Abstract
Background: Care for people with amyotrophic lateral sclerosis (ALS) has altered at King’s College Hospital over the last 20 years. The clinic has been a multidisciplinary, specialist, tertiary referral centre since 1995 with a large team with integrated palliative and respiratory care since 2006. We hypothesised that these changes would improve survival. Methods: In this retrospective observational study, patients diagnosed with El Escorial definite, probable and possible ALS between 1995–1998 and 2008–2011 were followed up. The primary outcome measure was a chi-square test for the proportion of each cohort surviving. Kaplan-Meier survival analysis and Cox multivariate regression were secondary analyses. Results: There was low reporting of some interventions. Five hundred and forty-seven people were included. Survival between the cohorts was significantly different (p = 0.022) with a higher proportion surviving during 2008–2011. Survival time was 21.6 (95% CI 19.2–24.0) months in the 2008–2011 cohort compared to 19.2 years (15.6–21.6) in the 1995–1998 cohort (log rank p = 0.018). Four hundred and ninety-three cases were included in the Cox regression. Diagnostic cohort was a significant predictor variable (HR 0.79 (0.64–0.97) p = 0.023). Conclusions: These results support the hypothesis that integrated specialist clinics with multidisciplinary input improve survival in ALS.
Introduction
Amyotrophic lateral sclerosis (ALS) is a neurodegenerative disease in which there is progressive loss of spinal and cortical motor neurons leading to death from respiratory failure often within a median of two years (Citation1,Citation2). Because of the devastating nature of the disease and the impact on patients and families, specialist clinics with expertise in ALS have developed, usually with an associated multidisciplinary team (MDT), the aim being to improve quality and quantity of life through better medical and social care. As well as multidisciplinary care, three other interventions are widely used in ALS. Riluzole is used to prolong survival, but the effect is modest (Citation3,Citation4). There is evidence that non-invasive ventilation (NIV) as a treatment for neuromuscular respiratory failure also improves survival as well as quality of life (Citation5), and gastrostomy is used for dysphagia impacting nutritional intake (Citation6).
Riluzole, non-invasive ventilation and gastrostomy, have only been in use for the last 20 years, and in widespread use more recently still, largely through the medium of multidisciplinary teams. Although riluzole can be prescribed by a neurologist, in the UK, NIV and gastrostomy for ALS are usually accessed through an MDT allied to a specialist clinic or community team, since they are used at advanced stages of ALS when needs are complex and multifaceted.
Clinical data on those attending a specialised tertiary care centre in South East London have been collected since 1995 as part of the South East ALS (SEALS) register. The King’s MND service was initiated in 1989 and formally established with multidisciplinary input as the King’s MND Care and Research Centre in 1995. However, full integration of specialist nursing, diet planning, physiotherapy, speech and language therapy, and especially with respiratory, psychological, and palliative care services was not achieved until around 2006. Because of the significant experience base of the team tempered by geographical confinement, a key strategy has been exchange of knowledge with local care teams, similar to other specialist MDTs across the country and in modern healthcare systems worldwide.
Two studies based in Ireland have previously shown that multidisciplinary care improves quality and quantity of life (Citation7,Citation8). In England, a study comparing survival of people treated at a general neurology clinic with to those at a specialised multidisciplinary care clinic also showed the specialised clinic improved survival, although in Italy a population based study found there was no difference between people seen in clinics with MDTs and those not (Citation9,Citation10). It has been proposed that improved survival is a result of the accurate timing of interventions by specialist teams (Citation7). The importance of timing of interventions has also been shown by prognostic modelling (Citation11).
We therefore sought to assess whether survival and care provision has changed over time by comparing disease duration in people diagnosed and managed within the early, small MDT, and those seen 10 years later and managed within the expanded team with more sophisticated treatment protocols.
Materials and methods
Data sources
Data were obtained from the SEALS Register, which has been described previously. This register is now part of the MND Register for England, Wales and Northern Ireland which was approved by the South East London Research Ethics Committee on 01/06/2015 study number 15/LO/0810.
is a consort diagram that summarises the numbers in each cohort and how many records were available for analysis.
