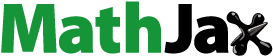
ABSTRACT
In this paper we study the impact of firm relocations on commuting distance and the probability of married couples and cohabiting couples with children separating. We use Swedish register data for the period 2010–16 and select employees of relocating firms with one workplace and more than 10 employees. Focusing on this sample allows us to use plausibly exogenous variation in the commuting distance arising from the relocation. We extend the literature on the effect of commuting on relationship stability by reducing the possibility for unobserved time-variant factors to bias our estimates. While previous literature has focused on the difference between short- and long-distance commuting, we focus on changes in the commuting distance that are externally induced by firm management. We find a small but statistically significant negative effect of increased firm relocation distance on family stability. A 10 km change in commuting distance leads to a 0.09 percentage point higher probability of separation if the commuter remains with the firm for the next five years.
1. INTRODUCTION
Commuting times of workers in Europe have been slowly but steadily increasing in the last decade.Footnote1 Policymakers and firms sometimes weigh the costs and benefits of expanding labour markets and increasing average commuting distances. The cost–benefit analyses of commuting often consider the economic and environmental effects of commuting. Fewer studies consider the social impacts of commuting. In particular, commuting time may influence the quality of partnerships. Time spent travelling could decrease time spent with family members and contributing to household chores, which may increase stress. Ultimately, the commuting situation could become unbearable and lead to separation. However, wage compensation and increased employability may also result from a longer commute and thus the total impact of commuting on relationship stability is an empirical question. Currently, evidence about the impact of commuting time on relationship stability is scarce.
Commuting distance is a job characteristic usually known to the job seeker when choosing to accept the job. We can understand commuting distance as a joint equilibrium outcome on a job market and a housing market. Therefore, we are not usually able to model commuting distance as randomly allocated. Commuting distance is also influenced by the employment structure of couples, which is indicated by the fact that dual-earner households are less likely to migrate (e.g. Jarvis, Citation1999a, Citation1999b, Citation1999c; Green, Citation1997). Green et al. (Citation1999) find that long-distance commuters are the ones that enjoy the benefits of commuting the most, whereas the costs of commuting are usually borne by the partner of the commuter.
If a firm moves to a new location, this relocation might not be expected by an employee. The particular circumstances of any employee should not influence the relocation decision in large firms. A change in commuting distance could be considered a random shock to both the commuting distance and job quality.
In this paper we focus on employees of single-site firms with more than 10 employees in 2010 or 2011. First, we examine the properties of relocating firms and check that they are similar to non-relocating firms in baseline characteristics. We then estimate the impact of relocation-induced commuting distance changes on family stability outcomes. Our main results point to a small but significant effect of an increase in the commuting distance on the probability of separation.Footnote2
Subsequently, we consider other sources of variation in the commuting distance: job change and residential move. We find that change in commuting distance related to job change is weakly and statistically non-significantly associated with separation whether the original firm relocated or remained stationary. Residence moves during firm relocation do not have a significant association with separation. Residence moves away from a stationary firm were associated with significantly lower separation probability. Moving while working in a stationary firm seems to be the most endogenous choice, as a decision to invest in a common good of a new house can be reasonably expected to depend on partnership expectations.
2. LITERATURE REVIEW
This study is related to several strands of literature. We follow the family stability literature in uncovering how social and economic factors influence separation and divorce. Theoretical works provide frameworks for empirical studies, including observational studies about the association of long-distance commuting and family stability. Next, we build on the firm relocation literature methodologically by examining the impact of relocations on socio-economic outcomes. Further, it is useful to compare our results with firm closure studies that have also considered family stability an outcome.
2.1. Theories of marital stability
Kley (Citation2015) identifies several theories of marital stability, which she uses to support her empirical model. In particular, there is social exchange theory (Kelley & Thibaut, Citation1978) and microeconomic theory of divorce (Becker et al., Citation1977). These theories are not mutually exclusive and often suggest similar predictions.
According to social exchange theory, marital stability is influenced by unfolding social exchange processes within and outside of the couple. This theory was applied early by Levinger (Citation1965), who recognizes attractions, barriers to leaving and the presence of attractive alternatives to the relationship.
In the context of our study, long-distance commuting potentially changes the relationship value for one or both partners by reducing the time the couple spends together and by changing bargaining power distribution within the couple. Long-distance commuting could influence attractive alternatives to the partnership both ways. On the one hand, less time to socialize outside of family and work may decrease the perceived alternatives to the relationship. On the other hand, as Kley (Citation2015) mentions, the commuter may likely have friends at work that are not shared with the less mobile partner and can increase alternatives to the relationship.
According to the microeconomic theory of divorce, the value of staying in a partnership is central to family stability. The partnership value depends on partner match, division of labour within the household, and investment in couple-specific capital. The partner match is related to the labour division within the couple. While Becker et al. (Citation1977) argued that the negative correlation of wage-earning powers should increase couple stability, newer empirical studies contest this theory. For example, Kley (Citation2015) finds that while labour participation of women in Western Germany is associated with the probability of separation, it is not significantly associated in Eastern Germany. The partner match is improved by homogeneity in complementary factors such as age and education level. The couple-specific capital includes shared homeownership and children. These assets may stabilize relationships because they lose value at separation. However, the value of couple-specific capital may be reduced during long-distance commuting as the more mobile partner has less time to use it, and the less mobile partner may need to do more chores. Kley finds that women, in particular, may be affected by long commutes as they are responsible for the bulk of household chores.
