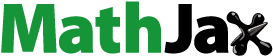
ABSTRACT
This paper challenges the traditional perspective of a territorial core–periphery pattern as an organizing principle of space by introducing a relational perspective through an empirical approach. We study spatial processes of knowledge creation among advanced producer services in Germany between 2009 and 2019. We use a unique longitudinal dataset to analyse if German regions have become more integrated into the intra-firm location networks nationally and globally, and how this translates into the processes of centralization and peripheralization. The current conceptualization of core–periphery in Germany is too static and does not reflect the economic development of the regions. We find that there is no trend of urban areas becoming more integrated and peripheral areas less integrated into these networks. Instead, our research finds a discrepancy between the federal territorial classification and the functional orientation of German regions. Regions classified as peripheral have not generally decreased in connectivity to other regions. In contrast, some have increased their connectivity and have become more central in relational terms. Overall, we identified peripheralization and centralization as a relational process with dynamic, overlapping and trans-scalar networks.
1. INTRODUCTION
The topic of this paper is the German urban system and its changes. We analyse the changes over time, using the example of intra-firm location networks of advanced producer services (APS). Our interest lies in the development of core–periphery patterns from a relational perspective, that is, the intensity of being connected among each other. We want to find out how the development of connectivity in Germany differs between urban and rural regions from 2009 to 2019. In addition, we investigate to what extent this development differs if we further distinguish between connectivity to locations outside and inside of Germany – and how the findings fit to the governmental territorial classification of urban, semi-urban and rural regions in Germany.
There are two different understandings of the concepts of core and periphery. The conventional understanding of core–periphery has a spatial or territorial dimension: locations in cities with easy accessibility (airports, etc.) are urban, whilst locations far away from these with less accessibility are peripheral. In contrast, a relational perspective takes a different understanding of core–periphery. It argues from a network perspective that places with many connections to other places are central, whilst places with few connections are peripheral. Thus, geographically peripheral locations can also become central. The Globalization and World Cities (GaWC) research group has developed the Interlocking Network Model to measure the connectivity between regions. We use this method as a starting point and extend it in various ways. In contrast to the GaWC considering only the few most connected German cities, we consider the whole country. Further, in contrast to static observations of one point in time, we conduct a temporal comparison between 2009 and 2019. Lastly, we mirror our findings against the territorial conceptualization of core and periphery introduced by the German Federal Office for Building and Regional Planning (BBSR). We contribute to the manageable quantity of research taking a relational perspective in spatial analyses, and provide evidence why this approach is useful for looking at the changing urban system.
The paper is structured as follows. Section 2 discusses the theoretical background, first on territorial core–periphery patterns and the spatial characteristics of APS. The focus then lies on relational core–periphery patterns created by locations of knowledge-intensive firms. Section 3 examines the German urban system and existing relational studies. Section 4 follows with an operationalization of APS, the federal delimitation of core and periphery in Germany, as well as the methods used. The results in section 5 are divided by (1) an analysis of how the overall connectivity development in Germany differs between urban and rural regions between 2009 and 2019; (2) a more detailed analysis differentiating between connectivity changes within Germany and outside of Germany; and (3) how these developments fit to the governmental territorial classification of urban, semi-urban and rural regions. The paper concludes with a discussion of the results obtained and thoughts on possible further considerations in analysing the complexities of the core–periphery patterns.
2. THEORETICAL BACKGROUND
Our conceptual basis draws strongly from Dicken and Malmberg (Citation2001). Those authors review the wide research field of the firm–territory nexus and note a wide range of systemic notions. The existing concepts differ in their definition of the terms firm and territory, as well as the way and extent in which they consider the spatial dimension. Broadly, there are two streams: an arena or territorial type of system which originates in geographical proximity and a relational type of system, sometimes also called functional (Dicken & Malmberg, Citation2001). Below, we briefly review both, but focus on the later from the perspective of core–periphery patterns.
