Abstract
Advances in analytical methodologies and an avalanche of digitized data have opened new avenues for (digital) journalism research—and with it, new challenges. One of these challenges concerns the sampling and evaluation of data using (non-validated) search terms in combination with automated content analyses. This challenge has largely been neglected by research, which is surprising, considering that noise slipping in during the process of data collection can generate great methodological concerns. To address this gap, we first offer a systematic interdisciplinary literature review, revealing that the validation of search terms is far from acknowledged as a required standard procedure, both in and beyond journalism research. Second, we assess the consequences of validating search terms, using a multi-step approach and investigating common research topics from the field of (digital) journalism research. Our findings show that careless application of non-validated search terms has its pitfalls: while scattershot search terms can make sense in initial data exploration, final inferences based on insufficiently validated search terms are at higher risk of being obscured by noise. Consequently, we provide a step-by-step recommendation for developing and validating search terms.
Introduction
Together with an inundation of digitized data available through journalistic websites, news aggregators, online archives, and social media platforms, high-performance computation power and advanced analytical methods have opened new avenues for communication science and (digital) journalism research (Boumans and Trilling Citation2016; Baden et al. Citation2022). Consequently, scholarship utilizing this rich inventory with automated content analysis approachesFootnote1 to investigate journalistic communication has lately seen a detrimental increase (for overviews, see Hase, Mahl, and Schäfer Citation2022; Digital Journalism special issue edited by Karlsson and Sjøvaag Citation2016). Moreover, text-as-data procedures such as topic modeling or word embeddings are gaining momentum in practical and application-oriented areas, such as computational journalism (Stray Citation2019).
At the same time, these increasingly popular computational approaches have ignited debates about principles for their valid and robust application. Accordingly, researchers have started to discuss the sensitivity of results to often arbitrary choices around preprocessing (Denny and Spirling Citation2018; Maier et al. Citation2020) and modeling (Roberts, Stewart, and Tingley Citation2016; Wilkerson and Casas Citation2017). In addition, multiverse approaches have recently been used to assess how possible combinations of analytic decisions affect study outcomes (Pipal, Song, and Boomgaarden Citation2022). However, one step that has rarely been critically examined concerns the practice of automated data collection; hence, we know little about the sensitivity of automated content analyses, their results, and the conclusions drawn from them to sampling errors and noise potentially introduced by the use of non-validated search terms.
This lack of knowledge is surprising, given that although researchers seem to do a poor job of choosing adequate search terms to identify relevant documents (King, Lam, and Roberts Citation2017), the strategy is frequently applied to collect large amounts of data (Barberá et al. Citation2021). Moreover, well-established performance metrics to validate the quality of search terms are rarely used (Stryker et al. Citation2006)—probably due to (a naïve) trust in haphazardly selected search terms. Computational techniques and ready-made tools offer a wealth of opportunities to scholarship; at the same time, they bear the risk of passing “large amounts of (relevant and irrelevant) data to the machine, hoping that the algorithm will identify and rely on the ‘right’, valid patterns” (Baden et al. Citation2022, 4). This can ultimately lead to a phenomenon called ‘apophenia’, that is, “seeing patterns where none actually exist” (Boyd and Crawford Citation2012, 668). This risk, in combination with the use of non-validated search terms and the practice of distant reading, makes it more likely that noise will slip in unnoticed during the process of data collection, which can arguably elicit methodological concerns regarding the representativeness of the data and the credibility and validity of the results.
By its nature, this problem is neither discipline- nor subject-specific. However, in order to contextualize the consequences of using non-validated search terms in combination with automated procedures, this study focuses on (digital) journalism research, a research field for which the outlined problem is particularly relevant. To start, online archives providing collections of digitized news content (e.g. MediaCloud), social media data (e.g. CrowdTangle), or topic-specific information (e.g. Global Terrorism Database (GTD)) provide access to and facilitate retrieval of journalistic communication. Consequently, such archives have become some of the most essential resources for journalism researchers and data journalists in recent decades (Blatchford Citation2020). Operating through search term queries, these archives enable scholars and practitioners not only to compile large datasets in a resource-efficient manner but also to conduct basic analytical tasks, such as assessing the prevalence of certain terms over time or across news outlets, or their co-occurrence in media coverage. In addition, a look at research practice within the field points to a growing number of studies that collect data using search term sampling in online archives and that employ automated text methods to investigate key research areas in (digital) journalism research, such as news quality (e.g. Jacobi, Kleinen-von Königslöw, and Ruigrok Citation2016), actors (e.g. Walter Citation2019), or topics in news reporting (e.g. Hase et al. Citation2021). Both the increasing diversity of digital databases and their functional logic, as well as the widespread use of search term sampling together with automated methods to study journalistic communication, underscore the significance of systematically addressing the risks posed by non-validated search terms in the field of (digital) journalism research.
In taking on this challenge, this article provides a threefold contribution, offering: (1) an interdisciplinary systematic literature review of research in (digital) journalism research and other fields to evaluate the scope of the problem outlined above; (2) an evaluation of the consequences of using non-validated search terms for the results of automated content analyses by applying a multi-step approach, using climate change communication in digital news media as a test case; and (3) a step-by-step recommendation for developing and validating search terms, which is not only relevant for data sampling but also informs studies applying dictionary analyses. More broadly, we aim to encourage researchers to engage in a controlled and transparent practice of automated sampling by systematically validating their data, as this ultimately engenders confidence in the representativeness of their data and the validity of their findings.
The Inconsistency of Search Term Sampling
Data sampling marks the beginning of most empirical analyses; as such, critical reflection is merited as to whether the data is truly relevant and representative, since this not only determines the results and conclusions drawn but also ultimately impacts their generalizability (Stryker et al. Citation2006). Thus, the first decision that content analytical researchers are faced with is defining the relevant universe of documents and how to sample them. In this article, we focus on search term sampling due to three guiding observations (cf. Barberá et al. Citation2021; King, Lam, and Roberts Citation2017; Lacy et al. Citation2015; Stryker et al. Citation2006): first, previous research suggests that search terms are widely used to perform automated data sampling; second, the selection and application of search terms is considered a low-threshold approach for resourcefully retrieving data of interest; and third, search terms can be applied to numerous activities, including matching, counting, and dictionary methods. Therefore, we argue that our approach can be extended to applications far beyond data gathering.
Quality Criteria and Performance Metrics
Both manual and automated content analyses are subject to (somewhat) established quality criteria for evaluating the collection and analysis of the data and its reporting. However, while manual content analysis is considered a genuine method of communication science and, thus, the field has mostly agreed on relevant quality criteria, automated content analysis techniques originate from computational linguistics. As a result, subtle but important differences exist regarding the premises of quality criteria for automated approaches compared to the established standards in communication science (cf. DiMaggio 2015; Laugwitz Citation2021; Scharkow Citation2012). Specifically, while the most important quality criterion for manual content analyses is intercoder reliability, which determines whether different coders agree on how the same data should be coded, automated approaches are more concerned with the validity of the measurements and analyses. In the broadest sense, validity measures whether the operationalization actually captures the theoretical or conceptual construct and thus assesses the quality of a study’s inferences (Findley, Kikuta, and Denly Citation2021).