2.2. Association of long-distance commuting with family stability
This paper is also related to the literature about the effects of long-distance commuting. In particular, several recent studies analyse the association of long-distance commuting with family stability (Kley, Citation2015; Kley & Feldhaus, Citation2017; Sandow, Citation2014). Research has also been conducted on perceptions of partnership quality (Viry et al. (Citation2010)).
Sandow (Citation2014) uses the Swedish National Register and event history analysis with discrete-time logistic regression to examine whether long-distance commuting predicts couple separation. The study finds that the association differs according to gender and the duration of long-distance commuting. Couples who commute longer than five years seem to either selectively survive or to have become accustomed to the commuting lifestyle. The register data did not include either travel time or travel mode, and it was not possible to control for specific motivations for long-distance commuting. In this study, we estimate commuting time to see if the results from commuting distance generalize for commuting time. Sandow did not provide a causal interpretation of the data as the commuting behaviour may be endogenous to individual time-changing factors associated with relationship stability. In this paper, we address this limitation by using firm relocations as a source of variation in commuting distance.
Kley and Feldhaus (Citation2017) and Kley (Citation2015) use German survey data. Kley and Feldhaus (Citation2017) study long-distance commuting using the nationally representative sample Panel Analysis of Intimate Relationships and Family Dynamics (pairfam) with 2500 couples and monthly data over three years. Pairfam data allows one to control for an annual measure of marital quality and commuting for up to 2 residences. Furthermore, pairfam includes the timing of breakups and changes of residence and employment in months.Footnote3 The authors find that long-distance commuting of women longer than 1 h predicts lower family stability except for women living in the suburbs. These associations persist even after controlling for breakup predictors frequently found in the literature, such as social position of the partners, barriers to leaving the partnership, partnership quality and labour division within the household. Kley (Citation2015) studies the effect of commuting time on relationships. She uses monthly data from a three-year-long study on 890 inhabitants of two comparable German cities and tests hypotheses based on social exchange and economic theories of marital stability. The author controls for theoretically important predictors of marital stability, including indicators of the partner match (age at start of marriage, homogamy in education levels, division of labour within the household, couple-specific capital and barriers to leaving the relationship). While these predictors are theoretically important, they are likely interrelated, which could make the interpretation of the findings difficult. The study finds higher and significant associations of long-distance commutes of women for Eastern Germany and lower association for women of Western Germany. The author explains this difference by different social acceptance of the paid work of mothers.Footnote4 In Western Germany, the paid work of women predicts the separation of couples, while long-distance commuting has a smaller and less significant association with family stability.
Using the British Household Panel Study dataset, Nisic and Kley (Citation2019) study long-distance commuting and define long distance as longer than 1 h. They also consider moves longer than 50 km. The authors first describe the social structure of mobile couples and then focus on satisfaction with their social life. They find that women are affected by mobility more than men. They find a negative association of long commuting and satisfaction with social life for women who move because of their partner’s career prospects.
Viry et al. (Citation2010) view daily long-distance commuting as one of the cases of mobility. Their survey data includes a reason for commuting and information about other kinds of mobility (weekend commuting, long-distance relationships). They find that the effect of commuting can be mediated by the life course, the process by which one becomes mobile, social status, and national context (such as family policy regime, residential amenities such as daycare and after school programs, and quality of transportation systems).
For a comparison of main studies, see .
Table 1. Commuting longer than 30 km and family stability.
2.3. Firm relocations
This study is methodically inspired by the literature about firm relocations. The closest paper to our study is Mulalic et al. (Citation2014), who investigate wage compensation of workers at relocating firms. They estimate the causal effect of firm relocation using data from the register of companies in Denmark. The study finds that workers are compensated for the increase in commuting distance in the long term, while the short-term wage compensation is small. The authors deal with endogeneity in commuting distance by including worker fixed effects (FE) and firm FE in the empirical model. They then estimate their models in terms of first differences and consider both the short and long terms.
2.4. Firm closures
When we consider the effect of single-site firm relocation on the social life of the firm’s employees, one obvious comparison is with firm closures. In both cases, a given job bundle ceases to exist. The difference is that in the case of firm relocations, a similar job bundle appears at a different spatial location. The employee of a relocating firm thus has, ceteris paribus, a better position in terms of employment opportunities. Therefore, firm closures should impact family stability more strongly than firm relocations.
Rege et al. (Citation2007) use data from Statistics Norway from 1992 to 2005. They estimate a linear probability model of marital dissolution of workers in firms with more than 10 employees. They control for characteristics of couples, including socio-economic variables for both the couple and each of the spouses individually, indicators of spousal dependency (children, age and education homogamy), and downsizing experience of the wife. The marriages of husbands originally employed in firms that closed between 1995 and 2000 were 11% more likely to end in divorce by 2003 than comparable marriages of husbands in stable firms. The authors also test whether working in a firm before closing increases the divorce rate and find that it does not.
