and direction of the moderating effects of IT on the relationship between RP and VH. The regression results were given in , which indicated that after controlling for other variables, IT had no statistically significant moderating effect on the association between RP and VH ( p = .218).
Table 3. Moderating analysis.
Grouped regression analysis
To gain a more nuanced understanding of how IT and RP jointly affect VH, we further divided the whole samples into two separate groups: high RP and low RP. The results of grouped regression analysis were reported in , which shows that when people’s perceived risk of COVID-19 infection was high, IT was statistically significantly associated with VH (β = −0.047, p = .018). Likewise, when RP is low, IT was also substantially related to VH (β = −0.074, p = .000); however, the coefficient of IT has considerably increased, and the statistical significance level has also notably increased, indicating that the role of IT in influencing VH is more evident at a lower level of perceptions of COVID-19 risk.
Table 4. Group regression analysis.
Regional differences analysis
To explore the regional differences of associated factors of VH, we analyzed the data based on the eastern, central and western regions in China, the results of which were given in . Regarding RP, the coefficients in the eastern (p = .309) and central (p = .193) regions were negative but not statistically significant, while the coefficient in the western region was statistically significantly negative (β = −0.033, p = .045). Regarding IT, the coefficients were statistically significantly negative across all the three regions; however, the values of the coefficients increased from eastern (β = −0.089) to central (β = −0.055) and to western (β = −0.044) regions, whereas the significance level sequentially decreased from eastern (p = .000) to central (p = .018) and to western (p = .081) regions.
Table 5. Hierarchical regression analysis by region.
Discussions
Vaccination is not solely a medical issue, but also a socio-political one. COVID-19 VH has been widely documented in the literature.Citation37–39 Despite extensive literature on the reasons for COVID-19 VH, relatively limited attention has been focused on its socio-political aspects, particularly the role of IT in the context of VH in China. In this study, we fill this gap by analyzing how the interaction of COVID-19 RP and trust in institutions influenced VH, based on a nationally representative survey dataset (the CGSS2021) in China.
First, we found that both higher RP and higher IT are negatively correlated with COVID-19 VH. The results generally align with previous research findings.Citation40–42 In general, individuals with higher RP should exhibit high protective behaviors and/or low risk behaviors.Citation20 Nusair et al. found that persons at higher risk for infection were expected to be more willing to take preventive measures and to be more likely to be vaccinated than their peers.Citation43 Truong et al. found that RP and trust in the institutional and health system were closely linked to the extent of vaccine immunization.Citation41 Du et al. found that public trust in the vaccine system and the government can enhance vaccine confidence and reduce vaccine hesitancy.Citation44 Overall, the findings indicate that to promote willingness to receive vaccination, effective measures should be taken to increase the public’s risk awareness regarding infectious diseases and to establish and maintain public trust.
Second, we found that IT had no statistically significant moderating effect on the association between RP and VH, but that the role of IT in influencing VH is more evident and significant at a lower level of perceptions of COVID-19 risk. This is a more interesting and nuanced aspect that has been understudied in previous research. When people’s RP is very high, they may pay more attention to and worry about the risk of COVID-19 itself, rather than the relevant factors of vaccine and vaccine administration. In this case, even if IT increases, it may not significantly change people’s attitudes toward vaccination because people’s concerns and worries about the risk of COVID-19 exceed the relevant factors of vaccine and vaccine administration. However, in low-risk situations, people may pay more attention to and consider the relevant factors of vaccine administration (such as safety, effectiveness, etc.); at this time, the increase of IT may more easily influence people’s views and attitudes toward vaccine administration. Additionally, people with low RP do not initiate vaccination but may cooperate to get vaccinated based on trust in the government, healthcare institutions and society.Citation45 This implies that continuous attention and adaptation to changes in public RP and trust levels are necessary to ensure sustained vaccination efforts.
