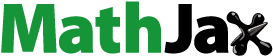
Abstract
Electroencephalogram (EEG) is the recording of electrical activity along the scalp. EEG measures voltage fluctuations generating from ionic current flows within the neurons of the brain. EEG signal is looked as one of the most important factors that will be focused in the next 20 years. In this paper, EEG signal discrimination based on non-linear bistable dynamical model was proposed. EEG signals were processed by non-linear bistable dynamical model, and features of EEG signals were characterized by coherence index. Experimental results showed that the proposed method could properly extract the features of different EEG signals.
Introduction
EEG is the recording of electrical activity of human scalp. EEG measures voltage fluctuations resulting from ionic current flows generated by different neurons of the human brain. In clinical contexts, EEG refers to the recording of the brain's spontaneous electrical activity over a short period of time, usually from 20 to 40 minutes, as recorded from multiple electrodes placed on human scalp. Diagnostic applications generally focus on the spectral content of EEG, that is, the type of neural oscillations that can be observed in EEG signals. EEG is most often used to diagnose epilepsy, which causes abnormalities in EEG readings. It is also used to diagnose sleep disorders, coma, encephalopathies, and brain death. EEG used to be a first-line method of some diseases, such as brain diseases,Citation1-3 Alzheimer diseases,Citation4-6 and in healthy children attention modulation.Citation7,8 Non-linear bistable dynamical model has been focused in biological signal analysis, healthcare, etc.
In this paper, EEG signal analysis based on non-linear bistable dynamical model was proposed. EEG signals were processed by non-linear bistable dynamical model, and features of EEG signals were characterized by coherence index. Results demonstrated that the proposed method in this study successfully extracted the features of different EEG signals.
Results and Discussion
EEG signal
EEG signal data was shown in . The signals were recorded and processed by non-linear bistable dynamical model.
Non-linear bistable dynamics model analysis
EEG data was combined with a 5 KHz sine wave and input into non-linear bistable dynamical model. The result was displayed in . Coherence index was calculated as the function of noise intensity.
The original EEG signal was recorded from the volunteers. Non-linear bistable dynamical model processing results indicated that signal1 was quite different from the other 2 signals because the experiment process of signal1 had much difference from other 2 signals. Signal2 and signal3 data shared the similar eigen peak position under the same outer stimulation noise. Experimental process of signal2 and signal3 were nearly the same, so they have the same outer stimulation. From the results, we could conclude that the non-linear bistable dynamical model could obtain characteristics from EEG signal. Because EEG signal was very complex, researchers had to spend much effort to remove the artifacts, and so on. The non-linear bistable dynamical model could obtain the characteristics without doing additional work, which was promising in human brain science research.
Methods
EEG data
Three EEG experiments data were recorded by some volunteers. The data was described as: cued motor imagery with online feedback with 4 classes from 5 subjects. EEG-data from 3 subjects during a BCI experiments. The experiment consists of 4 sessions for each subject. Each session consists of 5 runs. The EEG was sampled with 120Hz and filtered between 0.25 and 32Hz.Citation9,10
Non-linear bistable dynamics model
Non-linear bistable dynamics model has been tremendously paid attention in fields.Citation11-15 Non-linear bistable dynamics model possesses 3 basic elements: a bistabl model, a weak coherent input, and outer stimulation:(1)
(1)
is a outer stimulation.
is the input signal amplitude and
is the modulation frequency, and
is stimualtion intensity. The most common quantifiers for non-linear bistable dynamics model are the spectral amplification
and the output coherence index. Here we adopt coherence index method, which leads the following definition:
(2)
(2)
is the signal power spectral density, and
is outer stimulation in signal frequency range.
Conclusions
In this paper, EEG signal analysis based on non-linear bistable dynamics model was proposed. EEG signals were processed by non-linear bistable dynamical model, and features of EEG signals were characterized by coherence index. Research results showed that the proposed method could extract the features of different EEG signals. This method is promising in EEG signal non-linear analysis.
Disclosure of Potential Conflicts of Interest
No potential conflicts of interest were disclosed.