In line with our research focus, we concentrate on determining the validity of search terms, defined as their ability to accurately retrieve relevant documents of interest and to eliminate irrelevant ones (Stryker et al. Citation2006). While manual content analyses typically rely on trained coders who follow detailed operational rules and manually decide whether each individual case is of interest to the object of study, automated techniques and large-scale data work with other quality efforts. For this purpose, computational linguistics offers three performance metrics that measure the validity of search terms by comparing computational classifications with human-coded benchmarksFootnote2: precision, recall, and F1-score (cf. Manning and Schütze Citation2002, 267–71; Scharkow Citation2012, 133–36). Given that the dataset should resemble a defined population, researchers face the challenge of choosing either a single search term or a list of search terms that is capable of extracting relevant text documents and eliminating irrelevant ones. In the application of these performance metrics to data retrieval, precision can be defined as a metric that indicates whether a document retrieved by the search term is truly relevant: high precision means that many documents captured by the search term are truly relevant. In contrast, recall is a metric that denotes the proportion of relevant articles that are actually identified by the search term: a high recall indicates that the search term yields a large number of documents from among all those that are potentially relevant. In practice, precision and recall are often in conflict with each other—that is, high precision often comes at the expense of recall. Depending on their research interest, scholars have to decide whether precision or recall is more critical to their intended analysis. A combination of precision and recall is called the F1-score, or the harmonic mean of both measures: this value indicates an overall estimate of search term performance.
A (Naïve) Reliance on Haphazardly Chosen Search Terms
Given that automated content analyses (and the accompanying practice of distant reading) have recently become an inherent part of studies in (digital) journalism and other fields, it seems all the more necessary to validate the search terms used to sample large amounts of data in order to ensure that the retrieved content truly represents the targeted concept or discourse. However, a closer look reveals a remarkable contradiction that manifests itself in three ways.
Widespread Use of Search Term Sampling
Drawing on a review of content analyses published in communication science journals between 2000 and 2005, Stryker et al. (Citation2006) showed that 42% of all studies used search terms to compile data corpora. One decade later, King, Lam, and Roberts (Citation2017) confirmed that search term sampling remains one of the most common strategies for collecting large-scale datasets. Moreover, in addition to digital news databases such as ProQuest or MediaCloud, social media discourses are often tracked via search term- and hashtag-based approaches (e.g. Kirilenko and Stepchenkova Citation2014).
Choice of Inadequate Search Terms
However, some scholars have warned that search terms chosen by researchers, typically in a scattershot manner, may be far from optimal or even biased. For instance, King, Lam, and Roberts (2017, 974) asked people to pick search terms that they deemed suitable to selecting text documents, demonstrating that “even expert human users perform extremely poorly and are highly unreliable.” In a similar vein, Sobel and Riffe (Citation2015) showed that searching for country names to identify news coverage of those nations is highly ineffective, as it resulted in 91% false positives.
Neglect of Best Practices to Validate Search Terms
This contradiction is even more pronounced given that researchers are equipped with best practice guidelines to validate the quality of search terms and to report methodological details (Lacy et al. Citation2015; Stryker et al. Citation2006; Vogelgesang and Scharkow Citation2012). In line with these guidelines, researchers need to define the relevance criteria, create a manual benchmark, calculate performance metrics to measure the quality of the search terms, and transparently report all methodological details, including number of coders, amount of data, intercoder reliability, and sample size. However, studies indicate that scholars rarely make use of these guidelines: for instance, Stryker et al. (Citation2006) found that out of 83 content analyses, only 39% stated the search terms they used, and only 6% discussed their validity. In a similar vein, Lovejoy et al. (Citation2014) showed that a majority of studies were not transparent about their sample procedure.
Overall, the use of search term sampling suffers from a fundamental contradiction: even though researchers seem to do a poor job in choosing adequate search terms to identify relevant documents, they frequently use this strategy to collect large amounts of data. Additionally, well-established performance metrics to validate the quality of search terms are rarely stated, probably due to (a naïve) trust in haphazardly chosen search terms and an underestimation of the consequences. What remains unclear is, first, whether the lack of validation also applies to automated content analysis studies, and second, the extent to which this affects the results of such analyses. To investigate both gaps empirically, we ask:
RQ1. How widespread is the use of non-validated search terms in combination with automated content analyses in research in (digital) journalism and other fields?
RQ2. To what extent does the use of non-validated search terms affect the results of automated content analyses?
The Failure to Validate Search Terms (RQ1)
To address the issue outlined above, we provide a systematic literature review of studies that apply automated content analysis approaches in analyzing (third-party) data collected through search terms. This allows us to understand how widespread the lack of validation is and what future recommendations need to consider.
After several selection steps, 105 empirical studies from three core social science disciplines (communication science, political science, and sociology) published in English-language journals from 1990 to 2020 covered by Scopus were selected for the literature review (see Supplementary MaterialFootnote3 Section A for detailed information on sample selection and the coding procedure).
In line with guidelines on search term validation and on reporting the methodological details of validation procedures (as outlined above), we coded all studies according to whether they state their search terms, validation metrics, and relevance criteria in the retrieved articles as well as their intercoder reliability, number of coders, and amount of data used for human coding. To assess whether the use of non-validated search terms applies to journalism research, we coded whether the study investigated journalistic communication.
In a summary of answers to RQ1, 73.3% (n = 77) of all studies across disciplines stated the search terms they used to gather data (see ). Studies that did not provide search terms merely indicated, for instance, that they analyzed political coverage and included news stories that mention politicians or political parties, without specifying them. In total, only 12.4% of all studies referred to some sort of validation metric, and an equal number of studies outlined content-related criteria that a text had to meet in order to be considered relevant to sampling. In terms of human-based validation, only 7.6% of all studies made any reference to intercoder reliability; of those studies, only 11.4% and 15.2% reported the number of coders and the amount of data used for human-annotated validation, respectively.
Table 1. Results of the systematic literature review.
Examining studies dealing with journalistic communication highlights that the use of non-validated search terms is a problem that needs to be systematically addressed in the field of (digital) journalism research. In a comparison of disciplines, there seem to be differences in validation practices; however, due to small samples, these results should be considered with caution. Overall, the literature review illustrates that adequately reported measures of search term validation are more common in political science articles. In addition, of all the 105 studies we analyzed, only four studies described both validation metrics and intercoder reliability, and only two studies in our sample properly included all methodological details of the validation process, as stipulated by best practice recommendations.
Ideally, researchers who apply computational approaches to investigate large-scale data sampled by search terms will account for the quality of these terms—that is, the terms’ ability to retrieve relevant documents of interest and to eliminate irrelevant ones, which are likely to introduce noise. Unfortunately, however, the results of our literature review illustrate an underwhelming use of validation practices. This not only underscores the contradiction outlined above, it also points to—and here we echo Jünger, Geise, and Hänelt (Citation2022, 1483)—a “black box” of automated data collection, “whose inner structure, functions, and operations are potentially unclear in the research process.” This ultimately reinforces the need to evaluate the consequences of non-validated search terms for the results of automated content analyses, in and beyond (digital) journalism research.
