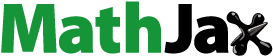
ABSTRACT
In this study, we examine the existence of an inverted U-shaped relationship between tourism and carbon emissions in 29 sub-Saharan African (SSA) countries using data from 1995 to 2019. Departing from the previous studies, we have also grouped SSA countries into low- and middleincome countries, thereby estimating three panels. We have used a wide range of panel estimation techniques to examine this linkage, including the dynamic common-correlated effects (DCCE), the Driscoll-Kraay estimators, and the fully-modified OLS (FMOLS). Our results show that the relationship between tourism and carbon emissions depends largely on the estimation technique used and the level of income of the individual countries. Overall, we can conclude that there is primafacie evidence of an inverted U-shaped relationship between tourism and carbon emissions in middle-income countries and all SSA countries combined, but not in low-income countries. This means that the perceived adverse effects of tourism expansion on environmental pollution may only be experienced in the early stages of tourism development and that as the tourism sector develops, tourism is likely to promote energy conservation through sustainable tourism or green tourism, thereby leading to a reduction in carbon emissions. Policy implications are discussed.
1. Introduction
The relationship between tourism and environmental degradation has attracted a conglomerate of empirical literature in recent years. To date, there have been three contentious views on the dynamic relationship between these two macroeconomic variables. The first view posits that tourism leads to environmental degradation via an increase in energy consumption. Even though tourism development contributes significantly to economic growth and development, it may also lead to environmental degradation in the form of CO2 emissions at both national and international levels. In the main, the proponents of this view argue that, since most of the tourism activities lead to an increase in energy consumption, which is largely generated from non-renewable energy sources such as coal, natural gas, oil, etc., an increase in tourism inflow or tourism development is likely to lead to an increase in carbon emissions, thereby leading to an increase in environmental degradation. To put it slightly differently, this view argues that, since tourism activities have a positive impact on energy consumption, they are, by design, contributing to one of the anthropogenic causes of global warming (see Scott, Peeters, and Gössling Citation2010; Becken and Simmons Citation2002). Some of the tourism activities that emit significant amounts of carbon emissions include transport-related activities such as scenic flights, jet boating and air travelling. The second view, however, maintains that it is possible for tourism to contribute significantly towards environmental protection in the long run, since the revenue generated from tourism activities could be invested in the exploration of energy from renewable sources. Although tourism has the potential to lead to environmental degradation, a well-managed tourism that promotes the use of environment-friendly technology and transportation, can also contribute to environmental protection (see UNEP Citation2008).
Although the majority of previous studies have focused mainly on the linear relationship between tourism development and carbon emissions, some recent studies have also found an inverse U-shaped relationship between tourism and carbon emissions (see, for example, Bai and Zeng, 2019). According to the inverted U-shaped curve phenomenon, environmental pollution is expected first to rise in the early stages of tourism development and then to fall as tourism development progresses. The presence of an inverted U-shaped curve, therefore, implies that tourism exploration might increase carbon emissions only in the early stages of tourism development and that as the tourism sector develops, tourism could promote energy conservation through sustainable tourism or green tourism, thereby leading to a reduction in carbon emissions.
In this paper, we aim to examine the existence of an inverted U-shaped relationship between tourism and CO2 emissions in selected sub-Saharan African (SSA) countries during the period between 1995 and 2019. Although the existence of an inverted U-shaped relationship between carbon emissions and other macroeconomic variables has been investigated extensively in the literature, very few studies have explored fully the link between tourism development and carbon emissions within the inverted U-shaped curve framework. Moreover, the majority of previous studies in developing countries have concentrated mainly on Asia and Latin American countries, thereby leaving African countries, especially sub-Saharan African countries, with very little or no coverage.
As a departure from previous studies, we employ three SSA datasets, namely (i) low-income countries dataset; (ii) middle-income countries dataset; and (iii) full sample dataset for all SSA countries. We also employ a wide range of estimation techniques based on the DCCE, Driscoll-Kraay estimators, and FMOLS. Other estimation techniques used in our study include, among others, (i) four cross-sectional dependence tests, namely the Baltagi, Feng, and Kao (Citation2012) bias-corrected scaled LM test, Pesaran (Citation2004) scaled LM test, Pesaran (Citation2004) CD test, and Breusch and Pagan (Citation1980) LM test; (ii) second-generation unit root test by Pesaran (Citation2007) and (iii) the second-generation cointegration test by Westerlund (Citation2007). To our knowledge, this may be the first study of its kind to extensively examine this linkage in SSA countries using modern econometric techniques.
The rest of the paper is divided into four sections. In Section 2, we present an overview of previous studies regarding the relationship between tourism development and CO2 emissions in both developed and developing nations. Section 3 provides a summary of the methodology used, the empirical model specification and the data sources. Section 4 delves into the empirical analysis and the discussion of the results, while Section 5 concludes the study and gives some policy implications.
