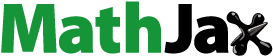
ABSTRACT
This paper focuses on the interactions between social capital and production structure in their relation with regional inequality. Combining survey data with available regional databases for the EU-15, it provides a panel data analysis of multidimensional inequalities based on the idea that social capital is a fundamental factor determining its regional levels, along with the economic specialization of regions. Results confirm an inverse relation between inequality and social capital. At the same time, they highlight the positive impact of production clusters on the regional environment. Findings suggest that the joint effect of predictors is ambiguous, and may so be dependent on the different sorts of local specialization and social capital endowment.
INTRODUCTION
This paper contributes to the debate on regional disparities, and how they can possibly be reduced. Drawing upon both economic and sociological literature, it provides a multidimensional study of the role of social capital in the socioeconomic dynamics of regions.
Many previous analyses of inequality have inferred on its interaction with social capital. The direction of the relation is still not clear, but an inverse one has often been recognized to be in place (Barone & Mocetti, Citation2016; De Blasio & Nuzzo Citation2012; d’Hombres, Elia, & Weber, Citation2013). In fact, social capital is still a vaguely defined concept, which in the socioeconomic literature identifies a complex variety of elements, such as civic involvement and the participation of citizens (Putnam, Citation1993), level of trust (Fukuyama, Citation1995), and different kinds of social relations (Bourdieu, Citation1986; Coleman, Citation1988).
The sociological analysis has naturally devoted a higher concern to the conceptual definition of its theoretical construct. The economic analysis has instead been more interested in its connections with economic variables (Barone, de Blasio, & Sestito, Citation2014). Many scholars have studied social capital in its positive effects on growth (Beugelsdijk & van Schaik, Citation2001, Citation2005, Citation2009; Helliwell & Putnam, Citation1995; Whiteley, Citation2000). At the regional scale, this often translated to digging into the ties social capital has been proved to have with the regional production structure, especially with clusters of industries and their impulse on local virtuous dynamics (Knack & Keefer, Citation1997; Rodríguez-Pose, Citation1998). Social capital indeed facilitates production diversification, and industries’ relatedness to form clusters (Boschma, Cortinovis, Xiao, & van Oort, Citation2016), which are assumed to boost regional performance substantially (Porter, Citation1990). All its constituting elements seem to make it more likely that ‘mere co-location is leveraged through active collaboration and that beneficial local conditions are strengthened through coordinated or joint action’ (Ketels & Protsiv, Citation2013, p. 8).
These complementary research interests have, however, rarely been combined in regional studies. On the one hand, most of the inequality analyses dealing with the link between social capital (i.e., those principally intended with regards to its social network definition) and economic inequalities often search for their drivers into the labour market structure, mainly focusing on the labour supply side. A lot has been written on wage inequalities and top income shares, and their intergenerational transmission; or about investments in human capital and returns to education, and the mechanisms governing social networks (Atkinson, Citation2007, Citation2013a, Citation2013b; Flap, Citation1991; Granovetter, Citation1974). Less has been researched about the influence of the labour demand side on different sort of inequalities (Atkinson, Citation1983).
On the other hand, studies exploring the production structure, especially at the regional level, have investigated the relation between social capital and growth. One aspect that is still mostly unclear is the trigger for a favourable environment to turn into a successful cluster location. Here the local endowments of social capital come into play: their importance as a driver of performances’ difference across locations has been increasingly recognized (Celata, Citation2009; Rodríguez-Pose & Crescenzi, Citation2008). Furthermore, as Rodríguez-Pose (Citation1998) wrote about the role of ‘local social structures’ when explaining the persistence of regional disparities in Europe, the existing social conditions may play an essential role in an area’s receptivity to and assimilation of technological change and economic processes. In addition, policy-makers are always more concerned with innovative clusters as a good way of boosting regional economic performance, promoting local development to leverage lagged regions, and so reduce territorial disparities (e.g., the European Union’s (EU) regional Cohesion Policy and the Smart Specialisation Strategies (S3); European Commission, Citation2014). Can we say these growth spillovers are actually inclusive, though? If we agree that places are made of people and they are the last recipients of any policy intervention in the end, the development of a territory can be considered equitable or not based on the implications for citizens’ well-being, and tackling spatial imbalances should not disregard the individual level of related inequalities.
The present research attempts to reconcile these close themes. The underlying hypothesis lies in the intuition that the effects social capital has, inversely with inequality and directly with growth, may be further researched by exploring the nexus between its regional endowments and the locally associated economic specialization. Therefore, the main research questions ask what are the effects of specific regional characteristics, alike social capital endowments, on multidimensional inequality in the EU-15? and what is the role of production structure within this connection?
More liveable regions are assumed to be those with a lower level of inequality and a higher level of social capital (Alesina & La Ferrara, Citation2002; Putnam, Citation1993).Footnote1 In this case, more flexible and adaptive business environments and production structures may occur on one side, and more cohesive and reactive social infrastructures and networks on the other. All this can hold also subject to other regional characteristics, such as the quality of institutions and infrastructures. Especially in the literature on urban sociology and city planning, the role of physical space and infrastructure endowments are recognized as relevant in determining differentials of individuals’ quality of life and community development (Park, Burgess, & McKenzie, Citation1925; Pastor, Sanchez-Lopez, & Ito, Citation2015).
Previous exploratory analyses of considered variables (Parente, Citation2017) provided evidence of the fact that regions where social capital are higher (lower) than average also have lower (higher) level of loss in human development (HD), together with better performances in terms of quality of local institutions, gross domestic product (GDP) and innovation. All this seems to be almost regardless of the regional degree of urbanization.
Some subsequent hypotheses see local specializations influencing the levels of inequality differently, based on: (1) the sort of social relations and cultural effects that productive specialization allows to foster; and (2) the spatial interactions that these effects can engender within related territories. In order to test these relations, a longitudinal analysis on data for about 205 regions in the period 2000–11 was applied.
Owing to the several approaches available for defining these complex concepts, it is worth clarifying how they were here measured. Inequality is intended as that of outcomes, specifically with regards to the multidimensional framework of HD economics and capability approach (Alkire & Foster, Citation2010; Sen, Citation1987, Citation2009). An attempt to consider at least the trust (Fukuyama, Citation1995) and networks (Putnam, Citation1993) components of social capital together was done. The definition of production clusters here assumed refers to the concept of agglomeration of firms, and especially to the extensive research carried out at the Stockholm School of Economics (Ketels & Protsiv, Citation2013; Ketels, Sölvell, & Lindqvst, Citation2008). Territorial unit of analysis is set at the EUROSTAT NUTS-2 regions, within the EU-15 only.Footnote2
The paper is structured as follows. The next section provides a more detailed discussion of the theoretical structure behind the investigated variables. The third section explains the data and methodology. The fourth section shows the results obtained by econometric analyses. The final section summarizes the main findings and draws some conclusions.
