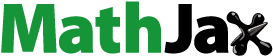
Abstract
We introduce a design that is able to face some of the challenges that digital news consumption is posing to traditional media effects methods like linkage analysis. The challenges include (1) memory errors and biases when reporting everyday news media consumption leading to (2) errors when linking mass media outlets to survey data; (3) personalization of media content, as well as (4) short-term dynamic processes. Mobile Intensive Longitudinal Linkage Analysis (MILLA) uses an innovative combination of smartphone data donations to capture media exposure and relevant media content, a mobile experience sampling questionnaire to capture immediate reactions to news, and the content analysis of uploaded news media content to measure media effects. The design is explained by using an example of negativity in the news and its effects on emotional reactions of recipients.
Advancing Method Summary
Method name: Mobile Intensive Longitudinal Linkage Analysis (MILLA)
Description of method: MILLA is combination of smartphone data donations (screenshots/photos) to capture relevant media content, a mobile experience sampling questionnaire to capture immediate reactions to news, and content analysis of uploaded news media content to measure effects of media content on recipients’ reactions.
Aim: It aims at measuring media effects in the digital media environment in a very fine-grained and dynamic way. MILLA is able to capture short-term dynamics of media reception and –effects – even within one day.
Data material: On the one hand, the screenshots and photos of relevant media content can be analysed by manual or automated content analysis on the other hand the design relies on self-report for immediate reactions to media content (longitudinal survey data).
Main contribution: The main contribution of the design is to overcome challenges of traditional linkage analysis, i.e. combining media content data with survey data to a personalized, dynamic, digital, hybrid media environment. Linkage analysis faces serious challenges when trying to assess effects of digital media because of personalized media content, biases and memory errors when trying to measure media consumption. Since MILLA is adaptive to the relevant and personalized media content participants receive, it is more suitable to capture digital news media effects and short-term dynamics of media selection and –effects.
Advantages: MILLA is a very fine-grained and adaptive design to measure media effects in a real-world environment. In contrast to traditional linkage analysis one is able to capture immediate reactions to specific media content (e.g. emotional reactions to negative news messages), in contrast to experimental designs, MILLA is able capture communication dynamics and longitudinal processes.
Challenges: Since the approach requires recipients to remember relevant reception situations and trigger the mobile surveys themselves, it requires a lot of diligence from the participants. MILLA is also potentially resource-heavy since participants need to make the data donations and fill the mobile surveys multiple times a day. Researcher certainly do not reach sample sizes of traditional linkage analysis.
Fields of application: Investigating immediate reactions to specific digital, potentially personalized content, topics, or pieces of news media and modelling short-term dynamics of news media usage and –effects.
Digitalization is changing our media landscape and the way we select and consume news. However, the methods repertoire of news media effects research was mostly built in an era of mass communication when there were relatively few news outlets, providing homogenous information to a larger audience. Especially linkage analysis, which is considered a “gold standard” in news media effects research, comes with the premise of mass communication since they combine panel surveys and measures of media exposure with content analysis of leading news outlets (De Vreese et al. Citation2017).
The basic idea of linkage studies is simple, yet powerful: Scholars link media content to attitudes, behaviour, emotions, knowledge, or other outcomes. More specifically, scholars measure (1) exposure to news and (2) the variable of interest – be it an attitude, public opinion, or behaviour – in a survey. Thereafter (3), they content analyse (leading) news media outlets and code media characteristics that are assumed to affect the variable of interest; finally (4), they link media content data to those participants that reported news media exposure. As we will show in this article, traditional linkage analysis is deeply connected to the era of mass communication and pose four challenges that could be tackled by a new linkage design – Mobile Intensive Longitudinal Linkage Analysis (MILLA). This special linkage design combines two sources of data: mobile experience sampling (mESM) data and content analysis of the exact media content participants received. The mESM design is used to measure (1) the exact and relevant online and offline media exposure by using smartphone data-donations, i.e. participants upload screenshots, photos, and links of the exact news items they received – be it print newspaper articles or online content. (2) Directly after media reception they fill out a short questionnaire (the experience sampling form) to assess the variables of interest. The media items participants uploaded with their smartphones can be (3) content analysed, and the characteristics of that media content can (4) be linked to the mobile questionnaires, similar to the initial idea of the linkage design.
