ABSTRACT
Cardiac fibrosis (CF) and heart failure (HF) are common heart diseases, and severe CF can lead to HF. In this study, we tried to find their common potential molecular markers, which may help the diagnosis and treatment of CF and HF. RNA library construction and high-throughput sequencing were performed. The DESeq2 package in R was used to screen differentially expressed mRNAs (DEmRNAs), differentially expressed lncRNA (DElncRNAs) and differentially expressed miRNA (DEmiRNAs) between different samples. The common DEmRNAs, DElncRNAs and DEmiRNAs for the two diseases were obtained. The ConsensusPathDB (CPDB) was used to perform biological function enrichment for common DEmRNAs. Gene interaction network was constructed to screen out key genes. Subsequently, real-time polymerase chain reaction (RT-PCR) verification was performed. Lastly, GSE104150 and GSE21125 data sets were utilized for expression validation and diagnostic analysis. There were 1477 DEmRNAs, 502 DElncRNAs and 36 DEmiRNAs between CF and healthy control group. There were 607 DEmRNAs, 379DElncRNAs,s and 42 DEmiRNAs between HF and healthy control group. CH and FH shared 146 DEmRNAs, 80 DElncRNAs, and 6 DEmiRNAs. Hsa-miR-144-3p, CCNE2, C9orf72, MAP3K20-AS1, LEF1-AS1, AC243772.2, FLJ46284, and AC239798.2 were key molecules in lncRNA-miRNA-mRNA network. In addition, hsa-miR-144-3p and CCNE2 may be considered as potential diagnostic gene biomarkers in HF. In this study, the identification of common biomarkers of CF and HF may help prevent CF to HF transition as early as possible.
Graphical abstract
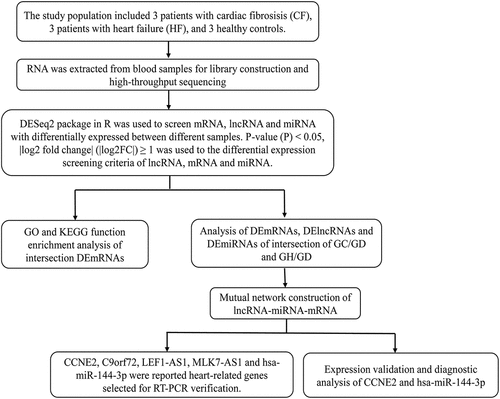
Introduction
Heart failure (HF), caused by overload and damage to the heart, has a high incidence of morbidity and mortality [Citation1]. Cardiac fibrosis (CF) is often caused by changes in the extracellular matrix (ECM) of the heart, which can change the myocardial structure, promote the development of cardiac dysfunction, induce arrhythmias, and also affect the clinical course and outcome of patients with HF [Citation2,Citation3]. Cardiac fibroblasts are basic cell type that composes the heart and are responsible for ECM homeostasis [Citation4]. CF is defined as a key component of HF and has a strong connection with the progression of HF [Citation5,Citation6].
The interleukin 33 (IL-33) knockout mice showed increased left ventricular hypertrophy, ventricular dilatation, and fibrosis compared with wild-type mice [Citation7]. The miRNAs act as negative regulators of protein translation by affecting mRNA stability [Citation8]. Hsa-miR-223 and hsa-miR-144-3P regulate fibrosis after myocardial infarction by targeting RAS p21 protein activator 1 (RASA1) and phosphatase and tensin homolog (PTEN), respectively [Citation9,Citation10]. Previous studies have shown that low hsa-miR-221 and hsa-miR-222 expression are associated with higher fibrosis and left ventricular hardness in patients with HF [Citation11]. The hsa-miR-197-5p has been shown to be associated with fibrosis in cardiac magnetic resonance imaging of patients with stage C or stage D HF [Citation12]. Interestingly, lncRNA has been found to be an important regulator of heart disease, for example, knockdown of lncRNA-Safe and over-expression of lncRNA-Crnd can alleviate CF [Citation13,Citation14]. These studies highlight that differential expression of mRNAs, miRNAs, and lncRNAs plays an important regulatory role in CF and CH.
Nowadays, gene therapy has become an attractive strategy for the treatment of HF, but there are also many challenges [Citation15]. Histologically, the severity of CF might have been connected with a higher mortality in patients with cardiac diseases, particularly those with HF [Citation16,Citation17]. The detection, prevention, and regression of CF have become important targets for improving HF treatment [Citation2]. Therefore, the analysis of the correlation of gene transcriptome data in CF and HF is of great importance for the later diagnosis and treatment. Previous studies have used transcriptome data to reveal potential biomarkers and developmental mechanisms of CF and HF [Citation18–20]. Transcriptome sequencing is a well-established method for analyzing the entire transcriptome and is commonly used to evaluate the differential expression of genes in case–control studies [Citation21]. However, few studies have used transcriptome sequencing to reveal common potential biomarkers of CF and HF. Thus, in order to determine common potential molecular markers for the early diagnosis and treatment of CF and HF, mRNA, miRNA and lncRNA expression data in CF and HF were analyzed. We selected differentially expressed mRNAs (DEmRNAs), differentially expressed miRNAs (DEmRNAs) and differentially expressed lncRNAs (DElncRNAs) shared by CF and HF to construct the mRNA-miRNA-lncRNA network. Finally, eight candidate molecules (hsa-miR-144-3p, CCNE2, C9orf72, MAP3K20-AS1, LEF1-AS1, AC243772.2, FLJ46284, and AC239798.2) may be used as the diagnosis and treatment targets of CF and HF. Although common biomarkers for CF and HF have been identified, the specific molecular mechanisms between them remain unclear. Identification of key biomarkers provides potential directions for further research. In other words, the identification of common biomarkers of CF and HF may help prevent CF to HF transition as early as possible.