2. Literature review
A number of studies have examined the relationship between tourism and carbon emissions, but with conflicting results. Studies that found tourism to contribute towards or to lead to environmental degradation include León, Arana, and Alemán (Citation2014), Solarin (Citation2014), Al-Mulali, Fereidouni, and Mohammed (Citation2015), Durbarry and Seetanah (Citation2015), Dogan, Seker, and Bulbul (Citation2017), Shakouri, Yazdi, and Ghorchebigi (Citation2017), Sharif, Afshan, and Nisha (Citation2017), Zaman, Moemen, and Islam (Citation2017), Sudharshan, Alam, and Lau (Citation2018), Balli et al. (Citation2019), Katircioglu et al. (Citation2020), Koçak, Ulucak, and Ulucak (Citation2020), Shi et al. (Citation2020), among others. León, Arana, and Alemán (Citation2014), for example, while examining the link between carbon emissions and tourism in developed and less developed countries using a balanced panel dataset during the period between 1998 and 2006, found that tourism contributes significantly to CO2 emissions in both less developed and developed countries. Solarin (Citation2014), while examining the determinants of CO2 emissions in Malaysia during the period from 1972 to 2010, found, inter alia, that tourist arrivals are active contributors to pollution in Malaysia. Al-Mulali, Fereidouni, and Mohammed (Citation2015), while examining the effect of tourism arrival on CO2 emissions from the transportation sector using data from 48 top international tourism destinations during the period 1995–2009, found that tourism has a significant impact on the transport sector carbon emissions in all of the selected countries except the European countries. Durbarry and Seetanah (Citation2015), while examining the impact of long-haul destinations on CO2 emissions in Mauritius during the period from 1978 to 2011, found that a short-run and long-run positive relationship exists between tourism development and carbon emissions. Dogan, Seker, and Bulbul (Citation2017), in examining the effects of energy consumption, real GDP, tourism and trade on carbon emissions in OECD countries using the dynamic ordinary least squares (DOLS) estimation technique, found, inter alia, that tourism contributes to the levels of gas emissions in the studied countries. Shakouri, Yazdi, and Ghorchebigi (Citation2017), in a study aimed at examining tourism development promoting carbon emissions in the selected Asia-Pacific countries during the period between 1995 and 2013, found that tourism has a positive and significant impact on carbon dioxide emissions in the long run. Sharif, Afshan, and Nisha (Citation2017), while examining the effect of tourism on carbon emissions in Pakistan during the period from 1972 to 2013, found that there is a positive interaction between carbon emissions and tourism in the long run. Zaman, Moemen, and Islam (Citation2017), while, inter alia, examining the link between tourism transportation expenditures and carbon emission in 11 transition economies during the period from 1995 to 2013, found that international tourism receipts and international tourism expenditures are associated with the higher CO2 emission. Sudharshan, Alam, and Lau (Citation2018), while examining the impact of tourism investment on tourism development and CO2 emission in the EU nations during the period between 1990 and 2013, found that tourism investment, which leads to an increase in tourism revenue also leads to a decrease in carbon emissions. Balli et al. (Citation2019), exploring the relationship between tourism, CO2 emissions and economic growth using a sample of Mediterranean countries, found that an increase in tourism receipts per capita leads to an increase in carbon emission per capita in the studied countries. Katircioglu et al. (Citation2020), in examining the impact of tourism on emission pollutants using data from Northern Cyprus from 1977 to 2015, inter alia, found that tourism has a long-run positive and significant impact on carbon emissions, thereby revealing that the tourism sector causes environmental degradation. Koçak, Ulucak, and Ulucak (Citation2020), in examining the impact of tourism developments on CO2 emissions in the most visited countries during the period from 1995 to 2014, found, inter alia, that tourism arrivals lead to an increase in carbon emissions, while tourism receipts lead to a decrease in carbon emissions. Shi et al. (Citation2020), in examining the multi-relationships between tourism, economic growth, CO2 emissions, and primary energy consumption at different stages of development among 147 countries during the period from 1995 to 2015, found that a 1% increase in expenditure on inbound tourism increases carbon emissions by 0.084% in low-income countries, while a 1% increase in the net inflow of international tourism leads to an increase in carbon emissions in low-income countries and high-income countries by 0.072% and 0.059%, respectively.
In contrast to the studies mentioned above, there are studies which found that tourism contributes towards a decrease rather than an increase in environmental degradation. These include studies such as Lee and Brahmasrene (Citation2013), Başarir and Çakir (Citation2015), Paramati, Alam, and Lau (Citation2018), Bojanic and Warnick (Citation2020), and Menyari (Citation2021), among other studies. Lee and Brahmasrene (Citation2013), for example, in a study aimed at examining the effect of tourism and economic growth on CO2 emissions in EU countries over the period from 1988 to 2009, found, inter alia, that tourism has a high significant negative impact on carbon emissions. Başarir and Çakir (Citation2015), while investigating the link between tourism, financial development, energy consumptions and CO2 emissions in Turkey and four main competitors of Turkish tourism in EU countries, namely France, Spain, Italy and Greece during the period from 1995 to 2010, found, inter alia, that one percentage increase in tourist arrival will decrease CO2 emissions by 0.11%. Paramati, Alam, and Lau (Citation2018), in examining the impact of tourism investment on tourism development and CO2 emissions in 28 EU nations, found that tourism investment has a long-run negative significant impact on carbon emissions. Bojanic and Warnick (Citation2020), in a study aimed at examining the relationship between tourism level and environmental performance using global data that spanned between 1995 and 2016, found, inter alia, that higher tourism density lowers greenhouse gas (GHG) emissions and leads to better environmental performance. Menyari (Citation2021), in examining the effects of tourism, economic growth, and electricity consumption on CO2 emission, using a panel dataset from four North African countries, namely Algeria, Egypt, Morocco and Tunisia during the period from 1980 to 2014, found that tourism has a negative effect on CO2 emissions, implying that tourism leads to an improvement in the quality of the environment.
