Abstract
Fact-checking databases, as important results of fact checkers’ epistemic work, are increasingly tied together in new overarching infrastructures, but these are understudied and lack transparency despite being an important societal baseline for whether claims are false. This article conceptualizes fact-checking as infrastructure and constructs a mixed-methods approach to examine overlaps and differences and thereby detect biases to increase transparency in COVID-19 misinformation infrastructure at scale. Analyzing Poynter and Google as such overarching infrastructures, we found only a small overlap. Fewer fact-checkers contribute to Google, with fewer stories than to Poynter. 75% of claims in Google are fact-checked by Asian and North American fact-checkers (44% for Poynter) but none by South Americans (20% for Poynter). More stories in Poynter originate from Facebook than outside social media (43% vs. 17%), while Google shows the opposite (16% vs. 38%). In Google, claims originate to a larger extent from public persons. We find similar large topics on “statistics” and “cures,” but also differences regarding smaller topics (e.g., “vaccines”) and types of misinformation (e.g., “virus characteristics”). Thus, the article shows that the infrastructures have inherent biases and argue that making visible such biases will increase transparency for stakeholders using it.
Introduction
With a gaining influence of digital media on everyday life during the pandemic, online misinformation has been a focal point for health institutions and governments alike. Fact-checking organizations and platforms are trying to catch up with false stories circulating in digital media networks by advancing fact-checking methods and creating databases for COVID-19 related misinformation stories. Here, especially collaborative approaches of fact-checking organizations have expanded, resulting for example in large digital fact-checking infrastructures made possible by for instance the International Fact-Checking Network (IFCN). The aim of this article is to conceptualize and analyze content in such overarching fact-checking infrastructures that we argue are important but understudied at scale in digital journalism research.
Within the last decades, fact-checking has become a new genre within journalism, with especially political fact-checking organizations aiming at educating the public, improving political behavior and journalism itself (Amazeen Citation2020; Graves and Anderson Citation2020). During the COVID-19 pandemic, health-related misinformation is the focal point of fact-checkers and fact-checked information is used, e.g., by data scientist to distinguish reliable from unreliable claims and news sources (Ball and Maxmen Citation2020). With regard to the COVID-19 pandemic, as during other health crises, media and journalists face specific expectations to report accurately without aggravating fear or panic, which easily result in rumors and other false information (e.g., Klemm, Das, and Hartmann Citation2019; Thomas and Senkpeni Citation2020).
The main research question of this article is twofold: how can fact-checking as overarching digital infrastructures be conceptualized and studied and what are the COVID-19 misinformation overlaps, differences and inherent biases of such infrastructures? By asking this question the article contributes to a better conceptualization and systematic analysis of fact-checking as infrastructures and provides empirical insights into COVID-19 misinformation infrastructures that can be point of departure for larger discussions on transparency as fact-checking ideal on infrastructure level. According to Singer (Citation2019), transparency is one of the main goals of fact-checking service providers and addresses methods as well as data as a means to establish trust (Shawcross Citation2016). Analyzing and visualizing overlaps and differences, and concluding on inherent biases is an additional entry point to approach the ideal of fact-checking transparency empirically on infrastructure level and discuss what it means to be transparent at this level. So far, very little documentation has been made of the underlying knowledge fact-checking databases contain (Lim Citation2018) and how they, for instance, epistemologically understand and classify misinformation (Graves, Nyhan, and Reifler Citation2016; Kalsnes Citation2018; Tandoc, Lim, and Ling Citation2018). This leaves fact-checking a black-boxed endeavor (Pasquale Citation2015) and this lack of transparency, we argue, is to some extent transferred to the overarching digital infrastructures, which collect fact-checked stories from various fact-checking organizations and make them accessible.
Existing academic studies have relied on various repositories of fact-checked stories as classifiers and their ability to detect, especially, political disinformation in the light of campaigns such as Brexit and U.S. president-elect Donald Trump (Allcott and Gentzkow Citation2017; Pierri, Artoni, and Ceri Citation2020; Shin et al. Citation2018; Vosoughi, Roy, and Aral Citation2018). Few studies have tested single fact-checker databases against each other to understand overlaps (Lim Citation2018), but to our knowledge not important overarching digital infrastructures that collect from many fact-checkers. Focusing on COVID-19 related misinformation, this article designs and carries out such tests and aims to measure the overlaps, but also differences that exist.
To do so, the article focuses on which information these overarching infrastructures of fact-checking provide and asks: 1) to what extent do two infrastructures overlap with regard to included stories and ratings, 2) who fact-checked the information, 3) where was the misinformation published? 4) who published the misinformation, and 5) which content was published? Using accounts of existing COVID-19 misinformation infrastructures from disinfo.euFootnote1, we selected and compared Poynter and Google Fact-check Explorer (referred to as “Google” onwards) for an in-depth comparison. These infrastructures and associated data were chosen, because they are some of the largest overarching infrastructures containing COVID-19 misinformation and differ in terms of funding. This could potentially influence how COVID-19 misinformation is handled – with one funded by the private company Google and the other financed through 18 funding bodies (mostly private foundations but also Facebook and Google News Initiative).Footnote2 COVID-19 misinformation as a case study is unique as it allows us to understand and detect infrastructure challenges at an international scale compared to, for instance, national political events.
Hence, the article contributes with in-depth empirical knowledge of overlaps and differences in COVID-19 misinformation in two large infrastructures, and in doing so helps to disclose their limitations and characteristics. This, we argue, contributes to the field of digital journalism and the subfield of journalistic epistemic work related to misinformation (see Ekström, Lewis, and Westlund Citation2020) with a different perspective on the results of the work at scale. This in turn can benefit researchers from fields studying misinformation as well as citizens and stakeholders that use such infrastructures as baseline sources for information and politics.
Theoretical Framework
Fact-Checking in Journalism and Transparency as an Ideal
Our conceptualization of misinformation in this article is empirically driven, closely tied to misinformation defined and listed by fact-checking organizations as entries in the databases. It is also based on studies, which developed careful conceptualizations of mis- and disinformation and the contested and politically oriented term “fake news” (Bechmann and O’Loughlin Citation2020; Gelfert Citation2018; Kalsnes Citation2018; Tandoc, Lim, and Ling Citation2018; Vargo, Guo, and Amazeen Citation2018; Wardle and Derakhshan Citation2017). A conceptualization that cuts across different stakeholders can be found in Buning et al. (Citation2018), who characterizes disinformation as “false, inaccurate, or misleading information designed, presented and promoted to intentionally cause public harm or for profit.” However, misinformation in comparison to disinformation is not necessarily created with the intention of causing harm or exerting influence (e.g., Carmi et al. Citation2020). Due to our empirically driven conceptualization, we do not distinguish between misinformation and disinformation but look at both independent of intentionality.