THE ROLE OF REGIONAL SOCIAL CAPITAL AND INDUSTRIAL CLUSTERS
Despite the increasing attention it has captured in social sciences, social capital remains a not clearly defined concept (Portes, Citation1998). It represents a set of components such as interpersonal trust, reciprocity norms and social relations. It has often been explained by referring to its relation with other concepts – especially that of social networks (Baker, Citation1990; Burt, Citation1992) – as well as by means of the values its components pertain to, they being either universalistic or particularistic values. On the one hand, it has meant general ethic norms are universally applied; on the other, social behaviours differ based on the network within they take place, for example, inside or outside family ties (De Blasio, Scalise, & Sestito, Citation2014). Nevertheless, it remains unclear if these components are all on the same level, or if they are self-reinforcing and cumulative (Dasgupta & Serageldin, Citation2000). Major studies and attempts at conceptualization came from Bourdieu (Citation1986), Coleman (Citation1988), Putnam (Citation1993, Citation2000) and Fukuyama (Citation1995). A main distinction in their theorizations can be found, first of all, in the reference to the individual or collective level. Bourdieu was the first to thoroughly define social capital as the set of effective and potential resources deriving from a stable social network. Similarly, Coleman identified it with the structure itself of networks between people, quantifiable with the skills deriving from socialization. Otherwise, Putnam and Fukuyama emphasized the collective resources of social capital. Even if Fukuyama places the accent more on its moral aspect and the values of trust, both of them imply that social capital is something related to the elements of a better society as a whole. Civic virtue and civicness, associationism, trustworthiness and organizational efficiency all come from shared reciprocity norms and collective initiatives taken by mutual agreement (Vergati, Citation2014).
In spite of their different focus of analysis, all authors agree on qualifying social capital as the basic prerequisite for the well-functioning of collective actions and social cooperation, strengthening connectedness among people, and favouring the emergence of a wide variety of social relations (van Schaik, Citation2002).
Especially, Putnam (Citation2000) insisted on the central relevance of such elements as mutual trust and social networks to favour prosperity at individual, firm, neighbourhood and country level. In addition, he considered it as the basis for democracy to work (Putnam, Citation1993), and as directly related to the quality of the institutions and of social cohesion to which the society might aim at (Putnam, 2004). However, what is the effect of different kinds of social capital on the level of inequality? Moreover, how does the extent of inequality affect the formation of social capital in a region? Here a generally negative association has been suggested, with higher levels of inequality accompanied by lower performances in social capital. The direction of the relation is less clear, and the kind of social capital investigated does matter a great deal. A distinction between bridging and bonding functional effects has been highlighted (Narayan, Citation2002; Putnam, Citation2000). The latter embodies horizontal connections, among equals within a community (likewise cohesiveness), whereas the former is vertical between communities. Higher endowments of bonding networks may easily lead to an inverse relation with inequality within the community members only, resulting in an augmented overall inequality between members of different communities (e.g., socioeconomic classes, hampering social mobility and increasing inequality in its sociological definition; a good example is Banfield, Citation1958; for a review, see Portes, Citation1998; and Vergati, Citation2000). Stronger bridging networks appear to be always positively beneficial instead (Narayan, Citation2002; Putnam, Citation1993).
De Blasio and Nuzzo (Citation2012) and Barone and Mocetti (Citation2016) produced interesting evidence in both senses about Italian regions. They found a significant association between several inequality measures and social capital indicators. The relation is negative in the case of bridging and linking ones, while positive for the bonding networks. An interesting aspect is that these associations seem to pass through – and be more evident in – the labour market. Several drivers can explain the negative association that goes from a low level of social capital (or a bonding one stronger than the bridging/linking ones) to a higher level of inequality. The authors highlight how these may be a lower productivity due to professionally unfair behaviour and higher costs borne by firms to counter them; inefficient job matching because of the prominent use of personal networks in job searches; poor propensity to entrepreneurship in an unfavourable business environment; and strong family ties that hamper women’s employment.
These considerations are some of the reasons why a look at the economic production structure and labour demand side may be of relevance in this regard. Soft elements of the competitive environment, such as social capital, are more likely to emerge as driving forces of development in a regional system, and appear easier to capture in a local rather than a national setting (Aranguren et al., Citation2010). An interesting piece of research on the role of different kinds of social capital in regional diversification and production specialization is by Boschma et al. (Citation2016). Drawing on previous work about economic payoffs of social capital by Knack and Keefer (Citation1997), Zak and Knack (Citation2001), Beugelsdijk and van Schaik (Citation2001, Citation2005) and Beugelsdijk and Smulders (Citation2009), Boschma et al. (Citation2016) found that bridging social capital is a key driver for regional diversification with ‘trust and active participation in bridging type of groups increase the probability of regions to diversify into new sectors’ (p. 24).
Indeed, regional specialization paths may be explained in terms of agglomeration externalities depending on the interactions between co-working firms. Specialization in just a small amount depends on territorial specificities, while a major role is played by industrial dynamics such as the localization ones Alfred Marshall described. As Marshall (Citation1920) was able to intuit, the proximity of firmsFootnote3 is not the whole story for them to work, and the process for a long-lasting and fair local development entails other components. This is also about the social climate, the so-called territorial milieu that permeates the industrial districts he could observe. Entrepreneurial environment and social norms deeply rooted in the places of industrialization can make a great difference and be the trigger for firms’ co-location spot to transform it into a Marshallian district, which embeds a specific amount of urbanization and localization externalities.
Since Marshall’s (Citation1920) precursory studies, further different approaches have been elaborated in the frame of regional studies’ spatial analysis (Becattini, Citation1990, Citation2000, Citation2009; Boschma & Giannelle, Citation2014; Gordon & McCann, Citation2000; Iammarino & McCann, Citation2006; Porter, Citation2003a, Citation2003b; Porter, Stern, & Artavia Lorea, Citation2013), and the role of firms’ clusters as engines of development has thus become relevant. The renewed interest in their impact on growth and regional progress became central again, especially during the 1990s, after Porter’s and Krugman’s (Citation1990, Citation1991) work. The object of the study had come to be the relation between presence of clusters and local (and national) economic performance. A positive association between the two has been emphasized, and Porter has contributed largely to the generalization of the industrial district concept. In his theory of clusters of firms, their relational network is one fundamental element of the business strategy: competitive advantages are not so much dependent on internal size but mostly on the degree of openness to external relations that favour knowledge exchange and circulation. In this framework, the cluster is defined as a ‘concentration of interconnected firms, specialised providers, services’ suppliers, firms in related sectors and concerned institutions (e.g., universities, standards agencies, trade associations), which compete and cooperate at the same time’ (Porter, Citation1998, p. 197). Despite the fact that some consider Porter’s school imperfect,Footnote4 its analytical clarity has made it suitable for use in an institutional setting, and has been adopted in both the United States and the EU as framework to cluster-mapping’s exercise (which to date are still the only available homogeneous source of statistics on regional production clustersFootnote5).