Challenges and Blind Spots of Traditional Linkage Analysis
We now describe four challenges for the traditional linkage analysis approach. The first three challenges are tightly connected with the digitalization of communication leading to fragmented, hybrid, and personalized media landscape. The fourth challenge rather represents a blind spot of traditional linkage studies: short-term dynamics in news media effects research.
Challenge 1: Digital Communication and Measures of Media Exposure
First of all, news sources have increased dramatically in the last decades. Consequentially, measuring media consumption with traditional retrospective self-reports became an increasingly complex task (De Vreese and Neijens Citation2016). Despite valuable efforts to improve the traditional retrospective measure of media usage, the hybrid media system, the always online and always connected environment of digital news use, and fragmented media have increased biases in reporting how much news a recipient used and which sources were used (Naab, Karnowski, and Schlütz Citation2019; Pew Research Center Citation2019; Scharkow Citation2016). In a hybrid media system with blurring lines between sources of information and increasing news usage via social media, it has become almost impossible for recipients to distinguish, let alone memorize and report, sources of online information. Reporting news usage accurately is, however, a prerequisite of traditional linkage studies and biases lead to increasing measurement errors (Scharkow and Bachl Citation2017).
Challenge 2: Digital Communication and Content Analyses
Increasing media sources and fragmented media diets do not only complicate the measurement of news exposure but also the analysis of relevant media content. It has been relatively easy to assess and link media content to large scale surveys when there were only a couple of television news shows and a handful of major newspapers that were setting the agenda (McCombs Citation2005). However, methods and designs need to adapt to a media environment, in which the agenda-setting function of mass media seems to shrink and is heavily dependent on the circumstances, e.g. the salience of an issue or temporal context (Geiß Citation2019).
Challenge 3: Personalized Media Content
A further challenge to traditional linkage analyses that comes with media digitalization is the personalization of media content (Bol et al. Citation2018). If news agendas and content differ from recipient to recipient it is impossible to conduct traditional linkage studies since they are based on the idea that each news outlet provides more or less the same information to all its users.
Challenge 4: Communication Dynamics
Many linkage studies are not only concerned with snapshots and the contemporaneous relationship of recipients’ attitudes, beliefs, or behaviour and news consumption but rather with developments or dynamics of news media effects. However, the traditional designs are (1) bound by the time-lags of largescale panels – usually weeks or months – and (2) variables that are not directly linked to the media content, i.e. general attitudes, opinions or behaviours. When it comes to short-term dynamics, e.g. the relationship between certain emotions and attention towards political news (Otto et al. Citation2020), traditional linkage studies should not be the method of choice. The same holds true for variables that are directly linked to a specific news item – be it a credibility judgement (Otto, Thomas, and Maier Citation2018) or obtained media gratifications (Wang, Tchernev, and Solloway Citation2012). These processes and reactions were, up until now, only assessable via experimental designs that do not account for communication dynamics. MILLA is especially suitable for short-term dynamic processes and fast-lived variables. While this blind spot is not necessarily tied to online communication, digitalization has increased short-term dynamics in communication.
Challenge 5: Making Sense of Audience Reactions – MILLA and the Audience Turn
In the last two decades, the audience has gained more attention in journalism, which is described as the “audience turn” in journalism studies. Technical developments and digitalization as described above have facilitated the interaction between journalists and the audience (Loosen and Schmidt Citation2012). On the side of journalism researchers, the audience turn comes with an individual-level, psychological perspective, which is not captured best by more macro-oriented linkage studies, but call for individual level research designs such as experiments, quasi-experiments, or factorial survey designs (Otto and Glogger Citation2020; Wahl-Jorgensen Citation2020). MILLA is a radical realization of the audience turn since it puts the perceptions and activities of the individual recipient in the focus of research – it takes the audience perspective seriously (see also Lecheler Citation2020).
Summing up these challenges in a provocative statement, one could say that a linkage analysis was perfectly suitable for the 20th century concept of mass communication, when there was a limited amount of media sources with few leading channels, newspapers, and magazines and media content was the same for a large audience, and dynamic processes developed rather over days, weeks, and months than over short-term. The “end of mass communication” (Chaffee and Metzger Citation2001, 369) as well as the focus on journalism studies on the audience make it necessary to update study designs for news media effects.