Study design
We chose two time-periods to study, one beginning in 1995, soon after clinic inception, and the other in 2008, selected to allow comparison between an evolving MDT and a more integrated and complete MDT. Power analysis showed that 199 cases in each group would allow detection of a 10% difference in survival at p = 0.05. We therefore selected four-year windows following each time-period, so that cohort 1 represented all people diagnosed between 1 January 1995 and 31 December 1998, and cohort 2, all people diagnosed between 1 January 2008 and 31 December 2011. Cohort 2 was followed for survival to 16 May 2016. To prevent bias from very long survivors, cohort 1 was followed for survival to the equivalent date, 16 May 2003. Survival time was calculated from date of diagnosis, as this is the earliest multidisciplinary care starts, to death or end date. Where people were lost to follow-up, the date of the most recent appointment was used as the end date. Riluzole and NIV data were collected where available. Data on gastrostomy were not available for the first cohort, so were not compared between the cohorts. For cohort 1 the only NIV data available were from people who were started on NIV at the tertiary centre. Cohort 2 included everyone who was set up with NIV at the tertiary centre and a few with documented follow-up where NIV was set up locally. In both cases the numbers of people on NIV are low so it is likely that cases are missing to follow-up.
Statistical methods
Chi square testing of the proportion of people surviving at the end of each time-point was performed. Univariate survival analysis was by Kaplan-Meier product limit distribution using the log rank test. Multivariate analysis was by Cox regression. The assumption of proportional hazards was checked by assessing whether diagnostic cohort was a time-dependent variable, and by assessing a log minus log survival against log survival timegraph. For multivariate analysis, we extracted sex, age of onset, site of onset (bulbar, spinal or other), onset to diagnosis interval (ODI), date of death or last follow-up, clinical phenotype (ALS, flail arm variant ALS, flail limb variant ALS), El Escorial category, riluzole use. Riluzole use was categorised using ‘intention to treat’ methodology. If someone had taken it once and then stopped, this would still be categorised as use. Where any variables were missing from a case record, that record was excluded from analysis involving that variable. Statistical analyses were carried out using IBM SPSS v22.0 (Citation12). was created using R version 3.3.1 with packages ‘ggplot2', ‘survival', ‘RcmdrPlugin.KMggplot2' (Citation13–17). All variables except sex were included in the Cox analysis as these have previously been described as having an effect on survival. Including sex as a covariate did not change the model. For comparison between El Escorial categories, as there were no ‘clinically-probable laboratory supported’ cases in the 1995 cohort, ‘probable’ and ‘clinically-probable laboratory supported’ were grouped together as ‘probable’ and analysed together throughout. Analysing the data with ‘clinically-probable, laboratory supported’ grouped with ‘possible’ did not change the model. We performed a sensitivity analysis to account for the possibility of missing NIV data by labelling the top 50% of survivors in each cohort as having received NIV.
Figure 2. Kaplan-Meier graph showing proportion of people alive at different time points. The dotted line is the 2008-2011 cohort, the solid line shows the 1995–1998 cohort. We have shown the 95% confidence intervals on the graph, indicated by the shaded areas and dotted lines. Overlap of the confidence intervals does not demonstrate lack of statistical significance (Citation24).
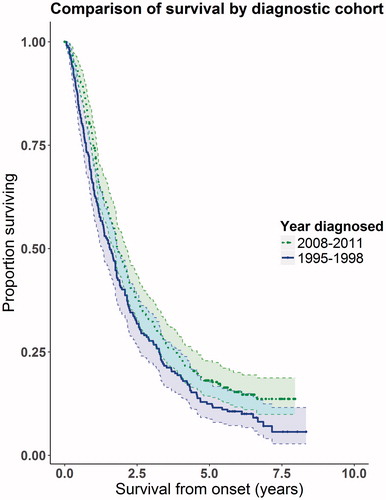
Results
Demographic characteristics of the two cohorts are shown in . Median time from onset to diagnosis was a month higher in cohort 2, and riluzole and NIV use were significantly higher in this cohort. Otherwise the two groups were similar.
Table 1. Summary statistics showing the case mix of each cohort.
There was a greater percentage of people alive in cohort 2 than cohort 1 at the endpoint of the study, 15% in cohort 2 compared with 8% in cohort 1 and this difference was significant (p = 0.02).