Especially in journalism, online fact-checking tools (Graves Citation2018) have become important in the fight against misinformation that spreads quickly in social media and beyond. According to Graves (Citation2018) fact-checking is characterized by the intention to increase knowledge through identifying purported facts based on research, expressed in statements from politicians and other persons that impact others’ lives. Fact-checking is furthermore ideally characterized by the absence of partisanship, advocacy and rhetoric. It is strongly rooted in journalism as many fact-checkers are to some extent affiliated with a news organization or have journalists working for them (Graves Citation2018; Singer Citation2019). The objectives of fact-checking organizations can be described as “informing the public, improving political rhetoric, and influencing other journalists” (Vargo, Guo, and Amazeen Citation2018, 2033). Beyond this, some fact-checking organizations also aim at holding publishers of disinformation accountable for their actions (Dias and Sippitt Citation2020).
Two leading ideals of fact-checkers are independence and transparency (Singer Citation2019). Koliska (Citation2021) suggests transparency as a means to counter challenges of declining trust in news media and summarizes many ways in which transparency can be understood within journalism, e.g., as a moral principle, a journalistic practice or a methodology and refers to the idea that “transparency in journalism aims to provide more information about hidden processes, rationales, and influences that impact news production” (Koliska Citation2021, 2). While Humprecht (Citation2020) compares different fact-checking organizations with regard to source transparency, our analysis of fact-checking infrastructures allows for an understanding of how content overlaps and differences on several dimensions might indicate inherent biases that when accounted for can be a way to “provide more information” of otherwise hidden structures in the quest to enhance transparency as an ideal.
Misinformation not only refer to claims, it also alters “previously established notions of what a trustworthy source is” (Steensen Citation2019, 186). With their ideals, fact-checkers directly address epistemological issues as they want to reveal unsupported statements and categorize their veracity, also in relation to source credibility. This approach is challenged in many ways. As Uscinski and Butler (Citation2013) point out, the assessment of a statements’ veracity often is restrained by its ambiguity and complexity or by the defined benchmarks. Furthermore, the approach itself is selective as fact-checkers decide what they check. This is especially challenging in what Waisbord (Citation2018, 1868) describes as “a crowded landscape of information flows.” The fact that journalism relies on diverse epistemologies adds to fact-checking probably being insufficient for facing “new challenges for public communication” associated with this complex digital environment (Waisbord Citation2018, 1874; cp. also Ekström, Lewis, and Westlund Citation2020). Furthermore, fact-checkers rely to some extent on external experts for their assessments despite lacking the ability to assess these experts (Godler, Reich, and Miller, Citation2020). In light of these challenges and in the Scandinavian tradition of source criticism (Steensen Citation2019), it is important to understand fact-checking as digital infrastructures used by researchers, citizens, journalists or politicians alike (e.g., Uscinski and Butler Citation2013). This article contributes to that aim.
Fact-Checking as Digital Infrastructure
To conceptualize fact-checking as digital infrastructure, we distinguish between databases of single fact-checkers and overarching digital fact-checking infrastructures and due to its understudied character focus on the latter.
Fact-checking organizations produce fact-checks that “feature a consistent set of elements: a claim, an analysis, a verdict, a list of sources, and so on” (Graves and Anderson Citation2020, 348–349). Fact-checks in this sense are not only provided as reported narrative but also as a set of datapoints such as the verdict or the fact-checked statement. This approach results in the journalistic product also being the database itself (Graves and Anderson Citation2020). Fact-checking organizations provide their fact-checks on the one hand as internal infrastructures in the form of their own databases.
On the other hand, fact-checks can feed into overarching digital infrastructures as they are provided by for instance Poynter or Google where individual fact-checkers choose to feed their content into collective infrastructures (Graves and Anderson Citation2020). Via both paths fact-checking organizations interact with their users such as other journalists, politicians or educators as the main users (Singer Citation2019).
Previous studies have addressed several perspectives to fact-checking – for example how journalists perceive fact-checking (Mena Citation2019), whether fact checks are effectual (Nyhan et al. Citation2020), how fact-checking is perceived by its users (Brandtzaeg and Følstad Citation2017) or a practitioners perspective (Amazeen Citation2019). A few studies also critically assessed fact-checking as infrastructure in the form of databases of single fact-checkers – namely Lim's (Citation2018) comparison of two US-located fact-checking organizations regarding overlapping stories and ratings and Humprecht's (Citation2020) comparison of a selection of fact checkers in Austria, Germany, UK and US with regard to source transparency.
However, to our knowledge our study is the first to systematically compare two overarching digital fact-checking infrastructures. The sum of all fact-checks from all fact-checking organizations should provide an idea of what journalists and other knowledge workers in the field of fact-checking collectively consider to be false (see e.g., Lim Citation2018). Yet, studies on the epistemology of journalism in relation to misinformation show that journalists differ greatly in what they consider to be false, depending on political regime, ownership, personal beliefs and social environment (see e.g., Godler and Reich Citation2013). This is consistent with the strong tradition in the field to focus less on how truthful or objective a claim is (Ekström, Lewis, and Westlund Citation2020; Godler, Reich, and Miller, Citation2020) but more so on the “context of justification” (Ettema and Glasser Citation1985). Also services provided by fact-checking organizations differ, for example, in the way fact-checked stories are presented or whether and how a rating system is used (Graves Citation2018). Therefore, we cannot assume that overarching digital infrastructures such as Poynter and Google contain the same fact-checked COVID-19 stories, or even the same rating of the stories. However, being transparent about such differences and potential biases will only strengthen the infrastructure further. A study that uses Poynter and Google in order to analyze COVID-19 related misinformation was conducted by Brennen et al. (Citation2020), and shows that most disinformation identified by fact-checkers was spread on social media platforms, coming from various sources, and that public figures play an important role in the spreading. However, this and other studies using these infrastructures (Kolluri and Murthy Citation2021; Luengo and García-Marín Citation2020; Patwa et al. Citation2021) would profit from a better understanding of the potential inherent biases.
According to Bowker et al. (Citation2010), knowledge infrastructure in general is often invisible, taken for granted (Star and Ruhleder Citation1994). Yet, Bowker et al. (Citation2010, 133) remind us that information is able to “promote combined (if uneven) development across a very broad range of work practices.” Digital fact-checking infrastructures, are a result of local work practices of journalists and other knowledge workers, but moved towards a global structure. The infrastructures serves various stakeholders in and outside the journalistic field, where “the daily work of one person is the infrastructure of another” (Star and Ruhleder Citation1996). Therefore, it is important to scrutinize systematic biases, competing accounts, overlapping standards or “outright contradictions” (Bowker Citation2000) to develop and sustain a strong infrastructure.
