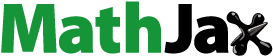
ABSTRACT
Economic inequality in South America decreased steadily since 2002. However, as the beginning of the 2010s marked the end of the commodities boom in the region, economic inequality showed constant or even increasing rates in some of these countries. The decrease in economic inequality has often been related to the impact of the macro economic changes, like the boom of the commodity prices and the institutional reinforcement, and changes in the labor market. Therefore, the proposedhyphotesis is that productive capacity improvement of the less educated has played a key role in reducing inequality. Productive capacity takes into consideration three variables related to hard and soft skills to work, which are educational coverage, internet access and life conditions. Results show that, in addition to demand forces, the improvement of the productive capacity of the less educated is positively and significantly related to the reduction of inequality in South America in 2002–2011.
1. Introduction
Inequality in South AmericaFootnote1 decreased during 2002–2011 in 7.19 Gini points. In this period, one of the most important reductions was the decrease in labor income inequality (Helfand, Rocha, and Vinhais Citation2009; Maurizio Citation2015; Azevedo et al. Citation2013). This decrease of the labor income inequality has been widely related to the demand forces of the labor market (Gasparini and Lustig Citation2011). One of the frequently studied causes is economic growth, related to the commodity prices boom, and the institutional reinforcement of the labor market. Their impact are in most of the cases positive, but modest (Lustig, Lopez, and Ortiz Citation2013; Adão Citation2015). Nonetheless, the reduction of economic inequality continued after 2011, when the demand forces of the labor market stopped their increase. However, since the early 2010s, inequality has shown a turnover with constant or even increasing rates in some parts of the region, showing signs of economic exhaustion (Cord et al. Citation2017; Lustig Citation2020). As these years mark the end of the commodity boom for the region, the development these countries achieved in reducing inequality was not entirely sustainable. For this matter, more causes need to be explored in order to explain the notable reduction of inequality in the region during the analized period.
Attending to the supply forces, related to changes of the labor maket, mostly estructural, we explore the improvement of the work capacity of the less educated. From the human capital theory, better productive capacity of the lowest educated would improve their productivity and consequently their wages, reducing inequality (Nafukho, Hairston, and Brooks Citation2004). Gasparini and Lustig (Citation2011), explained that basic education coverage expanded in the region during this period, which in fact could explain a reduction of inequality in attainment, which made the returns of education curve less steep (which helps to reduce the skill premium, i.e. the ratio of the wages of high skilled workers respect to unskilled workers). In the same line, Lustig, Lopez, and Ortiz (Citation2013) showed that, during this period, the reduction of income inequality in Brazil, could be explained by a fall in inequality of education and a fall in the steepness of the returns of education. As education, given the Human Capital Theory, can be one of the main factors that explain productive capacity, we use educational coverage as a proxy for productive capacity. However, this is not the only variable that should be considered, which is why we calculated an index of productive capacity that will be explained next.
The hypothesis in this paper is that productive capacity is a key factor to explain the inequality reduction in South America in 2002–2011. Due to the lack of information about productive capacity by quintiles in the region, we calculate the Productive Capacity Index (PCI) taking into consideration three variables related to hard and soft skills to work, which are: educational coverage, internet access and life conditions. We applied a panel data model where the dependent variable is the skill premium, that is, the ratio between the labor income of highly educated workers in relation to the labor income of workers with low education of the labor force. In addition to the PCI, we used the internal demand growth and terms of trade as variables to capture the demand side, the Labor Market Regulation Index (LMRI) and government public spending. The data sources are the Center for Distributive, Labor and Social Studies (CEDLAS) based on the Socio-Economic Database for Latin America and the Caribbean, the Harmonized Household Surveys of Latin America and the Caribbean (CMAEH) database by the Inter-American Development Bank (IDB Citation2019), the World Bank (Citation2019), and the Fraser Institute (Citation2019).
The main contribution of the present paper to the existent literature is that it provides evidence of the relationship between productive capacity of the lower quintiles, measured not only by educational coverage but also by internet access and life conditions (related to multidimensional poverty), with a reduction of the skill premium, which, is one of the main causes stated in the literature of the important reduction of inequality in South America during 2002–2011. Results show that, in addition to demand forces, the improvement of the productive capacity of the less educated is positively and significantly related to the reduction of inequality in South America in 2002–2011.
The remainder of this study is organized as follows. In Section 2, we revise the theoretical framework of the relationship between productive capacity and skill premium, which provides knowledge on poverty and inequality, and sintezises the poverty background in South America. Section 3 presents the data used in this paper, and our empirical strategy to determine that productive capacity has played a key role in reducing income inequality. Section 4 shows our suggested results, and the respective discussion of our findings. Finally, we end our study with our conclusions on Section 5.
2. Theoretical framework
South America achieved the largest income inequality decline in its recent history in 2002–2011. It was produced after two decades of rising (Cord et al. Citation2017), and before a tendency of moderate decrease (Amarante and Colacce Citation2018). In 1980–2002 the Gini index increased by 3.01 points, a 0.37% each year; in 2002–2011 it decreased by 7.19 points, a 1.5% per year and in 2012–2016 it reduced a 1.08% each year (World Bank Citation2019). It was also the biggest reduction in the recent history of the developing regions (Williamson Citation2015). What does explain the remarkable reduction of the income inequality in South America in 2002–2011?
Given that the contribution to the household per capita income in Latin America in 2009 was headed in an 80% by labor income and in a 15% by transfers from the public sector (Keifman and Maurizio Citation2012), inequality variations in the region had been explored from literature through this two paths.
Attending to the influence of labor incomes changes on the inequality decline, Helfand, Rocha, and Vinhais (Citation2009) in Brazil and Keifman and Maurizio (Citation2012) in Argentina, Brazil, Chile and Paraguay, applied a dynamic decomposition methodology (Lerman and Yitzhaki Citation1985) to confirm that it could explain over the 60% of the decline in inequality. Bérgolo and Cruces (Citation2011), in Argentina, Brazil, Chile and Uruguay, and Azevedo et al. (Citation2013) in Latin American countries, used a non-parametric decomposition method proposed by Barros et al. (Citation2006), arriving to a similar conclusion. The implementation or expansion of contributive and non-contributory transfer programs was the second cause of the decline in inequality, accounting for 15% of the overall reduction. Uruguay was the exception, where increases in transfers were the main cause of the decrease in inequality, followed by labor income.
Labor income inequality changes come as the most important factors to understand the income inequality reduction in South America. These show the same behavior in recent decades. After a rising tendency, wage inequality between 2002 and 2011 was reduced by 5.5 points, with an average annual variation of −0.96% per year. In 2011–2016 it was reduced by 1.2 points on average, with an average change of −0.39% each year (CEDLAS Citation2019).
The decrease in labor income inequality in South America in 2002–2011 has been widely related to economic growth in the same period, contributing to the global theory that states an inverse relationship between growth and inequality (Galbraith Citation2016). The South American economic growth in this period is mainly explained by the increase in the prices of raw materials in a context of the high prevalence of the commodities in exports, and a positive or neutral behavior of the most relevant related economic variables (Rosnick and Weisbrot Citation2014). The commodity price index increased by 225% in 2002–2011, while the raw materials accounted for 56% of South American exports in the period (IMF Citation2019).