Material and methods
Patients
The study population included three patients with CF, three patients with HF, and three healthy controls. All the patients are aged between 51 and 82 years old. Patients who were diagnosed with acute myocardial infarction 1 year later were diagnosed with CF and were included in the CF group. Detailed inclusion criteria for patients with myocardial infarction were as follows: (1) patients have chest pain or distress for >30 min within 24 h, and the myocardial enzymes creatine kinase (CK)-MB (the diagnostic cutoff value used is recommended to be 99th percentile of the upper limit of the reference value for normal people) and cardiac troponin T (cTnT) (within 3–12 h of the onset of acute myocardial infarction, cTnT can rise to 5 to 50 times that of healthy people) were higher than the normal range; (2) patients had a myocardial infarction for the first time; (3) patients did not receive medication or surgery before admission; (4) patients who had blood samples at three time points: before hospitalization, discharged and 6 months after myocardial infarction; (5) patients had complete clinical data, including gender, age, height, weight, etc. The detailed inclusion criteria for patients with HF were as follows: (1) patients must have had HF caused by myocardial infarction; (2) other indicators of patients should meet the diagnostic criteria for HF. Detailed exclusion criteria for patients with CF and HF were as follows: (1)Patients with myocarditis and other diseases caused by chest pain or distress; (2) Patients had a history of renal failure, advanced liver disease, malignancies, and other inflammatory diseases (psoriasis, rheumatoid arthritis, etc.); (3) patients were recurrent; (4) patients who had missing blood samples at three time points: before hospitalization, discharged, and 6 months after myocardial infarction; (5) patient had incomplete clinical data. Healthy controls included people who were considered healthy after a physical examination and did not have any disease.
RNA isolation
Whole blood samples were taken from each participant. The total RNA was extracted with TRIzol® Reagent [Citation22,Citation23]. The Nanodrop ND-2000 was used for detecting the concentration and purity of the proposed RNA. The integrity of the RNA was confirmed by agarose gel electrophoresis and the RNA integrity number (RIN) value was obtained by Agilent2100 Bioanalyzer [Citation24]. The total amount and concentration of RNA in a single library construction were 5 μg and ≥200 ng/μL, respectively.
MRNAs and lncRNA library construction, sequencing, and raw data processing
Illumina TruseqTM RNA sample prep kit was used to construct the specific library. Illumina Hiseq X-Ten platform was sequenced using the PE150 strategy [Citation25]. FastQC (http://www.bioinformatics.babraham.ac.uk/projects/fastqc/) [Citation26] was used to check the quality of sequencing and library construction. Fastx-Toolkit (http://hannonlab.cshl.edu/fastx_toolkit/) [Citation27] was used for quality control of raw reads. Specifically, adapter sequence, 5’ segment, 3’ segment, bases with quality <20 and reads with N > 10% were trimmed. The high-quality sequence obtained after quality control was aligned to the human reference genome (GRCh38) in the Ensemble database [Citation28] using the HISAT2 program (https://ccb.jhu.edu/software/hisat2/index.shtml). Expression of mRNAs and lncRNA were normalized and outputted with Stringtie (http://www.ccb.jhu.edu/software/stringtie/) [Citation29]. The abundance of transcripts was measured by fragments per kilobase of exon model per million mapped reads (FPKM).
MiRNA library construction, sequencing, and raw data processing
The length of 18–30 nt RNA was recovered from the total RNA to construct the small RNAs library. BGISEQ-500 platform was sequenced using the SE50 strategy [Citation30]. FastQC was used to check the quality of sequencing and library construction. Fastx-Toolki was used for quality control of raw reads. Specifically, joint sequence, bases with quality <20 and reads with N > 10% were trimmed. The sequences of length 18–32 nt were extracted. The Rfam (http://Rfam.sanger.ac.uk/) database in blast (http://blast.ncbi.nlm.nih.gov/) was used to annotate the measured small RNA [Citation31]. Clean reads were aligned to the human miRNA precursor and mature miRNA sequences in the miRBase database (http://www.mirbase.org/) [Citation32] using the Bowtie (http://bowtie-bio.sourceforge.net/index.shtml). The secondary structure of sequences (mapped to mature body regions) was predicted through RNAfold (http://rna.tbi.univie.ac.at/cgibin/RNAWebSuite/RNAfold.cgi). The transcription abundance of miRNAs was measured by miRDeep2 (https://github.com/Drmirdeep/drmirdeep.github.io/issues) [Citation33], and the expression level was homogenized by transcripts per million (TPM).
Differential analysis of mRNAs, lncRNAs, and miRNAs
The DESeq2 package in R was used to screen mRNAs, lncRNAs, and miRNAs with significant differences between different samples [Citation34]. The original read count (mainly to correct the sequencing depth) was standardized. The probability of hypothesis test (P-value) was calculated through the statistical model. Multiple hypothesis testing correction (Benjiamini and Hochberg method) was performed to obtain the corrected p value (false discovery rate, FDR). P-value (P) <0.05, |log2 fold change| (|log2FC|) ≥1 was the screening criteria of DElncRNAs, DEmRNAs, and DEmiRNAs.
Functional analysis of common DEmRNAs
To research the biological function of common genes, Gene Ontology (GO) [Citation35] and Kyoto Encyclopedia of Genes and Genomes (KEGG) [Citation36] pathway analyses were implemented by using the ConsensusPathDB (CPDB, http://cpdb.molgen.mpg.de/CPDB). P < 0.05 was the screening standard.
Construction of lncRNA-miRNA-mRNA network
Target mRNAs of common DEmiRNAs were predicted using six miRNA target gene prediction tools (PITA, RNA22, miRmap, microT, miRanda, and PicTar). Only miRNA-target pairs predicted by more than four algorithms can be selected. Then, the predicted target genes were intersected with the common DEmRNAs. The DEmiRNA-DEmRNA targeting and negative correlation pairs were screened out. Diana-lncbase V2.0 was used to predict the targeted relationship between common DEmiRNA and common DElncRNAs [Citation37]. Cytoscape was used to construct the targeted relational network diagram [Citation38]. Subsequently, DEmiRNA in the DEmiRNA-DElncRNA targeted relation pairs and the negative regulatory DEmiRNA-DEmRNA targeted relation pairs were intersects. In the end, lncRNA-miRNA-mRNA network was constructed.