All these academic contributions are indeed significantly relevant for their policy implications. In Europe, when talking about regional disparities and the policy interventions tackling them, EU Cohesion Policy is actually the related tool for European regions (Barca, Citation2009). Recently, it has been always more concerned with innovative industries, and their role within place-based S3. This policy framework is here relevant for at least three reasons. First, it provides the reference for the need for innovative development policies at the regional level, emphasizing the innovation dimension as a good way to lever lagged regions. Based on this, regions are called on always more attention to locally established strong specialization and innovations, and may be classified as leaders and followers according to their innovation pace (Hollanders et al., Citation2014). But what about the effects on the multidimensional inequalities within the regions in which these industries are located?
Second, it is tightly linked to the cluster policies already in place. Despite some substantive differences in the theory and scope of the two, S3 and clusters show undoubted similarities. Existing clusters in many cases embed important elements of the entrepreneurial discovery process that S3 aim to foster (Aranguren & Wilson, Citation2013). At the same time, innovative clusters are a classic outcome (or an emergent property) of S3 (Foray, David, & Hall, Citation2011).
Third, elements of social capital come to be central here once again. In fact, a main feature of S3 is it being an outcome of an entrepreneurial discovery process emerging from a consultation among local stakeholders. The kind and strength of social networks, working environments and reciprocity norms could definitely turn the tide of the process, subject to the quality of local institutions in pursuing their supporting role as well.
It has been in this area that some interesting contributions specific to the role of clusters in a sustainable regional growth in the EU have been produced (Ketels & Protsiv, Citation2013). Drawing on related US literature and evidence framework (Porter, Citation2003a, Citation2003b), Ketels and Protsiv’s (Citation2013) goal was to test if European regions where clusters and cluster initiatives are present have better performance in environmental and social outcomes as compared with the economic ones. They found they did. Nevertheless, clusters can be considered just as indicator of conditions that contribute to the outcomes of a more sustainable growth path and not their root cause.
DATA AND METHODOLOGY
To carry out the empirical analyses, data from different sources were merged. The European Social Survey (ESS, Citation2013) was used as the primary source for social capital endowments. It is a large scale, cross-country and repeated survey that provides information on the socioeconomic characteristics, beliefs, preferences and values of people in the surveyed countries. Considered survey items relate to interpersonal trust and social networks. These were identified by the reported level of trust, of fairness and of helpfulness for the first concept; and people stating unpaid work in organizations or political parties for the second. In particular, data about declared work for political parties were considered as a proxy for bonding social capital; while data for other organizations were taken as a proxy for bridging social capital (along the lines of Boschma et al., Citation2016; and Knack & Keefer, Citation1997). The aggregate mean value of the two was used as a measure for the social network element of social capital. Original microdata from the ESS responses were previously treated. Reported levels of trust were collapsed by regional medians and then aggregated by a simple arithmetic mean. The percentage average of respondents above the identified level of trust was considered as the regional indicator for it. As for unpaid work for political parties and other organizations, affirmative answers were reshaped in the form of percentages and then aggregated by regional averages, too.
As regards regional production specializations, these were considered by means of the results of previous exploratory analysis on the European Cluster Observatory (ECO) database.Footnote6 Information about 41 clusters’ categories (extracted as location quotients – LQs) were reduced to two indicatorsFootnote7 by means of principal component analysis (PCA) (Parente, Citation2017). Components were interpreted based on both the loading components recorded on each of the 41 clusters and the measured correlation of regional component scores to characteristics of regions.Footnote8 The two components were considered as indices for diversification and innovative specialization of the regional production structure in related industrial clusters.
As regards regional inequalities, the main measure used here as a proxy for multidimensional inequality is the percentage loss in HD due to the inequalities present in society (Alkire & Foster, Citation2010; UNDP, Citation2016, Citation2018). This measure has the pro of going beyond the traditional ones for inequality, focusing not only on the income distribution but also on those in additional domains considered fundamental by the HD economics approach (i.e., health and education). Matching Organisation for Economic Co-operation and Development (OECD) and Statistical Office of the European Communities (EUROSTAT) databases with the European Union Statistics on Income and Living Conditions (EU-SILC) survey and following Kovacevic (Citation2010), it was calculated yearly in the period 2000–11 per 205 selected regions comparing the estimated human development index (HDI) with its adjustment to within-region inequalities (Parente, Citation2018). The adjustment is operated through the estimation of Atkinson measures of inequality in the achievements of each of the three considered domains covered by the HDI (i.e., health, education and income). As explained by the authors who developed the inequality-adjusted HDI (IHDI) methodology now in use,Footnote9 the Atkinson index has a clear meaning: it is the share of per capita achievement wasted as a consequence of inequalities in the distribution of achievements (Salvareda, Nolan, & Smeeding, Citation2011). As a result, the deriving measure of loss gives information on the inequality in the distribution of a more comprehensive achievement of the development process instead than of the income’s alone.
The three estimated Atkinson indices were considered as well, both separately and in the form of their simple arithmetic mean (i.e., the coefficient of human inequality). Moreover, a Gini index was calculated on disposable incomes from the same data set.
Finally, two additional variables were used to control for the quality of institutions and local geography. The first was captured by the European quality of governance index (EQI) produced by the Quality of Government Institute (Charron, Dijkstra, & Lapuente, Citation2014). Absent, or discontinued, any related indicators at the considered regional level such as land use, infrastructures, accessibility of regions and geography were included by means of the degree of urbanization. The reason for this is the implicit amount of information that this variable communicates related to urbanization externalities and agglomeration dynamics.Footnote10
summarizes the variables deployed in the analysis presented below. The upper section reports all the measures of inequalities alternatively used as dependent variables in the estimation; the lower section includes all the explanatory variables.
Table 1. Variables and descriptive statistics.
A first descriptive assessment of correlations between considered variables confirms the conclusions suggested by the literature. Social capital is negatively associated with inequality indices (significantly up to –61%) and positively with quality of government (around 65%). Differences emerge when (social capital is) considered with distinction between (its) components. Within social networks, bridging relational ties are inversely associated with inequality (around 45% for the income one), while the bonding ones are always directly correlated instead ().
Table 2. Correlation between core variables.
On the basis of the theoretical framework presented above, some hypotheses on the relations between selected predictors were formulated to be tested. The model specification and its estimation were developed accordingly with a stepwise approach by a forward selection:
Hypothesis 1: The inverse relation, generally accepted by the literature, between income inequality and (many elements of) social capital can be extended to the case of a multidimensional measure of inequality.
Hypothesis 2: The relation tested by Hypothesis 1 may be boosted by higher levels of both diversification into industrial clusters and specialization of clusters’ business environment.
Hypothesis 3: The institutional environment is relevant and good local governance can help reduce inequalities, for example, by providing an equal setting of opportunities.
Hypothesis 4: The degree of urbanization, land use and infrastructures’ assets can change the patterns, lowering or increasing inequalities depending on the level of inclusiveness and relatedness they can facilitate.