Consequences of (Not) Validating Search Terms (RQ2)
Research Design
As discussed above, the use of non-validated search terms in combination with automated approaches and the practice of distant reading makes it more likely that noise will slip in during data collection. We define noise as “corruptions […] that may impact interpretations of the data, models created from the data and decisions made based on the data” (Zhu and Wu Citation2004, 177). Noise arises during the collection or processing of real-world datasets and can be caused by either “irrelevant” or “meaningless” data (Gupta and Gupta Citation2019, 466), by which we mean both random, unsystematic data and data that do not accurately represent the targeted latent construct or discourse and therefore may lead to erroneous conclusions.
To comprehensively evaluate the consequences of search term validation, we build on a multi-step approach (see ; following de Vries, Schoonvelde, and Schumacher Citation2018) by examining three different stages within a typical text-as-data project. Each stage relates to common research questions and frequently applied analyses within the field of (digital) journalism research and climate change communication—an issue that has recently received much scholarly attention (e.g. Bohr Citation2020; Hase et al. Citation2021). The outcomes of each of these stages can in turn serve as starting points for further analyses.
Figure 1. Research design.
Note. The terms “validated” and “non-validated” text corpus, DFM, and topic model reflect that they were constructed using the validated or non-validated search term. They do not refer to post-hoc validation procedures, like the validation of results from unsupervised procedures such as topic modeling approaches.
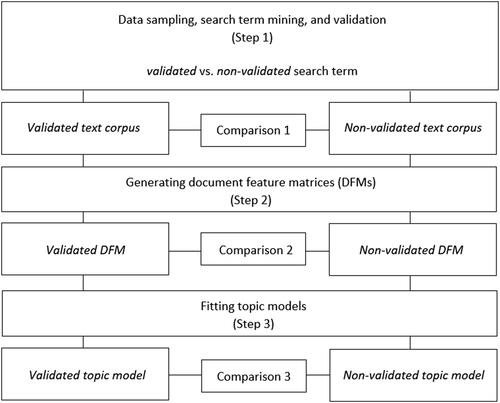
Accordingly, our research design is divided into three comparisons. At the first stage, we compare two text corpora: one operating with a validated search term (which has been carefully validated to ensure that the majority of articles truly represent the targeted discourse), and one featuring a non-validated search term (which has not been validated and thus may retrieve many irrelevant text documents, i.e. noise). Comparisons are drawn in relation to the corpus characteristics and the level of issue attention toward climate change. This comparison relates to common research questions in the field of (digital) journalism research, such as the identification of events associated with peaks in news media attention to climate change (e.g. Hase et al. Citation2021).
Thereafter, in the second stage, documents are preprocessed and turned into document feature matrices (hereafter referred to as DFM). Here, we compare the validated and non-validated DFMs by measuring the similarity of vocabulary. In research practice, DFMs often constitute the starting point for sentiment or dictionary analyses of news media coverage. This comparison relates to common research questions in the field of (digital) journalism research, such as the linguistic analysis of lexical and discursive formations around compounds, such as carbon footprint or carbon sinner, in news coverage (Nerlich and Koteyko Citation2009), or the identification of climate change frames based on search terms (Jang and Hart Citation2015).
We then proceed to the third stage by narrowing the focus to a standard automated content analysis methodology in communication and (digital) journalism research: topic modeling (for an overview, see Maier et al. Citation2018). Topic modeling is a popular bag-of-words approach that identifies underlying thematic structures (i.e. topics) in large text corpora. To draw comparisons, we estimate LDA topic models in both DFMs separately and assess the similarity between the validated model and the non-validated model in terms of topical content and prevalence. This approach allows us to consider the specific characteristics of both text corpora (e.g. size and vocabulary) and to show how two methodologically comparable but independent analyses (validated vs. non-validated search term) differ in their possible inferences. For this stage of comparison, we again drew inspiration from common research questions in (digital) journalism, such as the identification and analysis of topics and their prevalence in climate change reporting within a given national context or time period (Bohr Citation2020; Hase et al. Citation2021).
To address RQ2, we translate our research design into the following validation questions (VQ):
VQ1. To what extent do the validated and non-validated search terms affect the characteristics of the text corpora?
VQ2. To what extent do the validated and non-validated search terms affect the content of the document feature matrices?
VQ3. To what extent do the validated and non-validated search terms affect topical content and prevalence?
Data and Methods
The following section outlines the methodological steps required for each of our comparisons.
Step 1. Data sampling, search term mining, and validation
To perform analyses on digital news coverage of climate change, we used data from the Online Media Monitor (OMM; Brüggemann and Sadikni Citation2020), which provides daily updated worldwide monitoring of climate change discourses in online media. We sampled English-language news articles from 19 countries and 56 online news outlets spanning the period 2016–2020 (see Supplementary Material B1 for a detailed description of the dataset).
In line with our research design, we compared two different types of search terms—that is, a validated search term and a non-validated search term. The OMM operates with the terms climat* chang* OR global warm*, which have not been validated as to whether texts extracted by these terms actually represent climate change discourses; therefore, we defined these terms as the non-validated search term. Developing a valid search term—a procedure which we call search term miningFootnote4—can be accomplished in a variety of ways, from advanced techniques such as machine learning (e.g. King, Lam, and Roberts Citation2017) to expert-informed approaches (e.g. Rinke et al. Citation2022) and more low-threshold strategies (e.g. Stryker et al. Citation2006). We deliberately chose to adopt a low-threshold approach to enable broad applicability. In the following paragraphs, we describe each step in detail (see Supplementary Material B1 for additional information).
First, we defined the relevance criteria and created a manual benchmark. To do so, we drew a random sample of 500 articles using the non-validated search term to manually classify whether climate change was the main topic of a given article. An article was deemed irrelevant if it failed to cover climate change and only mentioned it in passing or in terms of comparison; these articles fall under our conceptualization of noise. In total, our sample was balanced—i.e. 56.6% (n = 283) of the 500 articles were coded as relevant, while 43.4% (n = 217) were rated as not relevant.
Second, to develop a valid search term, we applied an integrated approach to create a comprehensive list of climate change-related termsFootnote5. First, we reviewed previous studies on climate change communication that employed search term sampling; it is important to note that the purpose of this specific review was to obtain an overview of frequently used terms in the field, not to blindly adopt these terms. Second, we created a DFM based on all articles from the manual benchmark that were coded as relevant (n = 283), and we conducted frequency analyses to inspect the most frequent terms (n = 500) and collocations (bi- and trigrams; min. occurrence of n = 20), such as carbon dioxide or greenhouse gas emissions. This ultimately led to a comprehensive list of climate change-related terms.