The median survival time was 19.2 (95% CI 15.6–21.6) months for cohort 1 compared with 21.6 (95% CI 19.2–24.0) months for cohort 2 (log-rank test p = 0.018).
shows the Kaplan-Meier survival curves generated. Because three important prognostic factors, riluzole, NIV use, and diagnostic delay, were not balanced between the two cohorts, we performed a multivariate analysis using a Cox proportional hazards survival model; the results are summarised in .
Table 2. Results of the Cox proportional hazards survival analysis. The El Escorial Category ‘clinically-probable, laboratory supported’ only appeared in cohort 2 (2008–2011) and was grouped together with ‘probable’ for the analysis. Site of onset was classified into bulbar and spinal. Spinal onset included people with respiratory and limb onset. One person had cognitive onset reported only, and as the diagnosis was of ALS rather than a bulbar subtype, they were classed as spinal onset ALS.
The Cox regression model demonstrated a survival benefit for those people in cohort 2 (HR 0.79 (95% CI 0.64–0.97) p = 0.023) that could not be explained only by increased use of riluzole, NIV or increased diagnostic delay. Sensitivity analyses were performed to test whether the grouping of explanatory variables used (described in the Methods and legend) changed the outcome of the model with respect to the cohort. We found that cohort remained an independent predictor of survival in the model and being diagnosed in the later cohort was beneficial for survival. Recoding the top 50% of survivors in each cohort as NIV users made no difference to cohort being a significant variable in the model, despite NIV becoming a significant covariate in the Cox regression.
The test of proportional hazards was met based on graphical criteria. Additionally, running the Cox regression with diagnostic cohort as a time- dependent covariate showed that there was no significant interaction with time (p = 0.62).
The model explained approximately 3.8% of the variation in survival times for the cohorts, with age of onset accounting for more than 2% and diagnostic cohort just 0.1%, although this is still more than the contribution of riluzole ().
Table 3. Variation in data explained by model. Pseudo r-squared values were calculated using McFadden’s method (Citation18). The total variation was calculated using McFadden’s method on the overall model and by adding the calculation from each explanatory variable. As they vary slightly both values are presented here.
Discussion
There was a significant improvement in survival time of people who were diagnosed with ALS in the four years from January 2008 compared with those diagnosed in the four years from January 1995. Taking into account other confounding covariates, people diagnosed with ALS in the more recent cohort survived 2.5 months longer than those in the earlier cohort. In an exploratory analysis restricted to people with a diagnosis of ALS (excluding flail arm and flail leg variants), the median survival difference was 4.5 months higher in cohort 2 than cohort 1 (16.1 (95% CI 13.0–17.2) compared with 20.6 (18.4–22.8)) and this was also significant (log-rank p-value = 0.007) and was a significant predictor in the multivariate analysis using the same covariates as in our main model (HR 0.74 (95% CI 0.60–0.92)). This study supports previous studies that have shown that multidisciplinary care improves survival in people with ALS (Citation7–9).
This study uses a different design from most previous studies because we have tested the same centre at different time-points before and after a fully integrated MDT was in place, rather than different centres at the same times, one with and one without an MDT. Our study examines the effect of a highly specialised large MDT with integrated services, coordination with local teams, and implementation of cutting edge practice into standard of care. However, the use of historical controls for comparison of survival estimates may be flawed if unmeasurable systemic differences between cohorts cause the shift in disease trajectory, rather than the factor of interest.
Some cohort differences can be controlled for by inclusion of baseline characteristics in multivariate analysis. The only baseline variable that was significantly different between the groups was diagnostic delay, which was longer in cohort 2. It is not clear why this is. Higher population levels may lead to more pressure on the healthcare system increasing waiting times or, as education and outreach in the area cause increase in local clinician speciality, a formal confirmation at the tertiary centre may not be as urgent for initiation of some care. In support of this possibility, cohort 2 was larger than cohort 1 by 113 people. A relationship between high patient volumes and better outcomes is well documented, although it has not been studied extensively in ALS (Citation19). It is not clear whether care is improved through the extra experience gained, whether better services receive more referrals through reputation, or if both of these are true, representing a positive feedback loop (Citation20). Alternatively, it could be another artefact of increased population levels. Finally, specialist centres may increase knowledge and recognition of the disease. This is predicted to shift the population under study from more prevalent to incident, and if this is the case, we may be underestimating the survival difference (Citation21).