The economic growth is directly related to the reduction of wage inequality through the market forces. The terms of trade increased the South American aggregate domestic demand and, consequently, the labor demand, but their impact was different for tradable and non-tradable goods and services (De la Torre et al. Citation2015). The demand for tradables goods could be satisfied by national production and imports. As tradable goods are intensive in high skilled workers, the labor demand increase would affect less their wages. The demand for non-tradable goods and services, which are intensive in low skilled workers, cannot be satisfied by imports. Low-skill workers would be in greater demand and, consequently, their wages would be put under great pressure (Benguria, Saffie, and Urzúa Citation2018; Messina and Silva Citation2017).
Despite the positive impact of economic growth on wage equality, it seems to be moderate. Adão (Citation2015) applied a Roy-like model in Brazil to conclude that commodity prices explain 5–10% of the fall in earnings inequality in 2002–2011. Attending to the sustainability of the effects, 50% of the changes provoqued by the boom desapeared in the following years (Benguria, Saffie, and Urzúa Citation2018). Adão, Arkolakis, and Esposito (Citation2019) pointed out the pending structural reforms in the region to explain the minimal impact of the economic growth on inequality, and its sustainability.
Considering the pending structural reforms, in the case of the labor market, informality is in fact a real option for employers and employees. It reduces the pressure of labor demand on wages and minimizes its real effect because a large part is absorved by informality. In 2011, informality affected 75% of the South American workers (IDB Citation2019). The productive structure also did not contribute to reduce labor inequality. The traditional simplicity of the exports is concentrated in primary products (Hartmann et al. Citation2017) and the low productivity levels, that limits the possibility of competing in the global market for high value-added goods and services (Herrero-Olarte 2019), discourages the efforts to reduce conditions of wage inequality, beyond altruism.
Labor income decrease has also been related to the labor institucional reinforcement. In the twenty-first century, South American people claimed for a political change. They were weary of the neo-liberal Washington Consensus policies that have achieved more than questionable results in improving their quality of life (Easterly Citation2005; Rodrik Citation2005). Consequently, the progressive parties arrived in most South American governmentsFootnote2 offering to put in the center of the public policy the citizen needs (Serbin, Martínez, and Ramanzini Citation2012). In this context, the economic growth provided the necessary environment to sign into a law the new labor rules proposed by this new governments, with the widest possible consensus (Uriarte Citation2007). Most of the reforms helped to improve labor conditions, pendents from decades ago, but other were highly populists (ECLAC 2018). As a consequence, the LMRIFootnote3 passed from 4.7 in 2002 to 5.2 in 2011, but it was still below the global average, of 6.5 in 2011 (Fraser Institute Citation2019).
In practice, the impact of institutional changes on labor income inequality was modest. Alves et al. (Citation2012) in Uruguay, Ferreira, Firpo, and Messina (Citation2017) in Brazil and Maloney and Nuñez (Citation2004) in Colombia found evidence of the positive impact of rising minimum wages on inequality. The conclussion was the same, considering the collective bargaining reinforcement in Argentina (Casanova and Alejo Citation2015); the tightening of the application of labor legislation in Ecuador (Guzmán Citation2018); or the improvement of social security coverage in Latin America (Bertranou and Maurizio Citation2011; Keifman and Maurizio Citation2012). In all the cases there was an overall possitive relationship with the decrease in inequality, but nowadays it appears to be unremarkable.
The relationship between institutional changes and labor inequality is conditioned by the nature of the reforms developed and the remaining structural challenges of the region. In the labor market, at least a half of each decile worked informally, but in deciles 1 and 2, informality reached 88.8% of the workers in 2015 (IDB Citation2019). Informal workers are poorly affected by the labor legislation (Belman and Wolfson Citation2016) and consequently, their wages are marginally impacted by institutional changes. Indeed, the empirical analysis carried out in Argentina (Arcidiácono Citation2015), Chile (Grau, Miranda, and Puentes Citation2018) and Colombia (Maloney and Nuñez Citation2004) found a limited relationship betweeen changes in minimum wages and the wages of informal workers. Thus, there is evidence supporting that institutions do not play a role on inequality.
On the other hand, Amarante (Citation2016) found in an study for Latin America that formal wages and employment contributed to the increase in inequality. Aditionally, the impact of the institutional changes on labor inequality is also conditioned by the ineficiency of the state apparatus (Izquierdo, Loo-Kung, and Rojas-Suárez Citation2013), still conditioned by high levels of corruption (OECD et al. Citation2016). In contrast, some studies have found that, due to policies that attempted to increase labor formality, this labor formalization had an equalizing effect on income redistribution for Brazil and Argentina (Maurizio Citation2015). In addition, Keifman and Maurizio (Citation2012) suggested that the most important factor for reducing inequality is the change in labor income, but formalization in labor markets contributed to equality in Argentina, Brazil, Chile, Mexico and Uruguay in the 2000s.
Another possible explanation for this significant reduction in inequality in the region can be attributed to public spending. Specifically, social spending and monetary transfers. According to Clifton, Díaz-Fuentes, and Revuelta (Citation2020), a new policy and institutional cycle in Latin America, accompanied by the commodity boom that surged at the beginning of the 2000s, played a crucial role on reducing income inequality. They also affirm that, eventhough the effect of social spending in Latin America was small compared to the commodities boom and the institutional framework, it was also important on tackling inequality in the region. A similar result is presented by Tsounta and Osueke (Citation2014), Sánchez Ancochea (Citation2019) and Lustig, Pessino, and Scott (Citation2014), who determined that a higher spending on education and higher tax revenues had an important impact on reducing income inequality. Effectively, higher tax revenues derived from the commodities boom and new tax structures increased social spending in several countries benefited by high commodity prices. Finally, they highlight the significance that cash transfers to the poor had in many countries in the Latin American region, specifically cash transfers focused on education and health.
Beyond the impact of the macroeconomic changes, labor inequality is also related to structural changes in labor force characteristics. The main changes were increased participation of traditionally excluded groups and its improved capacity. Expanding social and labor market incorporation is extremely important for people’s wellbeing, specially for low-income groups that tend to be left out of social interactions and labor activities (Martínez Franzoni and Sánchez-Ancochea Citation2014). Certainly, the new policies on cash transfers and labor inclusion adapted in Latin America during the 2000s helped with the integration of marginalized parts of societies through access to educational systems.
Attending to the reduction of gender, race and spatial participation gaps, Ferreira, Firpo, and Messina (Citation2017) related them to the decline in earnings inequality during the growth period. In Bolivia, Canavire-Bacarreza and Rios-Avila (Citation2017) found similar results. In Latin America, Rodríguez-Castelán et al. (Citation2016) offered a regional vision, analyzing the context and founding similar results. In all cases, the relationship was proved but moderate, mainly because of the remaining challenges.
Work capacity is mainly conditioned by education. According to its evolution, the average percentage of the South American population that has completed primary school increased from 75.8% in 2002 to 83.5% in 2011 (IDB Citation2019). The percentage of the South American population between 18 and 64 years old with at least secondary education increased from 38.1% in 2002 to 49.5% in 2011 (IDB Citation2019). The average number of years of schooling in Latin America rose from three years in 1960, to five in 1980 and nine in 2010. Nowadays, it is still below US data (12 years) (Barro and Lee Citation2013).