Third, the study has demonstrated regional variations in the factors contributing to VH, confirming that VH is a complex phenomenon with significant geographical and contextual associations.Citation6,Citation26,Citation30 More specifically, no significant correlations were observed between RP and VH, yet notable negative correlations were evident between IT and VH in the eastern and central regions. This may be attributed to the fact that, although the epidemic situation in the eastern and central regions is potentially more severe where the population density is higher compared to the western region, medical resources and healthcare quality are even advanced, leading to a relatively less severe concern regarding the risk of COVID-19. Additionally, individuals in the eastern and central regions tend to have a greater emphasis on the safety and efficacy of vaccines, coupled with a higher level of trust in the institutions.Citation46 On the contrary, in the western regions, there is a significant negative correlation between RP and IT with VH. This may be because, despite the relatively mild epidemic situation in the western regions, medical resources and healthcare quality are even worse, leading to a higher focus on the risk of COVID-19. In addition, IT levels in the western regions may be relatively lower compared to the eastern and central regions, resulting in a less significant impact of IT on VH.Citation47 Thus, further research is needed to explore these influencing factors.
Limitations and implications
This study has a limitation in that the data collected were cross-sectional, which prevents us from determining the causal relationship between associated factors and COVID-19 VH over different time periods. The limitation was partially mitigated by the extensive and diverse sample, which closely mirrored the distribution of VH across the country. Future studies should aim to investigate the influencing factors with more rigorous designs, such as using time-series data or experimental methods. Another limitation is that it did not incorporate such factors as personal health status, interpersonal network, healthcare resources, and social media exposureCitation48 into the analysis. Follow-up analysis incorporating these factors will further enrich our understanding of the issue. Additionally, although all the respondents are adults, some of them are parents or caregivers of children, thus our findings remain relevant to children’s VH.
Furthermore, it is worthy to note that 39.8% of our samples were hesitant about getting vaccinated in 2021, but it was reported that the vaccination rate in China reached 97.6% by 2023.Citation49 To some extent, this phenomenon is similar to the situation in India, where the government’s openness and transparency led to a gradual decline in VH.Citation50 Thus, future research is expected to explore how China managed to achieve such a high vaccination rate, examine the role of RP and IT play in this process, and compare the differences in working mechanisms between China and India, two of the largest developing countries in the world.
With these limitations and future directions in mind, our results provide valuable implications for medical policymakers to recognize that vaccination is not merely a medical issue, but also a socio-political matter. To enhance vaccine coverage, corresponding measures aimed at bolstering the public’s trust in institutions should be implemented, tailored to the specific circumstances of different regions. In this regard, our research also carries practical implications for addressing other public health concerns.
Author contributions
All authors contributed to the study conception and design. Conceptualization: [Guiwu Chen]. Methodology: [Guiwu Chen] [Yiyun Yao]. Assessment: [YaoWen Zhang]. Formal analysis and investigation: [Yiyun Yao]. Writing-original draft preparation: [Fei Zhao]. Writing-review and editing: [Fei Zhao], [Guiwu Chen]. Funding acquisition: [Guiwu Chen]. Supervision: [Fei Zhao].
Disclosure statement
No potential conflict of interest was reported by the author(s).
Additional information
Funding
References
- Lane S, MacDonald NE, Marti M, Dumolard L. Vaccine hesitancy around the globe: analysis of three years of WHO/UNICEF joint reporting form data-2015-2017. Vaccine. 2018;36(26):3861–7. doi:10.1016/j.vaccine.2018.03.063.
- Larson HJ, Jarrett C, Eckersberger E, Smith DM, Paterson P. Understanding vaccine hesitancy around vaccines and vaccination from a global perspective: a systematic review of published literature, 2007–2012. Vaccine. 2014;32(19):2150–9. doi:10.1016/j.vaccine.2014.01.081.
- Löffler P. Review: vaccine myth-buster - cleaning up with prejudices and dangerous misinformation. Front Immunol. 2021;12:663280. doi:10.3389/fimmu.2021.663280.
- Kumar D, Chandra R, Mathur M, Samdariya S, Kapoor N. Vaccine hesitancy: understanding better to address better. Isr J Health Policy Res. 2016;5(1):2. doi:10.1186/s13584-016-0062-y.
- Quinn SC, Jamison A, An J, Freimuth VS, Hancock GR, Musa D. Breaking down the monolith: understanding flu vaccine uptake among African Americans. SSM Popul Health. 2018;4:25–36. doi:10.1016/j.ssmph.2017.11.003.