Keldenich and Lücke (Citation2018) use the German Socioeconomic Panel (SOEP) from 1984 to 2015.Footnote5 They use involuntary job loss as a source of variation, including firm closures. For robustness checks, they look at firm closures: They estimate a discrete-time proportional-hazard model, specifically a complementary log–log model for censored data. They use 1526 job losses due to involuntary job loss and find a 70% larger risk of divorce in the following year if the husband has experienced an involuntary job loss. They compare the results using only firm closures and find no substantive difference in results.
Our study links the family stability literature to the methodology of firm relocation studies. This allows us to provide the first causal estimates of the effect of commuting distance on the probability of couple separation. We also extend the firm relocation literature methodologically by estimating the lower bound of relocation-induced changes in commuting time. Our commuting-time algorithm based on geographical coordinates appears to be the first such algorithm in the literature; it allows for new avenues of research, including that on firm relocation.
Based on the literature review, we formulate the following hypotheses:
Hypothesis 1: Increase in commuting distance due to firm relocation increases long-term and medium-term risk of separation.
Hypothesis 2: Increase in commuting time due to firm relocation increases long-term and medium-term risk of separation.
3. DATA
In this study we use linked Swedish individual and firm register data from Statistics Sweden, obtained via secured institutional access. The data includes comprehensive administrative information about firms, employees and their partners. Our firm data were limited to single-site firms, allowing us to unambiguously define firm relocation as the change in easting and northing coordinates of the workplace. These coordinates are available in the administrative data in a 100-m precision. Due to firm and workplace definitions of Statistics Sweden, the stable and relocating firms we follow keep at least half of their workforce every year.
We restrict the sample further to firms with 10 or more employees in the baseline year, as relocation decisions of large firms are plausibly unrelated to the situation of individual workers (similar to Mulalic et al. (Citation2014)). We restrict the baseline years to 2010 and 2011 and study the workers in firms that belong to both baseline samples three and five years after each baseline year. The period 2010–11 was chosen as a relatively stable period following the 2008/09 economic crises. We also chose a five-year period of follow-up so our data span until 2016.Footnote6 From these firms, 702 firms in the baseline year relocated 5–200 km the following year. We restrict the relocating firms to those that relocated less than 200 km, because given larger relocations, workers may be more selective in deciding whether or not to stay with the firm. Another 5760 firms relocated 5 km or less. These shorter relocations should not have a noticeable impact on commuting distance or commuting time and so they are not included in the main analysis.Footnote7 From the firms that relocated 5–200 km, we selected employees who were either married or cohabiting with common children in the baseline year. This leaves 3424 employees who met our criteria and remained with the relocating firms three years after relocation, and 2197 remained five years after relocation, with attrition of 36% of workers. For a comparison, 287,266 employees worked in the firms that relocated 0–5 km three years after the relocation, and 222,759 remained five years after relocation, with an attrition of 22% of workers.
We use the following firm level variables from Statistics Sweden: workplace coordinates, industry code based on the Swedish Standard Industrial Classification (SNI)Footnote8, municipality code, number of employees and sales. Individual variables: gender, residential coordinates and municipality, education level, single-digit occupation, family status, duration of partnership, number of children under the age of 6 in the household, and education level of the partner. Variables are motivated mostly by inclusion in the literature ().
Table 2. Overview of variables used.
We construct the relocation distance of the firm, commuting distances and distances of any residential moves in meters. The precision of distances is 100 m2. We construct categorical values for marriage or cohabiting with children, for separation, for occupation and education categories.
We also estimate minimal commuting time using ArcGIS and considering available roads, one-way restrictions, tunnels and bridges, including the speed limits.Footnote9 Our model uses the following inputs: first, a table with firm coordinates in each year. We include only firms that relocate more 5–200 km in this table. Second, a table with employee residence coordinates and firm ID for each year. In this table, we include only the employees working in the relocating company in the baseline. The model matches firms and employees by year and firm ID, then calculates an optimization problem, minimizing the estimated commuting time. The output includes the estimated time of the commute and road distance of the calculated route. The correlation of estimated commuting time and road distance is 0.96 and the correlation of road distance with Euclidean distance is 0.97.
Our estimation of commuting time uses an assumption that everyone commutes by car. The Swedish Transport Administration Annual Report for 2017 (Trafikverket, Citation2018) gives the following statistics: ‘On a regular day, nearly 1 million people take a bus, almost as many people choose to cycle, 4.5 million take a car and 370,000 take a train. … More than 21,600 people take a domestic flight per day’ (p. 6). The vast majority of travel is by car or bus travel, which use public roads. The number of flights is negligible and we assume that it is used mostly for commutes longer than 200 km, which we do not consider in this analysis. Rail transport may introduce some measurement error, but it is limited by the relatively low use of rail in Sweden. Another possibly more serious cause of measurement error in our commuting time estimates is due to imprecise estimation of travel time. The estimate could be biased upwards due to low compliance with road speed limits, which is only about 40% (p. 18). It could also be biased downwards due to traffic congestion, which is documented and monitored in the metropolitan areas Stockholm, Gothenburg, and Malmö (p. 11).
We constructed education homogamy based on education levels of both partners in the couple (we used the seven levels used by Statistics Sweden). Couple education homogamy was rated as 1 if the education levels were the same.