Beccaria, Maurizio, and Vázquez (Citation2015) found evidence of that in Argentina, Parro and Reyes (Citation2017) and Rodríguez-Castelán et al. (Citation2016) in Chile and to Wang, Lustig, and Bartalotti (Citation2016) and Jaume (Citation2018) in Brazil. The reward for improved primary school coverage wourld be greater than improvements in tertiary education. Consequently, the skill premium, that is, the gap between low and high skills wages, should follow an inverted U-shape. We analyze the trend of the skill premium in Appendix 1. We applied a Mincer equation in South American countries in the period 2005–2015 using the (CMAEH) database provided by the IDB (Citation2019). An increasing average trend can be observed in each South American country in the return perceived by unskilled workers in relation to skilled workers. Therefore, we assume a downward trend in the skill premium.
Several studies support the idea that the reduction of inequality can be explained by the reduction of the skill premium. The research by Fernández and Messina (Citation2018) presents a detailed analysis of Argentina, Chile and Brazil with regard to factors affecting income inequality. The study suggests that the skill premium (experience, schooling) plays a predominant role, because the decrease in inequality at the upper-tail can be explained by a reduction in the experience premium, i.e. the reduction of the premium is stronger in people with a higher academic level; while the education premium has a greater effect below the average for primary and secondary education. According to Azevedo et al. (Citation2013), the reduction in inequality is related to wages, since, according to their research, 64% of the variation in the Gini coefficient was attributed to the reduction in the skill premium.
Behind the relationship between skill premium and inequality variations is the human capital theory, that assumes higher productivity when education is improved (Schultz Citation1961; Becker Citation1993). Since the wage is the payment for the work done, the capacity of workers to contribute to productivity is a wage variable. If low-skilled workers increased their contribution to productivity more than the high-skilled workers, they would be better paid. Increased productivity would translate in higher incomes for low-skilled workers because they would contribute more to it, reducing the skill premium. We analyze the contribution of different skills to productivity in Appendix 2. We applied a Panel Fixed Effect (FE) model to nine South American countries in the period 2000–2016 to determine the relationship between the wages of unskilled and skilled workers and productivity. The productivity coefficient is higher when we applied the model considering low-skilled wages as the independent variable. Therefore, we assume that low-skilled workers could be improving their contribution to productivity more than the high-skilled workers.
Attending to the link between skills and productivity, workers’ capacity is not just related to the educational coverage.Footnote4 Which are the variables related to the workers’ capacity, beyond their education, that impact on their productivity and, consequently, on labor inequality?
Workers’ capacity to contribute to productivity depends primarily on academic knowledge and soft abilities (Cunha and Heckman Citation2007). They condition each other and, at the same time, are determined by their lifestyle (Fiszbein, Cosentino, and Cumsille Citation2016). Experience could be another variable to take into account, if it had not been for the reduction of the experience premium across all age groups in Latin American countries in the 2000s (Rodríguez-Castelán et al. Citation2016).
Academic knowledges are mainly literacy and simple math skills. They are directly related to school attendance, traditionally considered in the analysis of skill inequality, and the quality of education. The quality of education is the big challenge. Half of the children under 15 years of age enrolled in school do not acquire a basic level of proficiency in reading, mathematics and science, according to the Programme for International Student Assessment (PISA) results. Less than 1% perform among the highest levels of proficiency in mathematics, reading or science. In contrast, 12% of students in OECD countries perform at the two highest levels in mathematics, and 8.5% reach these levels in reading and science. The 81% and 75% of Latin American students achieved the lowest possible level in mathematics and science, respectively, almost doubling the rates of OECD countries (OECD Citation2018). In recent years, an improvement in reading and mathematics has been observed (UNESCO Citation2017). The quality of education depends on a group of structural variables, caregiver programs and processes (Naudeau et al. Citation2011) highly codependent between them, that are being recently widely explored with the aim of improve the children capacity after their first years of education.
Soft skills are cognitive, like learning ability, critical thinking, problem solving, communication skills, teamwork or the capability to prioritize tasks; and socio-emotional, suchs as compassion, positive attitude and values, among others (National Association of College and Educators Citation2015). Despite their importance in development, they have been poorly measured in the region. A person’s soft skills depend, among other variables, on the improvement of quantity and quality of the experiences that he or she could have to generate new skills in the present and reinforce the possibility of acummulating more competencies in the future (Alfes et al. Citation2010). In this context, the Internet has become the biggest tool for multiplying and diversifying people’s experiences and turning them into new knowledges, albeit through a screen. As a result, there is a positive relationship between the access to the Internet and the cognitive and socio-emotional skills, widely explored by Calvert et al. (Citation2005), Cranmer (Citation2006) and Jackson et al. (Citation2006).
As shown by literature, there is a positive relationship between Information and Communication Technologies (ICT) in general, and internet access in particular, with productivity (Bertscheck Citation2012; Draca, Rafaella, and John Citation2007). Internet access of the lowest deciles reduces their productivity gap with higher-educated workers, as proposed by Gasparini et al. (Citation2011) for Latin America in 1990–2010. Consequently, inequality would be reduced (Robinson et al. Citation2015). Navarro (Citation2010) found that in six Latin American countries, people who use internet in the workplace and at home have better wages, also if they are self-employed workers. The difference was higher than in industrialized countries. Available national studies show the same result. In Ecuador, the increase in broadband availability is related with a 7.5% increase in individual labor income over the next two years (Katz and Callorda Citation2013). To De Los Ríos (Citation2010), those who became Internet users in Peru from 2007 to 2009 increased their salaries more than the non-users. This patterns is also observed in the rural area of the country (Atasoy Citation2013).
Considering the influence of living conditions, both academic and soft skills have some structural constrains for improvement related to the multidimensional poverty (van Ijzendoorn and Juffer Citation2006). Hill and Jodi (Citation1995) presented a conceptual model with which they explained the negative consequences of poverty on children in the short and in the long run. In the former, poverty could reduce academic achievement of children and make them more likely to drop school. In the latter, future labor productivity may be conditioned by the restricted education received in their childhood. Specifically, Schady et al. (Citation2014) found evidence that wealthier children perform better than their poorer peers; and conditioned nutrition affected the human capacities to improve their knowledge (WHO Citation1998; Vogl Citation2014; Lundborg, Nystedt, and Rooth Citation2014). Furthermore, illiteracy rates play a key role in worsening income disparities and life expectancy, infering that living conditions affect not only the productive capacity of individuals, but also their wellbeing and health (Messias Citation2003).
3. Data sets and the methodological approach
In order to test the hypothesis that a greater increase in the productive capacity of less qualified workers in relation to the productive capacity of qualified workers reduced income inequality, we took into consideration the skill premium. For this, we estimated the population structure according to their educational level and subsequently the average labor income of these groups. For this purpose, the methodology applied by the CEDLAS based on the SEDLAC database (2019) was taken into consideration. Skill premium is defined as the ratio between the labor income of highly educated workers in relation to the labor income of workers with lower educational level of the labor force. We defined people with high education (skilled workers) as those who report more than 13 years of schooling; while people with low education (unskilled workers) are those who have between 0 and 8 years of schooling. Due to the theoretical approach and empirical evidence, the trend of this ratio is expected to decrease over time.