2.1. Territorial core–periphery patterns
In geography and spatial planning, the concept of core and periphery is traditionally used in a topographical sense. The periphery is at the edge of a region and, hence, geographically remote from the core city (Copus, Citation2001). The spatial demarcation of peripheral regions is often based on attributional data such as population and employment (Copus, Citation2001). According to this concept of periphery, higher transportation and travel costs as well as weak agglomeration effects lead to increasing disparities between central and peripheral regions (Berman et al., Citation2020). In turn, this leads to high costs of service provisions, a poorly developed research and development (R&D) sector and low rates of innovation (Eder, Citation2018). Furthermore, periphery is often associated with sparsity of population and poor local and interregional infrastructure facilities and has therefore a negative connotation (Martinus et al., Citation2020). Peripheral regions are seen as disadvantaged compared with core regions (Copus, Citation2001). Periphery as a remote location implies that this condition remains unchanged over time, as the distance to the city centres and the low population density are not changing dynamically (Kühn, Citation2015). Periphery is usually described through the perspective of the core (Pugh & Dubois, Citation2021), whereby the core is the opposite of periphery. Broadly, the core has a critical mass of innovative firms, a high level of suppliers and supporting organizations, strong technological resources, and absorptive capacity (Mudambi & Santangelo, Citation2016). The core can be delimited from the periphery by having a higher population, job and infrastructure density, and the presence of institutions of higher education. However, there exists some conceptual fuzziness in terms of thresholds and how you define quantitatively a critical mass or high levels thereof (Pugh & Dubois, Citation2021).
From the innovation-oriented perspective of knowledge-intensive services firms – here, APS firms – locating in core regions has several advantages. APS are important economic agents that transfer knowledge to other agents (Gallego & Maroto, Citation2015). Their business is based on exchanging or selling knowledge to customers, whereby co-creation and customer interaction are important (Kohtamäki & Partanen, Citation2016), which makes locating in agglomerations beneficial (Muller & Doloreux, Citation2009). In addition, firms profit from a large local market and skilled workers, knowledge-spillover processes between firms and a high supply of resources like transportation infrastructure (Wagner & Growe, Citation2022). However, Hanssens and Derudder (Citation2011) show that the pattern is more complex: the geography of APS in the context of economic globalization shows a crisscross of transaction links between smaller cities as well as between larger and smaller cities. This multitude of connections does not have one dominant region, but an overlap of spheres of influence beyond national borders and Euclidean distance, indicating that the geography of APS is characterized by the firms’ inclusion in the hierarchies of multinational companies (Hanssens & Derudder, Citation2011). In addition, the definition of the term ‘region’ has changed throughout paradigmatic shifts in spatial analyses – one of such shifts being the change from conventional territorial interpretations towards the radically different spatiality of flow and relational networks of connectivity (Macleod & Jones, Citation2007).
2.2. Relational core–periphery patterns
The relational or functional approach (Dicken & Malmberg, Citation2001) considers relations as either physical flows like transactions between firms or non-physical flows like knowledge spillovers, based on Castell’s theory on the ‘space of flows’ (Castells, Citation2000). Here, our focus lies on non-physical relations in the form of knowledge flows within a firm’s location network as operationalized in Sassen’s research. Increasing globalization alters the way knowledge-intensive firms span their value-chains and thus their locations across space. Sassen (Citation2001) states that APS play a predominant role in the globally networked knowledge economy and the changing core–periphery patterns. Following the development of the firms they service, APS firms themselves have globalized during the last decades. As a result, several cities have become main markets for these advanced services through corporate presences and innovative knowledge clusters, altering the geographies of globalization by forming transnational urban systems (Sassen, Citation2019). These transnational urban systems result in a new geography of centrality cutting across existing core-periphery patterns in the world economy (Sassen, Citation2001).
In terms of relational thinking, the concept of networks is beneficial for making sense of cities and regions (van Meeteren et al., Citation2016). Also, van Meeteren (Citation2021) makes a case that the centre–periphery relationship is ‘more meaningfully depicted as a network’ (p. 263). In network science, core–periphery is a structural and analytical pattern based on the idea that understanding the relationship between core and periphery is important for understanding the system as a complex entity (Nordlund, Citation2018).
Networks are characterized by a core – a central and densely connected set of nodes – and a periphery – a non-central and sparsely connected set of nodes linked to the core. All nodes of the core are topologically central, but not all central nodes form a network core. A specific kind of network structure are core/periphery type of networks (Csermely et al., Citation2013). They have a highly connected core which also often contains hubs and peripheral nodes, which are preferentially connected to the core but usually not connected to other peripheral nodes (Csermely et al., Citation2013). The core–periphery structure often implies a power relation in the form of peripheral dependency and core dominance (Nordlund, Citation2018). This originates from the dependency school, arguing that the underdevelopment in the periphery is a result of its relationship with the centre (Frank, Citation1967; Santos, Citation1970).