Third and finally, we performed the validation of search terms, or the calculation of performance metrics to ensure that the terms determined during the search term mining process returned as many relevant documents as possible without including too many false positives. To do this, researchers must determine satisfactory values for precision and recall prior to the analysis. For this study, we opted for a search term with equally high precision and recall, requiring that both values exceeded .8. We calculated performance metrics for up to 40 combinations of predetermined search terms (see Supplementary Material B1) by comparing the share of articles that cover climate change as their main topic (according to the manual benchmark) with the share of articles featuring the respective search terms (according to the automated classification). Ultimately, we decided on a search term that met our threshold, with values at precision = .86, recall = .83, F1 = .84.Footnote6 With regard to the subsequent analysis, our validated search term captured 83% of all relevant articles, and 86% of the articles retrieved by this search term truly deal with climate change communication. Nevertheless, this means that 14% of the articles are not relevant and therefore probably represent noise. However, the goal of this study was not to determine the “perfect” search term but rather to investigate whether researchers using a validated vs. a non-validated search term arrive at different results. More importantly, this approach transparently quantifies the ratio of meaningful data to noise, which also determines how confident researchers and readers can be about the validity of the obtained results. In contrast to the validated search term, only 56% of the documents retrieved by the non-validated search termFootnote7 represented the targeted discourse, which means that 44% are likely to be noise.
Step 2. Generating and comparing DFMs
In order to conduct our comparison at the DFM level, we carried out several preprocessing steps, all of which were applied identically to both the validated text corpus and the non-validated text corpus. First, we identified and removed duplicates by comparing whether the first 50 words of news articles were identical. Second, we used Part-of-Speech (POS) tagging to identify entities (min. occurrence of n = 50)—i.e. persons, organizations, locations, and noun phrases (bi- and trigrams, min. occurrence of n = 100); in addition, we extracted collocations (bi- and trigrams, min. occurrence of n = 1,000). All detected entities, noun phrases, and collocations were manually validated in order to exclude misclassifications or meaningless collocations. The concatenation of expressions split across multiple terms is of particular relevance, because a concept such as climate change, which contains two separate terms, carries a specific meaning (Lucas et al. Citation2015, 258). In a third step, we tokenized all other words to unigrams, applied lower-case conversion, and removed punctuation, numbers (expressions such as CO2 were included as compound tokens), and stop words using the list implemented in the R-package quanteda (Benoit et al. Citation2018). In addition, we extended this list by adding words unique to our corpora, such as indian express app. We deliberately decided not to eliminate pronouns or adverbs (cf. Campbell and Pennebaker Citation2003), nor to stem or lemmatize words, as these processes decrease the validity of the results (cf. Schofield and Mimno Citation2016). Finally, we applied relative pruning to eliminate extremely rare and frequent terms—i.e. terms that occurred in less than 1% or more than 99% of all news articles. The resulting validated and non-validated DFMs contained 5,133 and 5,077 unique terms, respectivelyFootnote8.
To compare both matrices, we first considered differences in ranks (each up to rank 100) resulting from the absolute frequencies of features. The simplest form of computational content analysis, frequency distribution analysis, often looks at the most commonly used words (e.g. Benoit Citation2020); as a result, variations in ranks can lead to different inferences. Second, we evaluated variations of individual features based on their relative frequencies. To do this, we calculated the differences in relative frequencies and the relative frequency ratio, i.e. the ratio of the relative word frequencies of the non-validated DFM and the validated DFM (Damerau Citation1993).
Step 3. Fitting and comparing topic models
To draw comparisons at this stage, we applied unsupervised machine learning using topic modeling with latent Dirichlet allocation (LDA; Blei, Ng, and Jordan Citation2003). Due to its mixed-membership approach, topic modeling assumes that a document contains several topics, represented by the distribution of words (Maier et al. Citation2018). To estimate a suitable number of topics (K) for the validated model and the non-validated model, we considered statistical fitness measures and estimated a span of reference models with 5 ≤ K ≤ 100 in steps of K = 5, using the R-package ldatuning (Nikita Citation2020). To further reinforce our decision, we calculated additional fitness assessments based on the structural topic model framework (Roberts, Stewart, and Tingley Citation2019). Specifically, we evaluated semantic coherence (i.e. internal consistency of a topic) and exclusivity (i.e. exclusivity of each topics’ top words) for reference models with 5 ≤ K ≤ 150 in steps of K = 5 (see Supplementary Material B2). In addition, we evaluated the qualitative interpretability of topics by looking at the topics’ top words and top articles. After conducting this procedure, we decided on K = 45 for the validated model and K = 80 for the non-validated model. It was important to perform the estimates for K separately—and not to choose the same K for better comparability—since the purpose of our approach is to show how two methodologically comparable but independent analyses differ with respect to potential inferences.
To assure robust topic model results, we estimated LDA prototypes (with R-package ldaPrototype, Rieger, Rahnenführer, and Jentsch Citation2020) on both DFMs. To determine the prototype, 100 LDA models were calculated using the same variable settings (alpha = beta = 1/K), and the model whose topics bore the greatest similarity to the topics of all other models was selected as the prototype. The LDA prototype thus ensures that the model is not an extreme or unstable expression of a certain constellation of variables (Rieger, Rahnenführer, and Jentsch Citation2020). The similarity measure for prototypes is the mean similarity to the other models (similarity of multiple sets by clustering with local pruning, S-CLOP): these values are .88 (validated model) and .83 (non-validated model) for the prototypes used here.
We first compared the topics of the two LDA prototypes by assessing the similarity of the word-topic matrices φ using cosine similarity (cf. Niekler and Jähnichen Citation2012) and the corresponding clustering function in the R-package tosca (Koppers et al. Citation2020). Following Reber’s (Citation2019) comparative approach, we then formed pairs from the topics of the two models that are most similar—i.e. for each topic in one model, the most similar topic in the other model was identified (multi-matches were possible). Based on the similarity values of these pairings, we determined topics that have no direct counterparts in the other model: these non-matches do not exhibit a cosine similarity of >.4 to any topic of the other LDA (cf. Reber 2019, 109). We also looked at the differences in the relative corpus share of the pairings, considering the evolution of highly similar or disparate topics. In addition, we manually classified topics as relevant (i.e. topics referring to climate change, its causes, consequences, solutions to tackle it, societal engagement, scientific studies, or assessment reports); topics that did not address climate change or only cited it as a reference or point of comparison were coded as noise. It is important to note that noise topics do not reflect a broader perspective or a different facet of the climate change discourse; rather, they clearly do not contain climate change as a primary topic. To this end, we evaluated the top 20 terms and articles with high probabilities for each topic. Intercoder reliability established for a random sample of 40 topics showed good agreement (Krippendorff’s α = .71; see Supplementary Material C2 for detailed information on the coding procedure).
Results
In line with our research design, this section is divided into three subsections. We start at the level of the text corpora (VQ1), continue with comparing DFMs (VQ2), and finish with topic model results (VQ3).