Beyond Traditional Linkage Studies: Linking Survey and Digital Trace Data
One could argue that the combination of surveys and digital trace data, i.e. linking online media tracking and traditional questionnaire self-reports is able to overcome some of the challenges presented here. After all, digital trace data are much more objective, does not rely on participants’ memory performance and is, thus, not prone to the biases that are presented in Challenge 1 and 2 (see e.g. Stier et al. Citation2020). However, of course, these data are (a) limited to online media and not able to capture the interplay of offline and online communication environments. (b) Digital trace studies are only able to measure immediate reactions to media content if the user actively engages and leaves a digital trace (e.g. liking, commenting, clicking). In a similar vein, the survey data come with similar weaknesses: it is impossible to capture immediate responses as well as variables that are specific to a certain media content (e.g. “How trustworthy is the Tweet you just read?”) MILLA provides the opportunity to get more fine-grained reactions to the exact media content that participants just received and, thus, more detailed reactions. Finally, (c) web-tracking approaches face the problem of closed, proprietary social media platforms, where the providers block attempts from web-tracking tools to gather data (Menchen‐Trevino Citation2013). Since participants in a MILLA design upload screenshots and media content themselves, these data-donations allow the researcher to be independent from data access from social media companies (see also Ohme et al. Citation2020). In sum, MILLA is not only able to face some of the problems that traditional linkage studies have, but also some of the weaknesses of newer, computational approaches. Finally, as we will show later, combining experience sampling and other digital data provide opportunities that go beyond the analysis that will be presented here.
Mobile Intensive Longitudinal Linkage Analysis
MILLA is able to face the aforementioned challenges by combining a fine-grained panel design – the mobile experience sampling method (mESM) – with a longitudinal and individual content analysis of the exact media items the recipients used and uploaded. The design makes use of three sources of data – (1) mobile experience sampling (mESM) to asses recipients’ immediate reactions to news content;(2) data donations, i.e. screenshots, photos or links of the news item the recipient just used; and (3) a content analysis of this media content (see ).
Figure 1. Schematic depiction of a longitudinal linkage analysis of three consecutive points of measurement (t). Note. Number of occasions and time intervals between each occasion might differ across individuals depending on when they fill out a questionnaire/receive a media message.
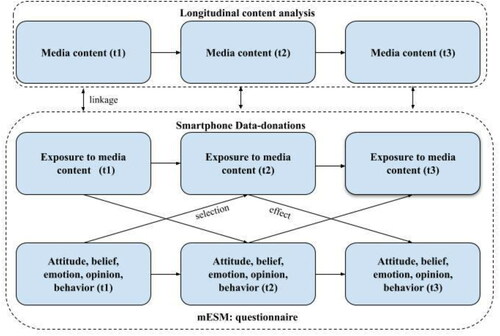
The crucial feature of experience sampling is the idea of measuring a variable in-situ, that means, almost in real-time and close to the situation of appearance and multiple times a day, after being alerted by a random or fixed signal or by an event (Kubey, Larson, and Csikszentmihalyi Citation1996; Naab, Karnowski, and Schlütz Citation2019). Using this intensive longitudinal design allows to face the challenges mentioned above: In-situ measurements are close to the reception situation and, thus, diminish memory errors, providing more precise measures of media exposure (Greenberg et al. Citation2005; Naab, Karnowski, and Schlütz Citation2019). The data-donations provide us the exact, relevant, and individual media content, which can be content analysed and linked to the experience sampling questionnaire, similar to a traditional linkage design.
The Example: The Dynamic Relationship between News Framing and Emotional States
To illustrate the procedure for MILLA, we use a traditional question of communication research as an example, namely the relationship between specific content features of political news and emotional reactions. The body of research on the relationship between negativity and emotional reactions is large (e.g. Lecheler, Bos, and Vliegenthart Citation2015; Uribe and Gunter Citation2007) and as an example, it allows us to illustrate that MILLA can be a powerful tool in the methods repertoire of communication and journalism scholars.
We explicate a study conducted in April, 2016. The assumptions are straightforward but perfectly suited to show how media content and reactions to this content can be measured and analysed by means of MILLA. We attempt to investigate whether negative political news, i.e. news that focus on political failures, scandals, and problems affect recipients’ immediate emotional reactions. Based on recent research (e.g. Lecheler, Bos, and Vliegenthart Citation2015), we expect that negative tone affect emotional reactions, i.e. anger, fear, and joy (H1). Moreover, we expect dynamics in emotional reactions: Lagged negative news characteristics affect subsequent emotional reactions (H2) (Otto et al. Citation2020). Such fast-lived dynamics could hardly be tested with traditional linkage analyses or traditional experimental approaches.