Real time-polymerase chain reaction (RT-PCR) analysis
The total RNA in the blood samples from three CF patients, five HF patients, and five healthy controls was extracted by TRIzol® Reagent. The RT-PCR analysis sample was inconsistent with the preparation of the library sample. FastQuant cDNA synthesis kit (KR106, TIANGEN) was used for mRNA reverse transcription. MiRNA First Strand cDNA Synthesis kit (Tailing Reaction) (B532451-0020, Sangon Biotech) was used for miRNA reverse transcription. RT-PCR of mRNA and lncRNA was performed using SuperReal PreMix Plus (SYBR Green) SuperReal reagent (FP205, TIANGEN). RT-PCR of miRNA was performed using MicroRNAs qPCR Kit (SYBR Green Method) (B532461-0002, Sangon Biotech). Each experiment was repeated three times. Glyceraldehyde-3-phosphate dehydrogenase (GAPDH), actin beta (ACTB) and hsa-U6 were used as internal control for gene detection. Among them, hsa-U6 is the internal reference of miRNA. Hsa-U6 primers and reverse primer of hsa-miR-144-3P were obtained from the MicroRNAs qPCR Kit. Relative expression levels of genes were calculated using the 2−ΔΔCt method [Citation39].
All experimental procedures were approved by the Clinical Research Ethics Committee of the Shijiazhuang People’s Hospital (2021–019). Written consent was obtained from all the patients.
Expression validation and diagnostic analysis of key DElncRNAs and DEmRNAs
The GSE104150 (miRNA data from nine cases of HF and seven healthy controls) and GSE21125 (mRNA data from 18 cases of HF and nine healthy controls) data sets were obtained from the Gene Expression Omnibus (GEO) database [Citation40]. We used these two data sets for expression validation and diagnostic analysis of key DElncRNAs and DEmRNAs. The receiver operating characteristic (ROC) analysis was performed by using pROC package in R language. The sensitivity and specificity at the cutoffs were determined according to a previous study [Citation41].
Statistical analysis
In this study, GraphPad Prism was used for data statistics. For RT-PCR verification experiment, relative expression levels of genes were calculated using the 2−ΔΔCt method. The expression difference of mRNAs, lncRNAs, and miRNAs in CF patient group (GC), HF patient group (GH), and healthy control group (GD) was statistically analyzed by t-test. P < 0.05 was considered as statistical significance.
Results
HF is caused by overload and damage to the heart. CF is often caused by changes in the ECM of the heart. CF is defined as a key component of HF and has a strong connection with the progression of HF. In order to determine common potential molecular markers for the early diagnosis and treatment of CF and HF, we performed transcriptome sequencing analysis. The study population included three patients with CF, three patients with HF, and three healthy controls. RNA was extracted from blood samples for library construction and high-throughput sequencing. DESeq2 package in R was used to screen DEmRNAs, DElncRNAs, and DEmiRNAs between different samples. Subsequently, functional enrichment analysis, mutual network construction of lncRNA-miRNA-mRNA, in vitro validation, and diagnostic analysis were performed. All results indicate that hsa-miR-144-3p, CCNE2, C9orf72, MAP3K20-AS1, LEF1-AS1, AC243772.2, FLJ46284, and AC239798.2 may be key molecular markers of CF and HF. Hsa-miR-144-3p and CCNE2 may be considered as potential diagnostic gene biomarkers in CF and HF. The identification of common biomarkers of CF and HF can help prevent CF to HF transition as early as possible.
Differential expression analysis of mRNAs and lncRNAs
There were 1,477 DEmRNAs in the GC/GD group. The volcano map of DEmRNAs is shown in . The heat map of DEmRNAs is shown in . There were 607 DEmRNAs in the GH/GD group. The volcano map of DEmRNAs is shown in . The heat map of DEmRNAs is shown in . In addition, we found 146 common DEmRNAs in GC/GD and GH/GD groups, involved in 65 up-regulated mRNAs () and 81 down-regulated mRNAs ().
Figure 1. Volcano map, heatmap and venn diagram analysis of DEmRNAs in the GC/GD and GH/GD groups.
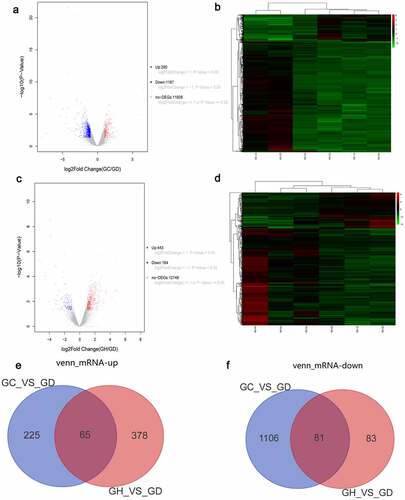
According to the above screening criteria, there were 502 DElncRNAs in the GC/GD group. The volcano map of DElncRNAs is shown in . The heat map of DElncRNAs is shown in . There were 379 DElncRNAs in the GH/GD group. The volcano map of DElncRNAs is shown in . The heat map of DElncRNAs is shown in . In addition, we found 90 common DElncRNAs in GC/GD and GH/GD groups, involved in 53 up-regulated lncRNAs () and 27 down-regulated lncRNAs ().
Figure 2. Volcano map, heatmap and Venn diagram analysis of DElncRNAs in the GC/GD and GH/GD groups.