Comparison 1. Text Corpora
Comparing the validated and non-validated corpora shows that over a five-year period (2016–2020), both corpora equally covered 19 countries and spanned 56 and 55 online news outlets, respectively (see Supplementary Material B1 and C1 for detailed information). However, the number of news articles in each corpus differed greatly: while the non-validated search term retrieved 97,828 articles, the validated search term resulted in a corpus comprising 45,532 articles. As our validation already indicated, this difference can be (partly) explained by the fact that the validated search term performs better at excluding irrelevant, noisy articles than the non-validated search term.
In terms of the amount of news coverage over time, suggests that issue attention evolves very similarly in both corpora, with two meaningful differences. While the non-validated corpus suggests that issue attention to climate change reached its global peak in January 2017, the validated corpus indicates that this peak occurred in June 2017. Another difference appears in September 2017, when the non-validated corpus points to an upswing creating a local peak, whereas the validated corpus shows only a moderate rise. At the level of individual countries, further nuances become apparent (see Supplementary Material C1).
To sum up our findings for VQ1, and in particular to answer the question of whether researchers working with the validated corpus versus the non-validated corpus would have come to similar conclusions, our answer must be maybe. If researchers were focusing on the general patterns of issue attention cycles in news coverage, the answer would likely be yes; however, if researchers are particularly interested in identifying and investigating global or local peaks (for instance, the coverage of events associated with these peaks), our comparison indicates that it most likely makes a difference whether the validated or the non-validated search term is used.
Comparison 2. Document Feature Matrices
While the comparison of unique terms (non-validated DFM: 5,153 features, validated DFM: 5,077 features) indicates a high degree of congruence—i.e. 4,706 overlapping features—our analysis of the feature’s frequencies points to clear differences. Together, the two lists of the top 100 features results in 113 features, with an overlap of 87 features and 26 (2 times 13) distinct features. The list of distinct features alone is revealing, as it unveils that in the non-validated DFM, generic terms such as today, come, or right (distinct in the non-validated DFM) are overrated compared to thematically meaningful features such as coal, carbon, or temperatures (distinct in the validated DFM). Looking at the rankings of the individual features resulting from their absolute frequency confirms this finding: words that are comparatively underrated in the non-validated DFM are thematically associated with the relevance criteria of the search term, such as emissions (rank 18, validated DFM vs. rank 63, non-validated DFMFootnote9) or environmental (rank 40, validated DFM vs. rank 74, non-validated DFM). In contrast, generic words that are not directly associated with the relevance criteria are relatively overrated in the non-validated DFM, such as day (rank 91, validated DFM vs. rank 59, non-validated DFM) or think (rank 78, validated DFM vs. rank 52, non-validated DFM). It seems that the lower precision of the non-validated corpus causes generic terms to be more prevalent in the list of high-frequency features (which is possibly indicative of noise), while thematically relevant terms tend to be underrepresented.
An analysis of differences in relative feature frequencies confirms this finding: in the non-validated DFM, the relative share of climate-specific terms appears to be underrated (see ). In particular, features with a small share in the non-validated DFM exhibit a high relative frequency ratio: fossil and fuels, for example, occur 24 and 11 times more frequently, respectively, in the validated DFM than in the non-validated DFM.
In sum, and to answer VQ2, we again found similarities but also differences between the two search terms. While our comparison of unique features indicates a large overlap, the comparisons of the absolute and relative frequencies of features shows differences—specifically, that climate-specific words are underrated and generic terms are overrated in the less-precise non-validated DFM. Our answer to the question of whether scholars reach different conclusions when using word frequency or collocation lists based on the validated DFM versus the non-validated DFM—e.g. to examine the sentiment of or narrative structures in news media coverage—must again be maybe. However, what our comparison clearly demonstrated is that the DFMs that researchers would use for such analyses are different.
Comparison 3. Topical Content and Prevalence
A quick glance at the hierarchical dendrogram clustering (see Supplementary Material C2) shows that the topics with the largest mean distance from the others are those generated from the non-validated DFM. Matching topics with the most similar topics from the other corpus results in 80 (non-validated) and 45 (validated) pairings. The average cosine similarity is .63 for topic pairings of non-validated topics and .67 for those of validated topics. In fact, every topic from the validated model has at least a similarity of .49 to one of the non-validated topics, which means that according to the above definition (≤ .4), there is no non-match. The situation is different for the non-validated topics, two of which cannot be clearly assigned to a similar topic from the validated model. These topics’ top words indicate that they come from the thematically unrelated portion of the corpus: topic NV62 is about migration and human rights, especially women’s rights, and topic NV74 is about economic growth and economic cycles. An analysis of the most representative articles confirms that these topics are associated with texts that do not meet the relevance criteria for climate change.
To gain further insights into the content of the topics, we classified each as relevant or noise (see Supplementary Material C2), with the latter consisting of irrelevant (e.g. “foreign policy” (NV8) or “art” (NV35), which are not directly related to climate change) or meaningless topics (i.e. topics with generic words, e.g. “noise” (NV24)). It turned out that 14 topics (31%) in the validated model and 53 topics (66%) in the non-validated model failed our relevance criteria and only mentioned climate change in passing. This finding mirrors the diverging values of precision of the validated and non-validated search terms.
In addition to differences in topical content, our results revealed variations in topical prevalence. The most frequent topics in both models reveal different topic structures and prevalence (see ). In addition, similar topics have different relative corpus shares (see ), while parallel topic evolutions clearly correlate with topic similarity (see ).
Summarizing the information to answer VQ3 again points to similarities between the validated and non-validated search terms but more clearly than before underlines the differences. Although all topics from the validated model also appear in the non-validated one, researchers using the non-validated search term to assess topics and their prevalence in news media coverage are likely to arrive at different results than those who analyze texts obtained using the validated search term; the model of the former contains considerably more topics that feature weak or nonexistent connections to the research focus. In addition, if researchers want to assess the importance of topics based on their corpus share, those working with the non-validated corpus would most likely reach different conclusions than those working with the validated search term corpus. The additional noise in the non-validated corpus is not distributed equally across topics, and comparing the salience of particular aspects in the climate change discourse based on this corpus could lead to erroneous conclusions. With regard to an explorative use of scattershot search terms for topic models, only the interpretation of topics with a large corpus share seems robust, both in terms of content and in terms of their temporal evolution.
Discussion and Recommendations for Future Research
Advances in analytical text-as-data approaches as well as the availability of large-scale digital data have opened a plethora of new avenues for communication science and (digital) journalism research. One key challenge that comes with these developments concerns the validity of samples retrieved by (non-validated) search terms—something researchers seem to neglect. This is particularly surprising, given that distant reading makes it more likely that noise will slip in unnoticed at this stage of the research process, which can provoke methodological concerns regarding the representativeness of the data and the validity of the results. Thus, the primary goal of this study was to assess the extent of the use of non-validated search terms in combination with automated text methods (RQ1), and whether and how much this practice affects the results of such approaches (RQ2).