Funding
This work is financially supported by National Natural Science Foundation (Grant No. 81000645), and Key Laboratory of Forestry Intelligent Monitoring and Information Technology of Zhejiang Province.
References
- Monastra VJ, Monastra DM, George S. The effects of stimulant therapy, EEG biofeedback, and parenting style on the primary symptoms of attention-deficit/hyperactivity disorder. Appl Psychophysiol Biofeedback 2002; 27:231-49; PMID:12557451; http://dx.doi.org/10.1023/A:1021018700609
- Strehl U, Leins U, Danzer N, Hinterberger T, Schlottke PF. EEG feedback for children with attention-deficit/hyperactivity disorder (ADHD). Preliminary results from a randomized, controlled study. Kindheit und Entwicklung 2004; 13:180-9; http://dx.doi.org/10.1026/0942-5403.13.3.180
- Holtmann M, Stadler C. Electroencephalographic biofeedback for the treatment of attention-deficit hyperactivity disorder in childhood and adolescence. Expert Rev Neurother 2006; 6:533-40; PMID:16623652; http://dx.doi.org/10.1586/14737175.6.4.533
- Zhao Z, Wang Y. A new method for processing end effect in empirical mode decomposition. Commun Circ Syst 2007; ICCCAS 2007; 841-5
- Babiloni C, Frisoni GB, Pievani M, Vecchio F, Lizio R, Buttiglione M, Geroldi C, Fracassi C, Eusebi F, Ferri R, et al. Hippocampan volume and cortical sources of EEG alpha rhythms in mild cognitive impairment and Alzheimer disease. Neuroimage 2009; 44:123-35; PMID:18805495; http://dx.doi.org/10.1016/j.neuroimage.2008.08.005
- Babiloni C, Ferri R, Binetti G, Vecchio F, Frisoni BG, Lanuzza B, Miniussi C, Nobili F, Rodriguez G, Rundo Fet al. Directionality of EEG synchronization in Alzheimer's disease subjects. Neurobiol Aging 2009; 30: 93-102; PMID:17573161; http://dx.doi.org/10.1016/j.neurobiolaging.2007.05.007
- Thomas BJ, Sara L, Peter F, Evangelos B. Using EEG spectral components to assess algorithms for detecting fatigue. Expert Syst Appl 2009; 36:2352-9; http://dx.doi.org/10.1016/j.eswa.2007.12.043
- Lutsyuk NV, Eismont EV, Pavlenko VB. Modulation of attention in healthy children using a course of EEG-feedback sessions. Neirofiziol Neurophysiol 2006; 38:458-65
- Velasco-Álvarez F, Ron-Angevin R, Blanca-Mena MJ. Free virtual navigation using motor imagery through an asynchronous brain-computer interface. Teleoperators Virtual Environ Presence 2010; 19:71-81; http://dx.doi.org/10.1162/pres.19.1.71
- Vidaurre C, Schlogl A, Cabeza R, Pfurscheller G. A fully on-line adaptive brain computer interface. Biomedizinische Technik 2004; 49:760-1
- Chapean-Blondeau F. Non-linear bistable dynamics model at phase noise in signal transmission. Phys Rev E 2000; 61:940-3; http://dx.doi.org/10.1103/PhysRevE.61.940
- Harmer GP, Davis BR, Abbott D. A review of non-linear bistable dynamics model: circuits and measurement. IEEE Trans Instrument Measur 2002; 51:299-309; http://dx.doi.org/10.1109/19.997828
- Jung P, Hanggi P. Amplification of small signals via non-linear bistable dynamics model. Phys Rev A 1991; 44:8032-42; PMID:9905956; http://dx.doi.org/10.1103/PhysRevA.44.8032
- Benzi R, Sutera A, Vulpiana A. The mechanism of non-linear bistable dynamics model. J Phys A 1981; 14:L4536; http://dx.doi.org/10.1088/0305-4470/14/11/006
- Wenning G, Obermayer K. Activity driven adaptive stochastic resonance. Phys Rev Lett 2003; 90:1-4; http://dx.doi.org/10.1103/PhysRevLett.90.120602