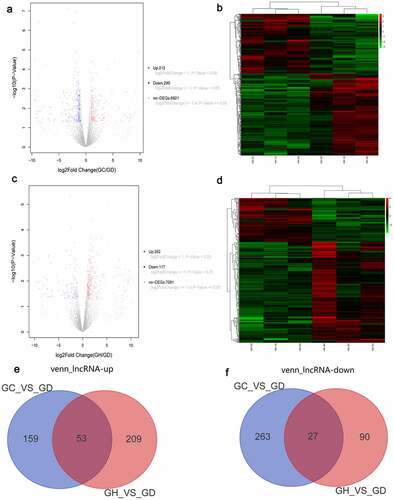
Differential expression analysis of miRNAs
A total of 36 DEmiRNAs in the GC/GD group were acquired. The volcano map of DEmiRNAs is shown in . The heat map of DEmiRNAs is shown in . A total of 42 DEmiRNAs in the GH/GD group were acquired. The volcano map of DEmiRNAs is shown in . The heat map of DEmiRNAs is shown in . In addition, we found six common DEmiRNAs in GC/GD and GH/GD groups, containing three up-regulated miRNAs () and three down-regulated miRNAs ().
Figure 3. Volcano map, heatmap and Venn diagram analysis of DEmiRNAs in the GC/GD and GH/GD groups.
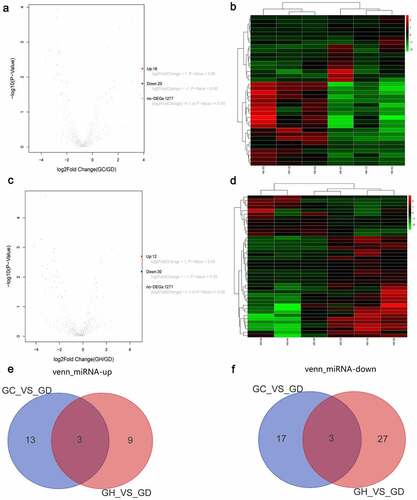
Functional analysis of common DEmRNAs
In terms of biological process (BP), DEmRNAs were involved in cellular response to stimulus, cell communication, and signal transduction. In terms of cell composition (CC), DEmRNAs were involved in plasma membrane, cell periphery, and intrinsic component of membrane. BP and CC enrichment results of the top 15 are shown in . In terms of molecular function (MF), DEmRNAs were involved in protein binding, signaling receptor activity and protein-containing complex binding (). In addition, we found that the DEmRNAs are mainly involved in PI3K-Akt signaling pathway, cellular senescence, and transcriptional misregulation in cancer ().
Figure 4. Significantlly enriched GO terms and KEGG pathways of common DEmRNAs.
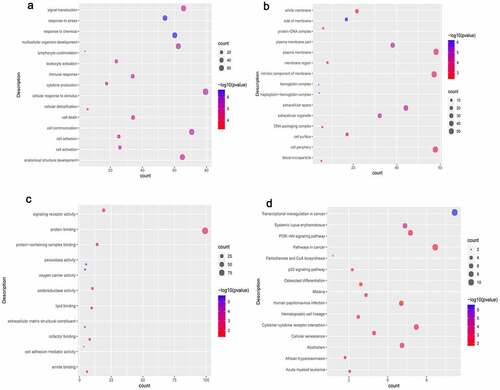
Mutual network construction
The predicted target genes (Table S1 and Table S2) were intersected with the common DEmRNAs, and the DEmiRNA-DEmRNA targeting and negative correlation pairs were screened out. A total of two DEmiRNA-DEmRNA targeting and negative correlation pairs were obtained (). In addition, we further verified the targeting relationship of hsa-miR-144-3p-CCNE2/C9orf72 using TargetScan software (http://www.targetscan.org/vert_72/) [Citation42]. The results again confirmed the targeting relationship between hsa-miR-144-3p and CCNE2 (Supplementary Fig. 1). A total of 31 DEmiRNA-DElncRNA targeting correlation pairs were obtained (Table S3). The targeted relationship network between them is shown in . In the end, only one common DEmiRNA was identified in the DEmiRNA-DEmRNA correlation pairs and DEmiRNA-DElncRNA correlation pairs. The lncRNA-miRNA-mRNA network of the DEmiRNA consists of 8 nodes and 7 edges, as shown in .
Table 1. DEmiRNA-DEmRNA targeted relationship
RT-PCR validation
In this study, CCNE2, C9orf72, LEF1-AS1, MLK7-AS1, and hsa-miR-144-3p were reported as heart-related genes and selected for RT-PCR verification. The primers are shown in . Compared with GD, CCNE2, C9orf72 had up-regulation trend and LEF1-AS1, hsa-miR-144-3p were down-regulation trend in GCs. Among which, LEF1-AS1 showed a significant difference. However, MLK7-AS1 had opposite with bioinformatics analysis. Small sample size may cause some errors. Meanwhile, compared with GD, CCNE2, C9orf72, MLK7-AS1 were up-regulation trend and LEF1-AS1, hsa-miR-144-3p had down-regulation trend in GH. Among which, C9orf72 showed a significant difference ().
Table 2. Primer sequence in the RT-PCR
Expression validation and diagnostic analysis of CCNE2 and hsa-miR-144-3p
RNA sequencing types of GSE104150 and GSE21125 data sets are miRNA and mRNA, respectively. We used these two data sets for expression validation and diagnostic analysis of identified genes. CCNE2, C9orf72, and hsa-miR-144-3p were randomly selected. In our previous analysis, we found that only CCNE2 and hsa-miR-144-3p were expressed in the corresponding data set. We found that the expression levels of CCNE2 and hsa-miR-144-3p were up-regulated and down-regulated in the blood of HF, respectively (). This result is consistent with our transcriptome sequencing. Simultaneously, diagnostic analysis of CCNE2 and hsa-miR-144-3p was performed. In the ROC curve analysis, the area under curve (AUC) of CCNE2 and hsa-miR-144-3p were 0.691 and 0.810, respectively (). It is indicated that CCNE2 and hsa-miR-144-3p may be considered the potential diagnostic gene biomarkers in HF.