3.1. Local context
In comparison with many other countries in Europe, Sweden is sparsely populated having a population density of 26 inhabitants/km2. The population density of the 10 largest urban areas spans between 3580 and 7724 inhabitants/km2. This reflects a settlement pattern dominated by a few larger cities (Statistics Sweden, Citation2022c).
Based on commuting patterns there are currently 70 local labour market regions, but the long-term trend indicates a continued decrease since improvements in the transport infrastructure have gradually made it possible to commute over longer distances (e.g., Sveriges Kommuner och Landsting, Citation2008). However, commuting long distances is still rather rare. In 2020, the average work trip distance was circa 21 km, and nearly 60% had shorter than 15 km to work. Most workers having a commuting distance between 30 and 150 km live close to larger cities, and these flows are usually directed towards larger city regions and specifically to the three metropolitan regions (Trafikanalys, Citation2020). Despite the proportion of workers having a long commute is low, a register-based study by Andersson et al. (Citation2018) showed that commutes longer than 100 km increased by 42% between 1990 and 2009. Rural-to-urban commuting represents the largest share of the long-distance commuters. It should be noticed that the flow of long-distance commuters represents less than 3% of the workers living in rural areas. In comparison with other countries, interregional commuting amounts to circa 7% of the workforce, which is substantially less than in many other European countries such as Germany, the UK and France. However, interregional commuting in the United States is less frequent than in Sweden (Eliasson et al., Citation2007).
From a COVID-19 pandemic point of view it has been argued that working from home patterns may have seen a structural shift potentially triggering fundamental changes in commuting patterns. Travel time is considered to be important for the willingness to accept a daily commute. Before the pandemic, 45 min was found to be a generally acceptable threshold (e.g., Huang et al., Citation2018; Van Ham, Citation2001). Similar results have been reported for Sweden (Sandow & Westin, Citation2010). To what extent the post-pandemic development has changed people’s preferences for where to work–and thus their commuting behaviour–is mainly an uncharted area of research. However, in a recent report by Statistics Sweden (Citation2022b) the results do not show much indication of large structural changes taking place in terms of working from home patterns. In the beginning of 2021, during the pandemic, 30% of the employed aged 15–74 worked from home at least half of their working days. In May 2022, after the removal of the COVID-19 Act on temporary infection control measures, this proportion had gone down to 17%. These results show that the proportion of people working from home is on the decrease suggesting a slow process of going back to a pre-pandemic situation.
In Sweden, it is common that partners living together are not married. About one third of all couples living together are not married (Statistics Sweden, Citation2022a). Only focusing on divorce rates are therefore misleading when studying relationship stability. In this study, we therefore include all couples living together as married, as registered partners or cohabiting with common children. While Sweden has one of the highest divorce rates in Europe (EUROSTAT, Citation2022), the majority of couples separating are not married (Statistics Sweden, Citation2022a). Separations are more common among younger couples. In the age of 25, nearly one sixth of the couples living together separates during one year, compared with 8% of couples in their 30s and around 1% for 65-year-olds (Statistics Sweden, Citation2020). While it is more common that couples with no children separates, 3.7% of all couples living together with common children separated during 2020. This separation rate has remained stable since 2015 (Statistics Sweden, Citation2021).
4. DESCRIPTIVE STATISTICS
Here we present the descriptive statistics. There were 32,870 single-site firms with more than 10 employees in total. A total of 26,099 of these did not relocate at all, 6005 firms relocated more than 0 and less than 5 km, 766 firms relocated 5–200 km. Firms that did not relocate employed 416,415 workers, firms that relocated more than 0 and less than 5 km employed 142,783 workers and firms that relocated 5–200 km employed 10,019 workers.
In the regression, only firms without missing control variables were included. There were 702 such firms that relocated 5–200 km in 2011 or 2012, employing 3910 employees meeting our criteria,Footnote10 of which 3424 remained with the firm three years after the relocation and 2197 remained five years after the relocation. Firms do not seem to be selective in relocating more than 5 km. shows that, prior to relocation, the firms relocating further have higher mean sales but the difference is not statistically significant.
Table 3. Baseline firm sample comparison by relocating.
Firms relocating 5–200 km are more likely to be in a large (metropolitan) location at the baseline ().
Table 4. Initial firm location by relocation distance.
shows the distributions of firms and employees among industries. We can see that construction, transportation and storage and administrative and support service industries are more predominant within firms that relocate 5–200 km.
Table 5. Industry distribution of firms and employees.
There may be a selection of employees into the kind of firms that relocate. The t-test reveals a significant difference in several potentially important variables before relocation between employees in firms that relocate shorter and longer distances ().Footnote11 Comparing with , we see that the commuting distance increased more for the employees in substantially relocating firms.Footnote12 Still, the majority of workers have a relatively short commuting distance after the relocation (median = 12.8, mean = 21 km) and 81% of workers have shorter commutes than 30 km.
Table 6. Baseline sample comparison by relocating.
Table 7. Year after relocation sample comparison by relocating.
5. IDENTIFICATION STRATEGY
For identification of the causal effect of commuting distance, we use firm relocations. To study precisely defined relocations, we focus on firms larger than 10 employees with one workplace. We observe workplace relocation when a workplace changes geographical coordinates and at least half of workplace employees remain with the firm. This is due to the workplace definition by Statistics Sweden. While for some employees the commuting distance has decreased as a result of workplace relocation, for others the distance has increased. However, the average commuting distance grows with average relocation distance.