In this article, we build on this framework and thus do not assume a high degree of overlaps and no differences and inherent biases. Instead, we account for these and discuss contradictions and variance, not only in terms of the categorization but also in terms of who, what and where the different databases have chosen to “look for” false claims. We expect the infrastructure studies, with their focus on scalable knowledge structures, to supplement the justification focus in the epistemology of journalism (Ekström, Lewis, and Westlund Citation2020).
Types and Consequences of Potential Differences and Biases
We propose to analyze different dimensions to account for differences and potential inherent biases in the overarching digital infrastructure. One of the dimensions is whether there are differences regarding who fact-checked the claims in the infrastructures (the fact-checking organizations). Due to the local-global character of the infrastructures, they may differ in how and which fact-checks are conducted and therefore directly design our understanding of what is false or misleading in a biased way. Lowrey (Citation2017), for example, observes that, at least in the U.S., fact-checking databases tend to be diversifying, e.g., regarding their link to journalism. Fact-checking organizations also differ in the ratings they use, which could affect how misinformation is perceived (Clayton et al. Citation2020).
We furthermore propose to investigate biases towards certain platforms, i.e., where the misinformation was circulated. This is important because some fact checkers might have a tendency to look for false claims in some places over others depending, e.g., on economic incentives and business models – both for fact-checkers and infrastructure providers. Different platforms also potentially affect how misinformation is perceived or spread (Shin et al. Citation2018). For example, platforms differ regarding users, with social media platforms also attracting people who distrust traditional media (cp. Zhuravskaya, Petrova, and Enikolopov Citation2020). Even though misinformation may occur many places at once, no attempts have been made to develop such an account.
As the spreading of misinformation also depends on who published the misinformation on platforms (cp. Grinberg et al. Citation2019; Walter et al. Citation2020), we look at this dimension as well. Even though public figures might not distribute most of the misinformation, they catch more attention with their misinformation than other distributors (Brennen et al. Citation2020). Platforms and content creators might also affect how much misinformation is trusted or believed, and therefore affect how effective the misinformation is (cp. Pennycook and Rand Citation2019).
Different topics and types of misinformation potentially have different consequences (van der Linden, Roozenbeek, and Compton Citation2020). Belief in false cures and treatments can even have potentially deadly consequences, for example. Furthermore, knowledge about the extent to which misinformation can be assigned to specific topics or types of misinformation probably helps in combatting the spread of misinformation (Charquero-Ballester et al. Citation2021). Even though we do not look at consequences of biases on the spread of misinformation in this article, knowledge about biases in fact-checking infrastructures will contribute to strengthen validity in future research.
Methodology and Methods
We apply a holistic approach for the probing and comparison of infrastructures, looking at two components: the overlap between fact-checking infrastructures and differences in comparison to each other ().
In the first component, we investigate the amount of overlap between infrastructures and focus on further describing it by looking into the overlapping stories and the associated ratings. By stories we mean fact-checkers’ published evaluations of the claims that were fact-checked. In the second component, we investigate the differences of the infrastructures by looking into who was behind the fact-checking, where the fact-checked claim was published, who published it, what topics and what type of misinformation the infrastructures contain. Examining this for each infrastructure allows conclusions about how they reflect the ideal of transparency and to directly compare them.
Data Extraction
When it comes to COVID-19, two main infrastructures exist that collect and give access to the fact-checked stories from several fact-checking organizations. The IFCN, a unit at the Poynter Institute, has managed the #CoronaVirusFacts Alliance since January 2020. It unites around 100 fact-checkers worldwide. Many members of the IFCN are also partners of Facebook with a view to debunking misinformation (Facebook Citation2020). Beside this infrastructure, we probe and analyze the fact-checking tools provided by Google, consisting of two tools launched in 2019: Fact-check Explorer and Fact-check Markup Tool. Both tools “aim to facilitate the work of fact-checkers, journalists and researchers.”Footnote3 The Google Fact-check Explorer does not only include fact-checked stories about COVID-19, but searches can be conducted accordingly. These two infrastructures not only differ regarding funding but also regarding eligibility, with Google authorizing fact-checkers for the Google Fact-check Explorer. These differences might result in variations regarding content and with regard to transparency about content and contributors.
We extracted stories published on the Poynter website using DataMiner (version 3.299.84, Software Innovation Lab LLC, Seattle, USA), and stories on the Google Fact-check Explorer website using their API with the search term “COVID-19” and in English. We analyzed stories published from the beginning of the COVID-19 epidemic in Europe, 1 February to 30 September 2020. We removed duplicates, resulting in two datasets with 8,719 stories (Poynter) and 1,217 (Google). Both datasets contained the title of the stories, ratings, fact-checking organization, date and a URL to the story with fact-checked explanations. The Poynter dataset also included country, publishing platform and an explanation of the rating. The Google dataset additionally included the fact-checker website and content creator.
Parameters and Methods
The extracted data is analyzed according to the two methodological components. For looking at the overlap between the infrastructures, we assess the inter-rater reliability of the two infrastructures inspired by Lim's (Citation2018) manual approach. We suggest a mixed-methods approach for comparison to assess the overlap between the infrastructures in a three-step procedure. First, we calculate and identify the overlap by comparing two computational techniques. Next, we validate the overlap using a manual approach and examine whether stories that overlap are rated similarly. To identify the overlap, we use a simple string-matching method based on Jaccard Similarity and a more advanced machine learning technique using document embedding. Jaccard Similarity (Jaccard Citation1901) is the number of overlapping words divided by the total number of words from both documents. The Jaccard similarity index is normalized between 0 and 1, with a score of one indicating identical documents. The document embedding was performed with BERT, using the sentence-transformers package with the pre-trained model “stsb-roberta-large” (Reimers and Gurevych Citation2019). The textual similarity of the claims is calculated using cosine similarity, with higher scores indicating higher similarity. Both computational methods were carried out in Python (Python Software Foundation, version 3.7.9, https://www.python.org/).
The second component consists of analyses of differences. We use manual coding to compare categories and topic modeling to identify the main topics in each infrastructure. provides an overview of the methods used in each of the components.
Table 1. Methods used for analyses by component and parameter.
Fact-checking organizations: We analyze differences regarding organization, location of the organization and applied ratings. We looked at the actual ratings and subsequently organized these many ratings in six categories: false, partly false, mixed, partly true, true and undefined. Where possible, for each story in each infrastructure we coded for the continent on which the fact-checker is located, based on country information available in the Poynter database (if necessary supplemented by information from the URL to the original story).
Platforms: Based on the extracted information about publishing platform or content creator, we organized the available information into six categories. We distinguish between three of the biggest social media platforms – namely Facebook, Twitter and WhatsApp – and summarize the other social media platforms (e.g., YouTube, Instagram, Line) in one category. The category “several different social media” includes stories with more than one mentioned social media platform. The stories were assigned to a separate category, if a social media platforms and another platform was mentioned (e.g., Website, news channel, media outlet). The stories were assigned to the sixth category (for example, stories published on news channels only), if no social media platform was mentioned.