The main explanatory variable is an index of own construction, the Productive Capacity Index (PCI) which takes into consideration a weighted average of the percentage of people belonging to the labor force with secondary education, the percentage of people with internet accessFootnote5,Footnote6 and the additive inverse of the Multidimensional Poverty Index (MPI). The initial weight assigned to each variable that composes it was 1/3. However, for robustness checks we re-calculated this index using different weights combinations. We are aware of the limitations that this index may present. First, it does not come from an official source and has not been used in previous studies. Nevertheless, considering the limitation of information of this type for Latin America, we considered that it can be a good proxy to measure productive capacity, because it takes into consideration the three (3) dimensions of productive capacity set out in the literature. The construction of this indicator together with its components was carried out by quintile of per capita income per household. The data source for both the generation of the dependent and explanatory variable is the CMAEH database by IDB (Citation2019). The PCI is therefore defined in Equation (1).
(1)
(1) where
is the percentage of the labor force with at least secondary education,
is the percentage of the population with internet access, and
is the quintile. The reason for considering the
variable is that the
attempts to capture multiple deprivations both at the household level and individually in health, education and living standards through 10 indicators related to these fields. Therefore,
determines the percentage of households that do not have any deprivation in these fields, which influences the degree of their productivity.
To estimate the model, we considered the ratio between the average PCI of quintiles 1 and 2 and the PCI of quintile 5 (Q1Q2/Q5 PCI ratio). This is in order to capture the relative development of this index with respect to these income quintiles. We use this quintiles in order to consider the difference between the two lower income quintiles and the top one, and have a proxy of income inequality (Chao et al. Citation2018). As mentioned above, the dependent variable is the skill premium between skilled and unskilled workers. Therefore, it is expected that an increase in the Q1Q2/Q5 PCI ratio is associated to a negative coefficient. The justification for this is that if the Q1Q2/Q5 PCI ratio increases, this means that there was an increase in the PCI of the quintiles (1 and 2) in relation to the PCI of quintile 5. In other words, this increase translates into an increase in the productive capacity of the lower quintiles relative to the higher quintile, so the skill premium is expected to decrease as there is a proportionally higher increase in the wages of the lower unskilled workers than the skilled workers. As the relationship is inverse, then a negative coefficient is expected. In , we can observe that this variable has tended to increase in the South American region, which implies that, although there has been an increase in the productivity capacity of all quintiles, the increase in this capacity has been of greater magnitude in the case of the lower quintiles.
Figure 1. Evolution of the PCI ratio between the average of Q1 and Q2 with respect to Q5 (Q1Q2/Q5 PCI ratio). Source: Authors’ elaboration.
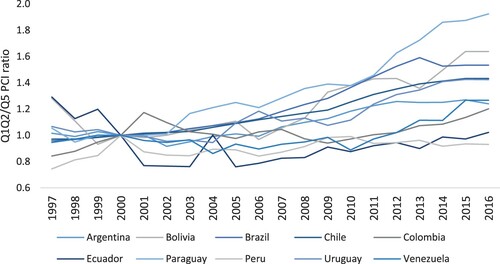
In addition to this explanatory variable, as controls related to economic growth we used the internal demand growth and the terms of trade. We also included the LMRI as a proxy variable for the institutional reinforcement of the labor market. Finally, we included a variable that captures the social spending as a percentage of GDP. These control variables have been used on the basis of what was explained in the theoretical section, which showed the different factors that can explain the reduction of inequality in the region. Data for these control variables come from the World Bank (Citation2019) and the Fraser Institute (Citation2019).
The estimation was made using a panel data model. The dependent variable is the log of the ratio of the labor income of the qualified workers in relation to the unqualified workers of the labor force. As we considered that there exist some time-invariant unobservable characteristics of each of the countries incorporated in the study that can be related to other variables included in the model, we prefered to estimate the model using the Fixed Effects Estimator (FE), this estimation was carried out with and without clustered standard errors. To correct for heteroskedasticity problems and the presence of panel correlation, as well as serial autocorrelation, estimations were made using panel-corrected standard errors (PCSE) and Driscoll-Kraay standard errors (SCC). Moreover, to account for dynamic effects and endogeneity in some of the variables included, we estimated the model using the Arellano and Bond estimator. Thus, the estimated equation is:
(2)
(2) where
represents the log of the ratio between skilled and unskilled wages of country
in year t, TOT is the terms of trade, and
is the error term.
The results for these estimations are in the following section. For these estimations, the variables skill premium, internal demand growth and the LMRI are stationary at level, while the Q1Q2/Q5 PCI ratio, the terms of trade and social spending, are stationary in first difference.
4. Results and discussion
The results of the estimations, using a data panel structure, are shown in .
Table 1. Results of the regressions of Equation (2).
As can be seen, the R2, is between 0.30 and 0.40. The coefficient of the main explanatory variable (Q1Q2/Q5 PCI ratio) is significant in all estimations. Similarly, it can be observed that it has the expected sign. This results can be seen as an evidence that the increase in the ratio generates a negative change in the skill premium, implying that the productive capacity of the low quintiles increased in greater proportion in relation to the highest quintile. Due the sign in the coefficient, there is an inverse relationship between the evolution of the PCI and the skill premium. Therefore, a 1% increase in the PCI of the lower quintiles (Q1 and Q2) in relation to the highest quintile implies a negative variation in the skill premium of between 0.54% and 0.95%. This implies, thus, that the productive capacity of the lowest quintiles generated a larger increase in the wages of least qualified workers in relation to those of the most qualified.
With regard to the other control variables, we can see that the internal demand is negatively correlated with the skill premium, which means that if there is an increase in the internal demand, skill premium will decrease. This an expected result as internal demand is a variable related to economic growth, and, as it was stated in the theoretical section, economic growth was negatively associated with income inequality; however, its magnitude is small (Adão Citation2015). A similar pattern is shown by the Terms of Trade, the same that was also included as a control related to economic growth, nonetheless, it is not significant, with the exception of the estimation by fixed effects with clustered standar errors. With regard to the LMRI, we can see that it also has a negative effect and, in general, a significant one on the evolution of the skill premium. Thus, results suggest that a 1% increase in this index generates a reduction in the skill premium of between 0.18% and 0.33%, except for the estimations with PCSE and SSC, which reflects the impact of labor market regulation on the ratio of salaries of skilled and unskilled workers. As stated in the literature, labor market policies such as the ones related to minimum wage, can reduce wage inequality (Casanova and Alejo Citation2015; Guzmán Citation2018); this is the reason why a negative coeffiencient associated with LMRI was expected. Finally, social expenditure has a negative coefficient associated, however, contrary to the claimed by the literature (Clifton, Díaz-Fuentes, and Revuelta Citation2020), it is not statistically significant (with exception for the first specification).
Our preferred specification is the one in which we used the Arellano and Bond estimator (GMM) because it admits to consider both, dynamic effects and endogeneity in the variables, which allows to have a more accurate effect. It is worth mentioning that in all the GMM estimations, a Sargan Test was performed to check the validity of the instruments included.
As mentioned above, for robustness checks, we re-calculated the PCI index in two ways: (1) we changed the weights of the components of the index, in particular, we gave a higher weight (50%) to the variable related to education, and the remaining 50% was distributed equally between the two remaining variables; (2) we calculated the index using a Principal Component Analysis (PCA). Results are shown in Appendix 3. In general, the direction, magnitude and significance of the variable of interest were maintained in these two estimates, which helps us to support the results obtained, and are evidence of the hypothesis stated.