However, over time, the network core usually has less fluctuations as it is more constrained and hence changes happen slowly. In contrast, the periphery has more fluctuations. In contrast to the territorial perspective, the network perspective argues that there is an opportunity for knowledge creation and innovation in the periphery (Glückler, Citation2014).
‘The core–periphery imagery has anchored, in spatial planning and economic thinking, the idea that one can objectively delineate and characterize peripheries as geographical objects’ (Pugh & Dubois, Citation2021, p. 270). What makes researching the core–periphery patterns difficult is its complexity and definition, as well as the missing empirical research about it. As Grillitsch et al. (Citation2021) argue, we need to open the ‘black box’ of regional development and understand the more complex scenario than a simple growth–decline binary. The authors find that firms located in the periphery are well able to compensate missing localized knowledge spillovers through more international and distant collaborations (Grillitsch et al., Citation2021). However, ‘we need more empirical research into different aspects of economic development and functions to establish more clearly what is actually going on in peripheral economies and firms’ (Pugh & Dubois, Citation2021, p. 270). Overall, current empirical literature acknowledges that peripheralization is a relational process with dynamic overlapping and trans-scalar networks, thus challenging the traditional perspective of a nested core–periphery pattern as an organizing principle of space.
2.3. Measuring connectivity between locations
The Globalization and World City (GaWC) research network has developed the Interlocking Network Model for empirically measuring inter-city relations. The research network uses Sassen’s research as a starting point for exploring how APS connect cities in globalization (Taylor et al., Citation2020). A city’s functional abilities are being measured in terms of trade, finance, technology, communications, and politics to create a hierarchical core–periphery pattern (Benton-Short et al., Citation2005). This spatial logic of APS firms shows that in a minority of regions, the capacity to acquire new knowledge is much higher than in most regions (Lüthi, Citation2011). In this minority of regions, there is a higher amount of information transferred as they are often the locations of leading-edge knowledge in specialized activities (Bathelt & Glückler, Citation2011). As a result, a functional core–periphery pattern emerges, based on the strategic locational choice of multi-branch firms and their use of cities and regions as locations of value-adding activities (Lüthi, Citation2011).
The Interlocking Network Model (INM) provides a possibility to empirically measure inter-city relations despite the chronic lack of data on inter-city relations (Taylor, Citation2001). It was developed to measure the connectivity between global cities based on multi-branch APS firms as they organize business activities across their offices worldwide (Pain & Hall, Citation2008). The model uses intra-firm networks of multi-branch, multi-location enterprises as a proxy for estimating potential flows of knowledge-creating information between cities and towns. It is assumed that knowledge is shared in intra-firm networks of the knowledge economy. Hereby, it is further assumed that more knowledge flows between headquarter and an office than between two offices. According to Taylor and Aranya (Citation2008), the main contribution of the INM to reveal the complexities of polycentric mega-city regions is threefold: first, inter-city relations show how the corresponding cities are functionally connected with each other; second, the total connectivity of a city on a regional scale provides an initial measure for its position in the functional urban hierarchy; and third, the total connectivity of a city on a global scale provides an alternative measure for its position in the functional urban hierarchy, emphasizing particularly the outside orientation of a city in terms of APS activities.
The INM has been further developed including statistical modelling of network formation processes. Examples include Liu et al. (Citation2015) using exponential random graph models, and Liu et al. (Citation2013) and Zöllner et al. (Citation2020) using stochastic actor-based modelling. Since we are not interested in statistically testing network formation processes but in a qualitative before–after analysis, we use the INM, respectively an extension of it, as will be explained in the methods section.
3. EMPIRICAL SETTING: GERMANY
This section investigates existing research on the German urban system with a focus on empirical relational studies about the distinct structure of the German urban system. Germany is an interesting case for studying the core–periphery patterns of the knowledge economy since it is Europe’s biggest economy in absolute terms, and it has a historically grown polycentric, decentralized-federal urban structure.