Conducting an interdisciplinary literature review, we found that the majority of studies do not use or report well-established performance measures to validate the quality of their search terms. This underscores the need to question whether researchers working with a validated search term would arrive at different results and conclusions than their colleagues who did not validate the search term they used, the latter of which might be less likely to retrieve documents that accurately represent the intended discourse. Built on a multi-step approach and an exploratory study of key research areas within the fields of (digital) journalism research and climate change communication, our answer to this question must be “it depends”. Specifically, we found overlaps between both corpora at all levels of comparison, but also considerable differences. For example, similarities were found in general patterns of issue attention over time, and all topics from the validated corpus were also found in the non-validated corpus. At the same time, differences were evident with respect to the global peak of media attention or word frequency lists, with climate-specific terms being underrated in the non-validated corpus. In addition, we found differences in topical content and prevalence in news coverage: the non-validated model contained almost twice as many noise topics as the validated model. Furthermore, differences were evident in the structure and the corpus share of the most prevalent topics.
Given these results, it is crucial to point out that the consequences of (not) validating search terms may vary depending on the analytic procedures and data, and that search terms chosen in a scattershot manner do not necessarily perform poorly. For instance, Jünger and Gärtner (Citation2021) were able to test substantial hypotheses despite highly noisy data. Although some readers of this study might argue that the differences we found are not of great concern—perhaps even negligible—we would contend that researchers can hardly account and control for noise in their data, especially when working with large datasets and automated approaches, since it is not feasible to manually inspect where exactly noise is hiding and which results are affected by systematic biases. We would also argue that post-hoc validation checks are not an appropriate means to detect and eliminate noise: while researchers can certainly manually filter out noise topics a posteriori, they cannot determine whether and where additional noise is included in supposedly relevant topics, nor can the prevalence of topics be corrected by excluding noisy documents. Hence, performance measures during the collection of data are needed in order to ensure that the data actually represent the intended discourse or latent construct. This not only provides a transparent and controlled way of sampling but also increases confidence in the representativeness of the data and the validity of the results. In summary, we conclude that a scattershot approach of using non-validated search terms, in combination with certain methods, can give initial clues and make sense for data exploration; however, we would argue that making final inferences based on insufficiently validated search terms poses the risk that the results may be affected or obscured by noise.
The paper at hand should be considered in light of limitations that may guide future research. First, we assessed the consequences of using non-validated search terms based on descriptive analyses; future studies may want to extend this view. Second, since our case study only drew on digital news coverage, future research should compare different types of data. Third, far beyond the validation of search terms, researchers are faced with different decisions to make throughout the entire research process, such as various preprocessing steps; thus, to assess the impact of the entire universe of researchers’ degrees of freedom on the results of automated content analyses, future studies should employ multiverse analyses (Pipal, Song, and Boomgaarden Citation2022).
We conclude this article by providing best practice recommendations to improve the practice of search term sampling (following and expanding Lacy et al. Citation2015; Stryker et al. Citation2006). Importantly, these recommendations are not restricted to utilizing search terms for data-gathering purposes; for instance, studies that apply off-the-shelf dictionaries or customize organic dictionaries may benefit from implementing these low-threshold guidelines as well. Accordingly, we recommend that researchers: (1) formalize the validation process—that is, carefully protocol every step and decision taken prior to and during data collection; (2) define the universe of relevant data beforehand, which includes an explicit definition of relevance criteria that have to be met; (3) triangulate inductive and deductive approaches, such as frequency or keyness analyses, to identify the most indicative terms during search term mining, including a consideration of semantic changes of concepts over time as well as varying national or cultural contexts; (4) carefully validate search terms identified in search term mining by using well-established performance metrics such as precision, recall, and F1-score; and (5) transparently state all methodological details of this process.
Following these steps improves not only the validity of datasets but also the reproducibility and robustness of scientific findings, to counter the less transparent or unsystematic application of search term sampling. Moreover, establishing these guidelines as standard quality criteria and implementing them in educational curricula would enhance the application of text-as-data approaches in and beyond the field of (digital) journalism research.
Supplemental Material
Download PDF (584.7 KB)SupplementaryMaterialR3_DJ.docx
Download MS Word (327.1 KB)Acknowledgements
We would like to thank our two anonymous reviewers, the editorial team, as well as Valerie Hase, Katharina Kleinen-von Königslöw, Laura Laugwitz, and Jonas Rieger whose thorough comments tremendously improved this article. In addition, we would like to thank Remon Sadikni for granting us access to the Online Media Monitor (OMM) to collect our data.
Disclosure Statement
No potential conflict of interest was reported by the author(s).
Additional information
Funding
Notes
1 For an overview ranging from rule-based approaches (e.g. dependency parsers) and off-the-shelf and organic dictionaries, to supervised (e.g. support vector machines) and unsupervised machine learning (e.g. topic modeling), see Benoit (Citation2020) or Boumans and Trilling (Citation2016).
2 It is worth noting that human-coded benchmarks are not without their pitfalls (DiMaggio Citation2015; Song et al. Citation2020).
3 Supplementary material is available at the project’s OSF repository, https://osf.io/qd3ah/?view_only=641abb335e1f409ba1036a01349fd502. The preregistration of our study can be found here, https://osf.io/qvmk5. Deviations from our preregistration are transparently described and justified in Supplementary Material A1.
4 We are indebted to an anonymous reviewer for this apt term and the helpful differentiation between search term mining and search term validation.
5 In addition, we applied various automated keyword extraction strategies, although these were inferior to the approach outlined here (see Supplementary Material B1).
6 To test the possibility that the optimization of the search term led to overfitting, we recalculated performance metrics on 200 additional randomly selected and manually coded articles. This showed that our search term is slightly overfitted, with a precision of .70, a recall of .83, and a F1-score of .76.
7 It is important to note that we could not calculate recall for the non-validated search term and therefore cannot compare this value for both search terms: to do so, we would have needed a search term even broader than the terms climat* chang* OR global warm*. However, we deemed this sufficient for the purpose of our study.
8 Both the DFMs and the replication codes for analyses based on the DFMs can be accessed via the project’s OSF repository, https://osf.io/qd3ah/?view_only=641abb335e1f409ba1036a01349fd502.
9 The lower the rank, the higher the absolute frequency of the features.
References
- Baden, Christian, Christian Pipal, Martijn Schoonvelde, and Mariken A. C. G. van der Velden. 2022. “Three Gaps in Computational Text Analysis Methods for Social Sciences: A Research Agenda.” Communication Methods and Measures 16 (1): 1–18. https://doi.org/10.1080/19312458.2021.2015574.
- Barberá, Pablo, Amber E. Boydstun, Suzanna Linn, Ryan McMahon, and Jonathan Nagler. 2021. “Automated Text Classification of News Articles: A Practical Guide.” Political Analysis 29 (1): 19–42. https://doi.org/10.1017/pan.2020.8.
- Benoit, K. 2020. “Text as Data: An Overview.” In The SAGE Handbook of Research Methods in Political Science and International Relations, edited by Luigi Curini, Robert J. Franzese, and James F. Adams, 461–497. Los Angeles, London, New Delhi, Singapore, Washington DC, Melbourne: SAGE reference.