The identifying assumptions: The relocation needs to be uncorrelated to the error term of the separation regression. Further, the relocation should not affect separation behaviour if the commuting distance is held constant.
5.1. Empirical model
In common situations, separation and divorce may be endogenous to commuting distance. Therefore, in our main specification, we use only the quasi-experimental variance in the commuting distance coming from firm relocations that are more likely to be exogenous. Following Mulalic et al. (Citation2014) we use the first difference of a FE model:
(1)
(1) where
denotes the separation of individual
, considered as a change in partnership status,
denotes time-differencing operator,
is commuting distance,
includes time-variant control variables and
includes time-invariant control variables with a time-varying effect.
denotes a firm FE. In the analytical samples of this model, we include only those who remain with the firm and do not move residence in the corresponding three- and five-year periods.
In this setting, there is a possibility for unobserved variables at the individual level. Therefore, FE are needed. We use a linear probability model with FE. Another possibility would be multinomial logit with FE. We choose the linear probability model because the interpretation of coefficients is more straightforward. Odds ratios are incomparable without knowing the baseline probabilities, which the FE multinomial logit unfortunately cannot compute (Pforr, Citation2014).
We estimate commuting time as a function of work and residence coordinates using network analysis in ArcGIS, taking into account the road network, including speed limits, one-way roads and tunnels. This allows us to run an analogous regression with estimated commuting time as the independent variable of interest:
(2)
(2) In order to compare the coefficients of relocation-induced changes in commuting distance with other common sources of variation in commuting distance, we also estimate the following model:
(3)
(3) where
is a set of sources of variation, including relocation, job change, residential move and other.
is a dummy variable representing the source of variation in commuting distance of individual
.
5.2. Checking for ex ante family stability differences
The external validity of our estimates would be low if the kind of workers who leave a company after relocation is also the kind of a person more likely to separate from a partner. To check for this possibility we calculate a family stability ranking based on employees in non-relocating firms. First, we regress separation on control variables in a sample of workers from non-relocating firms. We then compute an out-of-sample prediction of separation for the workers in relocating companies in the baseline year. This prediction is our baseline family stability ranking. We then look at whether this ranking predicts remaining in relocating firms. We find that remaining in the relocating firms is not predicted by the stability ranking, with or without using the control variables ( and ).
Table 8. Remaining with the firm three and five years after relocation.
Table 9. Remaining with the firm three and five years after relocation.
For more details about the other sources of variation, see Appendix A in the online supplemental data. For example, we find non-significant results in probability of separation for both workers who leave a stationary firm, and for workers who leave a relocating firm.
6. MAIN RESULTS
shows the results of the main regressions. While three years after relocation there is a weak positive significant effect of commuting distance, five years after relocation there is a stronger positive significant effect of relocation-induced change in the commuting distance on the separation of workers who remain in substantially relocating firms for at least five years. This effect on separation probability is significant but relatively small. Using firm FE reduces the significance of the estimate. For a 10 km change in commuting distance, this translates to a 0.086 percentage point change in the probability of separation. This risk of separation is small in comparison with the average of 15% of couples in the sample separating over five years. The average of separations among the studied workers is similar to the share of separations found in Sandow’s (Citation2014) study analysing all couples (11%). For a 50 km change in the commuting distance, the effect would be 0.43 percentage points, which is a moderate effect on family stability. Although the effect is small, these results show that when commuting distances increases due to firm relocations, the probability of separation increases over time and with distance. An increased risk of separation with longer commuting distances is in line with results from a previous study of Swedish couples (Sandow, Citation2014).Footnote13
Table 10. Changes in separation probability three and five years after relocation.
The specification with estimated commuting time had unexpected results. shows effects that are 10 times smaller than the corresponding estimate for the commuting distance, and they are not statistically significant at even the 10% level. These results point to limitations of using ArcGIS for calculating commuting times without recourse to traffic data.
Table 11. Main analysis with commuting time.
6.1. Urban versus rural areas
We also control for relocations within and between large and medium/small municipalities (). The first dummy refers to direction of change of the firm location: Within small/medium municipalities, From large (to small/medium) or into large municipality. The second interacted dummy refers to change in residence (if any). Including these controls does not change the results substantively. Commuting changes within firms that stay in medium or small municipalities or move into large municipalities lead to even higher separation rates than firms that move out of large municipalities into smaller ones or firms that stay in large municipalities for those who stay five years with the relocating firm.
Table 12. Controlling for relocations within and between large and medium/small municipalities.
6.2. Heterogeneity across groups
shows heterogeneous effects across groups. Families with young children may be particularly strongly influenced by commuting distance as children require care that is typically unequally distributed if one of the partners has to commute long distances. We interact the regression with having any children and find a significant positive effect for workers with children five years after relocation.
Table 13. Heterogenous effects across groups.
Education homogamy is a much stronger predictor of separation than relocation distance as couples with same education level are less likely to separate. The probability of separation for couples that have the same education level is increasing with relocation distance. However, this increase is smaller than the effect of educational homogamy itself.
Controlling for a partner’s change of commuting distance makes the result of commuting distance non-significant even five years after relocation and smaller than in the main specification.