This specification was replicated by means of both fixed effects and generalized least square regression with random effects. This allowed one to assess the validity of estimated coefficients, the consistency of the specified model and to search for the most efficient estimates. Equation (1) in matrix form for FE then becomes:(2)
(2) where
is the time-invariant
regressor matrix;
is the unobserved time-invariant entity effect (i.e., the region-specific intercept); and
is the error term; and for RE becomes:
(3)
(3) which adds the possibility to being able distinguish between the between-entity error
and the within-entity error
.
A crucial distinction between the two models is whether the unobserved entity effect includes elements that are correlated with the regressors (Greene, Citation2008). Fixed effects are designed to study the causes of changes within a person or entity, hence they will account for variations within regions. A time-invariant characteristic cannot cause such a change because it is constant for each unit, and that is why it is omitted from the analysis (Kohler & Kreuter, Citation2009).
A key issue with respect to the choice is therefore , which can be checked using the Hausman specification test comparing the coefficient vectors from two estimators (Stock & Watson, Citation2007). It is thus used to check for endogeneity, and in the case of panel data analysis it is useful to detect which is better between random and fixed effects (Baum, Citation2006). As the null hypothesis is the absence of correlation, fixed effects are the best fit in case it is rejected. It was thereby performed on the models specified by equations (2) and (3); it failed to reject the null hypothesis.
Another fundamental assumption of the regressions model is that the error terms are normally distributed, such as . In this specific case, though, the distribution of residuals cannot be normal because the dependent variable does not range on the entire real numbers’ axis. Since the estimated measure of multidimensional inequality (i.e., the dependent variable in the model) is limited on the [0–1] interval by construction, so will be the distribution of errors. To account for this constraint, a further specification of the model was run in order to check for its consistency. A truncated regression, limited on the pertaining interval, was estimated. The significance of the results did not change, and outputs are shown together with the previous specifications’ ones in the following sections.
Finally, the risk of endogeneity had to be addressed. The level of inequality in a region may be thought to inversely affect trust or social network, too. It is also true that when inequality is lower, prosocial behaviours are more likely to occur. Macro-studies usually conclude that income inequality depresses social capital, while micro-studies seem to produce more contrasting results (d’Hombres et al., Citation2013). Owing to these considerations, some precautions were adopted. The seven available ESS survey waves were taken with a time lag of two years as against the dependent variable, so to limit the risk of reverse causality and of endogeneity in the model. Since social capital is assumed to have an ambiguous direction with the levels of inequality, its lagged values were preferred. This excludes the simultaneous effects inequality may have on social capital and limits the risks of endogeneity for this variable.
Furthermore, some additional checks for this occurrence were performed on the model estimates. The significant correlation of residuals to other predictors of the model, and their interactions, was tested through bivariate regressions, and both excluded. Also, omitted variables bias was checked by the total variance inflation factors, and it gave negative results.
RESULTS
reports the coefficients of the first estimated regression to test for Hypothesis 1, comparing outputs of the relation with social capital for different measures of inequality.
Table 3. Regressions estimates and standard errorsa for all dependent variables.
The first finding worth mentioning is that trust is always inversely related with inequality and statistically significant (at 95% confidence interval), except for the Atkinson measure calculated on life expectancy’s distribution. Social networks result in an inverse relation, too, and statistically significant, except for one occurrence. Looking at the magnitude of the effects, it is interesting to note how the social networks are stronger than trust, especially for income inequality: it is doubled for the Atkinson measure, and even more for the Gini. This might reflect the considerations suggested by the literature about the transmission of income inequality (even intergenerational) that sees in the labour market and social networks some of its main drivers.
At the same time, the quality of government always shows a significantly negative effect. This confirms the assumption that the institutional setting is an essential prerequisite for fairer societies.
Another interesting result is that health inequality appears as the dependent variable that works worst for this specification of the model. This might be related to the fact that the variables still omitted are more explanatory of concealed drivers of inequality for this domain than for that of income and education. The latter are much more interrelated with the kind of socioeconomic characteristic of regions considered in this analysis, and consequently with the associated labour market mechanisms.
In order to test the other hypotheses, predictors on production structure and their interaction with social capital variables were added. summarizes the results for the complete model specified in equation (1).
Table 4. Regressions estimates and standard errorsa for all dependent variables.
The results of the descriptive analyses seem to be confirmed, showing the relevance of the considered relations, with the exception of the degree of urbanization. The results confirm the major findings from the literature as well. The relation between social capital variables and inequality appears to be always an inverse one. Hypothesis 1 is significant again: it is confirmed for both the loss in HD and the human inequality coefficient. A more relevant effect seems to be that of social networks.
Looking at Hypothesis 2, related coefficients also report an inverse relation with inequality. When production specialization occurs through clusters and innovative business environments, inequality reduces by two units. Diversification may be facilitated by better endowments of trust on the one hand. Higher levels of specialization and a more dynamic business environment may facilitate social capital’s spillovers through voluntary work and informal exchanges on the other. In fact, considering the interaction between the two blocks of predictors, results say it is significant, too. Recalling the model of equation (1), the explicit form of the interaction effects becomes the following:(4)
(4) The presence of a significant interaction indicates that the effect of one predictor variable on the response variable is different at different values of the other predictor. If there were no interaction term, β would be interpreted as the unique effect of trust on inequality. While β is now interpreted so only when cluster diversification is equal to zero and the effect of trust on inequality is now (β + ϕ). Because of the interaction, the effect of having higher levels of trust is different if a region has more or less diversification of its production structure into clusters of related industries.Footnote11 The term γ is instead the effect of production diversification when trust is equal to zero. The term (γ + ϕ) is different at every one of the infinite values of trust. The empirical relationship between production structure and inequality may then be ambiguous. Previous hypotheses assumed it to be dependent on the kind of social capital (bridging or bonding) related to the nature of the specialization spillovers per each economic activity.
Results seem to support Hypothesis 3. An increase in the quality of local government may mean a decrease in the level of inequality. The outcome for Hypothesis 4 instead fails to be significant. Despite evidence of the fact that more highly densely populated areas usually embed higher levels of inequality (Glaeser, Resseger, & Tobio, Citation2008), the regional scale may have made it less effective in capturing the information of interest, because it misses the spatial concentration dynamics associated with it. To address this shortage, two other options were considered: a dummy variable for the presence of a capital city in the region; and an indicator for the most populous cities. However, none produced better results.
Goodness of fit measured by R2 is always > 0.60, meaning the amount of variance of inequality explained by selected predictors is significant enough ().
RE coefficients include both the within- and between-region effects. In the case of social capital, it would mean that for each change of one unit of trust across years and between regions, inequality would decrease by 1% on average per 1% more people reporting a level of trust higher than the regional median, as per 3% more people working in voluntary organizations ().
Table 5. Random and fixed effects compared, coefficients and standard errors.a
In FE, the impact of social networks appears slightly increased and that of trust reduced. Owing to time-invariant availability of data, this model specification fails to account for the quality of institutions (Tables and ). Also, the errors ui are assumed here to be correlated with the regressors, and the standard deviation (SD) within their groups is always low, while a very high intraclass correlation suggests wide disparities across panels (Baltagi, Citation2008).