Whilst the populations of the largest cities such as Berlin, Hamburg, Munich, Cologne, Frankfurt, Stuttgart and Dusseldorf have been growing until the advent of the COVID-19 pandemic, small and medium-sized cities lost inhabitants from 2005 to 2019 (BBSR, Citation2021a) but seem to have regained them (BBSR, Citation2022). Although the more centrally located ones grow again, a decreasing density in the use of demographic and economic assets can still be observed in eastern Germany today (Nelle et al., Citation2017). Heider (Citation2019) shows that various urban centres have increased their specialist knowledge bases and the share of knowledge-based service employment still increases today (Statista, Citation2021).
The dynamics of knowledge exchange in APS firms have increased regional economic inequality in Germany (Heider, Citation2019). There is an increasing polarization between the development of metropolitan regions and sparsely populated rural areas in Germany (BBSR, Citation2020b). Whilst growth impulses in Germany increasingly concentrate in a few large city regions, many peripheral rural areas increasingly must cope with job and population losses (BBSR, Citation2020b). Nearly all peripheral rural areas in eastern Germany and parts of western Germany are affected by these developments. In line, also the Federal Government Commissioner for the New Federal States (Citation2018) argues that the continuous shrinkage of structurally weak rural East German regions is a form of peripheralization.
Germany’s most important economic sectors in 2019 are automotive, mechanical engineering, chemistry/pharma, electronics and electrical engineering (Statista, Citation2020). Important centres and economic engines are the 17 metropolitan regions, for example, Hamburg, Munich, Nuremberg, Berlin–Brandenburg and Frankfurt–Rhine-Main (BBSR, Citation2021b). In each metropolitan region there is a polycentric internal structure (Krätke & Brandt, Citation2009). For example, the metropolitan region Hanover–Brunswick–Göttingen consists of three urban centres with different economic profiles (Krätke & Brandt, Citation2009). Hanover hosts the service economy; Brunswick is an industrial centre in combination with the nearby VW Wolfsburg plant and Göttingen is a centre of knowledge intensive industries and services as well as science. In contrast to almost all other European countries, Germany does not have one dominant centre but rather a polycentric urban system (BBSR, Citation2010), which makes a relational analysis over time highly interesting.
There are few relational studies about knowledge-intensive intra-firm networks in Germany with time comparisons. Hoyler (Citation2011) analyses Germany’s connectivity changes from 2000 to 2008 in relation to the global world city network of APS firms. The findings show that German cities have experienced a connectivity decline and, hence, lower integration into the world city network. Three out of the top 10 global cities facing the highest decrease in relative connectivity are in Germany: Cologne, Düsseldorf and Frankfurt (Derudder et al., Citation2008). The author follows a top-down approach, taking a small sample of the 100 largest global firms in 315 cities worldwide, amongst them just 14 German cities. This has some implications in the results, as they only provide a small proportion of the linkages of few German cities.
In contrast, bottom-up approaches start their data collection at the national scale and integrate more firms (Lüthi et al., Citation2018). Growe and Blotevogel (Citation2011) combine a relational and a territorial perspective by analysing connectivity changes within Germany for 20 metropolitan regions and their development in terms of APS employment. They confirm Hoyler’s (Citation2011) results concerning the negative connectivity development of Dresden within Germany between 2002 and 2009. The knowledge-based employment increased the most between 1997 and 2007 in the metropolitan regions which already had a large labour pool: Munich, Frankfurt, Hamburg and Berlin. The most dynamic regions are Cologne and Munich. Besides this affinity between knowledge-based work and large metropolitan regions, there is still an East–West divide in the German urban system, as only one region – Berlin – is in the east of Germany.
Growe (Citation2012) compares territorial data in the form of APS employment with relational data in the form of physical commuter flows. The three metropolitan regions of Berlin, Munich and Rhine-Main show different developments from 1997 to 2007. Berlin is monocentric: most employees are in the few core cities of the region. The author observes a shrinking in the hinterlands whilst the core is growing, so to speak a centralization process. In Rhine-Main, the employees are in centres of the surrounding cities to a larger extent. The author observes a growth in centres of medium and upper centrality and a catch-up of rural areas. In the metropolitan region of Munich, most employees are located in other counties in the urban hinterland and the author observes a growth in these areas without cores. So to speak, the regions of Rhine-Main and Munich show peripheralization processes.