Content creators: We used the information about origin to analyze differences in who used the different platforms to publish misinformation and distinguished between individuals, public persons (such as politicians and celebrities) and organizations. Public persons were identified when a concrete name was mentioned, assuming that these persons are known to a larger audience. Organizations cover all public authorities and news channels, if recognizable, and persons identifiable as journalist. Otherwise, stories fall into an undefined category.
Types of misinformation: We coded a subset of stories (Google, all stories from March N = 226; Poynter random 10% of the March set N = 252) for the type of misinformation by two raters and assessed the agreement using Krippendorff's Alpha (Citation2018). The score was .74 for both Google and Poynter. In cases of disagreement, raters subsequently agreed on the type. The type of misinformation was determined bottom-up by two researchers from the Google stories and were labeled “cure, prevention & treatment,” “conspiracy,” “political measures,” “vaccine & test kits,” “virus characteristics & numbers” and “other,” and represent different kinds of COVID-19 related misinformation (see also Charquero-Ballester et al. Citation2021). The clustering was inspired by a typology of narratives developed by the EU DisinfoLab (EU DisinfoLab Citation2020).
Topics: To identify topics across stories in the two datasets, we applied topic modeling using BERT (Reimers and Gurevych Citation2019) (see supplementary material A for technical details). The output clusters the stories close to each other when they are textually similar.
We look at topics and types of misinformation separately, as the automated approach for topics probably provides a more detailed picture of fact-checked misinformation content, whereas types of misinformation are better suited to illustrate differences and are less dependent on the chosen parameters.
We performed statistical analysis on all comparisons where possible. As we compared distributions with categorical variables, we tested for significant differences using the chi-square test for independence. In cases where the test was significant (meaning that the two distributions were different from each other), we performed a post-hoc test by calculating the adjusted residuals to test which categories within the distributions differed from each other. We applied a significance threshold of 0.05, but corrected for multiple comparisons across the five chi-square tests (resulting in a threshold of 0.01) as well as within each chi-square test using the Bonferroni correction.
Contextualizing through Documentation
To further contextualize the analyses based on our socio-technical theoretical understanding of the infrastructure (Bowker et al. Citation2010), we use the documentationFootnote4 provided by Poynter and Google as means to transparency. Poynter provides information about all contributors to the infrastructure and the procedures. IFCN members are, according to the code of principles, committed to nonpartisanship and fairness, to transparency of sources, funding, organization and methodology, and to open and honest corrections. Hence, the Poynter database is transparent regarding contributors, eligibility and methods. In comparison, the Google infrastructure provides information that is less transparent regarding membership. The Google Fact-check Explorer allows searches for fact-checkers. However, an exhaustive list needs to be extracted by the user via an API. Consulting Google elicited that around 200 organizations contribute to the infrastructure. Eligibility information is provided on several websites. Contributors need to meet the guidelines of Google that include compliance with standards for accountability, transparency, readability, site misrepresentation and a correction policy. Instead of demanding nonpartisanship, websites for political entities are not eligible. All ClaimReviewed fact-checks of an eligible organization are included in the infrastructure. Publishers are periodically reviewed according to the guidelines, but single entries are not controlled. The Poynter infrastructure also provides additional information and services for users, such as weekly reports or graphs on topic clusters in the infrastructure. The search results provide immediate information about fact-checkers, rating, date, country and story title. Additional information is provided via links to the original fact-check on the member’s website. Even though a lot of information is provided, extraction of it, e.g., via an API, is not provided. The Google Fact-check Explorer provides a search for individual search terms. Search results can be filtered by language and most recent entries and includes, besides the information we extracted, also links to similar topics. Provided information can be extracted via an API. In comparison to the Poynter infrastructure, this offers an advantage for researchers. The Google Markup Tool provides an easy way for fact-checkers to use ClaimReview to signal to Google that their article is a fact-check. IFCN and the fact-checking community had a central role in developing the ClaimReview standards. Google does not provide analyses itself. It is purely a search interface and dependent on the markup of fact-checkers. The Poynter website is not only a search interface but aims at informing as well, e.g., through the provided reports and analyses. In the next section, we will present our study results of overlaps and differences in the two infrastructures and in the final section we will discuss the implications of our findings.
Results
Overlap between Google and Poynter
We did not find a large overlap between the two infrastructures. On the contrary, when using the best performing computational method (BERT), we found that 3.3% of the stories in the Poynter infrastructure were also included in the Google infrastructure, and 23.9% vice versa. However, not necessarily by the same fact-checker or with the same rating.
To allow for manual human control, we chose the first 500 pairs of stories with the highest overlap scores for each of the methods, with a cut-off of 0.71 (cosine similarity for BERT) and 0.19 (Jaccard similarity). For each pair of stories identified by the computational methods, one human rater coded whether there was a true overlap, thereby excluding false positives. The manual rating showed that 267 (53%) were matching stories (true positives) in the Jaccard similarity sample and 291 (58%) in the BERT sample. Aiming for a high percentage of true positives, we chose to use the BERT sample for further analysis (details can be found in supplementary material B in Tables S1–S3). This confirms that the two databases provide different infrastructures of information about false claims. That is, different approaches to the definition of what is false (Godler and Reich Citation2013), are differently reflected in the infrastructures.
Assessment of Ratings for Overlapping Stories
We looked at whether stories that were defined as overlapping in the 500 pairs with the highest scores in cosine similarity (N = 291) were assigned to the same rating categories, which was the case for 261 (89.7%) pairs. For another 21 pairs, the difference can be explained by missing ratings in one of the infrastructures. That is, only for ten pairs, the ratings differed between the infrastructures (3.4%) implicating that fact-checkers agree upon their evaluation of statements.
Differences Regarding Fact-Checking Organizations
We analyzed which fact-checkers contributed most to each infrastructure. shows that three of the five top contributors are the same. However, the other two differ, just as the extent to which each fact-checker contributes differs. Based on all stories in the datasets, the three identical contributors among the top five do not contribute the same number of stories to each infrastructure, but contribute less to the Google infrastructure. Either fact-checkers do not use ClaimReview in English for all the stories they check or the same keyword search does not pick up the same stories. Furthermore, we see that 50 different fact-checkers contribute to Google but almost 100 to Poynter within the topic of COVID-19. That is, only around a fourth of all fact-checkers (50 out of 200) eligible for the Google infrastructure contribute to detecting COVID-19 related misinformation. Those contributing to Poynter also contribute with more stories on average than those contributing to Google. This illustrates that the Poynter infrastructure is designed to collect as much COVID-19 related misinformation as possible, while the Google Fact-check Explorer has a broader coverage of topics and not only focuses on COVID-19 misinformation. This indicates that for COVID-19 misinformation Poynter is the more exhaustive infrastructure.