Table 6. RE and FE on Atkinson measures in single dimensions, coefficients and standard errors.a
Goodness of fit measured by the F- and Wald χ2-tests that account for coefficients in the model to be different from zero are always significant, meaning that the specification is relevant.
Based on all this, the model specification under equation (1) can be considered significant, and according to the performed statistical tests, the RE one specified by equation (3) should be assumed to be the best fit.
CONCLUSIONS
In conclusion, the analysis in this paper confirms an inverse relation between inequality and social capital, extending the findings of many studies on income inequality to a multidimensional measure of it. At the same time, the need for a distinction between different components of social capital is highlighted. Findings state that the magnitude of the effect may be higher for social networks than trust. In terms of policy, this is relevant in the extent it supports the institutional setting and actions adopted in favour of associationism and civic society organizations, which can be considered a fundamental pillar of more cohesive and less unequal societies. Still, it has to be acknowledged that the size of these effects slightly changes according to the indicator used to measure inequality. It is no coincidence that in the case of income inequality (and in the aggregate indices that include it), social networks play a major role, while when considering the inequality in education or health, trust is the element that matters the most. In line with existing studies on this (Barone & Mocetti, Citation2016; De Blasio & Nuzzo, Citation2012), the labour market appears to be the reasonable driver for the interactions between social capital (inevitably more in terms of social networks) and inequalities.
In addition, results highlight the positive impact of industrial clusters on regional environment. Findings suggest that the joint effect of predictors is ambiguous, though. In particular, with regards to the regional production structure, being able to distinguish between social capital elements and their relation with specialization in different economic activities would be necessary. Having an intuition of which production sectors are more reactive to the influence of social capital would be valuable in terms of policy. It could help sustain economic activities that are proved to be more supportive of social endowments, along with related training and education.
The scale of the analysis may have played a role in understanding the considered dynamics. Interactions associated with the kind of production specialization, and evidence of the effects of social capital of individuals, are certainly more tangible elements in a urban environment. Also, the spatial effects of these phenomenon were lost within the wider regional level adopted in the case of the analysis presented in this paper. Infrastructure accessibility and land-use patterns would have provided a more solid basis upon which to interpret the studied relations than the degree of urbanization at such a broad scale (Ganong & Shoag, Citation2017).
Some considerations can be done with respect to the interaction this variable may have with other predictors. As stated, the results could also be very much susceptible to the physical geography of the considered spaces. And this is also relevant in terms of level of governance. Since the architecture of (urban) places is proved to have an impact on favouring, or not, knowledge spillovers and exchange (Florida, Citation2005; Lefebvre, Citation1991), regional and urban planning (Iacofano & Goltsman, Citation2007) aware of the issue and coordinated with the local industrial specialization could better support the ideal mix of socioeconomic activities to be implemented. The ultimate goal would be that of exploiting these positive effects in order to reinforce local social capital endowments, and in this way reduce the associated multidimensional inequalities. In this regards, the evidence provided by Glaeser, Laibson, and Sacerdote (Citation2002) about the higher personal investments in social capital by individuals who are employed in more sociable occupations (e.g., knowledge intensive services) could be relevant. The impossibility to derive a significant indicator for separate levels of the variables, and a substantive lack of detailed data about organizations where unpaid work was conducted by surveyed people, made it not possible in this exercise.Footnote12
Although there are the mentioned limits, the analysis presented in this paper proves the possibility of combining profitably approaches specific to economics of inequality, sociology and economic geography into one multidisciplinary study on regional inequalities, and extending them to multidimensional measures of socioeconomic inequalities the findings generally considered about the sole income one.
Owing to the questions it leaves unanswered, further research may integrate these results using a qualitative analysis at a lower territorial level to match them with more punctual information covering sparse (or missing) dimensions. Another interesting question concerns the level of mutual influence between elements of social capital in their relation with inequalities. Moreover, an improved formulation for the hypotheses should account separately for economic specializations that foster bonding (bridging) social capital and low (high) interest in space creation associated with higher (lower) inequality (e.g., high-tech clusters, also due to their selective requirements in terms of employment, versus knowledge creation and creative industries, thanks to engendered spillovers in terms of social relations and cultural effects).
ACKNOWLEDGEMENTS
The author thanks professors G. Croce, M. Raitano and M. Tancioni for helpful comments and suggestions. The author also gratefully acknowledges the precious support provided by Professor S. Protsiv in the use of the European Cluster Observatory database. All remaining errors are the author’s alone.
DISCLOSURE STATEMENT
No potential conflict of interest was reported by the author.
ORCID
Francesca Parente http://orcid.org/0000-0002-1945-7794
Notes
1 A specification is due: while for elements such as trust there is wide consensus on the inverse relation with inequality, for those about social networks’ endowments this is more vague and ambiguous – strictly depending on the kind of considered relations.
2 According to the 2010 NUTS classification, and excluding the French, Spanish and Portuguese overseas departments. This level ensures: wider and more homogeneous availability of HD variables for countries of interest; comparability with regional performance and innovation indicators not available yet at a lower territorial specification. The restriction is to the EU-15: a more homogeneous statistical coverage at a wider time span for considered variables, especially the industrial clusters.
3 The concept of proximity can be intended not just in terms of geographical vicinity, but also of social and institutional ones, and it entails a variety of complementary elements. For an in-depth elaboration on this, see Boschma (Citation2005).
4 For a review on different typologies of local systems of production, see Markusen (Citation1996), Iammarino and McCann (Citation2006), and Celata and Rossi (Citation2009).
5 US Cluster Mapping in the USA, and Cluster Mapping Tool in the EU.
6 Based on the NACE Rev. 2 classification system, it combines industries from different production sections into groups that can therefore include both manufacturing and service industries related to a specific specialization. For a detailed definition of the considered categories, and a list of which of four-digit industries they are made up of, see the Observatory website: http://www.clusterobservatory.eu/index.html.
7 The number of components to be extracted was decided by combining the eigenvalue criterion with the scree test (for a review of the available criteria for the number of components to be used to represent the underlying common factor in principal component analysis (PCA), see Di Franco & Marradi, Citation2013).
8 Vectors of component loadings are obtained by multiplying each element of the eigenvector (i.e., the weights to be attributed to the variables in the linear combination that produces the component) by the root of the related eigenvalue (Di Franco & Marradi, Citation2013). The component scores are standardized values obtained through linear combination of all the variables included in the PCA and represent the best possible synthesis of the data (Di Franco, Citation2014). The first component seemed to relate to the degree of diversification of regional business structures: its correlation with the count of industries in which regions have LQs > 2 was 60%. The second component pertained more to the business environment features, and content of innovation. It showed a strong correlation with both the regional innovation scoreboard (55%) and the number of patents (43%, when the correlation between these two is 50%).