Apart from the above studies, there are a few studies that have attempted to examine the existence of either a non-linear relationship between tourism and carbon emissions or the relevance of a tourism-induced environmental Kuznets curve in different countries. These include studies such as Katircioǧlu (Citation2014), Paramati, Alam, and Chen (Citation2017), Sherafatian-Jahromi et al. (Citation2017), Bi and Zeng (Citation2019), Ehigiamusoe (Citation2020), Ișik et al. (Citation2020), Raza et al. (Citation2021), Jiaqi et al. (Citation2022) and Sharma et al. (Citation2023), among others. Katircioǧlu (Citation2014), for example, while examining the relevance of the tourism-induced environmental Kuznets curve hypothesis in Singapore from 1971 to 2010, found evidence in support of a tourism-induced EKC. Paramati, Alam, and Chen (Citation2017), while, inter alia, examining the dynamic link between tourism, economic growth, and CO2 emissions in developed and developing economies, found that the impact of tourism on carbon emissions is reducing much faster in developed economies than in developing economies, thereby providing support for the environmental Kuznets curve (EKC) hypothesis on the link between tourism growth and CO2 emissions. In examining the link between tourism and CO2 emissions nexus in Southeast Asian countries, Sherafatian-Jahromi et al. (Citation2017) found that an inverted U-shaped relationship exists between tourism and carbon emissions in the countries under study. While examining the nonlinear and spatial impact of tourism on CO2 emissions in China, using the provincial panel data from 2003 to 2016, Bi and Zeng (Citation2019) found that there is an inverse U-shaped link between tourism and CO2 emissions. Ehigiamusoe (Citation2020), while examining the non-linear relationship between tourism, growth and environment in African countries, found that tourism has a U-shaped non-linear impact on environmental degradation. Ișik et al. (Citation2020), while examining the relevance of the environmental Kuznets curve (EKC) hypothesis using data from seven (G7) countries over the period 1995–2015, found that the tourism-induced EKC hypothesis is only valid for France. Raza et al. (Citation2021), in examining the existence of a non-linear relationship between tourism, economic growth, urbanisation, and environmental degradation in top tourist arrival destinations over the period from 1995 to 2017, found that there is an inverted U-shaped relationship between tourism and environmental degradation. In other words, there is a point beyond which further increase in tourism leads to a decrease in environmental degradation. Jiaqi et al. (Citation2022), while examining the spillover of tourism development on CO2 emissions using data from the top 70 tourist countries, found that an inverted-U-shaped relationship exists between tourism and CO2 emissions. More recently, Sharma et al. (Citation2023) examined the impact of tourism sector development on carbon emissions in India within the context of the tourism – induced environmental Kuznets curve hypothesis. Using time series data from 1980 to 2019, the study found evidence in support of the tourism-induced EKC hypothesis in India.
Based on the studies reviewed in this section, it is evident that the results of the previous studies on the relationship between tourism and carbon emission are inconclusive at best and differ from country to country. Moreover, the majority of these studies did not examine the link between tourism and carbon emissions through the lens of the inverted U-shaped curve framework, which qualifies the novelty of the current study.
3. Model specification and methodology
The basic model used in this study to examine the relationship between tourism and CO2 emissions in sub-Saharan African (SSA) countries within the inverted U-shaped curve framework can be presented as follows:
(1)
(1) where:
CO2 = Carbon emissions
TA = Tourism arrivals
TA2 = square of tourism arrivals
y = real GDP per capita (economic growth)
Urban = Urbanisation
Trade = Exports + Imports/GDP.
The above model can be re-parameterised as follows:
(2)
(2) where:
i = ranges from 1 to N
t = ranges from 1 to T
= represents an intercept
−
= represent regression coefficients to be estimated
= error term.
According to the inverted U-shaped curve framework, the parameter should be positive and statistically significant, while
should be negative and statistically significant in order for the inverted U-shaped relationship between tourism arrivals and environmental degradation to prevail. If the opposite occurs, i.e.
is negative and
is positive, then the relationship between tourism arrivals and carbon emissions is regarded as U-shaped instead of inverted U-shaped.
This study uses three techniques, namely the dynamic common correlated effects mean group (DCCE-MG), the Driscoll-Kraay standard errors, and the fully-modified OLS. The dynamic common correlated effects mean group approach was recently proposed by Chudik and Pesaran (Citation2015). It is an extension of the common correlated effects (CCE) approach, which was developed by Pesaran (Citation2006) for regressors that are weakly exogenous. Unlike the CCE approach, the DCCE-MG not only controls for cross-sectional dependence by including cross-sectional averages, but it also includes their lags in the estimation (see also Ahmad et al. Citation2021; Ditzen Citation2019).