5. Conclusion
The remarkable reduction in inequality experienced by Latin America during the period 2002–2011 has been widely studied in the literature. Several hypotheses have been put forward about what may be the causes that led to this significant reduction in one of the regions that has historically been one of the most unequal.
Because of the period in which this reduction occurred, it was not difficult to relate this decline to the economic growth of the countries in the region, which occurred, in large part, because of the commodities boom. Also, this period was characterized by a series of institutional reforms, especially in the labor market, which, as has been shown, could help to explain the reduction in income inequality in the region. Furthermore, there was a strong fiscal policy in the region with redistributive purposes, and, along with this, greater spending on education, which allowed for greater coverage during this period, compared to previous years.
However, these are not the only factors that explain this reduction in income inequality. For this reason, this paper explores the impact of an improvement in the productive capacity of those workers with less education, compared to workers with more years of education, in reducing the skill premium. Our index of productive capacity is a function of key aspects raised by the literature, such as coverage of education, access to internet, and living conditions. The results suggest that the productive capacity of the less educated is positively and significantly related to the reduction of inequality in South America in 2002–2011.
In terms of public policy, these results support the importance of investment in people's education, as well as global and widespread access to the internet for the entire population. In terms of living conditions, policies are needed to help families reduce the deprivations they currently experience, as this may condition their present and future outcomes. Indeed, the results show that the productive capacity of the less educated can be improved by decision makers to reduce inequality more easily than economic growth or institutional reinforcement.
Authors’ contributions
SHO contributed to the conceptualization, resources, writing, review, editing, project administration and supervision. FV contributed to the methodology, software, formal analysis and writing, review and editing.
Availability of data and materials
The data set analyzed in this study is drawn from public domain data of the World Bank, the Inter-American Development Bank, the International Labour Organization, the Center for Distributive, Labour and Social Studies (CEDLAS), the Fraser Institute and the International Telecommunication Union. Analysis code is available from the authors on request.
List of abbreviations | ||
PCI | = | Productive Capacity Index |
LMRI | = | Labor Market Regulation Index |
CEDLAS | = | Center for Distributive, Labor and Social Studies |
SEDLAC | = | Socio-Economic Database for Latin America and the Caribbean database |
CMAEH | = | Harmonized Household Surveys of Latin America and the Caribbean |
IDB | = | Inter-American Development Bank |
ITC | = | Information and Communication Technology |
PISA | = | Programme for International Student Assessment |
MPI | = | Multidimensional Poverty Index |
Q1Q2/Q5 PCI ratio | = | Average PCI of quintiles 1 and 2 and the PCI of quintile 5 |
FE | = | Fixed effects |
PCSE | = | Panel-corrected standard errors |
SCC | = | Driscoll-Kraay standard errors |
PPP | = | Purchasing Power Parity |
Disclosure statement
No potential conflict of interest was reported by the author(s).
Notes
1 The countries considered in this paper are Argentina, Bolivia, Brazil, Chile, Colombia, Ecuador, Peru, Paraguay, Uruguay and Venezuela.
2 In Argentina, Kirchner came to government in 2003; in Brazil, Da Silva in 2003; in Bolivia, Morales in 2006; in Chile, Bachelet in 2006; in Ecuador, Correa in 2006; in Uruguay, Vázquez in 2004; and in Venezuela, Chávez in 1999.
3 The LMRI includes the (1) hiring regulations and minimum wage; the (2) mandated cost of worker dismissal; the (3) hiring and firing regulations; the (4) centralized collective bargaining hour’s regulations, and the (5) conscription.
4 Indeed, Rodríguez-Castelán et al. (Citation2016) applied a Mincer equation controlled by educational levels that shows an R-squared of less than 0.3 between skill premium and labor income inequality in nine South American countries in the period 1993–2013.
5 The variable of the percentage of the population with Internet access by quintile could not be estimated for all years due to the nature of household surveys. For the years in which this variable was not available, the information was completed using data from the International Telecommunication Union. However, these years do not consider the information disaggregated by quintiles.
6 Wages are expressed in real terms, based on the PPP conversion factor. The dependent variables are unskilled and skilled wages. The independent variables are the variation of labor productivity, production, unemployment, minimum wage, and inflation. To interpret the elasticity, we took the natural logarithm of the variables, with the exception of the unemployment rate and inflation due to the nature of the data. Due to the lagged impact that some independent variables have on the dependent variable, we considered lags in some explanatory variables.
References
- Adão, Rodrigo. 2015. Worker Heterogeneity, Wage Inequality, and International Trade: Theory and Evidence from Brazil. Job Market Paper. Massachusetts Institute of Technology.
- Adão, Rodrigo, Costas Arkolakis, and Federico Esposito. 2019. “Spatial Linkages, Global Shocks, and Local Labor Markets: Theory and Evidence.” NBER Working Paper No. 25544.
- Alfes, Kerstin, Catherine Truss, Emma Soane, Chris Rees, and Mark Gatenby. 2010. Creating an Engaged Workforce: Findings from the Kingston Employee Engagement Consortium Project. London: Study of Chartered Institute of Personnel and Development.
- Alves, Guillermo, Verónica Amarante, Gonzalo Salas, and Andrea Vigorito. 2012. “La desigualdad del ingreso en Uruguay entre 1986 y 2009.” Instituto de Economía (IECON) Documentos de Trabajo No. 12-03.
- Amarante, Verónica. 2016. “Income Inequality in Latin America: A Factor Component Analysis.” Review of Income and Wealth 62: S4–S21.
- Amarante, Verónica, and Maira Colacce. 2018. “More Unequal or Less? A Review of Global, Regional and National Income Inequality.” CEPAL Review 124 (1): 7–32.
- Arcidiácono, Malena. 2015. “Salario Mínimo y Distribución salarial: Evidencia para Argentina 2003.” CEDLAS Working Papers No. 0192.
- Atasoy, Hilal. 2013. “The Effects of Broadband Internet Expansion on Labor Market Outcomes.” ILR Review 66 (2): 315–345.
- Azevedo, João, Maria Dávalos, Carolina Diaz-Bonilla, Bernardo Atuesta, and Raul Castañeda. 2013. “Fifteen Years of Inequality in Latin America: How Have Labor Markets Helped?” World Bank Policy Research Working Paper No. 6384.
- Barro, Robert, and Jong-Wha Lee. 2013. “A New Data Set of Educational Attainment in the World, 1950–2010.” Journal of Development Economics 104: 184–198. doi:https://doi.org/10.1016/j.jdeveco.2012.10.001.
- Barros, Ricardo, Mirela de Carvalho, Samuel Franco, and Rosane Mendonça. 2006. “Uma Análise das Principais Causas da Queda Recente na Desigualdade de Renda Brasileira.” Instituto de Pesquisa Econômica Aplicada – IPEA Discussion Papers No. 1203.
- Beccaria, Luis, Roxana Maurizio, and Gustavo Vázquez. 2015. “Recent Decline in Wage Inequality and Formalization of the Labour Market in Argentina.” International Review of Applied Economics 29 (5): 677–700. doi:https://doi.org/10.1080/02692171.2015.1054369.
- Becker, Gary. 1993. Human Capital: A Theoretical and Empirical Analysis with Special Reference to Education. Chicago (in press).