A strength of this study is that the centre acts as its own control. All the specialists seeing patients from cohort 1 were also seeing patients from cohort 2. Thus, differences in survival cannot be attributed to centre effects or systemic differences in practice. However, this also leads to a weakness of the study, since care itself has changed over the time-period studied, with use of non-invasive ventilation (NIV) and nutritional intervention with gastrostomy now part of routine care. NIV use has been steadily increasing over time. In 2010 the UK National Institute for Health and Clinical Excellence published the NIV guideline for MND which stated that NIV was cost effective.
We included available NIV data in the Cox proportional hazards model as people on NIV had improved survival as shown by Kaplan-Meier analysis and the provision of NIV recorded between cohorts was significantly different. There was almost no change to the Cox proportional hazards model with or without NIV status, but this may be because of missing data. NIV is set up in the tertiary centre which is where the NIV data for this study come from. Due to the low levels of NIV set up in this study we estimate we are missing data from community services. It has been shown that for people with respiratory impairment, NIV can improve median survival by 48 days, and maintain quality of life scores, with a differential benefit for those without significant bulbar involvement (Citation5). The difference in survival between our cohorts was 105 days, over twice the benefit reported for NIV. Furthermore, our sensitivity analysis in which we artificially boosted the effect of NIV, making it a significant predictor of survival, did not alter the survival advantage of the second cohort. We therefore cautiously conclude that the MDT provides benefits that are additional to provision of interventions alone, although trial evidence on the benefits of early NIV is needed to confirm this. Regardless, the improvement in survival can be taken to show that, since it is likely that gastrostomy and NIV improve outcomes, the specialist team uses these treatment strategies and others effectively.
Although we were able to fit a highly significant survival model, the variation in survival time the model variables could explain was low. This is not unusual in modelling. For example, despite highly significant genetic associations in a genome-wide study of seven million genetic variants in 40,000 people, only about 10% of heritability is explained, with almost none explained by highly significant associations (Citation22). Many different genetic, environmental and other factors influence ALS survival, explaining why the proportion of variance explained by our model is low (Citation23).
We have shown that survival of people attending a specialist ALS clinic has improved over a 13-year period, and that this effect is probably not fully accounted for by improvements in treatments available during that time. This supports the notion that specialist integrated multidisciplinary care provides benefit in ALS that can be measured in survival improvement. It provides evidence that integration of palliative care services, respiratory care and wheelchair therapists, along with an MDT meeting, can improve outcomes in ALS. Further work is needed to determine whether the benefit of an MDT also results in improved quality of life.
Declaration of interest
The authors have no conflicts of interest to declare.
Acknowledgements
This paper represents independent research part funded by the National Institute for Health Research (NIHR) Biomedical Research Centre at South London and Maudsley NHS Foundation Trust and King’s College London. Funding was also received by the Motor Neurone Disease Association and NIHR Collaboration for Leadership in Applied Health Research and Care South London at King’s College Hospital NHS Foundation Trust. This is an EU Joint Programme – Neurodegenerative Disease Research (JPND) project. The project is supported through the following funding organisations under the aegis of JPND – www.jpnd.eu (United Kingdom, Medical Research Council (MR/L501529/1) and Economic and Social Research Council (ES/L008238/1)). The work leading up to this publication was funded by the European Community’s Health Seventh Framework Programme (FP7/2007–2013; grant agreement number 259867), Horizon 2020 Programme (H2020-PHC-2014-two-stage; grant agreement number 633413) and the Charities Research Motor Neurone and Motor Neurone Disease Association. The funding sources played no role in the preparation of this manuscript.
Funding
The funding grant number is MNDA Al-Chalabi/Mar15/931-794 Al-Chalab. The grant was supplied by the Motor Neuron Disease Association.
References
- Chio A, Logroscino G, Hardiman O, Swingler R, Mitchell D, Beghi E, et al. Prognostic factors in ALS: a critical review. Amyotroph Lateral Scler. 2009;10:310–23.
- Talbot K. Motor neuron disease: the bare essentials. Pract Neurol. 2009;9:303–9.
- Bensimon G, Lacomblez L, Meininger V. A controlled trial of riluzole in amyotrophic lateral sclerosis. ALS/Riluzole Study Group. N Engl J Med. 1994;330:585–91.