Following previous studies such as those of Chen et al. (Citation2023), and Chaudhry, Yusop, and Habibullah (Citation2022), the DCCE model can be expressed as follows:
(3)
(3) where:
Y represents the dependent variable (CO2)
represents the lagged dependent variable (which is now treated as one of the independent variables) for country (i) at time period (t).
is a set of the independent variables [tourism arrivals (TA), tourism arrivals squared (TA2), economic growth (y), urbanisation (URB), and trade (Trade)]
and
denotes unobserved common elements.
represents the lags of cross-sectional averages.
represents the error term.
The D-K’s mathematical form can be expressed as follows:
(4)
(4) where:
Y = Dependent variable
Z = Independent variables
i = countries
t = time period.
As a robustness check, the study also employed the fully-modified OLS (FMOLS) to corroborate the DCCE-MG and the Driscoll and Kraay (Citation1998) estimators. The FMOLS estimation technique has been found to be appropriate for heterogeneous cointegrated panels (Pedroni Citation2000). The technique has been found to lead to efficient and consistent estimation of cointegrating vectors and to address the endogeneity problem (see Christopoulos and Tsionas Citation2004).
3.1. Data
The study used annual panel data for 29 sub-Saharan African countriesFootnote1 from 1995 to 2020. The data was decomposed into three income groups, namely low-income countries, middle-income countries and all SSA countries. The selection of the study period was based on the availability of reliable data on the key variables included in the study. The data were largely obtained from the World Bank online database as well as from specific countries’ reports ().
Table 1. Definitions of variables.
4. Empirical analysis
4.1. Cross-sectional dependence and unit root tests
Owing to the possible presence of cross-sectional dependence (CD) among the countries included in our study, it is important for us first to check the presence of cross-sectional dependence among the studies countries. For this purpose, four tests are used, namely Breusch–Pagan LM, Pesaran scaled LM, Bias-corrected scaled LM by Baltagi, Feng, and Kao (Citation2012), and Pesaran CD. The results of these tests are reported in .
Table 2. Cross-sectional dependence test.
The results reported in show that there is cross-sectional dependence among the countries included in this study. This implies that the first-generation unit root tests may not be sufficient to establish the presence of stationarity among the variables included in our model. Hence, we have to use the second-generations unit root tests, such as the cross-sectionally augmented IPS unit root test (CIPS). The results of the stationarity tests based on CIPS are presented in .
Table 3. The results of the second-generation panel unit root tests – CIPS panel unit test.
The results reported in show that all the variables included in this study are integrated of order 1 [i.e. I(1)], since the variables are only stationery after first difference.
4.2. Westerlund (Citation2007) cointegration
Since cross-sectional dependence was found to exist among the countries included in this study, it is important to use the second-generation cointegration tests, such as the Westerlund (Citation2007) cointegration test, to examine the existence of a long relationship among the variables included in the model. The results of the Westerlund (Citation2007) cointegration test are reported in .
Table 4. Westerlund (Citation2007) panel cointegration test.
The results reported in show that cointegration among the variables included in our model has been confirmed by Westerlund (Citation2007) Robust P-value, which has been found to be statistically significant in all the country groups, i.e. low-income, middle-income and all countries.
4.3. Empirical results
Given the presence of cross-sectional dependence in our study, it is important that we use estimators that address the cross-sectional dependence when examining the impact of tourism arrivals on carbon emissions in the studied SSA countries. For this purpose, the study used two commonly used estimators, namely the dynamic common correlated effects (DCCE), which was recently developed by Chudik and Pesaran (Citation2015), and the Driscoll-Kraay (D-K) estimators. In addition, the study employed the FMOLS estimation approach to establish whether the result would still hold even if the cross-sectional dependence were not accounted for. The results of the DCCE-MG and Driscoll-Kraay (D-K) estimators are reported in and , respectively, while the results of FMOLS are reported in .
Table 5. DCCE results.
Table 6. The result of regression with Driscoll-Kraay standard errors.
Table 7. FMOLS results.
The empirical results reported in , based on the DCCE-MG, show that when all SSA countries and middle-income countries are considered, an inverted U-shaped relationship was found to prevail between tourism and carbon emission. This has been confirmed by the coefficients of the tourism arrivals and tourism arrivals squared, which have been found to be positive and negative, respectively, in all SSA and middle-income SSA countries. Unlike in all SSA and middle-income countries, the results of the low-income countries show that tourism arrivals have no significant impact whatsoever on carbon emissions. This finding has been confirmed by the coefficients of tourism and tourism squared in low-income countries, which both have been found to be statistically insignificant.
The results of the D-K standard error approach reported in , on the other hand, show that when pooled OLS regression results were considered, an inverted U-shaped relationship between tourism and carbon emissions was rejected in all the study countries, irrespective of whether the test was conducted on low-income, middle-income or all SSA countries combined. Instead, the results show that there is evidence of a U-shaped relationship between tourism and carbon emissions in both middle-income countries and all SSA countries combined. This has been confirmed by the coefficients of the tourism and tourism squared, which have been found to be negative and positive, respectively.