- Belman, Dale, and Paul Wolfson. 2016. “What Does the Minimum Wage Do in Developing Countries? A Review of Studies and Methodologies.” ILO Working Papers: Conditions of Work and Employment Series No. 62.
- Benguria, Felipe, Felipe Saffie, and Sergio Urzúa. 2018. “The Transmission of Commodity Price Super-Cycles.” NBER Working Paper No. 24560.
- Bérgolo, Marcelo, and Guillermo Cruces. 2011. “Labor Informality and the Incentive Effects of Social Security: Evidence from a Health Reform in Uruguay.” IDB Working Paper No. 3829.
- Bertranou, Fabio, and Roxana Maurizio, eds. 2011. Trabajadores independientes, mercado laboral e informalidad en Argentina. Buenos Aires.
- Bertscheck, Irene. 2012. “ICT, Internet and Worker Productivity.” In The New Palgrave Dictionary of Economics, edited by Palgrave Macmillan. London: Palgrave Macmillan. doi:https://doi.org/10.1057/978-1-349-95121-5_2941-1
- Calvert, Sandra, Victoria Rideout, Jennifer Woolard, Rachel Barr, and Gabrielle Strouse. 2005. “Age, Ethnicity, and Socioeconomic Patterns in Early Computer Use: A National Survey.” American Behavioral Scientist 48 (5): 590–607. doi:https://doi.org/10.1177/0002764204271508.
- Canavire-Bacarreza, Gustavo, and Fernando Rios-Avila. 2017. “On the Determinants of Changes in Wage Inequality in Urban Bolivia.” Journal of Human Development and Capabilities 18 (4): 464–496. doi:https://doi.org/10.1080/19452829.2017.1353350.
- Casanova, Luis, and Javier Alejo. 2015. “El efecto de la negociación colectiva sobre la distribución de los ingresos laborales: Evidencia empírica para Argentina en los años dos mil.” ILO Documento de trabajo No. 8.
- CEDLAS. 2019. Socio-Economic Database for Latin American and the Caribbean, SEDLAC (Center for Distributive, Labor and Social Studies, CEDLAS, and The World Bank). Database.
- Chao, Fengqing, Danzhen You, Jon Pedersen, Lucia Hug, and Leontine Alkema. 2018. “National and Regional Under-5 Mortality Rate by Economic Status for Low-Income and Middle-Income Countries: A Systematic Assessment.” The Lancet Global Health 6 (5): e535–e547.
- Clifton, J., D. Díaz-Fuentes, and J. Revuelta. 2020. “Falling Inequality in Latin America: The Role of Fiscal Policy.” Journal of Latin American Studies 52 (2): 317–341.
- Cord, Louise, Oscar Barriga-Cabanillas, Leonardo Lucchetti, Carlos Rodríguez-Castelán, Liliana Sousa, and Daniel Valderrama. 2017. “Inequality Stagnation in Latin America in the Aftermath of the Global Financial Crisis.” Review of Development Economics 21 (1): 157–181. doi:https://doi.org/10.1111/rode.12260.
- Cranmer, Sue. 2006. “Children and Young People’s Uses of the Internet for Homework.” Learning, Media and Technology 31 (3): 301–315. doi:https://doi.org/10.1080/17439880600893358.
- Cunha, Flavio, and James Heckman. 2007. “Identifying and Estimating the Distributions of Ex Post and Ex Ante Returns to Schooling.” Labour Economics 14 (6): 870–893. doi:https://doi.org/10.1016/j.labeco.2007.06.002.
- De la Torre, Augusto, Alain Ize, Guillermo Beylis, and Daniel Lederman. 2015. Jobs, Wages, and the Latin American Slowdown: Main Report. Washington, DC: Study on behalf of World Bank.
- De Los Ríos, Carlos. 2010. Impacto del uso de Internet en el Bienestar de los Hogares Peruanos: Evidencia de un panel de hogares 2007–2009. DIRSI. Accessed March 18, 2020. https://www.dirsi.net/sites/default/files/DIRSI-Impacto%20del%20Uso%20de%20Internet%20en%20el%20Bienestar%20de%20los%20Hogares%20Peruanos.pdf.
- Draca, Mirko, Sadun Rafaella, and Van Reenen John. 2007. “Productivity and ICTs: A Review of Evidence.” In The Oxford Handbook of Information and Communication Technologies, edited by R. Mansell, C. Avgerou, D. Quah, and R. Silverstone, 100–147. New York: Oxford University Press.
- Easterly, William. 2005. “National Policies and Economic Growth: A Reappraisal.” In Handbook of Economic Growth, edited by Philippe Aghion and Steven Durlauf, 1015–1059. Amsterdam.
- Fernández, Manuel, and Julián Messina. 2018. “Skill Premium, Labor Supply, and Changes in the Structure of Wages in Latin America.” Journal of Development Economics 135: 555–573. doi:https://doi.org/10.1016/j.jdeveco.2018.08.012.
- Ferreira, Francisco, Sergio Firpo, and Julián Messina. 2017. “Ageing Poorly? Accounting for the Decline in Earnings Inequality in Brazil, 1995–2012.” World Bank Policy Research Working Paper No. 8018.
- Fiszbein, Ariel, Clemencia Cosentino, and Belén Cumsille. 2016. The Skills Development Challenge in Latin America: Diagnosing the Problems and Identifying Public Policy Solutions. Washington, DC: Study on behalf of Inter-American Dialogue and Mathematica Policy Research.
- Fraser Institute. 2019. Economic Freedom. Database.
- Galbraith, James. 2016. “Cause: of Changing Inequality in the World.” Intereconomics 51: 55–60. doi:https://doi.org/10.1007/s10272-016-0577-z.
- Gasparini, Leoneardo, Sebastian Galiani, Guillermo Cruces, and Pablo Acosta. 2011. “Educational Upgrading and Returns to Skills in Latin America: Evidence from a Supply-Demand Framework, 1990–2010.” World Bank Policy Research Working Paper No. 5921.
- Gasparini, Leonardo, and Nora Lustig. 2011. “The Rise and Fall of Income Inequality in Latin America.” Documento de Trabajo No. 118, Universidad de La Plata, Centro de Estudios Distributivos, Laborales y Sociales (CEDLAS).
- Grau, Nicolás, Jorge Miranda, and Esteban Puentes. 2018. “The Effects of the Minimum Wage on Employment and Wages.” University of Chile, Department of Economics Working Paper No. 466.
- Guzmán, Wilson. 2018. “The Impact of the National Minimum Wage Policy on Wage Inequality in Ecuador.” Paper presented at the VI International Congress on Economics, Universidad San Francisco de Quito, Quito, 2018.
- Hartmann, Dominik, Miguel Guevara, Cristian Jara-Figueroa, Manuel Aristarán, and César Hidalgo. 2017. “Linking Economic Complexity, Institutions and Income Inequality.” World Development 93: 75–93. doi:https://doi.org/10.1016/j.worlddev.2016.12.020.
- Helfand, Steven, Rudi Rocha, and Henrique Vinhais. 2009. “Pobreza e Desigualdade de Renda no Brasil Rural: Uma Análise da Queda Recente.” Pesquisa e planejamento econômico 39 (1): 59–80.
- Herrero-Olarte, S. 2019. “La influencia de la productividad y de los factores de producción en las exportaciones de manufacturas sudamericanas.” Regional and Sectoral Economic Studies 19 (2): 79–98.