Discussion
Mechanisms underlying CF and HF progression are mediated by multiple molecules [Citation43–45], and numerous previous studies have been based on molecular studies of a single disease of CF or HF. Conspicuously, HF is invariably accompanied by development of CF [Citation46]. The blood transcriptome can reflect the state of the disease [Citation47,Citation48]. However, few studies have identified common novel biomarkers from the blood transcriptome of CF and HF patients. Therefore, our study aims to identify common molecular markers of CF and HF by analyzing transcriptome sequencing data to provide a potential theoretical basis for studying the transition from CF to HF. In this study, we identified a large number of DEmRNAs, DEmiRNAs, and DEmRNAs, and screened out 146 DEmRNAs, 80 DElncRNAs, and 6 DEmiRNAs shared by CF and HF. Subsequently, eight key molecular markers (CCNE2, C9orf72, hsa-miR-144-3p, MAP3K20-AS1, LEF1-AS1, AC243772.2, FLJ46284, and AC239798.2) were obtained through bioinformatics analysis.
Cyclin E2 (CCNE2) is a cyclin regulating protein that plays a role in G1/S transition in the cell cycle [Citation49]. During heart development, ugly duckling (udu) mutations lead to cardiomyocyte deficiency, which is caused by reduced cell proliferation, and the expression of the cell cycle regulator CCNE2 is reduced during this process [Citation50]. Cardiac fibroblasts are the main cell population of the heart, and an important feature is the ability to produce ECM [Citation51]. Activation and regeneration of fibroblasts are important factors for fibrosis [Citation51–53]. In addition, CCNE2 also promotes cell proliferation and migration [Citation54]. In this study, the expression of CCNE2 was up-regulated. Therefore, we speculated that it might be related to the proliferation and differentiation of cardiac fibroblasts, thus playing an important regulatory role in CF and HF. It is worth mentioning that CCNE2 had a potential diagnostic value for HF in our study.
The protein encoded by the chromosome 9 open reading frame 72 (C9orf72) gene plays an important role in endosomal transport [Citation55]. Interestingly, C9orf72 over-expression can inhibit autophagy in cell starvation [Citation56]. Previous studies have found that the interaction between toll-like receptor 2 (TLR2) and high-mobility group box 1 (HMGB1) participates in the pathogenesis of CF through inhibition of fibroblast autophagy [Citation57]. C9orf72 was an up-regulated gene in this study, so we speculated that it may regulate CF and HF by regulating the autophagy of fibroblasts. Moreover, C9orf72 is also a targeted gene for hsa-miR-144-3p, which further suggests that C9orf72 may play a key regulatory role in the progression of CF and HF.
Hsa-miR-144-3p has been confirmed to play a protective role in ischemia/reperfusion (I/R) by targeting the CUG triplet repeat-binding protein 2- cyclooxygenase-2 (CUGBP-COX2) signaling pathway to reduce I/R-induced cardiomyocyte death [Citation58]. The expression level of hsa-miR-144-3p was markedly down-regulated in myocardial infarction and left anterior descending, compared with normal control [Citation59]. Furthermore, it was confirmed that up-regulation of metastasis-associated lung adenocarcinoma transcript 1 (MALAT1) plays a key role in promoting myocardial apoptosis by targeting hsa-miR-144-3p [Citation59]. In addition, hsa-miR-144 can also improve mitochondrial biogenesis and reduce cell apoptosis by targeting Rac family small GTPase 1 (RAC-1), thereby protecting the heart from hyperglycemic induced damage [Citation60]. In this study, hsa-miR-144-3p was significantly down-regulated, and we speculated that it may participate in the regulation of CF and HF by regulating apoptosis of cardiomyocytes. Moreover, we further verified the targeting relationship of hsa-miR-144-3p-CCNE2/C9orf72 using TargetScan software. The results again confirmed the targeting relationship between hsa-miR-144-3p and CCNE2, which provided a direction for further study of the molecular mechanism of CF and HF. It is worth mentioning that hsa-miR-144-3p had a potential diagnostic value for HF in our study.
Functionally, LEF1 antisense RNA 1 (LEF1-AS1) knockdown can promote cell proliferation and migration [Citation61]. Although LEF1-AS1 has been rarely studied in heart disease, it is up-regulated in many diseases such as cell lung cancer and esophageal squamous cell carcinoma, and promotes cell proliferation [Citation62,Citation63]. MAP3K20 antisense RNA 1 (MAP3K20-AS1) also known as MLK7-AS1, has been mostly studied in cancer, and its reduced expression plays a role in promoting the proliferation of cancer cells [Citation64,Citation65]. In this study, LEF-AS1 and MAP3K20-AS1 were down-regulated and up-regulated, respectively. Therefore, we speculated that LEF-AS1 and MAP3K20-AS1 may be involved in regulating the formation of CF and HF by regulating the proliferation of cardiac myocytes and cardiac fibroblasts. It is noteworthy that the lncRNA-miRNA-mRNA network was formed between hsa-miR-144-3p, CCNE2, C9orf72, LEF1-AS1, MAP3K20-AS1, AC239798.2, AC243772.2, and FLJ46284, which may play an important role in regulating CF and HF through mutual regulation.
GO uses structured vocabularies (or terms) to describe the molecular functions, biological roles, and cellular locations of gene products [Citation66]. In this study, GO enrichment analysis was used to evaluate the potential mechanism of common DEmRNAs. The results showed that these DEmRNAs participated in a variety of biological functions (for example, cell communication and protein binding). Moreover, both cell communication and protein binding are involved in regulating the progression of heart disease [Citation67–70]. In addition, KEGG analysis showed that common DEmRNAs were significantly enriched in PI3K-Akt and cellular senescence signaling pathways. PI3K-Akt and cellular senescence signaling pathways are involved in regulating the occurrence and progression of heart disease via regulating cell survival, apoptosis, growth, cardiac contractility, and even the transcription of related genes [Citation71,Citation72]. The results of GO and KEGG functional analysis suggest that intersecting DEmRNAs may regulate the progression of CF-HF by participating in different biological functions.