Table 2. Differences by fact-checkers and infrastructure.
We investigated the ratings used by the fact-checkers in more detail and saw that, in general, there are large differences between the two infrastructures (). First of all, we found 25 different rating categories used in Poynter, but 71 in Google, indicating non-standardized infrastructure with uneven work practices (Bowker et al. Citation2010). A reason for this might be that fact-checkers contributing to Google are more diverse than those contributing to Poynter, which can be partly explained by the described differences in eligibility regulations, with Poynter relying mainly on IFCN members who follow the Code of Principles. Regarding ratings, it seems that IFCN membership leads to more harmonization than eligibility based on Google standards. Furthermore, “false” is the most commonly used category in both infrastructures. However, the percentage of “false” rated stories is much higher in Poynter than in Google. The percentage of “misleading” rated stories is comparable. We found, based on the created rating categories, that stories not rated as false only account for a small percentage in Poynter. In Google, we find more stories that are labeled true and stories for which no rating is provided. These differences can probably also be explained by differences between the infrastructures in documentation and eligibility. Researchers should be aware that the Google infrastructure is more heterogeneous regarding ratings. The findings also indicate that standards lead to a greater harmonization among fact-checkers and confirm the high heterogeneousness regarding rating systems in general. The results indicate a need for improved standardization of rating categories across fact-checkers, potentially pre-defined in the user interface of infrastructures, precisely because they rely on local journalistic work practices (Bowker et al. Citation2010; Godler and Reich Citation2013).
Table 3. Fact-checkers and ratings by infrastructure.
Looking at the location of fact-checking organizations, the two infrastructures had a significantly different distribution across continents (Χ2(6) = 796.64, p < 0.001). shows that many stories in each infrastructure can be assigned to a fact-checker located in Asia (32.8% and 43.1%, respectively). Both infrastructures have comparable number of stories from fact-checkers in Africa and unassigned stories. Otherwise, there are large differences between the infrastructures. North America is much more prominent in Google than Poynter, whereas Europe is more represented in Poynter. Stories from South American fact-checkers could not be found in Google. All in all, these findings confirm the international orientation of Poynter and an orientation toward America and Asia of Google. Even though these distributions do not show how much misinformation is found on each continent related to COVID-19, they illustrate differences in the effort to detect disinformation on a global scale and researchers interested in a specific continent can use this information to select infrastructures accordingly.
Figure 2. The location (continent) of the fact-checkers for both infrastructures in percent. Asterisks indicate a significant difference in a category using post-hoc testing.
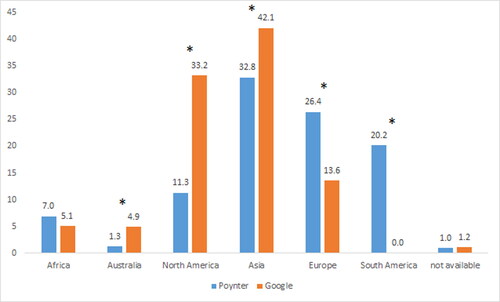
We additionally analyzed the time the fact-checked stories were reviewed, and found significant differences between the infrastructures in that Poynter included more misinformation stories reviewed at the beginning of the pandemic (March and April) (see in the supplementary material C).
Differences in Publishing Platforms
Regarding publishing platforms, we found significant differences between the infrastructures (Χ2(7) = 766.11, p < 0.001). Nearly half of the stories included in Poynter are based on claims published on Facebook (42.6%), followed by claims published on several social media platforms (21%) and claims for which no social media platform was mentioned (17.2%) (see ). Many IFCN fact-checkers carry out paid fact-checks for Facebook, and as our findings indicate, these fact-checkers are the backbone of the Poynter infrastructure. For Google, we saw that publishing platforms outside social media were the dominant source (with 38.1%). Stories included in Google are more often based on claims published on more than one social media platform (19.3%) than only on Facebook (16.1%). However, for a large percentage (21.9%), the information could not be extracted. Furthermore, in both infrastructures the provided information did not allow for insight into non-social media platforms in more detail, for example distinguishing between mainstream or alternative media. More detailed documentation would increase research possibilities and help better understand where misinformation is propagated. Here, both infrastructures and researchers using them could benefit from increasing transparency and usability. The fact that claims published on Facebook are very present in Poynter challenges to some extent independency of fact-checkers from perceived funding as Facebook contributes to funding the IFCN.
Differences in Content Creators
The two infrastructures differed significantly regarding content creators (Χ2(3) = 704.48, p < 0.001). A large percentage of stories in Poynter (89.1%) fall into the undefined category of content creators. In Google, this percentage is smaller (64.2%) albeit still substantial (). Furthermore, most of the detected misinformation is spread by public persons, followed by individuals and organizations. Our analyses clearly showed that even though information can be extracted to some extent, both fact-checking infrastructures – Poynter more so than Google – need to improve documentation of who claimed misinformation. For example, for Poynter only the information “Facebook” could be extracted in many cases, but not the information of who used Facebook to publish misinformation. We manually looked up the undefined cases – based on the information that can be extracted – in a subset of 300 stories in the Poynter dataset using the provided URLs and found that 83.3% of undefined stories in this subset can be assigned to individuals, 5.2% to public persons, 10% to organizations and 1.5% remain undefined. We also looked at the undefined stories in Google, and here we found that 83.2% can be assigned to individuals, 7.3% to public persons and 6.4% to organizations (leaving 3.1% undefined). This indicates that more stories in Google are based on claims from public persons than in Poynter, which probably affects how the detected misinformation spreads (Brennen et al. Citation2020; Pennycook and Rand Citation2019).
Differences in Content
Types of Misinformation
We found significant differences between the types of misinformation in the infrastructures (, Χ2(5) = 16.38, p = 0.006). Whereas Poynter contains more stories related to “political measures” (albeit not significantly, due to the conservative correction for multiple comparisons), Google contains more stories about “virus characteristics & numbers.” Yet, they are surprisingly similar for the other types (examples of entries for each type are provided in the supplementary material D in Table S4). Differences in date of fact-checking or location of fact-checking organizations, as accounted for earlier, might influence the difference in types of misinformation, as attention to different types of misinformation probably changes over time and differs across countries. Even though the categorization was based on the Google infrastructure, the small differences regarding the “other” category justifies its application to Poynter as well.
Topics
We found surprising similarities for the large topics, but differences for the smaller topics (see and , and supplementary material E). Remarkably, Google had two topics related to vaccines, while the Poynter database had none, nor was “vaccine” among the most representative words in the topics. Possibly, claims relating to vaccines were outliers in the topic detection for Poynter as the minimal cluster size was 50 (versus only 10 for Google).