- Yasmin F, Najeeb H, Moeed A, Naeem U, Asghar MS, Chughtai NU, Yousaf Z, Seboka BT, Ullah I, Lin CY. et al. COVID-19 vaccine hesitancy in the United States: a systematic review. Front Public Health. 2021;9:770985. doi:10.3389/fpubh.2021.770985.
- Joshi A, Kaur M, Kaur R, Grover A, Nash D, El-Mohandes A. Predictors of COVID-19 vaccine acceptance, intention, and hesitancy: a scoping review. Front Public Health. 2021;9:698111. doi:10.3389/fpubh.2021.698111.
- Hudson A, Montelpare WJ. Predictors of vaccine hesitancy: implications for COVID-19 public health messaging. Int J Environ Res Public Health. 2021;18:15. doi:10.3390/ijerph18158054.
- Andrade G. Predictive demographic factors of covid-19 vaccine hesitancy in Venezuela: a cross-sectional study. Vacunas. 2022;23:S22–s25. doi:10.1016/j.vacun.2021.07.009.
- Samo AA, Sayed RB, Valecha J, Baig NM, Laghari ZA. Demographic factors associated with acceptance, hesitancy, and refusal of COVID-19 vaccine among residents of Sukkur during lockdown: a cross sectional study from Pakistan. Hum Vaccin Immunother. 2022;18(1):2026137. doi:10.1080/21645515.2022.2026137.
- Wang J, Ji Q, Dong S, Zhao S, Li X, Zhu Q, Long S, Zhang J, Jin H. Factors influencing vaccine hesitancy in China: a qualitative study. Vaccines (Basel). 2021;9(11):1291. doi:10.3390/vaccines9111291.
- Zhang H, Zheng P, Zhang J, Qiu Q, Huang B, Feng H, Zhang Y, Chen X, Xu J, Dong X. Vaccine hesitancy among parents and its influencing factors: a cross-sectional study in Guangzhou, China. Hum Vaccin Immunother. 2021;17(12):5153–5161. doi:10.1080/21645515.2021.1984131.
- Zou H, Huang Y, Chen T, Zhang L. Influenza vaccine hesitancy and influencing factors among university students in China: a multicenter cross-sectional survey. Ann Med. 2023;55(1):2195206. doi:10.1080/07853890.2023.2195206.
- Zhou Y, Lin Z, Wan X, Liu J, Ding J, Zhang C, Ren K, Li D, Wu Y. COVID-19 vaccine acceptance and hesitancy in patients with Parkinson’s disease. Front Public Health. 2022;10:977940. doi:10.3389/fpubh.2022.977940.
- Xu B, Zhang Y, Chen L, Yu L, Li L, Wang Q. The influence of social network on COVID-19 vaccine hesitancy among healthcare workers: a cross-sectional survey in Chongqing, China. Hum Vaccin Immunother. 2021;17(12):5048–5062. doi:10.1080/21645515.2021.2004837.
- Chen Y, Zhang MX, Lin XQ, Wu H, Tung TH, Zhu JS. COVID-19 vaccine hesitancy between teachers and students in a college, a cross-sectional study in China. Hum Vaccin Immunother. 2022;18(5):2082171. doi:10.1080/21645515.2022.2082171.
- Chen H, Li X, Gao J, Liu X, Mao Y, Wang R, Zheng P, Xiao Q, Jia Y, Fu H. et al. Health belief model perspective on the control of COVID-19 vaccine hesitancy and the promotion of vaccination in China: web-based cross-sectional study. J Med Internet Res. 2021;23(9):e29329. doi:10.2196/29329.
- Zhuang W, Zhang J, Wei P, Lan Z, Chen R, Zeng C, Shi Q, Qiao G. Misconception contributed to COVID-19 vaccine hesitancy in patients with lung cancer or ground-glass opacity: a cross-sectional study of 324 Chinese patients. Hum Vaccin Immunother. 2021;17(12):5016–5023. doi:10.1080/21645515.2021.1992212.
- Brewer NT, Weinstein ND, Cuite CL, Herrington JE. Risk perceptions and their relation to risk behavior. Ann Behav Med. 2004;27(2):125–30. doi:10.1207/s15324796abm2702_7.
- Johnson BB, Kim B. Cross-temporal relations of conditional risk perception measures with protective actions against COVID-19. Social Sci Med. 2023;324:115867. doi:10.1016/j.socscimed.2023.115867.