Collectively, a number of DElncRNA, DEmiRNAs, and DEmRNAs were identified in this study. However, this experiment has certain limitations. Firstly, the sample size of RT-PCR experiments is small and more blood samples from patients with CF and HF are needed to verify the data of this study. Secondly, the hsa-miR-144-3p network should be functionally validated by experimental evidence in a cell model. Third, the specific molecular mechanism of the identified genes in CF and HF is still unclear, and a large number of experiments are needed to further study their molecular mechanism.
Conclusion
In this study, 146 DEmRNAs, 80 DElncRNAs, and 6 DEmiRNAs were shared by CF and HF. The identification of hsa-miR-144-3p, CCNE2, C9orf72, MAP3K20-AS1, LEF1-AS1, AC243772.2, FLJ46284, and AC239798.2 in the lcRNA-miRNA-mRNA network implies that they may be involved in the progression from CF to HF. Moreover, according to the ROC analysis results, it is suggested that hsa-miR-144-3p and CCNE2 may be considered as potential diagnostic gene biomarkers in HF. Our study can provide a theoretical basis for the diagnosis and mechanism research of the development process of CF-HF patients and provide potential directions for the latter molecular mechanism research.
Highlights
Hsa-miR-144-3p and CCNE2 may be potential diagnostic gene biomarkers in HF
Eight candidate genes may be used as the diagnosis and treatment targets of CF and HF
Identification of common biomarkers may help prevent CF to HF transition as early as possible
Authors’ contributions
Conception and design: S.W.
Administrative support: X.H.
Data analysis and interpretation: T.L. and Q.C.
Collection and assembly of data: Y.Y. and L.X.
Provision of study materials or patients: X.Z., E.W., and Y.L.
All authors have made important contributions to data analysis, drafting the article or revising the article.
Availability of data and materials
The datasets generated during and/or analyzed during the current study are available from the corresponding author on reasonable request. The transcriptome data have been uploaded to Gene Expression Omnibus (accession number GSE196656 and GSE196421).
Consent for publication
All of the authors have agreed to the publication of the work.
Ethics approval and consent to participate
All participants were informed as to the purpose of this study, and that this study complied with the Declaration of Helsinki. All experimental procedures were approved by Shijiazhuang People’s Hospital (2021-019).
Supplemental Material
Download Zip (443.3 KB)Disclosure statement
No potential conflict of interest was reported by the author(s).
Supplementary material
Supplemental data for this article can be accessed here.
Additional information
Funding
References
- Snipelisky D, Chaudhry SP, Stewart GC. The many faces of heart failure. Card Electrophysiol Clin. 2019;11(1):11–20.
- Gyöngyösi M, Winkler J, Ramos I, et al. Myocardial fibrosis: biomedical research from bench to bedside. Eur J Heart Fail. 2017;19(2):177–191.
- López B, González A, Ravassa S, et al. Circulating biomarkers of myocardial fibrosis: the need for a reappraisal. J Am Coll Cardiol. 2015;65(22):2449–2456.
- Travers JG, Kamal FA, Robbins J, et al. Cardiac fibrosis: the fibroblast awakens. Circ Res. 2016;118(6):1021–1040.
- Babür Güler G, Karaahmet T, Tigen K. Myocardial fibrosis detected by cardiac magnetic resonance imaging in heart failure: impact on remodeling, diastolic function and BNP levels. Anadolu Kardiyol Derg. 2011;11(1):71–76.
- Yuan J, Liu H, Gao W, et al. MicroRNA-378 suppresses myocardial fibrosis through a paracrine mechanism at the early stage of cardiac hypertrophy following mechanical stress. Theranostics. 2018;8(9):2565–2582.
- Veeraveedu PT, Sanada S, Okuda K, et al. Ablation of IL-33 gene exacerbate myocardial remodeling in mice with heart failure induced by mechanical stress. Biochem Pharmacol. 2017;138:73–80.
- Wojciechowska A, Braniewska A, Kozar-Kamińska K. MicroRNA in cardiovascular biology and disease. Adv Clin Exp Med. 2017;26(5):865–874.
- Liu X, Xu Y, Deng Y, et al. MicroRNA-223 regulates cardiac fibrosis after myocardial infarction by targeting RASA1. Cell Physiol Biochem. 2018;46(4):1439–1454.
- Yuan X, Pan J, Wen L, et al. MiR-144-3p enhances cardiac fibrosis after myocardial infarction by targeting PTEN. Front Cell Dev Biol. 2019;7:249.
- Verjans R, Peters T, Beaumont FJ, et al. MicroRNA-221/222 family counteracts myocardial fibrosis in pressure overload-induced heart failure. Hypertension. 2018;71(2):280–288. Dallas Tex: 1979.
- Liu W, Zheng J, Dong J, et al. Association of miR-197-5p, a circulating biomarker for heart failure, with myocardial fibrosis and adverse cardiovascular events among patients with stage C or D heart failure. Cardiology. 2018;141(4):212–225.
- Hao K, Lei W, Wu H, et al. LncRNA-Safe contributes to cardiac fibrosis through Safe-Sfrp2-HuR complex in mouse myocardial infarction. Theranostics. 2019;9(24):7282–7297.
- Zheng D, Zhang Y, Hu Y, et al. Long noncoding RNA crnde attenuates cardiac fibrosis via Smad3-Crnde negative feedback in diabetic cardiomyopathy. FEBS J. 2019;286(9):1645–1655.
- Fargnoli AS, Katz MG, Bridges CR, et al. Gene therapy in heart failure. Handb Exp Pharmacol. 2017;243:395–421.
- Azevedo CF, Nigri M, Higuchi ML, et al. Prognostic significance of myocardial fibrosis quantification by histopathology and magnetic resonance imaging in patients with severe aortic valve disease. J Am Coll Cardiol. 2010;56(4):278–287.