Figure 6. Clustering of the Poynter infrastructure stories after embedding using BERT. The closer the stories are located to each other, the closer their textual similarity. The colors represent the 11 topics. Outliers (grey dots) were not assigned to any topic.
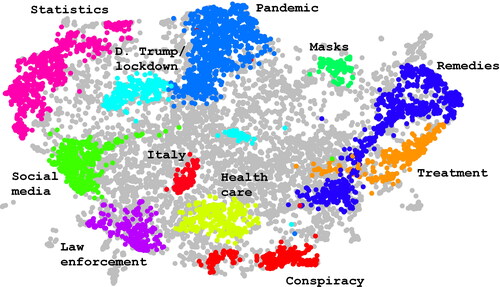
Figure 7. Clustering of the Google infrastructure stories after embedding using BERT. The closer the stories are located to each other, the closer their textual similarity. The colors represent the 13 topics. Outliers (grey dots) were not assigned to any topic.
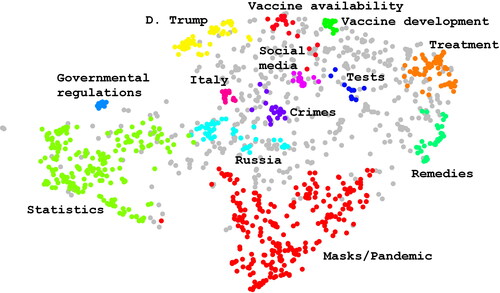
Although the topic analysis covers the period from February to September, the results are comparable to the type analysis covering only March. Both topic and type analysis find similar representations in both infrastructures for many topics/types, even though there are differences between the infrastructures. Regarding content, both infrastructures are comparable with regard to the coverage of topics and types of misinformation. However, researchers should be aware of the smaller differences when interested in a specific type of misinformation or topic.
In general, our findings show that stakeholders relying on the infrastructures should be aware of the differences as the analyzed aspects affect perception and spread of misinformation (e.g., Brennen et al. Citation2020; Grinberg et al. Citation2019; Shin et al. Citation2018; van der Linden, Roozenbeek, and Compton Citation2020).
Discussion and Conclusion
The main contribution of this article has been to suggest a new conceptual and analytical perspective on fact-checking, namely that of overarching digital infrastructures and the identification of overlaps, differences and inherent biases that when accounted for can add to ideals of transparency understood as information on hidden structures (Humprecht Citation2020; Koliska Citation2021; Singer Citation2019). By analyzing the infrastructures with these focal points, the article adds to existing epistemological studies with contexts at scale (see e.g., Ekström, Lewis, and Westlund Citation2020; Godler and Reich Citation2013). The article does so by showing at scale how fact-checking, as digital infrastructures that have downstream effects in society when used by a diverse set of stakeholders, are biased by favoring some content types, platforms, countries and continents etc. over others. These inherent infrastructural biases along with the lack of overlap and the use of a variety of different labels does not contradict but rather supplement existing epistemic accounts on other levels (Graves and Anderson Citation2020; Steensen Citation2019; Uscinski and Butler Citation2013; Waisbord Citation2018). However, including overarching digital fact-checking infrastructures as an integral part of studying epistemology disclose important inherent biases that is otherwise difficult to show at scale as collective results of epistemic work practices (Bowker et al. Citation2010).
By systematically comparing two of the largest infrastructures for COVID-19 misinformation – Poynter and Google – we have analyzed how such infrastructure “color” stakeholders’ and thus society’s beliefs of what is false in different ways because they disclose very different stories as shown in the overlap analysis. Differences between, and biases within, infrastructures can be explained by their characteristics (Bowker et al. Citation2010), such as differences in organizational structure (international network vs. no membership), different eligibility rules (common Code of Principles vs. Google guidelines) and different funding (NGO dependent on expenditure vs. private company). This indicates that our initial assumption that the ownership structure does play a role for the content of the infrastructure and that there is no real unifying global infrastructure of fact-checked stories, but rather supplementary ones (Lim Citation2018). We argue that biases and differences probably affect the findings of studies examining the spread or perception of misinformation dependent on the infrastructures. Future studies should address this issue by examining the effect of the identified differences on studies of misinformation, and similar audits are needed in more infrastructures. Not only will fact-checking as a genre in journalism profit from more transparency in fact-checking infrastructures, but also computational studies within and outside the field of digital journalism (e.g., Kolluri and Murthy Citation2021; Luengo and García-Marín Citation2020; Patwa et al. Citation2021) that would rely to some extent on fact-checking.
Our study is limited in investigating publishing platforms and content creators by the limited information in the infrastructures and the study has especially shown the lack of information and consistent labels and entries. Future research should aim to extend analyses of these aspects by basing the classification on more raters, especially when more detailed information becomes available, thereby making the classification more complex.
The finding that overarching digital fact-checking infrastructures are only to some extent transparent with regard to contributors and information about the fact-checked claims, poses the question how transparency should be addressed by those infrastructures. Transparency in journalism is a rather contested ideal (Ananny and Crawford Citation2018) and other concepts such as source criticism (Steensen Citation2019) or accuracy are used instead or in addition to it (i.e., as also highlighted in this study by including accounts of who has fact-checked). Full transparency contradicts for example copyright issues, the protection of personal information, addressing users in a comprehensive way or the fact that digital sources are often not archived or accessible for a longer period due to the dynamic nature of the digital landscape (Zelizer Citation2019).
However, fact-checkers continue to live up to this ideal and we do not argue for digital fact-checking infrastructures to be fully transparent. Instead, we argue that transparency with regard to some aspects need to be increased in order to use these infrastructures in an accountable way. As Graves and Anderson (Citation2020) argue, fact-checking is journalism that can be used as a database. We argue that also fact-checking infrastructures are combined databases, however they do not follow the same logic as Graves and Anderson (Citation2020) describe for single fact-checking databases and fact-checked stories. Our study contributes to a better understanding of their logic and characteristics by critically assessing them as sources themselves (cp. Steensen Citation2019). However, future studies need to address them in a similar way as single fact-checking databases have been addressed in research, e.g., with regard to how users perceive them, how journalists perceive them or how effective they are (cp. Brandtzaeg and Følstad Citation2017; Mena Citation2019; Nyhan et al. Citation2020). Especially, as they – as results of journalistic practices and as response to misinformation – are challenged by big data and the digital landscape for example in terms of epistemology (e.g., Ekström, Lewis, and Westlund Citation2020; Lewis and Westlund Citation2015). The conceptualization, analytical framework and empirical case study of this article are first stepping stones to that aim but additional research is necessary to further critically assess them as infrastructures for information about what is false.