- Hill, Martha, and Sandfort Jodi. 1995. “Effects of Childhood Poverty on Productivity Later in Life: Implications for Public Policy.” Children and Youth Services Review 17 (1-2): 91–126. doi:https://doi.org/10.1016/0190-7409(95)00005-w.
- Inter-American Development Bank. 2019. Harmonized Household Surveys of Latin America and the Caribbean (CMAEH). Database.
- International Monetary Fund. 2019. Primary Commodity Price System. Database.
- Izquierdo, Alejandro, Rudy Loo-Kung, and Liliana Rojas-Suárez. 2013. “Macroprudential Regulations in Central America.” IDB Working Paper No. 392.
- Jackson, Linda, Alexander von Eye, Frank Biocca, Gretchen Barbatsis, Yong Zhao, and Hiram Fitzgerald. 2006. “Does Home Internet Use Influence the Academic Performance of Low-Income Children?” Developmental Psychology 42 (3): 429–435. doi:https://doi.org/10.1037/0012-1649.42.3.429.
- Jaume, David. 2018. “The Labor Market Effects of an Educational Expansion: A Theoretical Model with Applications to Brazil.” CEDLAS Documento de Trabajo, No. 220.
- Katz, Raúl, and Fernando Callorda. 2013. El impacto del despliegue de la banda ancha en Ecuador. DIRSI. Accessed March 18, 2020. https://dirsi.net/web/web/es/publicaciones/detalle/impacto-del-despliegue-de-la-banda-ancha-en-el-ecuador.
- Keifman, Saúl, and Roxana Maurizio. 2012. “Changes in Labour Market Conditions and Policies.” Their impact on Wage Inequality During the Last Decade. UNU-WIDER Working Paper 2012/14.
- Lerman, Robert, and Shlomo Yitzhaki. 1985. “Income Inequality Effects by Income Source: A New Approach and Applications to the United States.” Review of Economics and Statistics 67 (1): 151–156. doi:https://doi.org/10.2307/1928447.
- Lundborg, Petter, Paul Nystedt, and Dan-Olof Rooth. 2014. “Height and Earnings: The Role of Cognitive and Noncognitive Skills.” Journal of Human Resources 49 (1): 141–166.
- Lustig, N. 2020. Inequality and Social Policy in Latin America (No. 2011).
- Lustig, Nora, Luis Lopez, and Eduardo Ortiz. 2013. “Deconstructing the Decline in Inequality in Latin America.” World Bank Policy Research Working Paper 6552.
- Lustig, Nora, Carola Pessino, and John Scott. 2014. “The Impact of Taxes and Social Spending on Inequality and Poverty in Argentina, Bolivia, Brazil, Mexico.” Peru, and Uruguay: Introduction to the Special Issue. Public Finance Review 42 (3): 287–303.
- Maloney, William, and Jairo Nuñez. 2004. “Measuring the Impact of Minimum Wages. Evidence from Latin America.” In Law and Employment: Lessons from Latin America and the Caribbean, edited by James Heckman and Carmen Pagés, 109–130. Cambridge, MA.
- Martínez Franzoni, Juliana, and Diego Sánchez-Ancochea. 2014. “The Double Challenge of Market and Social Incorporation: Progress and Bottlenecks in Latin America.” Development Policy Review 32 (3): 275–298.
- Maurizio, Rozana. 2015. “Transitions to Formality and Declining Inequality: Argentina and Brazil in the 2000s.” Development and Change 46 (5): 1047–1079.
- Messias, Erick. 2003. “Income Inequality, Illiteracy Rate, and Life Expectancy in Brazil.” American Journal of Public Health 93 (8): 1294–1296.
- Messina, Julián, and Joana Silva. 2017. “The Role of Labor Demand Conditions in Wage Inequality Trends.” In Wage Inequality in Latin America: Understanding the Past to Prepare for the Future, edited by Julián Messina and Joana Silva, 117–153. Washington, DC.
- Nafukho, Fredrick Muyia, Nancy Hairston, and Kit Brooks. 2004. “Human Capital Theory: Implications for Human Resource Development.” Human Resource Development International 7 (4): 545–551. doi:https://doi.org/10.1080/1367886042000299843.
- National Association of College and Educators. 2015. Job Outlook 2016: Attributes Employers Want to See on New College Graduates’ Resumes, in: National Association of College and Educators. Accessed March 18, 2020. https://www.naceweb.org/career-development/trends-and-predictions/job-outlook-2016-attributes-employers-want-to-see-on-new-college-graduates-resumes/.
- Naudeau, Sophie, Naoko Kataoka, Alexandria Valerio, Michelle Neuman, and Leslie Kennedy. 2011. Investing in Young Children: An Early Childhood Development Guide for Policy Dialogue and Project Preparation. Washington, DC (in press).
- Navarro, Lucas. 2010. “The Impact of Internet Use on Individual Earnings in Latin America.” INESAD Development Research Working Paper Series No. 11/2010.
- OECD, CAF, ECLAC. 2018. Latin American Economic Outlook 2018: Rethinking Institutions for Development. Paris (in press).
- Organisation for Economic Co-operation and Development. 2016. Skills in Ibero-America: Insights from PISA 2012. OECD. Accessed March 18, 2020. https://www.oecd.org/latin-america/Skills-IberoAmerica.pdf.
- Parro, Francisco, and Loreto Reyes. 2017. “The Rise and Fall of Income Inequality in Chile.” Latin American Economic Review 26 (3), doi:https://doi.org/10.1007/s40503-017-0040-y.
- Robinson, Laura, Shelia Cotten, Hiroshi Ono, Anabel Quan-Haase, Gustavo Mesch, Wenhong Chen, Jeremy Schulz, Timothy Hale, and Michael Stern. 2015. “Digital Inequalities and Why They Matter. Information.” Communication & Society 18 (5): 569–582. doi:https://doi.org/10.1080/1369118X.2015.1012532.
- Rodríguez-Castelán, Carlos, Luis López-Calva, Nora Lustig, and Daniel Valderrama. 2016. “Understanding the Dynamics of Labor Income Inequality in Latin America.” World Bank Policy Research Working Paper No. 7795.
- Rodrik, Dani. 2005. “Growth Strategies.” In Handbook of Economic Growth, edited by Philippe Aghion and Steven Durlauf, 967–1014. Amsterdam.
- Rosnick, David, and Mark Weisbrot. 2014. “Latin American Growth in the 21st Century: The ‘Commodities Boom’ That Wasn’t.” CEPR Reports and Issue Briefs No. 2014-09.
- Sánchez Ancochea, Diego. 2019. “The Surprising Reduction of Inequality During a Commodity Boom: What Do We Learn from Latin America?” Journal of Economic Policy Reform, https://www.tandfonline.com/doi/full/https://doi.org/10.1080/17487870.2019.1628757
- Schady, Nobert, Jere Behrman, Maria Araujo, Rodrigo Azuero, Raquel Bernal, David Bravo, Florencia Lopez-Boo, et al. 2014. “Wealth Gradients in Early Childhood Cognitive Development in Five Latin American Countries.” IDB Working Paper No. 482.
- Schultz, Theodore. 1961. “Investment in Human Capital.” American Economic Review 51 (1): 1–17.