- Miller RG, Mitchell JD, Moore DH. Riluzole for amyotrophic lateral sclerosis (ALS)/motor neuron disease (MND). Cochrane Database Syst Rev. 2012;3:CD001447.
- Bourke SC, Tomlinson M, Williams TL, Bullock RE, Shaw PJ, Gibson GJ. Effects of non-invasive ventilation on survival and quality of life in patients with amyotrophic lateral sclerosis: a randomised controlled trial. Lancet Neurol. 2006;5:140–7.
- Stavroulakis T, Walsh T, Shaw PJ, McDermott CJ. Gastrostomy use in motor neurone disease (MND): A review, meta-analysis and survey of current practice. Amyotroph Lateral Scler Frontotemp Degener. 2013;14:96–104.
- Rooney J, Byrne S, Heverin M, Tobin K, Dick A, Donaghy C, et al. A multidisciplinary clinic approach improves survival in ALS: a comparative study of ALS in Ireland and Northern Ireland. J Neurol Neurosurg Psychiatry. 2015;86:496–501.
- Traynor BJ, Alexander M, Corr B, Frost E, Hardiman O. Effect of a multidisciplinary amyotrophic lateral sclerosis (ALS) clinic on ALS survival: a population based study, 1996-2000. J Neurol Neurosurg Psychiatry. 2003;74:1258–61.
- Aridegbe T, Kandler R, Walters SJ, Walsh T, Shaw PJ, McDermott CJ. The natural history of motor neuron disease: assessing the impact of specialist care. Amyotroph Lateral Scler Frontotemporal Degener. 2013;14:13–9.
- Zoccolella S, Beghi E, Palagano G, Fraddosio A, Guerra V, Lepore V, et al. ALS multidisciplinary clinic and survival. Results from a population-based study in Southern Italy. J Neurol. 2007;254:1107–12.
- Knibb JA, Keren N, Kulka A, Leigh PN, Martin S, Shaw CE, et al. A clinical tool for predicting survival in ALS. J Neurol Neurosurg Psychiatry. 2016;87:1361–1367.
- IBM. IBM Inventorspss Advanced Statistics; 2016.
- Sou T, Nagashima A. RcmdrPlugin.KMggplot2: R Commander Plug-in for Data Visualization with 'ggplot2'. 2016.
- T T. _A Package for Survival Analysis in S_. version 2.38. 2015.
- Team RC. R: A language and environment for statistical computing. Vienna, Austria: R Foundation for Statistical Computing; 2016.
- Therneau T, Grambsch PM. Modeling Survival Data: Extending the Cox Model. New York: Springer; 2000.
- Wickham H. ggplot2: Elegant Graphics for Data Analysis. New York: Springer-Verlag; 2009.
- McFadden D, Conditional logit analysis of qualitative choice behavior. Frontiers in Economics. New York: Academic Press; 1974.
- Gandjour A, Bannenberg A, Lauterbach KW. Threshold volumes associated with higher survival in health care: a systematic review. Med Care. 2003;41:1129–41.
- Luft HS, Hunt SS, Maerki SC. The volume-outcome relationship: practice-makes-perfect or selective-referral patterns? Health Serv Res. 1987;22:157–82.
- Hardiman O, Chalabi AA, Brayne C, Beghi E, van den Berg LH, Chio A, et al. The changing picture of amyotrophic lateral sclerosis: lessons from European registers. J Neurol Neurosurg Psychiatry. 2017. [Epub ahead of print]. doi: 10.1136/jnnp-2016-314495
- van Rheenen W, Shatunov A, Dekker AM, McLaughlin RL, Diekstra FP, Pulit SL, et al. Genome-wide association analyses identify new risk variants and the genetic architecture of amyotrophic lateral sclerosis. Nat Genet. 2016;48:1043–8.
- Zufiría M, Gil-Bea FJ, Fernández-Torrón R, Poza JJ, Muñoz-Blanco JL, Rojas-García R, et al. ALS: A bucket of genes, environment, metabolism and unknown ingredients. Progr Neurobiol. 2016;142:104–29.
- Cumming G, Finch S. Inference by eye: confidence intervals and how to read pictures of data. Am Psychol. 2005;60:170–80.