However, when fixed effects regression results were considered, the results show that there is an inverted U-shaped relationship between tourism and carbon emissions in both middle-income and all SSA countries, but not in low-income countries. This has been confirmed by the coefficients of tourism and tourism squared, which have been found to be positive and negative, respectively, in both middle-income and all SSA countries, but insignificant in low-income countries. These results have been found to be consistent with the results from the random-effects GLS regression, which also confirmed the presence of an inverted U-shaped relationship between tourism and carbon emissions in the case of middle-income and all SSA countries but not in low-income countries. As in the case of fixed-effects regression results, coefficients of tourism and tourism squared in the random-effects GLS results were found to be positive and negative, respectively, in middle-income and all SSA countries, but not in low-income countries. Overall, the results of D-K estimators show that the inverted U-shaped curve hypothesis between tourism and carbon emissions is found to prevail in middle-income and all SSA countries when fixed-effects and random-effects GLS results are considered, but not when pooled OLS regression results are considered. However, in the case of low-income countries, the results show that the inverted U-shaped curve hypothesis between tourism and carbon emissions is rejected by all the D-K regression results, namely the pooled OLS, the fixed-effects and the random-effects GLS estimators. This shows that there is a prima-facie evidence that an inverted U-shaped relationship exists in middle-income and all SSA-countries combined, but not in low-income countries. Therefore, in the main, these results corroborate the results of the dynamic common correlated effects MG, which found that an inverted U-shaped curve prevails in middle-income and all-SSA countries combined, but not in low-income SSA countries.
The results of the DCCE-MG and Driscoll-Kraay (D-K) estimators for middle-income and all SSA countries combined have been more or less supported by the results of the FMOLS, which have been reported in . As reported in this table, the results show that when the FMOLS estimator is used, an inverted U-shaped relationship is found to prevail between tourism and carbon emissions in middle-income and all SSA countries combined. This finding has been supported by the coefficients of the tourism and tourism squared in both specifications, which have been found to be positive and negative, respectively. Contrary to the findings of the middle-income and all SSA countries combined, the results of the low-income countries based on the FMOLS estimator show that there is a distinct U-shaped relationship between tourism and carbon emissions. This finding has been confirmed by the coefficients of tourism and tourism squared, which have been found to be negative and positive, respectively. Overall, the results show that there is prima facie evidence in support of an inverted U-shaped relationship between tourism and carbon emissions in middle-income and all SSA countries combined. This finding is consistent with previous studies, such as Sherafatian-Jahromi et al. (Citation2017) in the case of Southeast Asian countries, Jiaqi et al. (Citation2022) in the case of the top 70 tourist countries, and Raza et al. (Citation2021) in the case of top tourist arrival destination, among others. In the case of low-income countries, we could not find any evidence of the inverted U-shaped curve hypothesis. This applies irrespective of the estimator used in the analysis.
5. Conclusion
This study aimed to examine the relationship between tourism development and carbon emissions within the context a non-linear framework. In the main, the study aimed to examine the existence of an inverted U-shaped relationship between tourism and CO2 emissions in 29 sub-Saharan African (SSA) countries during the period between 1995 and 2019. Departing from previous studies, the study estimates three datasets, namely (i) low-income countries dataset; (ii) middle-income countries dataset; and (iii) all SSA countries dataset. The study also employed a wide range of estimation techniques to examine this linkage, namely the dynamic common correlated effects (DCCE) recently developed by Chudik and Pesaran (Citation2015) and the Driscoll-Kraay (D-K) estimator. In addition, the study employed the FMOLS estimation approach, which accounts for endogeneity. The findings of this study show that the magnitude of the relationship between tourism and carbon emissions depends largely on the estimation technique used and the individual countries’ income group. When the DCCE-MG is used as an estimator, the results support an inverted U-shaped relationship between tourism and carbon emission in middle-income countries and all SSA countries combined. However, when the low-income SSA specification is considered, the results show that tourism has no significant impact whatsoever on carbon emissions. The results of the Driscoll-Kraay (D-K) estimator also confirmed the presence of an inverted U-shaped relationship between tourism and carbon emissions in middle-income and all SSA countries combined but not in low-income countries when fixed effects and random effects-GLS estimators are used. However, no evidence of the inverted U-shaped relationship between tourism and carbon emissions was found in any country groups when the pooled OLS estimator was used. These results, therefore, tally with those of the DCCE-MG. The presence of an inverted U-shaped relationship between tourism and carbon emissions, which has been found to predominate in middle-income and all SSA countries combined when the DCCE-MG and D-K estimators are used, has also been corroborated by the results of the fully modified OLS (FMOLS). Indeed, the results of the FMOLS show that, in both middle-income and all SSA countries combined, there is a distinct inverted U-shaped relationship between tourism and carbon emissions. However, unlike the DCCE-MG and D-K estimators, the FMOLS found that in low-income countries, there is a distinct U-shaped relationship between tourism and carbon emissions. Overall, we can conclude that there is prima-facie evidence of an inverted U-shaped relationship between tourism and carbon emissions in middle-income and all SSA countries combined, but not in low-income countries. This means that the perceived negative impact of tourism expansion on environmental pollution may only be experienced in the early stages of tourism development and that as the tourism sector develops, tourism is likely to promote energy conservation through sustainable tourism or green tourism, thereby leading to a reduction in carbon emissions. Hence, it is recommended that middle-income SSA countries accelerate policies aimed at sustainable tourism since the exploration of such policies will not only expand their tourism industry but may also result in a substantial reduction in their carbon emissions. In the case of low-income countries, the neutrality relationship between tourism and carbon emissions, as supported by two out of three estimators, suggests that policies aimed at the expansion of the tourism sector should be intensified as further development of the tourism sector in those countries is highly unlikely to have any adverse significant effects on the environment.