Growe (Citation2013) shows that APS firms have a high affinity to locate in urban centres, hence cores show higher connectivity values. However, the author observes positive relative changes mainly in regional hinterlands and negative relative changes mainly in core cities between 2002 and 2009. This indicates a saturation effect in the major cities: as more APS firms locate outside these cores, the surrounding areas gain in relative importance. This is especially distinct in Munich.
Growe and Volgmann (Citation2016) analyse connectivity changes inside the German urban system from 2002 to 2009 and find that the distribution of connectivity became less polarized. In addition, results show a low performance of East Germany, especially Leipzig, Chemnitz, Dresden and Berlin are the four city regions with the highest negative relative connectivity change. This is surprising for Berlin as a high political effort is made to promote the knowledge economy in the capital. Berlins has a share of 18% of all metropolitan functions in the country, it is Germany’s biggest city, has a certain power and is dominant in territorial terms. The authors further find that the East–West divide is still visible: eastern regions are rather unimportant hubs within the knowledge economy networks in Germany, whereas western German cities such as Hamburg, Cologne, Dusseldorf and the large hub of Munich in the south have gained in importance. Overall, the largest share of connectivity was found in the three largest cities and in the huge polycentric Rhine-Main city region.
Wagner and Growe (Citation2022) find an absolute economic re-urbanization in Berlin/Potsdam, Leipzig and Hamburg, meaning that the core develops better than the surrounding based on employment change. The authors find a relative economic re-urbanization in the Munich region, meaning that the entire region is developing well with a slight overhang in the core. The study did not find any regions with a relative economic regionalization, where a region is developing well with a slight overhang in the surrounding area.
From this conceptual section, we conclude that the difficulty of researching core–periphery patterns concern the complexity of definitions from territorial to relational, the varying spatial scales as well as the temporal changes. From the empirical section, we conclude that there exists some research about connectivity changes within Germany for major regions and from previous decades. The innovative contribution of our empirical analysis lies with the inclusion of the international connectivity and the relationship between global and local relations. Further, we collected current data, and our analysis covers the whole country. In addition, we advance the relational core–periphery discussion by mirroring our findings against the spatial categories using innovative visualizations.
3.1. Research questions
The focus of our research are the changing core–periphery relationships in German regions between 2009 and 2019, based on the locational behaviour of APS firms. The objective is to explore the dynamic spatiality of economic activities by assessing whether and to what extent German core and peripheral regions are on a path of integration through the intra-firm networks of APS firms, that is, how the processes of centralization and peripheralization work in Germany. The findings will be compared against the backdrop of the territorial classification of urban, semi-urban and rural regions based on the definition of the BBSR (Citation2020a). We analyse the following questions:
How does the development of connectivity in Germany differ between urban and rural regions between 2009 and 2019?
In what way does this development differ if we further distinguish between connectivity to locations outside of Germany (called globalism) and connectivity to locations inside Germany (called localism)?
How does this development fit to the territorial classification of urban, semi-urban and rural regions based on the definition of the BBSR?
4. METHODS AND DATA
In this section we explain our conceptualization for measuring core–periphery patterns and our unique relational longitudinal dataset. Hereby, the focus is on a before–after comparison, not a statistical time-series analysis.
4.1. Measuring core–periphery patterns
We use the INM as a basis to reveal the complexities of core–periphery patterns in Germany and extend it according to the critiques raised by Derudder et al. (Citation2010). These authors explain that the sample is not comparable over time since the number of firms in each sector changes. Due to mergers, acquisitions or bankruptcies, there are fewer and fewer firms in the sample over time. We counter this issue in the same way as Derudder et al.: we use for robust data a predefined number of firms for each sector selected via a ranking of their importance, in our case in terms of size measured by employees. In addition, we also go beyond a limited set of world cities and even include the entire area of Germany and all global locations where the firms have offices.
Derudder et al. (Citation2010) raise the issue that there is a possible underestimation of changes in the highly connected cities at the top of the ranking: they have less possibility to acquire new offices as all firms already have major offices in these cities. However, this is not a problem for our research since we analyse relative rank changes, that is, the order of functional urban areas (FUAs), and whether other FUAs have become relatively more connected. In addition, Growe and Blotevogel (Citation2011) did not find any statistical evidence for this when studying changes within the German urban system (localism) between 2002 and 2009. Their explanation is the relatively balanced structure of the German urban system, compared with the more hierarchical global city network.