Supplementary_Material_v5.docx
Download MS Word (195.3 KB)Acknowledgements
The authors would like to thank developer Peter B. Vahlstrup for retrieving data through the Google fact-checker API, student assistants Marie Damsgaard Mortensen and Petra de Place Bak for helping out with the manual coding. Furthermore, we thank special issue editors, and anonymous reviewers for their insightful comments and suggestions.
Disclosure Statement
No potential conflict of interest was reported by the author(s).
Additional information
Funding
Notes
1 https://www.disinfo.eu/coronavirus, last access 31 August 2020.
2 A list of funders can be found here: https://www.poynter.org/major-funders/, accessed 2021/08/06.
3 https://toolbox.google.com/factcheck/about, last access 7 October 2020.
4 We additionally asked Alexios Mantzarlis, News and Information Credibility Lead at Google and former director of the IFCN, for missing information.
References
- Allcott, H., and M. Gentzkow. 2017. “Social Media and Fake News in the 2016 Election.” Journal of Economic Perspectives 31 (2): 211–236.
- Amazeen, M. A. 2019. “Practitioner Perceptions: Critical Junctures and the Global Emergence and Challenges of Fact-Checking.” International Communication Gazette 81 (6-8): 541–561.
- Amazeen, M. A. 2020. “Journalistic Interventions: The Structural Factors Affecting the Global Emergence of Fact-Checking.” Journalism 21 (1): 95–111.
- Ananny, M., and K. Crawford. 2018. “Seeing without Knowing: Limitations of the Transparency Ideal and Its Application to Algorithmic Accountability.” New Media & Society 20 (3): 973–989.
- Ball, P., and A. Maxmen. 2020. “The Epic Battle against Coronavirus Misinformation and Conspiracy Theories.” Nature 581 (7809): 371–374.
- Bechmann, A., and B. O’Loughlin. 2020. Democracy & Disinformation: A Turn in the Debate. KVAB Thinkers’ Report, 37. Koninklijke Vlaamse Academie van Belgie voor Wetenschappen en Kunsten. https://www.kvab.be/sites/default/rest/blobs/2557/Final%20Report%20Dem%20&%20Desinfo.pdf.
- Bowker, G., K. Baker, F. Millerand, and D. Ribes. 2010. “Toward Information Infrastructure Studies: Ways of Knowing in a Networked Environment.” In International Handbook of Internet Research, edited by J. Hunsinger, L. Klastrup, and M. Allen, 97–117. Dordrecht, the Netherlands: Springer.
- Bowker, G. C. 2000. “Biodiversity Datadiversity.” Social Studies of Science 30 (5): 643–683.
- Brandtzaeg, P. B., and A. Følstad. 2017. “Trust and Distrust in Online Fact-Checking Services.” Communications of the ACM 60 (9): 65–71.
- Brennen, J. S., F. M. Simon, P. N. Howard, and R. K. Nielsen. 2020. Types, Sources, and Claims of COVID-19 Misinformation, 13. Oxford, United Kingdom: Reuters Institute for the Study of Journalism. https://reutersinstitute.politics.ox.ac.uk/types-sources-and-claims-covid-19-misinformation.
- Buning, M, et al. 2018. A Multi-Dimensional Approach to Disinformation: Report of the Independent High Level Group on Fake News and Online Disinformation. Luxembourg: Publications Office of the European Union.
- Carmi, E., S. J. Yates, E. Lockley, and A. Pawluczuk. 2020. “Data Citizenship: Rethinking Data Literacy in the Age of Disinformation, Misinformation, and Malinformation.” Internet Policy Review 9 (2): 1–22.
- Charquero-Ballester, M., J. G. Walter, I. A. Nissen, and A. Bechmann. 2021. “Different Types of COVID-19 Misinformation Have Different Emotional Valence on Twitter.” Big Data & Society 8 (2): 205395172110412.
- Clayton, K., S. Blair, J. A. Busam, S. Forstner, J. Glance, G. Green, A. Kawata, et al. 2020. “Real Solutions for Fake News? Measuring the Effectiveness of General Warnings and Fact-Check Tags in Reducing Belief in False Stories on Social Media.” Political Behavior 42 (4): 1073–1095.
- Dias, N., and A. Sippitt. 2020. “Researching Fact Checking: Present Limitations and Future Opportunities.” The Political Quarterly 91 (3): 605–613.
- Ekström, M., S. C. Lewis, and O. Westlund. 2020. “Epistemologies of Digital Journalism and the Study of Misinformation.” New Media & Society 22 (2): 205–212.
- Ettema, J., and T. Glasser. 1985. “On the Epistemology of Investigative Journalism.” Communication 8: 183–206.
- EU DisinfoLab. 2020. The Few Faces of Disinformation, 7. https://www.disinfo.eu/wp-content/uploads/2020/05/20200512_The-Few-Faces-of-Disinformation.pdf
- Facebook 2020. Facebook’s Third-Party Fact-Checking Program. https://www.facebook.com/journalismproject/programs/third-party-fact-checking
- Gelfert, A. 2018. “Fake News: A Definition.” Informal Logic 38 (1): 84–117.
- Godler, Y., and Z. Reich. 2013. “How Journalists Think about Facts.” Journalism Studies 14 (1): 94–112.
- Godler, Y., Z. Reich, and B. Miller. 2020. “Social Epistemology as a New Paradigm for Journalism and Media Studies.” New Media & Society 22 (2): 213–229.
- Graves, L. 2018. “Boundaries Not Drawn: Mapping the Institutional Roots of the Global Fact-Checking Movement.” Journalism Studies 19 (5): 613–631.
- Graves, L., and C. Anderson. 2020. “Discipline and Promote: Building Infrastructure and Managing Algorithms in a “Structured Journalism” Project by Professional Fact-Checking Groups.” New Media & Society 22 (2): 342–360.
- Graves, L., B. Nyhan, and J. Reifler. 2016. “Understanding Innovations in Journalistic Practice: A Field Experiment Examining Motivations for Fact-Checking: Understanding Innovations in Journalistic Practice.” Journal of Communication 66 (1): 102–138.
- Grinberg, N., K. Joseph, L. Friedland, B. Swire-Thompson, and D. Lazer. 2019. “Fake News on Twitter during the 2016 U.S. presidential Election.” Science (New York, N.Y.) 363 (6425): 374–378.
- Humprecht, E. 2020. “How Do They Debunk “Fake News”? A Cross-National Comparison of Transparency in Fact Checks.” Digital Journalism 8 (3): 310–327.
- Jaccard, P. 1901. Étude comparative de la distribution florale dans une portion des Alpes et du Jura [Text/html,application/pdf].
- Kalsnes, B. 2018. “Deciding What’s True: The Rise of Political Fact-Checking in American Journalism.” Digital Journalism 6 (5): 670–672.