Step 1: Capturing Media Exposure and Received Media Content with Data-Donations
When conducting a mESM study, one can distinguish time-contingent and signal-contingent designs from event-contingent designs. In time-contingent studies, participants fill out the experience sampling form after a pre-defined interval (e.g. two hours); in signal-dependent designs, participants are alerted randomly on a mobile device to fill out a questionnaire and then get asked about their activities (e.g. media usage) within the last hours. Event-dependent approaches, in contrast, use specific events or situations to trigger the assessment, e.g. every time a participant sees a politician in the media or every time the participant uses a certain app on the Smartphone. This design is recommended for MILLA; after all, participants need to upload the received news item, which is only possible directly after media exposure. Following this approach, participants in this study (N = 96) were asked to upload the media item (by taking a photo or screenshot) and fill the experience sampling form every time they perceived a media item (newspaper articles or articles on the Internet) that contained information about a politician.Footnote1
Step 2: Capturing Immediate Reactions to Media Exposure with Mobile Experience Sampling
In the short questionnaires that followed each contact with a political news item, we asked the participants about how they felt while watching or reading the news item.Footnote2 We assessed emotions with a single item asking how angry (M = 2.78, SD = 1.61), fearful (M = 1.93, SD = 1.16), and happy (M = 2.33, SD = 1.29) the participants felt while reading the article. All emotions were measured on a 6-point Likert scale ranging from 1 (did not feel … at all) to 6 (felt very …).Footnote3
Step 3: Content Analysis of Exact Media Content
Since participants uploaded (screen-)shots and links we were able to exactly analyse the media content participants used.Footnote4
For the purpose of this article, we focus on text-based media items (print newspaper and online media) only, leaving 447 media items for our further analyses (see , Table A1).Footnote5 For this study, we were especially interested in the negativity of a news item. Following Lengauer, Esser, and Berganza (Citation2012), we measured negativity with two items: (1) The level of negative tonality indicated whether the news item gave an overall positive, negative, neutral (no tone), or mixed impression of politics. (2) The individual tonality measured the level of a negative or positive tone towards political actors or organizations. It was coded as predominately negative, for example, when the news item comprised mistrust, accusation of incompetence, or negative traits of an individual actor; as predominately positive when the item contained indicators like compliments, portrayals of merit, or positive traits of an individual actor. We built a mean score for both variables reaching from 0 (minimum) to 2 (maximum) (M = 0.81, SD = 0.69). After four and five training sessions, respectively, the three coders reached high inter-coder agreement (negative tonality: Holsti = .85, individual tonality: Holsti = .86).
Table 1. Number of respondents, occasions, and media items.
Step 4: Linking mESM Data and Content Analysis
We restructured the survey data to long format. Therefore, each occasion for each participant is a single case. Similarly, for the long format content analysis dataset, every media item is a single case. We assigned a unique ID to each item in both datasets. Based on the ID, we transferred the media content to the survey data.
Step 5: Analysing Intensive Longitudinal Data
mESM data combined with content analysis data has two important benefits when linking media content to attitudes or behaviours. First, it shows a hierarchical data structure with measurement occasions nested within participants capturing both intra- and inter-individual effects and therefore allowing to analyse media effects as within-person processes. Second, mESM data allows to model media effects whenever they appear in a natural reception situation for each individual by taking into account continuous time, whereas data in traditional linkage analysis is usually aggregated to specific occasions like days or weeks (discrete time). In other words, MILLA is not only more detailed, when it comes to the measurement of specific media content, it also provides the opportunity to gather fine-grained data about the time of media reception. This can be of great value for researchers who have assumptions about the specific times of media reception (e.g. before going to bed) or about specific time lags. In order to account for these characteristics, several statistical approaches can be used (e.g. Hamaker et al. Citation2018). For our empirical example, we are especially interested in estimating within-person relations. This can be done with multilevel-models centreing the level-1 predictors (Curran and Bauer Citation2011). A multilevel-model to determine the effects of negativity on negative and positive emotions can be written in a combined form as
with
representing the emotional reaction to the media item at time t for respondent i, where t refers to the time when individuals fill out a questionnaire. Therefore, the time intervals between occasions might differ between individuals. Coefficients
(autoregressive effect from the emotional reaction on subsequent emotional reactions),
(contemporaneous effect at the same time), and
(cross-lagged effect from negativity on subsequent emotional reactions) represent effects from time-variant level-1 variables. Of course, the researcher is able to control for different time lags or even treat the time lag between measurement occasions as a moderator for media effects. In contrast, the coefficients
and
represent effects from time-invariant level-2 variables that do not change over the course of the study. Coefficient
represents individuals’ deviation from the intercept (
) that represents an average of individuals’ mean levels in emotional reactions across time. Thus, the model can be considered as a mixed effects model in which
represents the random coefficient.