- Giuntella O, Hyde K, Saccardo S, Sadoff S. Lifestyle and mental health disruptions during COVID-19. Proc Natl Acad Sci USA. 2021;118(9). doi:10.1073/pnas.2016632118.
- Bendau A, Plag J, Petzold MB, Ströhle A. COVID-19 vaccine hesitancy and related fears and anxiety. Int Immunopharmacol. 2021;97:107724. doi:10.1016/j.intimp.2021.107724.
- Larson HJ, Clarke RM, Jarrett C, Eckersberger E, Levine Z, Schulz WS, Paterson P. Measuring trust in vaccination: a systematic review. Hum Vaccin Immunother. 2018;14(7):1599–1609. doi:10.1080/21645515.2018.1459252.
- Siegrist M, Cvetkovich G, Roth C. Salient value similarity, social trust, and risk/benefit perception. Risk Anal. 2000;20(3):353–62. doi:10.1111/0272-4332.203034.
- Wachinger G, Renn O, Begg C, Kuhlicke C. The risk perception paradox–implications for governance and communication of natural hazards. Risk Anal. 2013;33(6):1049–65. doi:10.1111/j.1539-6924.2012.01942.x.
- Seddig D, Maskileyson D, Davidov E, Ajzen I, Schmidt P. Correlates of COVID-19 vaccination intentions: attitudes, institutional trust, fear, conspiracy beliefs, and vaccine skepticism. Social Sci Med. 2022;302:114981. doi:10.1016/j.socscimed.2022.114981.
- Allington D, McAndrew S, Moxham-Hall V, Duffy B. Coronavirus conspiracy suspicions, general vaccine attitudes, trust and coronavirus information source as predictors of vaccine hesitancy among UK residents during the COVID-19 pandemic. Psychol Med. 2023;53(1):236–247. doi:10.1017/S0033291721001434.
- Jennings W, Stoker G, Bunting H, Valgarðsson VO, Gaskell J, Devine D, McKay L, Mills MC. Lack of trust, conspiracy beliefs, and social media use predict COVID-19 vaccine hesitancy. Vaccines (Basel). 2021;9(6):593. doi:10.3390/vaccines9060593.
- Kang K, Xu X, Dong R, Tian G. The risk perception of COVID-19 and pandemic-related behaviors: a moderated mediation model of political trust and self-efficacy. Psychol Health Med. 2023;2023:1–14. doi:10.1080/13548506.2023.2197648.
- Bussink-Voorend D, Hautvast JLA, Vandeberg L, Visser O, Hulscher M. A systematic literature review to clarify the concept of vaccine hesitancy. Nat Hum Behav. 2022;6(12):1634–1648. doi:10.1038/s41562-022-01431-6.
- Sudharsanan N, Favaretti C, Hachaturyan V, Bärnighausen T, Vandormael A. The effect of framing and communicating COVID-19 vaccine side-effect risks on vaccine intentions for adults in the UK and the USA: a structured summary of a study protocol for a randomized controlled trial. Trials. 2021;22(1):592. doi:10.1186/s13063-021-05484-2.
- Gentile A, Pacchiotti AC, Giglio N, Nolte MF, Talamona N, Rogers V, Berenstein A, Castellano VE. Vaccine hesitancy in Argentina: validation of WHO scale for parents. Vaccine. 2021;39(33):4611–4619. doi:10.1016/j.vaccine.2021.06.080.
- Cheng L, Ma W, Xia X, Wang L. Quality-achieving reliability assessment of rolling bearing based on bootstrap maximum entropy method and similarity method. Sci Prog. 2022;105(2):368504221102737. doi:10.1177/00368504221102737.
- Durmuş Sarıkahya S, Güden E, Sümeyye Yorulmaz D. Childhood vaccine hesitancy in two regions with different socioeconomic backgrounds in Turkey. Z Gesundh Wiss. 2023:1–0. doi:10.1007/s10389-023-01854-z.
- Zhang X, Lin Z. Evaluation of water resource utilization efficiency in provincial areas of China based on the unexpected output SBM Model. J Environ Public Health. 2022;2022:9554730. doi:10.1155/2022/9554730.