- Benoit, K., Kohei Watanabe, Haiyan Wang, Paul Nulty, Adam Obeng, Stefan Müller, and Akitaka Matsuo. 2018. “Quanteda: An R Package for the Quantitative Analysis of Textual Data.” Journal of Open Source Software 3 (30): 774. https://doi.org/10.21105/joss.00774.
- Blatchford, Annie. 2020. “Searching for Online News Content: The Challenges and Decisions.” Communication Research and Practice 6 (2): 143–156. https://doi.org/10.1080/22041451.2019.1676864.
- Blei, D. M., A. Y. Ng, and M. I. Jordan. 2003. “Latent Dirichlet Allocation.” Journal of Machine Learning Research 3: 993–1022.
- Bohr, Jeremiah. 2020. “Reporting on Climate Change: A Computational Analysis of U.S. Newspapers and Sources of Bias, 1997–2017.” Global Environmental Change 61: 102038. https://doi.org/10.1016/j.gloenvcha.2020.102038.
- Boumans, Jelle W., and Damian Trilling. 2016. “Taking Stock of the Toolkit: An Overview of Relevant Automated Content Analysis Approaches and Techniques for Digital Journalism Scholars.” Digital Journalism 4 (1): 8–23. https://doi.org/10.1080/21670811.2015.1096598.
- Boyd, Danah, and Kate Crawford. 2012. “Critical Questions for Big Data: Provocations for a Cultural, Technological, and Scholarly Phenomenon.” Information, Communication & Society 15 (5): 662–679. https://doi.org/10.1080/1369118X.2012.678878.
- Brüggemann, M., and R. Sadikni. 2020. “Online Media Monitor on Climate Change (OMM): Analysis of Global Tweets and Online Media Coverage.” https://icdc.cen.uni-hamburg.de/en/omm-mediaanalysis.html.
- Campbell, R. Sherlock, and James W. Pennebaker. 2003. “The Secret Life of Pronouns: Flexibility in Writing Style and Physical Health.” Psychological Science 14 (1): 60–65. https://doi.org/10.1111/1467-9280.01419.
- Damerau, Fred J. 1993. “Generating and Evaluating Domain-Oriented Multi-Word Terms from Texts.” Information Processing & Management 29 (4): 433–447. https://doi.org/10.1016/0306-4573(93)90039-G.
- de Vries, Erik, de Martijn Schoonvelde, and Gijs Schumacher. 2018. “No Longer Lost in Translation: Evidence That Google Translate Works for Comparative Bag-of-Words Text Applications.” Political Analysis 26 (4): 417–430. https://doi.org/10.1017/pan.2018.26.
- Denny, Matthew J., and Arthur Spirling. 2018. “Text Preprocessing for Unsupervised Learning: Why It Matters, When It Misleads, and What to Do About It.” Political Analysis 26 (2): 168–189. https://doi.org/10.1017/pan.2017.44.
- DiMaggio, Paul. 2015. “Adapting Computational Text Analysis to Social Science (And Vice Versa).” Big Data & Society 2 (2): 205395171560290. https://doi.org/10.1177/2053951715602908.
- Findley, Michael G., Kyosuke Kikuta, and Michael Denly. 2021. “External Validity.” Annual Review of Political Science 24 (1): 365–393. https://doi.org/10.1146/annurev-polisci-041719-102556.
- Gupta, Shivani, and Atul Gupta. 2019. “Dealing with Noise Problem in Machine Learning Data-Sets: A Systematic Review.” Procedia Computer Science 161: 466–474. https://doi.org/10.1016/j.procs.2019.11.146.
- Hase, Valerie, Daniela Mahl, and Mike S. Schäfer. 2022. “Der „Computational Turn“: Ein „Interdisziplinärer Turn“? Ein Systematischer Überblick Zur Nutzung Der Automatisierten Inhaltsanalyse in Der Journalismusforschung.” Medien & Kommunikationswissenschaft 70 (1–2): 60–78. https://doi.org/10.5771/1615-634X-2022-1-2-60.
- Hase, Valerie, Daniela Mahl, Mike S. Schäfer, and Tobias R. Keller. 2021. “Climate Change in News Media Across the Globe: An Automated Analysis of Issue Attention and Themes in Climate Change Coverage in 10 Countries (2006–2018).” Global Environmental Change 70: 102353. https://doi.org/10.1016/j.gloenvcha.2021.102353.
- Jacobi, Carina, Katharina Kleinen-von Königslöw, and Nel Ruigrok. 2016. “Political News in Online and Print Newspapers.” Digital Journalism 4 (6): 723–742. https://doi.org/10.1080/21670811.2015.1087810.
- Jang, S. Mo, and P. Sol Hart. 2015. “Polarized Frames on “Climate Change” and “Global Warming” Across Countries and States: Evidence from Twitter Big Data.” Global Environmental Change 32: 11–17. https://doi.org/10.1016/j.gloenvcha.2015.02.010.
- Jünger, Jakob, and Chantal Gärtner. 2021. “Distilling Issue Cycles from Large Databases: A Time-Series Analysis of Terrorism and Media in Africa.” Social Science Computer Review 39 (6): 1272–1291. https://doi.org/10.1177/0894439320979675.
- Jünger, Jakob, S. Geise, and M. Hänelt. 2022. “Unboxing Computational Social Media Research: From a Datahermeneutical Perspective: How Do Scholars Address the Tension Between Automation and Interpretation?” International Journal of Communication 16: 1482–1505.
- Karlsson, Michael, and Helle Sjøvaag. 2016. “Introduction: Research Methods in an Age of Digital Journalism.” Digital Journalism 4 (1): 1–7. https://doi.org/10.1080/21670811.2015.1096595.
- King, Gary, Patrick Lam, and Margaret E. Roberts. 2017. “Computer-Assisted Keyword and Document Set Discovery from Unstructured Text.” American Journal of Political Science 61 (4): 971–988. https://doi.org/10.1111/ajps.12291.
- Kirilenko, Andrei P., and Svetlana O. Stepchenkova. 2014. “Public Microblogging on Climate Change: One Year of Twitter Worldwide.” Global Environmental Change 26: 171–182. https://doi.org/10.1016/j.gloenvcha.2014.02.008.
- Koppers, Lars, Jonas Rieger, Karin Boczek, and Gerret von Nordheim. 2020. Tosca: Tools for Statistical Content Analysis. https://CRAN.R-project.org/package=tosca.
- Lacy, Stephen, Brendan R. Watson, Daniel Riffe, and Jennette Lovejoy. 2015. “Issues and Best Practices in Content Analysis.” Journalism & Mass Communication Quarterly 92 (4): 791–811. https://doi.org/10.1177/1077699015607338.
- Laugwitz, Laura. 2021. “Qualitätskriterien Für Die Automatische Inhaltsanalyse. Zur Integration Von Verfahren Des Maschinellen Lernens in Die Kommunikationswissenschaft.” https://osf.io/preprints/socarxiv/gt28f/.
- Lovejoy, Jennette, Brendan R. Watson, Stephen Lacy, and Daniel Riffe. 2014. “Assessing the Reporting of Reliability in Published Content Analyses: 1985–2010.” Communication Methods and Measures 8 (3): 207–221. https://doi.org/10.1080/19312458.2014.937528.