Emotional Reactions to News: Example Results
The results explicate how to answer substantive questions in news media effects research as well as facing challenges (especially challenge 4) that were mentioned above. There is a small autoregressive effect of fear (γ10 = −0.11, SE = 0.05, p = .041), but neither for other emotional reactions, nor did the emotional reactions in one time point affect emotions at a subsequent time point (see ). We found a contemporaneous effect (γ20 = 0.75, SE = 0.21, p < .001) and a lagged effect (γ30 = 0.61, SE = 0.21, p = .003) of negativity on anger. That means negativity in a specific news item does not only affect the immediate emotional reaction after reading a particular article but also does lead to higher than expected levels of anger at subsequent times. In other words, some of the effects of negativity “carry over” to subsequent news reception occasions. Again, a result that hardly can be found with any other design in communication research.
Table 2. Fixed effects on emotional reactions (media effects).
The time-invariant level-2 predictors showed no effects with one exception. Participants with higher levels of education showed lower levels of fear (γ03 = −0.61, SE = 0.23, p = .012) supporting earlier notions of citizens with high political efficacy and sophistication being less prone to fear reactions towards political information (Valentino, Gregorowicz, and Groenendyk Citation2009).
Added Value of MILLA: Tackling the Challenges of Research on Digital News Usage
In this article and the exemplary study presented above, we show a new way of linking media content data and panel surveys to investigate news media effects. MILLA comprises the idea to use data-donations from participants, i.e. screenshots, photos, as well as links, and to a content analysis of these news items with smartphone surveys. This approach is able to face some of the challenges that communication research in general and linkage studies in particular face when analysing digital news media effects.
Challenge 1 and 2: Measuring Media Exposure and Analyzing Relevant News Content
The idea of measuring media exposure with mESM is straightforward: if one of the problems of exposure measures are memory errors causing biases in both the amount of time spend on the media and the sources used (Scharkow Citation2016), measuring media usage directly after or closer to the reception should curb this source of error. By donating screenshots, photos, and links, the researcher is able to extract the information about the media sources that is relevant to the research question at hand. Moreover, it is possible to gain access to the exact media content recipients received, and the researcher is able to circumvent barriers of social media companies to access media content.
Challenge 3: Personalized Media Content
MILLA takes into account recipients’ individual and possibly personalized news agenda. In traditional linkage analyses, only a sample of news items can be analysed and, thus, there is a gap between analysed news content and the items that recipients really used no matter how tailored and fragmented a news diet might be.
Challenge 4: Short-Term Dynamics in News Consumption
mESM is not only especially suitable to measure media exposure but also to capture immediate reactions to media content. Since the surveys are completed in-situ, i.e. Directly after media exposure, it is possible to measure variables that are very fast-lived and/or linked to the specific media content. We showed that negativity does not only affect negative emotions such as anger at the same measurement occasion but also the effect can spill over to the next news reception. To date, it was only possible to measure immediate reactions to news media within experimental settings that, however, do not account for dynamic processes (Feldman et al. Citation2013). While our exemplary study was concerned with media effects, MILLA would be also suitable for studies on news selection (see also ).
Challenge 5: Focus on the Audience
As we show here, MILLA perfectly represents the audience turn in journalism research. It puts the individual recipient and their reactions in the focus. Taking into account the example here, it also contributes to the “emotional turn” in journalism studies (Wahl-Jorgensen Citation2020). Similar to the audience turn, the focus of attention is on the recipient and obviously on affective processes (Lecheler Citation2020). The recipient in general and fast-lived (affective) processes in particular are the main point of interest for MILLA studies; the design, thus, perfectly represents the audience turn in journalism (research).
These considerations show that MILLA is able to fill a gap between experimental designs and traditional linkage designs. Experimental designs are able to capture immediate reactions to complex and very specific media content but come with a threat towards external validity due to artificial stimuli, reception environments, and forced exposure. Experiments, furthermore, are not able to assess dynamic processes as described above. Linkage designs, in contrast, can model dynamics and do not come with the disadvantages of an artificial reception situation but are not able to capture immediate reactions, fast-lived variables, and the effects of specific media items – only to name a few weaknesses here. Finally, MILLA complements online tracking methods that do not rely on self-report of media usage and might be suitable to measure online media usage as a whole. However, they are, of course, limited to digital communication and come with further constraints and obstacles when trying to assess social media content.