9 The Alkire and Foster (Citation2010) adaptation of the Foster, Lopez-Calva, and Szekely (Citation2005) method. That is why this index is somewhere also referred to as ‘FLS IHDI’.
10 Also said, Jacobs externalities from the studies developed by the American urbanist Jane Jacobs (Jacobs, Citation1969), these are related to the proximity of firms from different industries, and the knowledge spillovers promoting innovation and growth, which their variety facilitates. They differ from the classic Marshall–Arrow–Romer (MAR) externalities because these focus on the proximity of firms from common industries. Other relevant speculations in this regard have been produced, especially by Porter (Citation1990) and, more recently, by Boschma (i.e., the related variety; Frenken, van Oort, Verburg, & Boschma, Citation2004). For a review, see Glaeser, Kallal, Scheinkman, and Shleifer (Citation1992).
11 Another way of saying this is that the slopes of the regression lines between inequality and trust are different for the different categories of production clusters’ structure. ϕ indicates how different those slopes are.
12 The only ESS wave reporting such detail, distinguishing 10 sorts of voluntary organizations, is that from 2002.
REFERENCES
- Alesina, A., & La Ferrara, E. (2002). Who trusts others? Journal of Public Economics, 85, 207–234. doi: 10.1016/S0047-2727(01)00084-6
- Alkire, S., & Foster, J. (2010). Designing the inequality-adjusted human development index (IHDI) (2010 HDR Background Paper No. 10).
- Aranguren, M. J., Franco, S., Ketels, C., Murciego, A., Navarro, M., & Wilson, J. R. (2010). Benchmarking regional competitiveness in the European cluster observatory. Europe Innova. Methodological Background Paper 1.
- Aranguren, M. J., & Wilson, J. R. (2013). What can experience with clusters teach us about fostering regional Smart Specialisation. Ekonomiaz N. 83, 2. cuatrimestre, 2013.
- Atkinson, A. B. (1983). The economics of inequality. Oxford: Oxford University Press.
- Atkinson, A. B. (2007). The distribution of earnings in OECD countries. International Labour Review, 146(1–2), 41–60. doi: 10.1111/j.1564-913X.2007.00004.x
- Atkinson, A. B. (2013a). Reducing income inequality in Europe. Journal of European Labor Studies, 2, 1–12. doi: 10.1186/2193-9012-2-12
- Atkinson, A. B. (2013b). Where is inequality headed? Arrow Lecture, Stanford University.
- Baker, W. E. (1990). Market networks and corporate behavior. American Journal of Sociology, 96, 589–625. doi: 10.1086/229573
- Baltagi, B. H. (2008). Econometric analysis of panel data. Chichester: Wiley.
- Banfield, E. C. (1958). The moral reasons of a backward society. Glencoe: Free Press. Research Center in Economic Development and Cultural Change, The University of Chicago.
- Barca, F. (2009). An agenda for a reformed Cohesion Policy. A place-based approach to meeting European Union challenges and expectations.
- Barone, G., de Blasio, G., & Sestito, P. (2014, November 17). Capitale sociale, economia, politica economica. Workshops and Conferences. Rome: Banca d’Italia.
- Barone, G., & Mocetti, S. (2016). Inequality and trust: New evidence from panel data. Bank of Italy Research division.
- Baum, C. F. (2006). An introduction to econometrics using Stata. College Station, TX: Stata Press.
- Becattini, G. (1990). The Marshallian district as a socio-economic notion. In F. Pyke, G. Becattini, & W. Sengenberger (Eds.), Industrial districts and intra-firm collaboration in Italy. Geneva: IILS.
- Becattini, G. (2000). Dal distretto industriale allo sviluppo locale. Turin: Bollati Boringhieri.
- Becattini, G., Bellandi, M., & De Propris, L. (2009). A handbook of industrial districts. Cheltenham: Elgar.
- Beugelsdijk, S., & Smulders, S. (2009). Bonding and bridging social capital and economic growth ( CentER Discussion Paper; Vol. 2009-27). Tilburg: Tilburg University.
- Beugelsdijk, S., & van Schaik, T. (2001). Social capital and regional economic growth ( CentER Discussion Paper 2001-102). Tilburg: TilburgTilburg University.
- Beugelsdijk, S., & van Schaik, T. (2005). Social capital and growth in European regions: An empirical test. European Journal of Political Economy, 21, 301–324. doi: 10.1016/j.ejpoleco.2004.07.004
- Boschma, R. (2005). Proximity and innovation: A critical assessment. Regional Studies, 39(1), 61–74. doi: 10.1080/0034340052000320887
- Boschma, R., Cortinovis, N., Xiao, J., & van Oort, F. (2016). Quality of government and social capital as drivers of regional diversification in Europe, No 1610. Utrecht: PEEG Utrecht University.
- Boschma, R., & Giannelle, C. (2014). Regional branching and Smart Specialisation policy, No JRC88242, JRC – Technical Reports, S3 Policy Brief Series 6/2014. Luxembourg: Publications Office of the European Union.
- Bourdieu, P. (1986). The forms of capital. In J. G. Richardson (Ed.), Handbook of theory and research for the sociology of education (pp. 241–258). New York, NY: Greenwood.
- Burt, R. S. (1992). Structural holes. The social structure of completion. Cambridge, MA: Harvard University Press.
- Celata, F. (2009). Spazi di produzione. Una prospettiva relazionale. Turin: G. Giappichelli.
- Celata, F., & Rossi, U. (2009). Industrial district. In R. Kitchin & N. Thrift (Eds.), International encyclopaedia of human geography (pp. 389–395). London: Elsevier.
- Charron, N., Dijkstra, L., & Lapuente, V. (2014). Regional governance matters: Quality of government within European Union member states. Regional Studies, 48(1), 68–90. doi: 10.1080/00343404.2013.770141
- Coleman, J. (1988). Social capital in the creation of human capital. American Journal of Sociology, 94, S95–S120. doi: 10.1086/228943
- Dasgupta, P., & Serageldin, I. (2000). Social capital: A multifaceted perspective. Washington, DC: World Bank.
- De Blasio, G., & Nuzzo, G. (2012). Capitale sociale e disuguaglianza in Italia (QEF No. 116). Roma: Banca d’Italia.
- De Blasio, G., Scalise, D., & Sestito, P. (2014). Universalism vs. Particularism: A round trip from sociology to economics. SSRN Electronic Journal, 212. doi:10.2139/ssrn.2419937
- d’Hombres, B., Elia, L., & Weber, A. (2013). Multivariate analysis of the effect of income inequality on health, social capital, and happiness ( Report EUR 26488 EN). JRC Scientific and Policy Reports.
- Di Franco, G. (2014). Il poliedro coesione sociale. Analisi teorica ed empirica di un concetto sociologico. Milano: Franco Angeli.
- Di Franco, G., & Marradi, A. (2013). Factorial analysis and principal component analysis. Bologna: Il Mulino.