Controlling for a partner’s working status does not change the main results. Working partner is associated with larger likelihood of separation and working full-time has even stronger association.
Working in the same workplace as partner may change the impact of commuting distance. In our sample, 201 workers have partners in the same baseline workplace. Removing these workers does not change the main results substantively.
7. ROBUSTNESS
7.1. Different treatment specifications
To test the robustness of our results to the 5 km threshold for firm relocations, we replicated our main analysis using 1 and 10 km as alternative thresholds. Both show similar and significant results. 1–200 km relocations are more common, which brings a larger sample size but also concerns that these data include more small changes that should not have any impact on separation probability. 10–200 km relocations are less common but more likely to result in a substantive change in commuting distance or time. shows that the main result five years from relocation is robust to including firms relocating at least 1 and at least 10 km. The effect is growing with minimal relocation distance. For firms relocating at least 10 km, there is a large protective effect of an induced 30 km commute three years from relocation, but the commuting distance for these induced commuters has a negative effect on family stability.
Table 14. Is separation influenced by commuting induced by relocations longer than both 1 and 10 km?
7.2. Placebo group of firms moving 0–5 km
We tried to run the regression also for the control group of firms which moved only 0–5 km. Workers in these firms do not face large changes in transport costs so we expect no impact of commuting distance changes on separation rates. shows the results. As expected, the estimated effect is not statistically significant and 10–100 times smaller than the effect for the firms relocating 5–200 km.
Table 15. Placebo group: 0–5 km relocations.
7.3. Alternative specifications
We also replicated the applicable robustness checks from Mulalic et al. (Citation2014). We ran the main regression without the commuting distance. The coefficients of the control variables did not change in economically or statistically significant ways. We also considered changes in the set of control variables. Including profit as a control variable makes the main result non-significant. Profit is a rather noisy variable, which can be influenced by an accounting strategy, and therefore this variable is not used in our preferred specification. We ran a cubic specification and the only significant effect was the linear one five years after relocation, of similar size as the main regression. Rescaling the sales variable does not change the main results substantively. Regression using log of commuting time turns out weakly significant and with magnitude and sign corresponding to the regression with distance.
Possibly the main limitation of the study is the use of a linear probability model. We have also estimated logit specifications and there were positive and statistically significant results of similar magnitude as the main linear model. We chose the linear probability model for this study because the interpretation of coefficients is more straightforward.
7.4. Other sources of variation
As we can see in , when we contrast changes in commuting distance related to firm relocation versus other changes, the possibly more endogenous changes show the opposite correlation: people who change commuting distance for other reasons have, on average, more stable marital or cohabiting relationships. These ‘other’ reasons include moving residence, changing jobs, and combinations of firm relocation with moving or job change. We explore these sources in more detail in Appendix A in the online supplemental data.
Table 16. Analysis of relocation versus other sources of variation.
7.5. Removing top earners and part-time workers
Two groups of workers could potentially interfere with our results. First, part-time workers may be less affected by time constraints if they do not commute daily. In our data, we cannot identify part time workers precisely. Workers in the bottom income decile are more likely to be part-time workers. Second, the workers in the top income decile are probably among the most productive employees who could be more likely to influence firm relocation decision and break our identifying assumption. Removing both these groups should provide a sense of how much our estimates are sensitive to these concerns. In we see the results of the analysis with a restricted sample, removing the top and bottom income deciles. The effect for the restricted sample is quantitatively similar and more significant.
Table 17. Removing top and bottom income deciles.
8. CONCLUSIONS
This study analyses the effect of commuting distance and estimated commuting time on family stability using matched register data for firms and workers in Sweden. We mitigate endogeneity of commuting and family stability by focusing on changes in commuting distance that are due to firm relocations. We show that an increase in commuting distance due to firm relocations leads to a small and significant increase in the probability of separation five years later. The result is robust to including relocation-related changes in commuting distance of workers who leave the company a year after the relocation and to a battery of checks in the spirit of Mulalic et al. (Citation2014).
Focusing on commuting time as the channel through which commuting distance may influence family stability produced mixed results. Depending on the specification, the effect of commuting time may be consistent with the result of commuting distance or may be non-significant. Future research could potentially mitigate missing data problems by adding traffic data to time estimation model.
When comparing our results with observational studies on commuting distance, we find an effect in the same direction as the associations found in Sandow (Citation2014), Kley (Citation2015) and Kley and Feldhaus (Citation2017), but the effect is much smaller than the associations. This study implies that firm relocation induced changes in commuting distance have a measurable but small negative impact on family stability.
The estimated outputs can inform on best practices at firm and household level. Our results suggest that at firm level it may be beneficial to give extra support to workers whose commuting distance changes to longer commutes. This support may include psychological counselling, flexible working schedule, remote work days and similar measures. These may be particularly important for employees with small children. This support might also help with employee retention and improve their work–life balance and productivity.
At the worker household level our results suggest that a worker who values work–life balance and relationship satisfaction could consider looking for a new job if commuting distance increases in a problematic way. Short periods of long-distance commuting are not necessarily harmful to family stability so workers could still keep their relocated job while looking for a better job in terms of location nearer to residence.