Challenges and Pitfalls of MILLA
Of course, the MILLA design also comes with weaknesses. The researcher still relies heavily on self-report, both for the (relevant) media consumption and the variable of interest. While the in-situ measurement of both should decrease biases and memory errors the limitations of self-report and questionnaires still apply in contrast to tracking studies and digital trace data.
MILLA can be very demanding for participants. Filling out questionnaires, allowing researchers to assess screenshots and photos, as well as understanding and keeping in mind the instructions for the mobile questionnaire put a high demand on participants’ time resources, as well as cognitive abilities. Our experiences with asking participants to donate data was very positive, i.e. none of the participants refused to upload screenshots or photos and there are no technical skills necessary to take part in such a study besides filling questionnaires and taking screenshots. The biggest challenge on the side of the participants is staying alerted to potential events and triggers that call for action on their side. Thus, the researcher is highly dependent on the commitment and diligence of the participants. Since we have no control over the reception situation, it is impossible to identify whether participants have completed the procedure for every relevant situation – quite on the contrary it is likely that we are only dealing with a sample of potential news reception situations.Footnote6 Against this backdrop, MILLA might be particularly suitable for very specific media content, e.g. participants seeing reading information about a specific politician or news on a certain topic; in that case, the number of potential data donations and relevant media content would decrease and the study is not as demanding for participants. For general news media consumption, the design might not catch all the occasions. However, MILLA should still be more precise than traditional survey studies.
Research on mESM has identified a few factors that contribute to data quality: The higher participants’ commitment to the study, the better the data and the fewer measurement occasions are missed out. Short scales and questionnaires as well as monetary incentives, of course, contribute to high participant motivation (Fahrenberg et al. Citation2007). Of course, depending on the design of the study and topic, there might be privacy issues, e.g. when uploading names and photos of social media users – especially for individuals that do not take part in the study and do not give consent to the procedure. However, there are innovative ways to delete or prevent from using unnecessary data, e.g. by using automated blurring software.
Adding to the high demand on participants, the MILLA design could also be resource-intensive. It is almost impossible to draw bigger samples. However, while relying on smaller samples and thus “loosing” between-subject variance, MILLA comes with the advantage of potentially high within-subject variance due to many measurement points, which is crucial for statistical power in intensive longitudinal designs (Bell et al. Citation2014).
Conclusion
The example and description here can be interpreted as one way of conducting MILLA and combining media content with in-situ measurements. The possibilities to adapt the design are manifold: On the one hand, since media content is already digitalized, it would be worthwhile to combine the experience sampling data with automated content analysis. Scholars might use innovative extensions to the approach by adding GPS or physiological data, e.g. by providing participants with wearables (Pejovic et al. Citation2016). Moreover, combinations with web-tracking and other digital trace data would be valuable. While MILLA provides an immediate reaction to a specific news item, digital trace data could be used to (a) capture participants general media environment and (b) to assess other reactions on online and social media (e.g. clicking a link, liking, commenting etc.). This combination could lead to a very detailed (MILLA), but also comprehensive (digital trace data) picture of participants’ media environment.
In sum, MILLA provides a useful extension of the toolbox of communication researchers who are interested in news media effects. The strengths mentioned above, i.e. the adaption to personalized media, diverse and fragmented media landscape, and a hybrid and dynamic media system, make the method suitable to investigate digital news consumption of individual recipients. While the method and design are certainly not yet common in communication research, we hope that this introduction helps scholars to face some of the challenges that we describe above and to analyse and describe recipients’ communication reality in more detail than before.
Disclosure Statement
No potential conflict of interest was reported by the author(s).
Notes
1 All participants downloaded a smartphone app called MovisensXS version 0.7.4162 (movisens GmbH, Karlsruhe, Germany). Participants got an introduction to the app, and we provided a manual that explained its features.
2 Participants filled these questionnaires on multiple occasions per day for eight consecutive days. All information was immediately uploaded to a server as soon as participants got a connection to the Internet. Participants filled on average M = 8.9 questionnaires (SD = 5.8; min = 2; max = 26).