- Klemm, C., E. Das, and T. Hartmann. 2019. “Changed Priorities Ahead: Journalists' Shifting Role Perceptions When Covering Public Health Crises.” Journalism (London, England) 20 (9): 1223–1241.
- Koliska, M. 2021. “Transparency in Journalism.” In Oxford Research Encyclopedia of Communication, edited by M. Koliska, 1–23. Oxford, England: Oxford University Press. https://doi.org/10.1093/acrefore/9780190228613.013.883
- Kolluri, N. L., and D. Murthy. 2021. “CoVerifi: A COVID-19 News Verification System.” Online Social Networks and Media 22: 100123.
- Krippendorff, K. 2018. Content Analysis: An Introduction to Its Methodology. Sage Publications. https://uk.sagepub.com/en-gb/eur/content-analysis/book258450.
- Lewis, S. C., and O. Westlund. 2015. “Big Data and Journalism: Epistemology, Expertise, Economics, and Ethics.” Digital Journalism 3 (3): 447–466.
- Lim, C. 2018. “Checking How Fact-Checkers Check.” Research & Politics 5 (3): 205316801878684.
- Lowrey, W. 2017. “The Emergence and Development of News Fact-Checking Sites: Institutional Logics and Population Ecology.” Journalism Studies 18 (3): 376–394.
- Luengo, M., and D. García-Marín. 2020. “The Performance of Truth: Politicians, Fact-Checking Journalism, and the Struggle to Tackle COVID-19 Misinformation.” American Journal of Cultural Sociology 8 (3): 405–427.
- Mena, P. 2019. “Principles and Boundaries of Fact-Checking: Journalists’ Perceptions.” Journalism Practice 13 (6): 657–672.
- Nyhan, B., E. Porter, J. Reifler, and T. J. Wood. 2020. “Taking Fact-Checks Literally but Not Seriously? The Effects of Journalistic Fact-Checking on Factual Beliefs and Candidate Favorability.” Political Behavior 42 (3): 939–960.
- Pasquale, F. 2015. The Black Box Society: The Secret Algorithms That Control Money and Information. Cambridge, MA: Harvard University Press.
- Patwa, P., S. Sharma, S. Pykl, V. Guptha, G. Kumari, M. S. Akhtar, A. Ekbal, A. Das, and T. Chakraborty. 2021. “Fighting an Infodemic: COVID-19 Fake News Dataset.” In Combating Online Hostile Posts in Regional Languages during Emergency Situation, edited by T. Chakraborty, K. Shu, H. R. Bernard, H. Liu, and M. S. Akhtar, Vol. 1402, 21–29. Cham, Switzerland: Springer International Publishing. https://doi.org/10.1007/978-3-030-73696-5_3
- Pennycook, G., and D. G. Rand. 2019. “Fighting Misinformation on Social Media Using Crowdsourced Judgments of News Source quality.” Proceedings of the National Academy of Sciences of the United States of America 116 (7): 2521–2526.
- Pierri, F., A. Artoni, and S. Ceri. 2020. “Investigating Italian Disinformation Spreading on Twitter in the Context of 2019 European Elections.” PLoS One 15 (1): e0227821.
- Reimers, N., and I. Gurevych. 2019. “Sentence-BERT: Sentence Embeddings Using Siamese BERT-Networks.” arXiv preprint arXiv:1908.10084. http://arxiv.org/abs/1908.10084.
- Shawcross, A. 2016. Facts We Can Believe in: How to Make Fact-Checking Better. Beyond Propaganda Series, 44. London, United Kingdom: Legatum Institute; Transitons Forum. https://www.lse.ac.uk/iga/assets/documents/arena/archives/facts-we-can-believe-in-how-to-make-fact-checking-better-web-pdf.pdf.
- Shin, J., L. Jian, K. Driscoll, and F. Bar. 2018. “The Diffusion of Misinformation on Social Media: Temporal Pattern, Message, and Source.” Computers in Human Behavior 83: 278–287.
- Singer, J. B. 2019. “Fact-Checkers as Entrepreneurs.” Journalism Practice 13 (8): 976–981.
- Star, S. L., and K. Ruhleder. 1994. “Steps towards an Ecology of Infrastructure: Complex Problems in Design and Access for Large-Scale Collaborative Systems.” Proceedings of the Conference on Computer Supported Cooperative Work, 253–264.
- Star, S. L., and K. Ruhleder. 1996. “Steps toward an Ecology of Infrastructure: Design and Access for Large Information Spaces.” Information Systems Research 7 (1): 111–134.
- Steensen, S. 2019. “Journalism’s Epistemic Crisis and Its Solution: Disinformation, Datafication and Source Criticism.” Journalism 20 (1): 185–189.
- Tandoc, E. C., Z. W. Lim, and R. Ling. 2018. “Defining “Fake News”: A Typology of Scholarly Definitions.” Digital Journalism 6 (2): 137–153.
- Thomas, K., and A. D. Senkpeni. 2020. “What Should Health Science Journalists Do in Epidemic Responses?” AMA Journal of Ethics 22 (1): E55–60.
- Uscinski, J. E., and R. W. Butler. 2013. “The Epistemology of Fact Checking.” Critical Review 25 (2): 162–180.
- van der Linden, S., J. Roozenbeek, and J. Compton. 2020. “Inoculating against Fake News about COVID-19.” Frontiers in Psychology 11: 566790.
- Vargo, C. J., L. Guo, and M. A. Amazeen. 2018. “The Agenda-Setting Power of Fake News: A Big Data Analysis of the Online Media Landscape from 2014 to 2016.” New Media & Society 20 (5): 2028–2049.
- Vosoughi, S., D. Roy, and S. Aral. 2018. “The Spread of True and False News online.” Science (New York, N.Y.) 359 (6380): 1146–1151.
- Waisbord, S. 2018. “Truth is What Happens to News: On Journalism, Fake News, and Post-Truth.” Journalism Studies 19 (13): 1866–1878.
- Walter, N., J. Cohen, R. L. Holbert, and Y. Morag. 2020. “Fact-Checking: A Meta-Analysis of What Works and for Whom.” Political Communication 37 (3): 350–375.
- Wardle, C., and H. Derakhshan. 2017. INFORMATION DISORDER: Toward an Interdisciplinary Framework for Research and Policy Making. Council of Europe Report DGI, 109. Council of Europe. https://rm.coe.int/information-disordertoward-an-interdisciplinary-framework-for-researc/168076277c.
- Zelizer, B. 2019. “Why Journalism Is about More than Digital Technology.” Digital Journalism 7 (3): 343–350.
- Zhuravskaya, E., M. Petrova, and R. Enikolopov. 2020. “Political Effects of the Internet and Social Media.” Annual Review of Economics 12 (1): 415–438.