- Bastard P, Gervais A, Le Voyer T, Rosain J, Philippot Q, Manry J, Michailidis E, Hoffmann H-H, Eto S, Garcia-Prat M, et al. Autoantibodies neutralizing type I IFNs are present in ~4% of uninfected individuals over 70 years old and account for ~20% of COVID-19 deaths. Sci Immunol. 2021;6:62. doi:10.1126/sciimmunol.abl4340.
- Burki T. The online anti-vaccine movement in the age of COVID-19. Lancet Digit Health. 2020;2(10):504–5. doi:10.1016/S2589-7500(20)30227-2.
- Taylor S, Landry CA, Paluszek MM, Groenewoud R, Rachor GS, Asmundson GJG. A proactive approach for managing COVID-19: the importance of understanding the motivational roots of vaccination hesitancy for SARS-CoV2. Front Psychol. 2020;11:575950. doi:10.3389/fpsyg.2020.575950.
- Lazarus JV, Ratzan SC, Palayew A, Gostin LO, Larson HJ, Rabin K, Kimball S, El-Mohandes A. A global survey of potential acceptance of a COVID-19 vaccine. Nat Med. 2021;27(2):225–228. doi:10.1038/s41591-020-1124-9.
- Cipolletta S, Andreghetti GR, Mioni G. Risk perception towards COVID-19: a systematic review and qualitative synthesis. Int J Environ Res Public Health. 2022;19(8):4649. doi:10.3390/ijerph19084649.
- Truong J, Bakshi S, Wasim A, Ahmad M, Majid U. What factors promote vaccine hesitancy or acceptance during pandemics? A systematic review and thematic analysis. Health Promot Int. 2022;37(1). doi:10.1093/heapro/daab105.
- Schernhammer E, Weitzer J, Laubichler MD, Birmann BM, Bertau M, Zenk L, Caniglia G, Jäger CC, Steiner G. Correlates of COVID-19 vaccine hesitancy in Austria: trust and the government. J Public Health (Oxf). 2022;44(1):106–16. doi:10.1093/pubmed/fdab122.
- Nusair MB, Arabyat R, Khasawneh R, Al-Azzam S, Nusir AT, Alhayek MY. Assessment of the relationship between COVID-19 risk perception and vaccine acceptance: a cross-sectional study in Jordan. Hum Vaccin Immunother. 2022;18(1):2017734. doi:10.1080/21645515.2021.2017734.
- Du F, Chantler T, Francis MR, Sun FY, Zhang X, Han K, Rodewald L, Yu H, Tu S, Larson H, et al. The determinants of vaccine hesitancy in China: a cross-sectional study following the Changchun Changsheng vaccine incident. Vaccine. 2020;38(47):7464–7471. doi:10.1016/j.vaccine.2020.09.075.
- Burger J. Trust and consequences: role of community science, perceptions, values, and environmental justice in risk communication. Risk Anal. 2022;42(11):2362–2375. doi:10.1111/risa.14020.
- Ennab F, Qasba RK, Uday U, Priya P, Qamar K, Nawaz FA, Islam Z, Zary N. COVID-19 vaccine hesitancy: a narrative review of four South Asian countries. Front Public Health. 2022;10:997884. doi:10.3389/fpubh.2022.997884.
- Jin H, Wang H, Li X, Zheng W, Ye S, Zhang S, Zhou J, Pennington M. Economic burden of COVID-19, China, January–March, 2020: a cost-of-illness study. Bull World Health Organ. 2021;99(2):112–24. doi:10.2471/BLT.20.267112.
- Mahajan R, Gupta P, Kushawaha PS. Role of digital misinformation in analysing COVID-19 vaccine hesitancy. Int J Manag Pract. 2023;16(3):284–318. doi:10.1504/IJMP.2023.130353.
- Zhang M, Wang Y, Zhang T, Zhou J, Deng Y, Wang L, Du Y. Status of and perspectives on COVID-19 vaccination after lifting of the dynamic zero-COVID policy in China. Glob Health Med. 2023;5(2):112–17. doi:10.35772/ghm.2022.01063.
- Fang E, Liu X, Li M, Zhang Z, Song L, Zhu B, Wu X, Liu J, Zhao D, Li Y. Advances in COVID-19 mRNA vaccine development. Signal Transduct Target Ther. 2022;7(1):94. doi:10.1038/s41392-022-00950-y.