3 We assessed age (M = 34.72, SD = 14.96), gender (68.5% female), education (53.7% with highest educational degree), and political interest (M = 4.05, SD = 1.03) as control variables. Political interest was measured with five items on a 6-point scale (Cronbach’s α = .91).
4 When looking at the material, we had to exclude, however, 90 articles due to poor quality of the photos that rendered them impossible to be read. Moreover, sometimes respondents reported the media channel without uploading a picture that made the media items unidentifiable.
5 TV shows are hard to analyze by means of MILLA. This is due to the fact that many if not all news shows contain several shorter clips or stories and it is, of course, not possible to fill a questionnaire after each of these clips. For the purpose of this paper, we, therefore, decided not to take into account news shows.
6 Of course, automatic triggers could circumvent this problem. If the experience sampling procedure is triggered automatically, e.g. every time a participant closes a certain app on the smartphone (Bayer et al. Citation2018) this limitation becomes obsolete. However, the applications for these automatic triggers seem to be limited and also highly depending on the technical circumstances of a platform, app, or homepage.
References
- Bayer, J., N. Ellison, S. Schoenebeck, E. Brady, and E. B. Falk. 2018. “Facebook in Context(s): Measuring Emotional Responses across Time and Space.” New Media & Society 20 (3): 1047–1067.
- Bell, B. A., G. B. Morgan, J. A. Schoeneberger, J. D. Kromrey, and J. M. Ferron. 2014. “How Low Can You Go?” Methodology 10 (1): 1–11.
- Bol, N., T. Dienlin, M. Sax, S. Kruikemeier, S. C. Boerman, J. Strycharz, N. Helberger, and C. H. De Vreese. 2018. “Understanding the Effects of Personalization as a Privacy Calculus: Analyzing Self-Disclosure across Health, News, and Commerce Contexts.” Journal of Computer-Mediated Communication 23 (6): 370–388.
- Chaffee, S. H., and M. J. Metzger. 2001. “The End of Mass Communication?” Mass Communication and Society 4 (4): 365–379.
- Curran, P. J., and D. J. Bauer. 2011. “The Disaggregation of within-Person and between-Person Effects in Longitudinal Models of Change.” Annual Review of Psychology 62: 583–619..
- De Vreese, C. H., M. Boukes, A. Schuck, R. Vliegenthart, L. Bos, and Y. Lelkes. 2017. “Linking Survey and Media Content Data: Opportunities, Considerations, and Pitfalls.” Communication Methods and Measures 11 (4): 221–244.
- De Vreese, C. H., and P. Neijens. 2016. “Measuring Media Exposure in a Changing Communications Environment.” Communication Methods and Measures 10 (2-3): 69–80.
- Fahrenberg, J., M. Myrtek, K. Pawlik, and M. Perrez. 2007. “Ambulatory Assessment—Monitoring Behavior in Daily Life Settings.” European Journal of Psychological Assessment 23 (4): 206–213.
- Feldman, L., N. J. Stroud, B. Bimber, and M. Wojcieszak. 2013. “Assessing Selective Exposure in Experiments: The Implications of Different Methodological Choices.” Communication Methods and Measures 7 (3-4): 172–194.
- Geiß, S. 2019. “The Media’s Conditional Agenda-Setting Power: How Baselines and Spikes of Issue Salience Affect Likelihood and Strength of Agenda-Setting.” Communication Research: 009365021987496. doi:https://doi.org/10.1177/0093650219874968.
- Greenberg, B. S., M. S. Eastin, P. Skalski, L. Cooper, M. Levy, and K. Lachlan. 2005. “Comparing Survey and Diary Measures of Internet and Traditional Media Use.” Communication Reports 18 (1-2): 1–8.
- Hamaker, E. L., T. Asparouhov, A. Brose, F. Schmiedek, and B. Muthén. 2018. “At the Frontiers of Modeling Intensive Longitudinal Data: Dynamic Structural Equation Models for the Affective Measurements from the COGITO Study.” Multivariate Behavioral Research 53 (6): 820–841.
- Kubey, R., R. Larson, and M. Csikszentmihalyi. 1996. “Experience Sampling Method Applications to Communication Research Questions.” Journal of Communication 46 (2): 99–120.
- Lecheler, S. 2020. “The Emotional Turn in Journalism Needs to Be about Audience Perceptions.” Digital Journalism 8 (2): 287–291.