Like other empirical studies, this study has some limitations. For example, the study was constrained by the availability of reliable data for SSA countries. Only countries with sufficient data were included in this study. Hence, only estimators that could accommodate our dataset were used. Future studies may, therefore, wish to expand the scope of the current research when data become available by using other estimators to establish whether their findings will differ significantly from the findings of this study.
Disclosure statement
No potential conflict of interest was reported by the author(s).
Additional information
Funding
Notes
1 The countries included in this study are Angola; Benin; Botswana; Burkina Faso; Burundi; Cabo Verde; Central African Republic; Chad; Comoros; Congo, Dem. Rep.; Eritrea; Eswatini; Gambia, The; Ghana; Guinea; Kenya ; Lesotho; Mali; Mauritius; Namibia; Niger; Nigeria; South Africa; Sudan; Tanzania; Togo; Uganda; Zambia; Zimbabwe.
References
- Ahmad, M., A. Rehman, S. A. A. Shah, Y. A. Solangi, A. A. Chandio, and G. Jabeen. 2021. “Stylized Heterogeneous Dynamic Links among Healthcare Expenditures, Land Urbanization, and CO2 Emissions Across Economic Development Levels.” Science of The Total Environment 753: 142228. https://doi.org/10.1016/j.scitotenv.2020.142228.
- Al-Mulali, U., H. G. Fereidouni, and A. H. Mohammed. 2015. “The Effect of Tourism Arrival on CO2 Emissions from Transportation Sector.” An International Journal of Tourism and Hospitality Research 26 (2): 230–243.
- Balli, E., C. Sigeze, M. Manga, S. Birdir, and K. Birdir. 2019. “The Relationship Between Tourism, CO2 Emissions and Economic Growth: A Case of Mediterranean Countries.” Asia Pacific Journal of Tourism Research 24 (3): 219–232. https://doi.org/10.1080/10941665.2018.1557717.
- Baltagi, B. H., Q. Feng, and C. Kao. 2012. “A Lagrange Multiplier Test for Cross-Sectional Dependence in a Fixed Effects Panel Data Model.” Journal of Econometrics 170 (1): 164–177. https://doi.org/10.1016/j.jeconom.2012.04.004.
- Başarir, Ç, and Y. N. Çakir. 2015. “Causal Interactions Between CO2 Emissions, Financial Development, Energy and Tourism.” Asian Economic and Financial Review 5 (11): 1227–1238.
- Becken, S., and D. G. Simmons. 2002. “Understanding Energy Consumption Patterns of Tourist Attractions and Activities in New Zealand.” Tourism Management 23: 343–354. https://doi.org/10.1016/S0261-5177(01)00091-7.
- Bi, C., and J. Zeng. 2019. “Nonlinear and Spatial Effects of Tourism on Carbon Emissions in China: A Spatial Econometric Approach.” International Journal of Environmental Research and Public Health 16 (18): 3353. https://doi.org/10.3390/ijerph16183353.
- Bojanic, D. C., and R. B. Warnick. 2020. “The Relationship Between a Country’s Level of Tourism and Environmental Performance.” Journal of Travel Research 59 (2): 220–230. https://doi.org/10.1177/0047287519827394.
- Breusch, T., and A. Pagan. 1980. “The Lagrange Multiplier Test and Its Applications to Model Specification in Econometrics.” The Review of Economic Studies 47 (1): 239–254. https://doi.org/10.2307/2297111.
- Chaudhry, I. S., Z. Yusop, and M. S. Habibullah. 2022. “Dynamic Common Correlated Effects of Financial Inclusion on Economic Growth: Empirical Evidence from Organization of Islamic Cooperation (OIC) Countries.” International Journal of Emerging Markets. https://www.emerald.com/insight/1746-8809.htm
- Chen, Y. S., Z. Chen, S. Ali, M. I. Ullah, and M. K. Answer. 2023. “Dynamic Common Correlated Effects of Financial Inclusion on Foreign Direct Investment: Evidence from East-Asia and Pacific Countries.” Borsa Istanbul Review 23 (3): 541–549. https://doi.org/10.1016/j.bir.2022.12.002.
- Christopoulos, D. K., and E. G. Tsionas. 2004. “Financial Development and Economic Growth: Evidence from Panel Unit Root and Cointegration Tests.” Journal of Development Economics 73 (1): 55–74. https://doi.org/10.1016/j.jdeveco.2003.03.002.