Supplemental Material
Download PDF (216.4 KB)ACKNOWLEDGEMENTS
We are grateful for the comments received from the research seminar at the Department of Geography in Umea (Sweden) and the PhD Workshop in Microeconometrics in Bozen–Bolzano (Italy), 2019. Thanks to Erik Backström for help with the ASTRID database, and to Magnus Strömgren for his generous support of our efforts to estimate the commuting time with ArcGIS. We also thank Stepan Jurajda, referees from the CERGE-EI Working paper series and the anonymous journal referees for useful feedback, incorporated in this version of the paper. All remaining errors are the authors’ responsibility. This paper is based on a working paper by Hrehová et al. (Citation2021).
DISCLOSURE STATEMENT
No potential conflict of interest was reported by the authors.
Additional information
Funding
Notes
1 According to Numbeo, which provides traffic rankings since 2012 (https://www.numbeo.com/traffic/rankings_by_country.jsp), and calculations by the authors, among 27 countries included in the 2012 and 2018 indices, the population-weighted average of commuting time increased from 35.5 min one way in 2012 to 36.6 min in 2018. For further information about the methodology, see https://www.numbeo.
2 Sample size does not allow us to consider the potentially assymetrical impact of commuting distance.
3 For more information about pairfam, see Huinink et al. (Citation2011).
4 The social acceptance of female paid work is consistent with labour participation data: in 2005, 72% women in Eastern Germany participated in the labour market, in contrast to 65% of women in Western Germany (source: Federal Statistical Office Germany).
5 For more information on the dataset, see Wagner et al. (Citation2007).
6 We consider three years for a medium-term and five years for a long-term effect of induced commuting on relationship separation. We pool workers from both baseline years to increase the sample size.
7 We include them as a placebo test in the robustness section ().
8 The SNI classification is used to classify enterprises and workplaces according to the activity carried out and is based on the EU's recommended standard, commonly referred to as NACE (Statistics Sweden (Citation2007)). The third level, which groups production units into major economic activity groups, has been used to classify industries.
9 To move from estimating minimal commuting time to actual commuting time, it would be ideal to include information about traffic congestion. Unfortunately, we did not have these data and could not take the coordinate pairs out of the computer lab to Google Maps API.
10 An employee living with a partner in the same residence. Having a partner is defined as being married, having a registered partner or cohabiting with common children.
11 Employees in firms relocating longer distances are older. This suggests that more fragile younger families may have already selected out of the sample. Thus, our estimate of the effect of commuting distance could be a lower bound of the effect in the population.
12 We use substantial relocation as a shorthand for relocating 5–200 km.
13 Interestingly, when we interact commuting distance with a firm relocation-induced commute longer than 30 km, the threshold used in the literature, we find no effect of crossing a 30 km threshold on the effect of commuting distance.
REFERENCES
- Andersson, M., Lavesson, N., & Niedomysl, T. (2018). Rural to urban long-distance commuting in Sweden: Trends, characteristics and pathways. Journal of Rural Studies, 59, 67–77. https://doi.org/10.1016/j.jrurstud.2018.01.010
- Becker, G. S., Landes, E. M., & Michael, R. T. (1977). An economic analysis of marital instability. Journal of Political Economy, 85(6), 1141–1187. https://doi.org/10.1086/260631
- Eliasson, K, Westerlund, O, & Åström, J. (2007). Flyttning och pendling i sverige. Stockholm: Fritzes.
- EUROSTAT. (2022). Demographic indicator: Crude divorce rate. Accessed December 13, 2022. https://ec.europa.eu/eurostat/databrowser/view/tps00216/default/table?lang=en
- Green, A. E. (1997). A question of compromise? Case study evidence on the location and mobility strategies of dual career households. Regional Studies, 31(7), 641–657. https://doi.org/10.1080/00343409750130731
- Green, A. E., Hogarth, T., & Shackleton, R. E. (1999). Longer distance commuting as a substitute for migration in Britain: A review of trends, issues and implications. International Journal of Population Geography, 5(1), 49–67. https://doi.org/10.1002/(SICI)1099-1220(199901/02)5:1%3C49::AID-IJPG124%3E3.0.CO;2-O
- Hrehová, K., Sandow, E., & Lindgren, U. (2021). Firm Relocations, Commuting and Relationship Stability (Working Paper Series No. 694). CERGE-EI.
- Huang, J., Levinson, D., Wang, J., Zhou, J., & Wang, Z.-j. (2018). Tracking job and housing dynamics with smartcard data. Proceedings of the National Academy of Sciences, 115(50), 12710–12715. https://doi.org/10.1073/pnas.1815928115
- Huinink, J., Brüderl, J., Nauck, B., Walper, S., Castiglioni, L., & Feldhaus, M. (2011). Panel analysis of intimate relationships and family dynamics (pairfam): conceptual framework and design. ZfF – Zeitschrift für Familienforschung/Journal of Family Research, 23(1), 77–101. http://doi.org/10.20377/jfr-235
- Jarvis, H. (1999a). Housing mobility as a function of household structure: Towards a deeper explanation of housing-related disadvantage. Housing Studies, 14(4), 491–505. https://doi.org/10.1080/02673039982740. https://www.tandfonline.com/action/journalInformation?journalCode=chos20
- Jarvis, H. (1999b). Identifying the relative mobility prospects of a variety of household employment structures, 1981–1991. Environment and Planning A, 31(July), 1031–1046. https://doi.org/10.1068/A311031
- Jarvis, H. (1999c). The tangled webs we weave: Household strategies to co-ordinate home and work. Work, Employment Society, 13(2), 225–247. https://doi.org/10.1177/09500179922117926. http://wes.sagepub.com/cgi/alerts
- Keldenich, C., & Lücke, C. (2018). Unlucky at work, unlucky in love. http://journals.ub.uni-magdeburg.de/ubjournals/index.php/FEMM-WPS/article/view/123
- Kelley, H. H., & Thibaut, J. W. (1978). Interpersonal relations: A theory of interdependence. John Wiley & Sons.