- Lecheler, S., L. Bos, and R. Vliegenthart. 2015. “The Mediating Role of Emotions: News Framing Effects on Opinions about Immigration.” Journalism & Mass Communication Quarterly 92 (4): 812–838.
- Lengauer, G., F. Esser, and R. Berganza. 2012. “Negativity in Political News: A Review of Concepts, Operationalizations and Key Findings.” Journalism: Theory, Practice & Criticism 13 (2): 179–202.
- Loosen, W., and J.-H. Schmidt. 2012. “(Re-)Discovering the Audience.” Information, Communication & Society 15 (6): 867–887.
- McCombs, M. 2005. “A Look at Agenda-Setting: Past, Present and Future.” Journalism Studies 6 (4): 543–557.
- Menchen‐Trevino. E. 2013. “Collecting Vertical Trace Data: Big Possibilities and Big Challenges for Multi-Method Research.” Policy & Internet 5 (3): 328–339.
- Naab, T. K., V. Karnowski, and D. Schlütz. 2019. “Reporting Mobile Social Media Use: How Survey and Experience Sampling Measures Differ.” Communication Methods and Measures 13 (2): 126–122.
- Ohme, J., T. Araujo, C. H. de Vreese, and J. T. Piotrowski. 2020. “Mobile Data Donations: Assessing Self-Report Accuracy and Sample Biases with the iOS Screen Time Function.” Mobile Media & Communication. doi:https://doi.org/10.1177/2050157920959106.
- Otto, L. P., and I. Glogger.2020. “Expanding the Methodological Toolbox: Factorial Surveys in Journalism Research.” Journalism Studies 21: 947–965.
- Otto, L. P., F. Thomas, and M. Maier. 2018. “Everyday Dynamics of Media Skepticism and Credibility: An Ambulatory Assessment Study.” In Trust in Media and Journalism. Empirical Perspectives on Ethics, Norms, Impacts and Populism in Europe, edited by K. Otto and A. Köhler, 111–133. Wiesbaden, Germany: Springer VS.
- Otto, L. P., F. Thomas, M. Maier, and C. Ottenstein. 2020. “Only One Moment in Time? Investigating the Dynamic Relationship of Emotions and Attention toward Political Information with Mobile Experience Sampling.” Communication Research 47 (8): 1131–1154.
- Pejovic, V., N. Lathia, C. Mascolo, and M. Musolesi. 2016. “Mobile-Based Experience Sampling for Behaviour Research BT.” In Emotions and Personality in Personalized Services: Models, Evaluation and Applications, edited by M. Tkalčič, B. De Carolis, M. de Gemmis, A. Odić, and A. Košir, 141–161. Basel, Switzerland: Springer International Publishing.
- Pew Research Center. 2019. “Social Media Outpaces Print Newspapers in the U.S. as a News Source.” https://www.pewresearch.org/fact-tank/2018/12/10/social-media-outpaces-print-newspapers-in-the-u-s-as-a-news-source/
- Scharkow, M. 2016. “The Accuracy of Self-Reported Internet Use—A Validation Study Using Client Log Data.” Communication Methods and Measures 10 (1): 13–27.
- Scharkow, M., and M. Bachl. 2017. “How Measurement Error in Content Analysis and Self-Reported Media Use Leads to Minimal Media Effect Findings in Linkage Analyses: A Simulation Study.” Political Communication 34 (3): 323–343.
- Stier, S., J. Breuer, P. Siegers, and K. Thorson. 2020. “Integrating Survey Data and Digital Trace Data: Key Issues in Developing an Emerging Field.” Social Science Computer Review 38 (5): 503–516.
- Uribe, R., and B. Gunter. 2007. “Are `Sensational’ News Stories More Likely to Trigger Viewers’ Emotions than Non-Sensational News Stories?: A Content Analysis of British TV News.” European Journal of Communication 22 (2): 207–228.
- Valentino, N. A., K. Gregorowicz, and E. W. Groenendyk. 2009. “Efficacy, Emotions and the Habit of Participation.” Political Behavior 31 (3): 307–330.
- Wahl-Jorgensen, K. 2020. “An Emotional Turn in Journalism Studies?” Digital Journalism 8 (2): 175–194.
- Wang, Z., J. M. Tchernev, and T. Solloway. 2012. “A Dynamic Longitudinal Examination of Social Media Use, Needs, and Gratifications among College Students.” Computers in Human Behavior 28 (5): 1829–1839.
Appendix A – Measurement Occasions
Table A1. Frequency of measurement occasions.