- European Commission. (2014). Sixth report on economic, social and territorial cohesion. Luxembourg: Publications Office of the European Union.
- European Social Survey (ESS). (2013). Retrieved from www.europeansocialsurvey.org
- European Values Study (EVS). (2017). Retrieved from www.europeanvaluesstudy.eu
- Flap, H. D. (1991). Social capital in the reproduction of inequality, a review. Comparative Sociology of Family, Health and Education, 20, 6179–6202.
- Florida, R. (2005). Cities and the creative class. Oxford: Routledge.
- Foray, D., David, P. A., & Hall, B. H. (2011). Smart Specialisation: From academic idea to political instrument, the surprising career of a concept and the difficulties involved in its implementation (Working Paper 2011-001). Lausanne: Management of Technology and Entrepreneurship Institute.
- Foster, J. E., Lopez-Calva, L., & Szekely, M. (2005). Measuring the distribution of human development: Methodology and an application to Mexico. Journal of Human Development, 6, 5–25. doi: 10.1080/1464988052000342220
- Frenken, K., van Oort, F. G., Verburg, T., & Boschma, R. A. (2004). Variety and regional economic growth in the Netherlands. Papers in Evolutionary Economic Geography (PEEG) 0502, Utrecht University, Department of Human Geography and Spatial Planning, Group Economic Geography, revised Dec 2004.
- Fukuyama, F. (1995). Trust: The social virtues and the creation of prosperity. New York, NY: Free Press.
- Ganong, P., & Shoag, D. (2017). Why has regional income convergence in the U.S. declined? Journal of Urban Economics [Internet], 102, 76–90. doi: 10.1016/j.jue.2017.07.002
- Glaeser, E., Laibson, D. I., & Sacerdote, B. (2002). An economic approach to social capital. Economic Journal, 112(483), F437–F458. doi: 10.1111/1468-0297.00078
- Glaeser, E. L., Kallal, H. D., Scheinkman, J., & Shleifer, A. (1992). Growth in cities. Journal of Political Economy, 100(6), 1126–1152. doi: 10.1086/261856
- Glaeser, E. L., Resseger, M. G., & Tobio, K. (2008). Urban inequality (NBER Working Paper No. 14419).
- Gordon, I., & McCann, P. (2000). Industrial clusters: Complexes, agglomeration and/or social networks? Urban Studies, 37(3), 513–532. doi: 10.1080/0042098002096
- Granovetter, M. (1974). Getting a job: A study of contacts and careers. Cambridge, MA: Harvard University Press.
- Greene, W. H. (2008). Econometric analysis (6th ed.). Upper Saddle River, NJ: Prentice-Hall.
- Helliwell, J., & Putnam, R. (1995). Economic growth and social capital in Italy. Eastern Economic Journal, 21, 295–307.
- Hollanders, H., Es-Sadki, N., Buligescu, B., Rivera Leon, L., Griniece, E., & Roman, L. (2014). Regional innovation scoreboard. Belgium: Publications Office of the European Union.
- Iacofano, D., & Goltsman, S. (2007). The inclusive city: Design solution for buildings, neighbourhoods, and urban spaces. Berkeley, CA: MIG Communications.
- Iammarino, S., & McCann, P. (2006). The structure and evolution of industrial clusters: Transactions, technology and knowledge spillovers. Research Policy, 35(7), 1018–1036. doi: 10.1016/j.respol.2006.05.004
- Jacobs, J. (1969). The economy of cities. Random House, 201 East 50th Street, New York 10022.
- Ketels, C., & Protsiv, S. (2013). Clusters and the new growth path for Europe (Working Paper No. 14). Retrieved from WWWforEurope
- Ketels, C., Sölvell, Ö., & Lindqvst, G. (2008). Industrial specialization and regional clusters in the ten new EU member states. Competitiveness Review, 18(1/2), 104–130. doi:10.1108/105954208180874637 doi: 10.1108/10595420810874637
- Knack, S., & Keefer, P. (1997). Does social capital have an economic payoff? A cross-country investigation. Quarterly Journal of Economics, 112(4), 1251–1288. doi: 10.1162/003355300555475
- Kohler, U., & Kreuter, F. (2009). Data analysis using Stata (2nd ed.). College Station, TX: Stata Press.
- Kovacevic. (2010). Measurement of inequality in human development – A review (Human Development Research Paper 2010/35).
- Krugman, P. (1990). Cities in space: Three simple models (NBER Working Paper, 3607). National Bureau of Economic Research.
- Krugman, P. (1991). Geography and trade. Cambridge, MA: MIT Press.
- Lefebvre, H. (1991). The production of space. Cambridge, MA: Basil Blackwell.
- Markusen, A. (1996). Sticky places in slippery space: A typology of industrial districts. Economic Geography, 72(3), 293–313. doi: 10.2307/144402
- Marshall, A. (1920). Principles of economics (8th ed.). London: MacMillan (first edition 1890).
- Narayan, D. (2002). Bonds and bridges: Social capital and poverty. In S. Ramaswamy (Ed.), Social capital and economic development: Well-being in developing countries (pp. 58–83). Cheltenham: Edward Elgar.
- Parente, F. (2017). Productive structure and spatial inequalities: The role of innovative clusters. 13th IPGRC 2017 (pp. 990–1002). Manchester: University of Salford. Retrieved from http://usir.salford.ac.uk/43913/
- Parente, F. (2018). A multidimensional analysis of the EU regional inequalities. Social Indicators Research. doi: 10.1007/s11205-018-2000-6
- Park, R. E., Burgess, E. W., & McKenzie, R. D. (1925). The city. Chicago, IL: University of Chicago Press.
- Pastor, M., Sanchez-Lopez, A., & Ito, J. (2015). Linking innovation with inclusion. Demography, equity, and the future of San Diego. Los Angeles, CA: USC PERE.
- Porter, E. M. (1990). The competitive advantages of nations. London: Macmillan.
- Porter, E. M. (1998). Clusters and the new economics of competition. Harvard Business Review, 76, 77–90.
- Porter, E. M. (2003a). The economic performance of regions. Regional Studies, 37(6/7), 549–578. doi: 10.1080/0034340032000108688
- Porter, E. M. (2003b). Location, clusters, and company strategy. In G. Clark, M. Feldman, & M. Gertler (Eds.), Oxford handbook of economic geography. Oxford: Oxford University Press. Retrieved from https://www.hbs.edu/faculty/Pages/item.aspx?num=5432
- Porter, E. M., Stern, S., & Artavia Lorea, R. (2013). The social progress index. Washington, DC: Social Progress Imperative.
- Portes, A. (1998). Social capital: Its origins and applications in modern sociology. Annual Review of Sociology, 24, 1–24. doi: 10.1146/annurev.soc.24.1.1
- Putnam, R. D. (1993). Making democracy work: Civic traditions in modern Italy. Princeton, NJ: Princeton University Press.
- Putnam, R. D. (2000). Bowling alone: The collapse and revival of American community. New York, NY: Simon & Schuster.