- Chudik, A., and M. H. Pesaran. 2015. “Common Correlated Effects Estimation of Heterogeneous Dynamic Panel Data Models with Weakly Exogenous Regressors.” Journal of Econometrics 188 (2): 393–420. https://doi.org/10.1016/j.jeconom.2015.03.007.
- Ditzen, J. 2019. Estimating Long run Effects in Models with Cross-Sectional Dependence Using xtdcce2. Technical Report 7. CEERP Working Paper.
- Dogan, E., F. Seker, and S. Bulbul. 2017. “Investigating the Impacts of Energy Consumption, Real GDP, Tourism and Trade on CO2 Emissions by Accounting for Cross-Sectional Dependence: A Panel Study of OECD Countries.” Current Issues in Tourism 20 (16): 1701–1719. https://doi.org/10.1080/13683500.2015.1119103.
- Driscoll, J., and A. C. Kraay. 1998. “Consistent Covariance Matrix Estimation with Spatially Dependent Panel Data.” Review of Economics and Statistics 80: 549–560. https://doi.org/10.1162/003465398557825.
- Driscoll, J. C., and A. C. Kraay. 1998. “Consistent Covariance Matrix Estimation with Spatially Dependent Panel Data.” Review of Economics and Statistics 80 (4): 549–560. https://doi.org/10.1162/003465398557825.
- Durbarry, R., and B. Seetanah. 2015. “The Impact of Long Haul Destinations on Carbon Emissions: The Case of Mauritius.” Journal of Hospitality Marketing & Management 24 (4): 401–410. https://doi.org/10.1080/19368623.2014.914363.
- Ehigiamusoe, K. U. 2020. “Tourism, Growth and Environment: Analysis of Non-Linear and Moderating Effects.” Journal of Sustainable Tourism 28 (8): 1174–1192. https://doi.org/10.1080/09669582.2020.1729164.
- Gao, J., W. Xu, and L. Zhang. 2021. “Tourism, Economic Growth, and Tourism-Induced EKC Hypothesis: Evidence from the Mediterranean Region.” Empirical Economics 60: 1507–1529. https://doi.org/10.1007/s00181-019-01787-1.
- Hoechle, D. 2007. “Robust Standard Errors for Panel Regressions with Cross-Sectional Dependence.” The Stata Journal: Promoting Communications on Statistics and Stata 7 (3): 281–312. https://doi.org/10.1177/1536867X0700700301.
- Ișik, C., M. Ahmad, U. K. Pata, S. Ongan, M. Radulescu, F. F. Adedoyin, E. Bayraktaroğlu, S. Aydın, and A, Ongan. 2020. “An Evaluation of the Tourism-Induced Environmental Kuznets Curve (T-EKC) Hypothesis: Evidence from G7 Countries.” Sustainability 12: 9150. https://doi.org/10.3390/su12219150
- Jiaqi, Y., S. Yang, Y. Ziqi, L. Tingting, and B.S.X. Teo. 2022. “The Spillover of Tourism Development on CO2 Emissions: A Spatial Econometric Analysis.” Environmental Science and Pollution Research 29:26759–26774.
- Katircioǧlu, S. T. 2014. “Testing the Tourism-Induced EKC Hypothesis: The Case of Singapore.” Economic Modelling 41: 383–391. https://doi.org/10.1016/j.econmod.2014.05.028.
- Katircioglu, S., N. Saqib, S. Katircioglu, C. C. Kilinc, and H. Gul. 2020. “Estimating the Effects of Tourism Growth on Emission Pollutants: Empirical Evidence from a Small Island, Cyprus.” Air Quality, Atmosphere & Health 13: 391–397.
- Koçak, E., R. Ulucak, and Z. S. Ulucak. 2020. “The Impact of Tourism Developments on CO2 Emissions: An Advanced Panel Data Estimation.” Tourism Management Perspectives 33), https://doi.org/10.1016/j.tmp.2019.100611.
- Koenker, R. 2005. Quantile Regression, Econometric Society Monographs. Cambridge University Press.
- Lee, J. W., and T. Brahmasrene. 2013. “Investigating the Influence of Tourism on Economic Growth and Carbon Emissions: Evidence from Panel Analysis of the European Union.” Tourism Management 38: 69–76. https://doi.org/10.1016/j.tourman.2013.02.016.
- León, C. J., J. E. Arana, and A. H. Alemán. 2014. “CO2 Emissions and Tourism in Developed and Less Developed Countries.” Applied Economics Letters 21 (16): 1169–1173. https://doi.org/10.1080/13504851.2014.916376.
- Menyari, Y. E. 2021. “The Effects of International Tourism, Electricity Consumption, and Economic Growth on CO2 Emissions in North Africa.” Environmental Science and Pollution Research 28: 44028–44038. https://doi.org/10.1007/s11356-021-13818-5.
- Paramati, S. R., M. S. Alam, and C. F. Chen. 2017. “The Effects of Tourism on Economic Growth and CO2 Emissions: A Comparison Between Developed and Developing Economies.” Journal of Travel Research 56: 712–724. https://doi.org/10.1177/0047287516667848.