- Aoki T, Fukumoto Y, Sugimura K, et al. Prognostic impact of myocardial interstitial fibrosis in non-ischemic heart failure. -Comparison between preserved and reduced ejection fraction heart failure. Circ J. 2011;75(11):2605–2613.
- McLellan MA, Skelly DA, Dona MSI, et al. High-Resolution transcriptomic profiling of the heart during chronic stress reveals cellular drivers of cardiac fibrosis and hypertrophy. Circulation. 2020;142(15):1448–1463.
- Liang X, Bai Z, Wang F, et al. Full-Length transcriptome sequencing: an insight into the dog model of heart failure. Front Cardiovasc Med. 2021;8:712797.
- Liu CF, Ni Y, Moravec CS, et al. Whole-Transcriptome profiling of human heart tissues reveals the potential novel players and regulatory networks in different cardiomyopathy subtypes of heart failure. Circ Genomic Precis Med. 2021;14(1):e003142.
- Wang Z, Gerstein M, Snyder M. RNA-Seq: a revolutionary tool for transcriptomics. Nat Rev Genet. 2009;10(1):57–63.
- Wang S, Wang E, Chen Q, et al. Uncovering potential lncRNAs and mRNAs in the progression from acute myocardial infarction to myocardial fibrosis to heart failure. Front Cardiovasc Med. 2021;8:664044.
- Rio DC, Ares M Jr., Hannon GJ, et al. Purification of RNA using TRIzol (TRI reagent). Cold Spring Harb Protoc. 2010;2010(6): db.prot5439.
- Schroeder A, Mueller O, Stocker S, et al. The RIN: an RNA integrity number for assigning integrity values to RNA measurements. BMC Mol Biol. 2006;7:3.
- Nair SS, Luu PL, and Qu W, et al. Guidelines for whole genome bisulphite sequencing of intact and FFPET DNA on the Illumina HiSeq X Ten Epigenetics Chromatin . 2018;11(1):24.
- Andrews S. FastQC: a quality control tool for high throughput sequence data. Cambridge United Kingdom: Babraham Bioinformatics, Babraham Institute; 2010.
- Schmieder R, Edwards R. Quality control and preprocessing of metagenomic datasets. Bioinformatics. 2011;27(6):863–864.
- Church DM, Schneider VA, Graves T, et al. Modernizing reference genome assemblies. PLoS Biol. 2011;9(7):e1001091.
- Pertea M, Pertea GM, and Antonescu CM, et al. StringTie enables improved reconstruction of a transcriptome from RNA-seq reads nature biotechnology . 2015;33(3):290–295.
- Zhu FY, Chen MX, and Ye NH, et al. Comparative performance of the BGISEQ-500 and Illumina HiSeq4000 sequencing platforms for transcriptome analysis in plants Plant Methods . 2018;14:69.
- Kalvari I, Nawrocki EP, Ontiveros-Palacios N, et al. Rfam 14: expanded coverage of metagenomic, viral and microRNA families. Nucleic Acids Res. 2021;49(D1):D192–d200.
- Griffiths-Jones S, Grocock RJ, van Dongen S, et al. miRBase: microRNA sequences, targets and gene nomenclature. Nucleic Acids Res. 2006;34( Database issue):D140–4.
- Mackowiak SD. Identification of novel and known miRNAs in deep-sequencing data with miRDeep2. Curr Protoc Bioinformatics. 2011;36(1): Chapter 12:Unit 12.0. doi:10.1002/0471250953.bi1210s36.
- Love MI, Huber W, Anders S. Moderated estimation of fold change and dispersion for RNA-seq data with DESeq2. Genome Biol. 2014;15(12):550.
- The Gene Ontology Consortium, Gene ontology consortium: going forward. Nucleic Acids Res. 2015;43( Database issue):D1049–56.
- Kanehisa M, Goto S. KEGG: Kyoto encyclopedia of genes and genomes. Nucleic Acids Res. 2000;28(1):27–30.
- Karagkouni D, Paraskevopoulou MD, Tastsoglou S, et al. DIANA-LncBase v3: indexing experimentally supported miRNA targets on non-coding transcripts. Nucleic Acids Res. 2020;48(D1):D101–d10.
- Shannon P, Markiel A, Ozier O, et al. Cytoscape: a software environment for integrated models of biomolecular interaction networks. Genome Res. 2003;13(11):2498–2504.
- Livak KJ, Schmittgen TD. Analysis of relative gene expression data using real-time quantitative PCR and the 2(-Delta delta C(T)) method. Method (San DiegoCalif). 2001;25(4):402–408.
- Wen J, Yang H, Liu MZ, et al. Gene expression analysis of pretreatment biopsies predicts the pathological response of esophageal squamous cell carcinomas to neo-chemoradiotherapy. Ann Oncol. 2014;25(9):1769–1774.
- Šimundić AM. Measures of diagnostic accuracy: basic definitions. Ejifcc. 2009;19(4):203–211.
- Agarwal V, Bell GW, and Nam JW, et al. Predicting effective microRNA target sites in mammalian mRNAs. eLife. 2015;4 e05005 .
- Chen Y, Huang M, Yan Y, et al. Tranilast inhibits angiotensin II-induced myocardial fibrosis through S100A11/ transforming growth factor-β (TGF-β1)/Smad axis. Bioengineered. 2021;12(1):8447–8456.
- Qi Y, Tang Y, Yin L, et al. miR-129-5p restores cardiac function in rats with chronic heart failure by targeting the E3 ubiquitin ligase Smurf1 and promoting PTEN expression. Bioengineered. 2022;13(2):2371–2386.
- Hu L, Xu Y, Wang Q, et al. Yiqi Huoxue Recipe inhibits cardiomyocyte apoptosis caused by heart failure through Keap1/Nrf2/HIF-1α signaling pathway. Bioengineered. 2021;12(1):969–978.