For the INM, we first record all locations of one APS firm. The locations are rated on a scale from 0 (no presence) to 5 (company headquarter). The most common value is a 2 (standard presence). If a location has a special relevance within the intra-firm network (e.g., regional headquarters), we upgrade the value to 3 or even 4. If a location has a particularly low importance in the firm network (e.g., small agency), we downgrade the value to 1. These values are called service values. We repeat this for all the APS firms in our sample. The result is a basic matrix with cities in the rows and firms in the columns.
4.1.1. City interlock and interlock connectivity
In a second step, we calculate different measures that estimate how well connected the cities are within the intra-firm network of APS firms. The INM distinguishes between different connectivity values. The connectivity between two cities of a certain firm represents the so-called elemental interlock, calculated by multiplying the service values for one firm. The elemental interlocks for all firms located in these two cities are summarized to calculate the total connectivity between the two cities, the so-called city interlock. The city interlock is a proxy for the potential flow of knowledge-creating information between FUAs. The knowledge flow arises through intra-firm networks of APS firms operating through their locations in these FUAs.
Aggregating the city interlocks for a single city produces the interlock connectivity. This measure describes the importance of a city within the intra-firm network of all APS firms that have been analysed. A high interlock connectivity is the result of a large number of office locations from the respective firms in a city, a large amount of office locations worldwide from these firms and a high rating of the service values (Lüthi et al., Citation2018).
Ranking the interlock connectivity values for all FUAs produces a functional urban hierarchy. The higher the interlock connectivity of an FUA, the higher its position in this hierarchy. The functional urban hierarchy can be further divided into a national urban hierarchy – that is, the positioning of FUAs according to its connections inside a country – and a global urban hierarchy – that is, the positioning of FUAs according to its worldwide connections.
In technical terms, the interlock connectivity is a nodal attribute arising through weighted edges and represents a ‘node strength’ (Opsahl et al., Citation2010). In a network, nodes are connected by edges. displays a schematic representation of such a network. It serves as an example for our terminology. In our case, the links between cities have a strength represented by a numerical value. In network theory, this is called an edge weight. In the frame of the GaWC research, the edge weight is called city interlock. For the nodes, network theory uses several measures of centrality. The degree centrality in a weighted network results from the sum of the weights for each node. For example, a city’s degree centrality represents the number of ingoing ties into the city. In GaWC research, the degree centrality is called interlock connectivity.
These connectivity values can be calculated on different spatial scales, for example, on a regional, national or global scale. These trans-scalar networks can show different temporal developments. Hence, we differentiate between a connectivity value within Germany, which we call ‘localism’ – and a connectivity value outside of Germany, labelled ‘globalism’.
4.1.2. Localism and globalism
In our relational approach, the goal is to understand economic action in space. To identify changes in connectivity, calculating a relative increase or decrease in connectivity is central. Hereby, core–periphery does not end at the border of Germany, as our firms also operate globally. For this reason, we do a multi-scalar analysis.
Starting from this basic calculation of connectivity (city interlock and interlock connectivity), we then analyse the relative concentration of connections inside of Germany (localism) and outside of Germany (globalism) for each FUA. Localism measures the relative concentration of connections within Germany. For example, we calculate localism for Hamburg as:
(1)
(1) We then rank the localism values of the FUAs for 2009 and 2019 separately. Then we calculate the rank changes as the difference between a FUA’s rank in 2019 and in 2009. Also here it is important to emphasize that we focus on the rank changes, not the changes in terms of absolute localism values.
We differentiate between two types of globalism: traditional and new globalism. Traditional globalism, on the one hand, is the relative concentration of connections to New York and London. The reason for using this dyad abbreviated with NYLON is based on the findings of Taylor et al. (Citation2020). The authors demonstrate the pre-eminence is much higher and much more diverse than previously known (Taylor et al., Citation2020). The calculation of globalism is like the one for localism. Using the example of Hamburg again, we calculate the traditional globalism for Hamburg as:
(2)
(2) On the other hand, new globalism is the relative concentration of connections to Beijing, Hong Kong and Shanghai (Taylor & Sánchez-Moral, Citation2013). This new emerging dyad of contemporary globalization or China globalization that may dominate the 21st century (Taylor, Citation2012). For example, we calculate the new globalism for Hamburg as:
(3)
(3) We then add up both globalism values for each FUA and rank the globalism values of the FUAs for 2009 and 2019 separately. Then we calculate the rank changes as the difference between a FUA’s rank in 2019 and in 2009 and visualize these rank changes.