- Serbin, Andrés, Laneydi Martínez, and Haroldo Ramanzini. 2012. El regionalismo “post–liberal” en América Latina y el Caribe: Nuevos actores, nuevos temas, nuevos desafíos: Anuario de la Integración Regional de América Latina y el Gran Caribe 2012. Buenos Aires (in press).
- Tsounta, E., and A. Osueke. 2014. “What is behind Latin America’s Declining Income Inequality?” IMF Working Paper, 14/124.
- United Nations Educational, Scientific and Cultural Organization. 2017. “More Than One-Half of Children and Adolescents Are Not Learning Worldwide.” UNESCO Fact Sheet No. 46 UIS/FS/2017/ED/46.
- Uriarte, Oscar. 2007. La política laboral de los gobiernos progresistas. Uruguay (in press).
- van IJzendoorn, Marinus, and Femmie Juffer. 2006. “The Emanuel Miller Memorial Lecture 2006: Adoption as Intervention. Meta-Analytic Evidence for Massive Catch-up and Plasticity in Physical, Socio-Emotional, and Cognitive Development.” Journal of Child Psychology and Psychiatry 47 (12): 1228–1245. doi:https://doi.org/10.1111/j.1469-7610.2006.01675.x.
- Verbeeck, Marno. 2017. A Guide to Modern Econometrics. Chichester, England: John Wiley & Sons.
- Vogl, Tom. 2014. “Height, Skills, and Labor Market Outcomes in Mexico.” Journal of Development Economics 107: 84–96. doi:https://doi.org/10.1016/j.jdeveco.2013.11.007.
- Wang, Yang, Nora Lustig, and Otavio Bartalotti. 2016. “Decomposing Changes in Male Wage Distribution in Brazil.” In Income Inequality Around the World, edited by Lorenzo Cappellari, Solomon Polachek, and Konstantinos Tatsiramos, 49–78. Bingley.
- Williamson, Jeffrey. 2015. “Latin American Inequality: Colonial Origins, Commodity Booms or a Missed Twentieth-Century Leveling?” Journal of Human Development and Capabilities 16 (3): 324–341. doi:https://doi.org/10.1080/19452829.2015.1044821.
- World Bank. 2019. World Bank Open Data. Database.
- World Health Organization. 1998. Healthy Nutrition: An Essential Element of a Health-Promoting School. Geneva (in press).
Appendices
Appendix 1
The changes in education are related to the skill premium, that is the gap between low and high skills wages. As mentioned in the article, the skill premium had a downward trend in the period analyzed. To visualize this trend, we apply a Mincer equation for the years 2002–2015. This equation relates income from work to the type of education and other intrinsic characteristics of people, but not from work. We used the CMAEH database (IDB Citation2019) for Argentina, Bolivia, Brazil, Chile, Colombia, Ecuador, Paraguay, Peru, Uruguay and Venezuela. The equation is as follows:
(A1)
(A1) where
represents the natural logarithm of the working income per hour,
the individual;
is a binary variable that takes the value of 1 if the individual is an unskilled worker;
is its associated coefficient (it estimates the return of the labor wage associated with skilled workers in relation to unskilled workers);
the experience,
and
the coefficient associated with the experience;
represents a vector of control variables that includes sex, marital status, occupation type, country area, among others;
the coefficient associated with the vector of control variables and
the term of error.
We implemented a two stage Heckman correction model to correct the bias when selecting samples in a nonrandom way, in this case, of people who do not report an income corresponding to that of the main activity. For this we calculate a probit model where the dependent variable is occupied or not. From this, we obtained the inverse of the Mills ratio, used later as regressor in the model of ordinary minimum squares. The estimation was made with sample weights. Figure A1 shows the coefficient to each year and South American country. An increasing average trend can be observed in each South American country in the return perceived by unskilled workers in relation to skilled workers. In the case of Chile, due to the availability of data, the regression could only be estimated in five years within the analysis period, so its trend is inconclusive.
Appendix 2
An increasing average trend can be observed in each South American country in the return perceived by unskilled workers in relation to skilled workers. Behind this proposal remains the assumption that low skilled workers contribution to productivity increased more than the high skilled contribution, and, consequently, low skilled workers had a better pay for that. Given that the wage is the payment for work done, the capability of workers to contribute to productivity would be a wage variable. If the capability to contribute to productivity increases, wages also increase.
To analyze the relationship between productivity and skill premium we took the interannual variation of the natural logarithm of the South American index of labor productivity and the average of skilled and unskilled wages, considered in terms of Purchasing Power Parity (PPP), with 2000 as its base year. South American productivity is the natural logarithm of the average of each country, calculated as the contribution to output per worker, as expressed in Equation (A2).
(A2)
(A2) where
represents each South American country,
is the year,
is the Gross Domestic Product,
is the human capital,
is the workforce and
is the average years of schooling.
In order to account for this relationship, we estimated a panel data model for nine South American countries in the period 2000–2006, following Equations (5) and (6) for unskilled and skilled workers, respectively. How these equations should be estimated depends on the assumptions made about the individual effect, i.e. the time-invariant characteristics of each country, and its relationship with all the variables included in each equation. Given that we assume that these individual chatacteristics will be correlated with the variables included in each model, we prefer to use a Fixed Effects Model. As all variables included in the model are stationary at first difference, we used the first difference estimator, which also removes these time-invariant characteristics of each country (Verbeeck Citation2017).
(5)
(5)
(6)
(6) where
represent unskilled,
represent skilled,
represents each South American country,
is the year,
is the constant term,
is the coefficient associated with productivity,
is the productivity,
is the vector of estimated coefficients of the control variable matrix
and
is the error term. As robustness check, we estimated a second specification for each model, where we included time fixed effects. All of the specifications are estimated with clustered standard errors at country level. The results are shown in .
Table A1. Relationship between productivity and qualified and unqualified wages (2000–2016).
Both, the significance and the sign are as expected for each case. The productivity coefficient is higher and significant when applying the model that considers unskilled wages as an independent variable. Productivity gains translate into higher incomes for uneducated professionals than for skilled workers. Hence, this suggests that low skilled workers would be contributing more to productivity, which would result in the empirical evidence regarding the reduction in the skill premium. This results maintain after considering time fixed effects.
Appendix 3
As mentioned above, the models were estimated again, but this time considering the (2) recalculated indices. In the first place, it was decided to give greater weight to the education variable because there has been extensive literature on the relationship between education and the productive capacity of workers. Therefore, this new index was considered, precisely considering the explanatory power of workers’ education to determine their productivity. Results are shown in .
Table A2. Models estimation using PCI with different weights.
As can be seen, results are not much different from those obtained before. The PCI ratio is associated with a negative and significant coefficient, as well as before. A final robustness check was done by calculating the PCI using the Principal Component Analysis (PCA). In this case, we obtained one index per quintile, using PCA, and then obtained the ratio Q1Q2/Q5. We standardize our indexes so that it has a minimum value of 0 and a maximum value of 1. Results are shown in .
Table A3. Models estimation using PCA PCI
In this case, the expected negative sign and the magnitude of the coefficient of interest (ACP Q1Q2/Q5), maintained with respect of previous models. However, it is important to notice that the significance of the variable is lost when we estimate the models using clustered standard errors in the panel fixed effect specification and when we use PCSE. Nonetheless, our preferred specification (GMM) remains significant at a 95% level of confidence.