- Paramati, S. R., S. Alam, and C. K. M. Lau. 2018. “The Effect of Tourism Investment on Tourism Development and CO2 Emissions: Empirical Evidence from the EU Nations.” Journal of Sustainable Tourism 26 (9): 1587–1607. https://doi.org/10.1080/09669582.2018.1489398.
- Pedroni, P. 2000. Fully Modified OLS for Heterogeneous Cointegrated Panels. Department of Economics Working Papers 2000-03. Williams College.: Department of Economics.
- Pesaran, M. H. 2004. General Diagnostic Tests for Cross Section Dependence in Panels. Working Paper 0435. Cambridge: Faculty of Economics, University of Cambridge.
- Pesaran, M. H. 2006. “Estimation and Inference in Large Heterogeneous Panels with a Multifactor Error Structure.” Econometrica 74: 967–1012. https://doi.org/10.1111/j.1468-0262.2006.00692.x.
- Pesaran, M. H. 2007. “A Simple Panel Unit Root Test in the Presence of Cross-Section Dependence.” Journal of Applied Econometrics 22: 265–312. https://doi.org/10.1002/jae.951.
- Raza, S. A., M. A. Qureshi, M. Ahmed, et al. 2021. “Non-Linear Relationship Between Tourism, Economic Growth, Urbanization, and Environmental Degradation: Evidence from Smooth Transition Models.” Environmental Science and Pollution Research 28: 1426–1442. https://doi.org/10.1007/s11356-020-10179-3.
- Scott, D., P. Peeters, and S. Gössling. 2010. “Can Tourism Deliver its “Aspirational” Greenhouse gas Emission Reduction Targets?” Journal of Sustainable Tourism 18: 393–408. https://doi.org/10.1080/09669581003653542.
- Sharma, M., G. Mohapatra, A. K. Giri, A. Wijeweera, and C. Wilson. 2023. “Examining the Tourism–Induced Environmental Kuznets Curve Hypothesis for India.” Environment, Development and Sustainability https://doi.org/10.1007/s10668-023-04270-1.
- Shakouri, B., S. K. Yazdi, and E. Ghorchebigi. 2017. “Does Tourism Development Promote CO2 Emissions? Anatolia.” An International Journal of Tourism and Hospitality Research 28 (3): 444–452.
- Sharif, A., S. Afshan, and N. Nisha. 2017. “Impact of Tourism on CO2 Emission: Evidence from Pakistan.” Asia Pacific Journal of Tourism Research 22 (4): 408–421. https://doi.org/10.1080/10941665.2016.1273960.
- Sherafatian-Jahromi, R., M.S. Othman, S.H. Law, and N. W. Ismail. 2017. “Tourism and CO2 Emissions Nexus in Southeast Asia: New Evidence from Panel Estimation.” Environment, Development and Sustainability 19:1407–1423. https://doi.org/10.1007/s10668-016-9811-x.
- Sheraz, M., X. Deyi, J. Ahmed, S. Ullah, and A. Ullah. 2021. Moderating the effect of globalization on financial development, energy consumption, human capital, and carbon emissions: evidence from G20 countries.
- Shi, H., X. Li, H. Zhang, X. Liu, T. Li, and Z. Zhong. 2020. “Global Difference in the Relationships Between Tourism, Economic Growth, CO2 Emissions, and Primary Energy Consumption.” Current Issues in Tourism 23 (9): 1122–1137. https://doi.org/10.1080/13683500.2019.1588864.
- Solarin, S. A. 2014. “Tourist Arrivals and Macroeconomic Determinants of CO2 Emissions in Malaysia.” Anatolia. An International Journal of Tourism and Hospitality Research 25 (2): 228–241.
- Stein, D. I. 2018. “The Environmental Kuznets Curve.” In Reference Module in Earth Systems and Environmental Sciences. Elsevier. https://doi.org/10.1016/B978-0-12-409548-9.09278-2
- Sudharshan, R. P., SMd Alam, and C. K. M. Lau. 2018. “The Effect of Tourism Investment on Tourism Development and CO2 Emissions: Empirical Evidence from the EU Nations.” Journal of Sustainable Tourism 26 (9): 1587–1607. https://doi.org/10.1080/09669582.2018.1489398.
- UNEP. 2008. Climate Change Adaptation and Mitigation in the Tourism Sector: Frameworks, Tools and Practices. Paris Cedex, France: UNEP DTIE Sustainable Consumption and Production Branch Milan.
- Westerlund, J. 2007. “Testing for Error Correction in Panel Data*.” Oxford Bulletin of Economics and Statistics 69: 709–748. https://doi.org/10.1111/j.1468-0084.2007.00477.x.
- Zaman, K., M. A. Moemen, and T. Islam. 2017. “Dynamic Linkages Between Tourism Transportation Expenditures, Carbon Dioxide Emission, Energy Consumption and Growth Factors: Evidence from the Transition Economies.” Current Issues in Tourism 20 (16): 1720–1735. https://doi.org/10.1080/13683500.2015.1135107.