- Rodríguez-Pose, A. (1998). Social conditions and economic performance: The bond between social structure and regional growth in Western Europe. International Journal of Urban and Regional Research, 22, 443–459. doi: 10.1111/1468-2427.00151
- Rodríguez-Pose, A., & Crescenzi, R. (2008). Research and development, spillovers, innovation systems, and the genesis of regional growth in Europe. Regional Studies, 42(1), 51–67. doi: 10.1080/00343400701654186
- Salvareda, W., Nolan, B., & Smeeding, T. M. (2011). The oxford handbook of economic inequality. Oxford: Oxford University Press.
- Sen, A. K. (1987). The standard of living. Oxford: Oxford University Press.
- Sen, A. K. (2009). The idea of justice. Cambridge, MA: Belknap/Harvard University Press.
- Stock, J. H., & Watson, M. W. (2007). Introduction to econometrics (2nd ed.). Boston, MA: Pearson Addison Wesley.
- UNDP. (2016). Human development report 2016. Human development for everyone. Retrieved from http://www.undp.org/content/undp/en/home/librarypage/hdr/human_developmentreport2011.html
- UNDP. (2018). Human development report ( Technical Notes). Oxford: Oxford University Press.
- van Schaik, T. (2002). Social capital in the European values studies surveys. Tilburg: Tilburg University.
- Vergati, S. (2000). Affari di famiglia. Il neofamilismo: reti, valori, stili di vita. Bonanno: Acireale-Roma.
- Vergati, S. (2014). Capitale sociale, coesione sociale e integrazione sociale. In Il poliedro coesione sociale. Analisi teorica ed empirica di un concetto sociologico (pp. 89–118). Milan: Franco Angeli.
- Weighting ESS Data. (2014). Retrieved from https://www.europeansocialsurvey.org/docs/methodology/ESS_weighting_data_1.pdf
- Whiteley, P. F. (2000). Economic growth and social capital. Political Studies, 48, 443–466. doi: 10.1111/1467-9248.00269
- Wooldridge, J. M. (2002). Econometric analysis of cross section and panel data. Cambridge, MA: The MIT Press.
- Zak, P. J., & Knack, S. (2001). Trust and growth. Economic Journal, 111, 295–321. doi: 10.1111/1468-0297.00609
APPENDIX A
European Social Survey (ESS) questions (§2)
Selected questions from the survey were the following:
Literal Question A3: Using this card, generally speaking, would you say that most people can be trusted, or that you can’t be too careful in dealing with people?
Literal Question A4: Using this card, do you think that most people would try to take advantage of you if they got the chance, or would they try to be fair?
Literal Question A5: Would you say that most of the time people try to be helpful or that they are mostly looking out for themselves?
Literal Question B11–B17: There are different ways of trying to improve things in [country] or help prevent things from going wrong. During the last 12 months, have you done any of the following? Have you …
worked in political party or action group last 12 months?
worked in another organization or association last 12 months?
The answer to B11–B17 gave the respondent the chance to confirm, deny or refuse the question.
ESS survey and related data treatment (§2)
The average country sample size is approximately 1900 (being 1500 for the EVS instead). In each country, it is representative of resident citizens of 18 years and older living within private households, irrespective of nationality, citizenship or language. Many previous cross-section studies drew on the European Values Study (EVS) (e.g., Boschma et al., Citation2016; d’Hombres et al., Citation2013; van Schaik, Citation2002). The ESS was here preferred because of at least two reasons. It ensures more homogeneous territorial coverage on a closer time basis to match with other selected variables (the only EVS wave providing a regional coverage wider than ESS ones is that from 2008). The construction of related questions in the survey allows one to extract numeric instead of categorical variables. Where the ESS answer is a value ranging from 0 to 10 (whose the highest means that people can be trusted), the same question in the EVS (Citation2017) has a response of yes/no nature.
Among used variables, these are the only microdata from primary survey sources. In order to make them comparable with the rest of deployed regional database, some calculations were necessary. Trust items were in the shape of cardinal variables and were transformed into continuous ones by calculating the regional median of responses separately per each item per year. The number of people whose answer was equal to or higher than the identified threshold was divided by the total number of respondent per region. Obtained percentages for each of the three items were aggregated by means of a simple arithmetic mean. This allowed one to have unique averaged values of reported trust, fairness and helpfulness per each region.
Social relations’ items were in the form of a yes/no answer. The number of people answering yes to the questions on voluntary unpaid work was divided by the total number of respondents per region. The obtained percentages were aggregated by means of a simple arithmetic mean.
Missing observations and refusals in the answers were excluded. A post-stratification weight including design weight has been applied (Weighting ESS Data, Citation2014). Answers presented a total of 5% of missing data between ESS1 and ESS7. The UK and Germany were always available at the NUTS-1 level only. Therefore, NUTS-2 regions were assumed to be equal to their overall NUTS-1 reference. Where NUTS-2 where available for some years only, such as the case of France and Belgium, their percentage incidence on the above NUTS-1 was used to estimate NUTS-2 from available NUTS-1 in the missing years.
The first wave is ESS1 (2002), the last one ESS7 (2014), with a regular time delta of two years. The applied two-year lag in the model was: ESS1 for 2000–04, ESS2 for 2005–06, ESS3 for 2007–08 and so on. The analysis was also run with contemporary or following years matching, and the results did not differ significantly. The reason was considered as the nature of the variable itself, which needs a considerable period to produce any significant variation in values.
Location quotients and cluster strength (§2)
In order to get a sound understanding of knowledge- or capital-intensive cluster categories, the use of information on sector wage, productivity or added value would be preferable. Unfortunately, these statistics are still not available at length for the EU, so the categorization currently available on the European Cluster Observatory (ECO) is obtained by means of employment data. Despite this, this has to be borne in mind, as it can even create a certain bias towards employment-intensive clusters, the measure for specialization obtained by LQs is unaffected by differences of employment intensity across cluster categories. LQs are calculated by:The obtained results were interpreted as follows:
LQ = 0: the sector is not present in the considered region.
0 < LQ ≤ 1: the region, compared with the country, is not specialized in the sector.
LQ = 1: the sector is present as in the same amount in the region as in the country.
1 < LQ ≤ 2: the region, compared with the country, is specialized in the sector.
2 < LQ ≤ 10: the region, compared with the country, is highly specialized in the sector.
Results of endogeneity tests (§2) ()
Table A1. Truncated regression residuals regressed on predictors.
Results of graphic analyses of ordinary least squares (OLS) residuals (§2)
Comparing fixed and random effects (§2)
If they are both consistent, their point estimates should not differ greatly. If one of the estimators is inconsistent, its point estimates are likely to differ widely from those of a consistent one. The FE estimator is always consistent, but inefficient under the null hypothesis that . RE is both consistent and relatively efficient under that null hypothesis, but inconsistent under the alternative. The null hypothesis for the Hausman test is that RE is consistent and should be preferred. If we reject that null, RE is inappropriate and FE should be used instead ().