- Lucas, Christopher, Richard A. Nielsen, Margaret E. Roberts, Brandon M. Stewart, Alex Storer, and Dustin Tingley. 2015. “Computer-Assisted Text Analysis for Comparative Politics.” Political Analysis 23 (2): 254–277. https://doi.org/10.1093/pan/mpu019.
- Maier, Daniel, A. Niekler, Gregor Wiedemann, and Daniela Stoltenberg. 2020. “How Document Sampling and Vocabulary Pruning Affect the Results of Topic Models.” Computational Communication Research 2 (2): 139–152. https://doi.org/10.5117/CCR2020.2.001.MAIE.
- Maier, Daniel, A. Waldherr, P. Miltner, G. Wiedemann, A. Niekler, A. Keinert, B. Pfetsch, et al. 2018. “Applying LDA Topic Modeling in Communication Research: Toward a Valid and Reliable Methodology.” Communication Methods and Measures 12 (2–3): 93–118. https://doi.org/10.1080/19312458.2018.1430754.
- Manning, Christopher D., and Hinrich Schütze. 2002. Foundations of Statistical Natural Language Processing. 5th ed. Cambridge, MA. MIT Press.
- Nerlich, Brigitte, and Nelya Koteyko. 2009. “Compounds, Creativity and Complexity in Climate Change Communication: The Case of ‘Carbon Indulgences.” Global Environmental Change 19 (3): 345–353. https://doi.org/10.1016/j.gloenvcha.2009.03.001.
- Niekler, A., and P. Jähnichen. 2012. “Matching Results of Latent Dirichlet Allocation for Text.” http://asv.informatik.uni-leipzig.de/publication/file/210/nieklerjaehnichenICCM2012.pdf.
- Nikita, M. 2020. Tuning of the Latent Dirichlet Allocation Models Parameters: Comprehensive R Archive Network (CRAN). https://cran.r-project.org/web/packages/ldatuning/index.html.
- Pipal, Christian, Hyunjin Song, and Hajo G. Boomgaarden. 2022. “If You Have Choices, Why Not Choose (And Share) All of Them? A Multiverse Approach to Understanding News Engagement on Social Media.” Digital Journalism: 1–21. https://doi.org/10.1080/21670811.2022.2036623.
- Reber, U. 2019. “Overcoming Language Barriers: Assessing the Potential of Machine Translation and Topic Modeling for the Comparative Analysis of Multilingual Text Corpora.” Communication Methods and Measures 13 (2): 102–125. https://doi.org/10.1080/19312458.2018.1555798.
- Rieger, Jonas, Jörg Rahnenführer, and Carsten Jentsch. 2020. “Improving Latent Dirichlet Allocation: On Reliability of the Novel Method LDAPrototype.” In Natural Language Processing and Information Systems, Vol. 12089, edited by Elisabeth Métais, Farid Meziane, Helmut Horacek, and Philipp Cimiano, 118–125. Cham: Springer International Publishing. http://link.springer.com/. 10.1007/978-3-030-51310-8_11. Accessed March 26, 2021.
- Rieger, Jonas. 2020. “LdaPrototype: A Method in R to Get a Prototype of Multiple Latent Dirichlet Allocations.” Journal of Open Source Software 5 (51): 2181. https://doi.org/10.21105/joss.02181.
- Rinke, Eike Mark, Timo Dobbrick, Charlotte Löb, Cäcilia Zirn, and Hartmut Wessler. 2022. “Expert-Informed Topic Models for Document Set Discovery.” Communication Methods and Measures 16 (1): 39. https://doi.org/10.1080/19312458.2021.1920008.
- Roberts, Margaret E., Brandon M. Stewart, and Dustin Tingley. 2016. “Navigating the Local Modes of Big Data: The Case of Topic Models.” In Computational Social Science: Discovery and Prediction, edited by R. M. Alvarez, 51–97. Analytical methods for social research. Cambridge: Cambridge University Press.
- Roberts, Margaret E., Brandon M. Stewart, and Dustin Tingley. 2019. “Stm: An R Package for Structural Topic Models.” Journal of Statistical Software 91 (2): 1–40. https://doi.org/10.18637/jss.v091.i02.
- Scharkow, Michael. 2012. “Automatisierte Inhaltsanalyse Und Maschinelles Lernen.” Dissertation at Berlin University of the Arts. epubli. https://opus4.kobv.de/opus4-udk/frontdoor/deliver/index/docId/28/file/dissertation_scharkow_final_udk.pdf.
- Schofield, Alexandra, and David Mimno. 2016. “Comparing Apples to Apple: The Effects of Stemmers on Topic Models.” Transactions of the Association for Computational Linguistics 4: 287–300. https://doi.org/10.1162/tacl_a_00099.
- Sobel, M., and Daniel Riffe. 2015. “U.S. Linkages in New York Times Coverage of Nigeria, Ethiopia and Botswana (2004-13): Economic and Strategic Bases for News.” International Communication Research Journal 50 (1): 3–22.
- Song, Hyunjin, Petro Tolochko, Jakob-Moritz Eberl, Olga Eisele, Esther Greussing, Tobias Heidenreich, Fabienne Lind, Sebastian Galyga, and Hajo G. Boomgaarden. 2020. “In Validations We Trust? The Impact of Imperfect Human Annotations as a Gold Standard on the Quality of Validation of Automated Content Analysis.” Political Communication 37 (4): 550–572. https://doi.org/10.1080/10584609.2020.1723752.
- Stray, Jonathan. 2019. “Making Artificial Intelligence Work for Investigative Journalism.” Digital Journalism 7 (8): 1076–1097. https://doi.org/10.1080/21670811.2019.1630289.
- Stryker, Jo Ellen, Ricardo J. Wray, Robert C. Hornik, and Itzik Yanovitzky. 2006. “Validation of Database Search Terms for Content Analysis: The Case of Cancer News Coverage.” Journalism & Mass Communication Quarterly 83 (2): 413–430. https://doi.org/10.1177/107769900608300212.
- Vogelgesang, Jens, and Michael Scharkow. 2012. “Reliabilitätstests in Inhaltsanalysen.” Publizistik 57 (3): 333–345. https://doi.org/10.1007/s11616-012-0154-9.
- Walter, Stefanie. 2019. “Better Off Without You? How the British Media Portrayed EU Citizens in Brexit News.” The International Journal of Press/Politics 24 (2): 210–232. https://doi.org/10.1177/1940161218821509.
- Wilkerson, John, and Andreu Casas. 2017. “Large-Scale Computerized Text Analysis in Political Science: Opportunities and Challenges.” Annual Review of Political Science 20 (1): 529–544. https://doi.org/10.1146/annurev-polisci-052615-025542.
- Zhu, Xingquan, and Xindong Wu. 2004. “Class Noise Vs. Attribute Noise: A Quantitative Study.” Artificial Intelligence Review 22 (3): 177–210. https://doi.org/10.1007/s10462-004-0751-8.