- Burke RM, Lighthouse JK, Mickelsen DM, et al. Sacubitril/valsartan decreases cardiac fibrosis in left ventricle pressure overload by restoring PKG signaling in cardiac fibroblasts. Circ Heart Fail. 2019;12(4):e005565.
- Park HJ, Noh JH, Eun JW, et al. Assessment and diagnostic relevance of novel serum biomarkers for early decision of ST-elevation myocardial infarction. Oncotarget. 2015;6(15):12970–12983.
- Shen M, Gong R, Li H, et al. Identification of key molecular markers of acute coronary syndrome using peripheral blood transcriptome sequencing analysis and mRNA-lncRNA co-expression network construction. Bioengineered. 2021;12(2):12087–12106.
- Bayard Q, Meunier L, Peneau C, et al. Cyclin A2/E1 activation defines a hepatocellular carcinoma subclass with a rearrangement signature of replication stress. Nature Communications. 2018;9(1):5235.
- Budine TE, de Sena-tomás C, Williams MLK, et al. Gon4l/Udu regulates cardiomyocyte proliferation and maintenance of ventricular chamber identity during zebrafish development. Dev Biol. 2020;462(2):223–234.
- Moore-Morris T, Guimarães-Camboa N, Yutzey KE, et al. Cardiac fibroblasts: from development to heart failure. J Mol Med. 2015;93(8):823–830. Berlin Germany.
- Ivey MJ, Tallquist MD. Defining the cardiac fibroblast. Circ J. 2016;80(11):2269–2276.
- Tallquist MD, Molkentin JD. Redefining the identity of cardiac fibroblasts. Nat Rev Cardiol. 2017;14(8):484–491.
- Yang J, Dong Z, and Ren A, et al. Antibiotic tigecycline inhibits cell proliferation, migration and invasion via down‐regulating CCNE2 in pancreatic ductal adenocarcinoma. J Cell Mol Med. 2020;24(7 4245–4260).
- Farg MA, Sundaramoorthy V, Sultana JM, et al. C9ORF72, implicated in amyotrophic lateral sclerosis and frontotemporal dementia, regulates endosomal trafficking. Hum Mol Genet. 2014;23(13):3579–3595.
- Cali CP, Patino M, Tai YK, et al. C9orf72 intermediate repeats are associated with corticobasal degeneration, increased C9orf72 expression and disruption of autophagy. Acta Neuropathologica. 2019;138(5):795–811.
- Wu RN, Yu TY, Zhou JC, et al. Targeting HMGB1 ameliorates cardiac fibrosis through restoring TLR2-mediated autophagy suppression in myocardial fibroblasts. Int J Cardiol. 2018;267:156–162.
- Zhang X, Wang X, Zhu H, et al. Synergistic effects of the GATA-4-mediated miR-144/451 cluster in protection against simulated ischemia/reperfusion-induced cardiomyocyte death. J Mol Cell Cardiol. 2010;49(5):841–850.
- Gong X, and Zhu Y. Long noncoding RNA MALAT1 promotes cardiomyocyte apoptosis after myocardial infarction via targeting miR-144-3p bioscience reports . 2019;39(8 BSR20191103).
- Tao L, Huang X, Xu M, et al. MiR-144 protects the heart from hyperglycemia-induced injury by regulating mitochondrial biogenesis and cardiomyocyte apoptosis. FASEB J. 2020;34(2):2173–2197.
- Zhang C, Bao C, Zhang X, et al. Knockdown of lncRNA LEF1-AS1 inhibited the progression of oral squamous cell carcinoma (OSCC) via Hippo signaling pathway. Cancer Biol Ther. 2019;20(9):1213–1222.
- Yang J, Lin X, Jiang W, et al. lncRNA LEF1-AS1 promotes malignancy in Non-Small-Cell lung cancer by modulating the miR-489/SOX4 axis. DNA Cell Biol. 2019;38(9):1013–1021.
- Zong MZ, Feng WT, Du N, et al. Upregulation of long noncoding RNA LEF1-AS1 predicts a poor prognosis in patients with esophageal squamous cell carcinoma. Eur Rev Med Pharmacol Sci. 2019;23(18):7929–7934.
- Yan H, Li H, Li P, et al. Long noncoding RNA MLK7-AS1 promotes ovarian cancer cells progression by modulating miR-375/YAP1 axis. J Exp Clin Cancer Res. 2018;37(1):237.
- Zhang R, Li J, Yan X, et al. Long non‑coding RNA MLK7‑AS1 promotes proliferation in human colorectal cancer via downregulation of p21 expression. Mol Med Rep. 2019;19(2):1210–1221.
- Zhao Y, Fu G, Wang J, et al. Gene function prediction based on gene ontology hierarchy preserving hashing. Genomics. 2019;111(3):334–342.
- Bang C, Antoniades C, Antonopoulos AS, et al. Intercellular communication lessons in heart failure. Eur J Heart Fail. 2015;17(11):1091–1103.
- Valiente-Alandi I, Schafer AE, Blaxall BC. Extracellular matrix-mediated cellular communication in the heart. J Mol Cell Cardiol. 2016;91:228–237.
- Lam C, Casamian-Sorrosal D, Monteith G, et al. Heart-fatty acid binding protein in dogs with degenerative valvular disease and dilated cardiomyopathy. Vet J. 2019;244:16–22. London England: 1997.
- Wang Y, Zhu Y, Pu Z, et al. Soluble resistance-related calcium-binding protein participates in multiple diseases via protein-protein interactions. Biochimie. 2021;189:76–86.
- Qin W, Cao L. Role of PI3K/Akt signaling pathway in cardiac fibrosis. Molecular and Cellular Biochemistry. 2021;476(11):4045–4059.
- Yan C, Xu Z, Huang W. Cellular senescence affects cardiac regeneration and repair in ischemic heart disease. Aging Dis. 2021;12(2):552–569.