- Kley, S. (2015). The impact of job-related mobility and migration intentions on union dissolution. In C. Aybek, J. Huinink, & R. Muttarak (Eds.), Spatial mobility, migration, and living arrangements (pp. 139–158). Cham: Springer.
- Kley, S. A., & Feldhaus, M. (2017). Effects of female commuting on partnership stability in suburban and other residential regions. Population, Space and Place, 24(2). https://doi.org/10.1002/psp.2093
- Levinger, G. (1965). Marital cohesiveness and dissolution: An integrative review. Journal of Marriage and the Family, 19–28. https://doi.org/10.2307/349801
- Mulalic, I., Van Ommeren, J. N., & Pilegaard, N. (2014). Wages and commuting: Quasi-natural experiments’ evidence from firms that relocate. The Economic Journal, 124(579 (September)), 1086–1105. https://doi.org/10.1111/ecoj.12074
- Nisic, N., & Kley, S. (2019). Gender-specific effects of commuting and relocation on a couple’s social life. Demographic Research, 40, 1047–1062. https://doi.org/10.4054/DemRes.2019.40.36
- Pforr, K. (2014). Femlogit – Implementation of the multinomial logit model with fixed effects. The Stata Journal: Promoting Communications on Statistics and Stata, 14(December), 847–862. https://doi.org/10.1177/1536867X1401400409
- Rege, M., Telle, K., & Votruba, M. (2007). Plant closure and marital dissolution (No. 514). Discussion Papers. http://hdl.handle.net/10419/192496
- Sandow, E. (2014). Til work do us part: The social fallacy of long-distance commuting. Urban Studies, 51(3), 526–543. https://doi.org/10.1177/0042098013498280
- Sandow, E., & Westin, K. (2010). Preferences for commuting in sparsely populated areas: The case of Sweden. Journal of Transport and Land Use, 2(3/4), 87–107. https://doi.org/10.5198/jtlu.v2i3.21
- Statistics Sweden. (2007). SNI 2007 Swedish standard industrial classification. MiS 2007:2. SCB: Örebro.
- Statistics Sweden. (2020). Utflugna barn leder inte till att fler separerar. Accessed December 21, 2022. https://www.scb.se/hitta-statistik/artiklar/2020/utflugna-barn-leder-inte-till-att-fler-separerar/
- Statistics Sweden. (2021). There were 66 000 children who witnessed a separation in 2020. Accessed December 21, 2022. https://www.scb.se/en/finding-statistics/statistics-by-subject-area/living-conditions/living-conditions/children-and-their-families/pong/statistical-news/children-and-their-families-2020/
- Statistics Sweden. (2022a). Family formation and separation in corona times. 2020 and 2021 compared to 2015–2019. 9. Accessed December 21, 2022. https://www.scb.se/publication/48238/
- Statistics Sweden. (2022b). Hemarbete under coronapandemin [Working from home during the Corona pandemic]. https://www.scb.se/hitta-statistik/statistik-efter-amne/arbetsmarknad/arbetskraftsundersokningar/arbetskraftsundersokningarna-aku/pong/publikationer/arbetskraftsundersokningarna-aku-2022–tema-hemarbete-under-coronapandemin/
- Statistics Sweden. (2022c). Localities and urban areas 2020. https://www.scb.se/publication/47139
- Sveriges Kommuner och Landsting. (2008). Pendlare utan gränser? En studie om pendling och regionförstoring. Stockholm: Sveriges kommuner och landsting (SKL)
- Trafikverket. (2018). The Swedish Transport Administration Annual Report 2017. Borlänge: Trafikverket
- Trafikanalys. (2020). Förvärvsarbetare och deras arbetsresor. PM, 2020:6. Stockholm: Trafikanalys
- Van Ham, M. (2001). Workplace mobility and occupational achievement. International Journal of Population Geography, 7(4), 295–306. https://doi.org/10.1002/ijpg.225
- Viry, G., Widmer, E. D., & Kaufmann, V. (2010). Does it matter for us that my partner or I commute? Spatial mobility for job reasons and the quality of conjugal relationships in France, Germany, and Switzerland. Zeitschrift für Familienforschung, 22(2), 149–170. http://dx.doi.org/10.20377/jfr-272
- Wagner, G. G., Frick, J. R., & Schupp, J. (2007). The German socio-economic panel study (SOEP) – Scope, evolution and enhancements. Schmollers Jahrbuch, 127(1), 139–169. https://papers.ssrn.com/sol3/papers.cfm?abstract%7B%5C_%7Did=1028709. https://doi.org/10.3790/schm.127.1.139