4.2. Data
The starting point of our analysis is the German federal definition and delimitation of core and periphery. We use the territorial conceptualization of core and periphery based on the Spatial Planning Report of the German BBSR (). The BBSR categorizes Germany at the municipality level. There are three categories based on the share of population and the population density: urban areas, semi-urban areas and rural areas. Regions are urban if at least half of the population lives in large or medium-sized cities with a population density of minimum 150 inhabitants/km². Regions are semi-urban if the population density is less than 150 inhabitants/km². If the population share in large or medium-sized cities is lower than 50% and the population density below 100 inhabitants/km², the region is rural (BBSR, Citation2020a).
Figure 2. Three-level classification of Germany according to the Federal Office for Building and Regional Planning (BBSR) definition 2014.
Source: Authors’ own map.
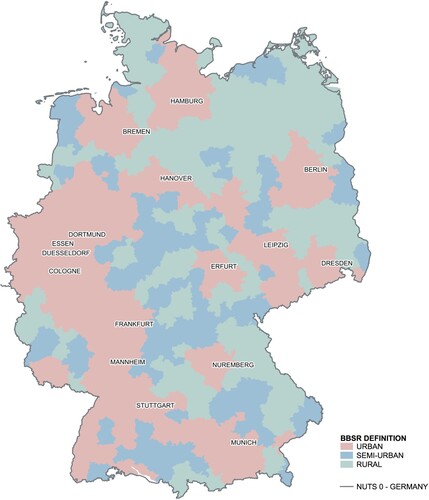
For our analysis of the core–periphery connectivity patterns in Germany, we choose a relational conceptualization. We use 186 FUAs – or agglomerations – as analytical building blocks. FUAs better reflect the actual functional orientation of firms, communities and people and allow a more realistic mapping of the economic activity (Garas et al., Citation2015). The concept of FUAs was developed by the European Spatial Planning Organization Network (ESPON) (Citation2005). They are defined as having an urban core of at least 15,000 inhabitants and over 50,000 in total population. For each FUA, the potential area has been calculated that can be reached within 45 minutes by car from the FUA centre. This implies that the FUA is conceptualized as a functional entity rather than an administrative territory of a continuous built-up area. An important aspect are the border regions. We include all FUAs that have their urban core in Germany, even if they have a small fraction of their area in another country. shows large urban areas in the south and west of the country, as well as large rural areas in the north-east and south-east of Germany.
In operationalizing APS, we follow a sector approach proposed by Legler and Frietsch (Citation2006) and Gehrke et al. (Citation2013). The authors publish a list of knowledge-intensive services based on the German classification of commercial sectors (WZ-2008). The relevant industries show a high share of participation in activities of knowledge generation and activities for strengthening the advancement of human capital (Gehrke et al., Citation2013). We include eight APS sectors in our analysis as shown in .
Table 1. Advanced producer services (APS) sectors included in the analysis.
Following the GaWC method and its improvements suggested by Derudder et al. (Citation2010), we build the 2019 dataset. We identify the 30 largest firms in terms of employees for each APS subbranch using the German Hoppenstedt database – one of the largest business data providers in Germany. The database includes over 245,000 profiles of German companies. Overall, we collect location information for 240 APS firms in 2939 worldwide locations.
For 2009, we use a similarly constructed dataset from Lüthi et al. (Citation2011). This means that our final dataset is not a pure panel data since we do not follow the same firms 2009 and 2019. However, it is not a pooled cross section either, since we do not take random samples, but the 30 largest firms in terms of employees for the defined subsectors. We conduct a before–after analysis of these two time points using the INM.
We focus on rank changes and not on overall connectivity changes. Derudder et al. (Citation2010) illustrate the difference for Chicago. The city had approximately the same level of connectivity in both time points. But Chicago nevertheless lost 12 places in the ranking because other cities have relatively become more connected. This is exactly what we are interested in, so analysing rank changes is fine for our case.
A last comment concerns the critique that firms rather than cities are agents of change. It is visible from the calculation of the INM that this is incorporated in the calculation itself. So, the argument that change is more than cities ‘rising or falling’ we agree – but rising or falling means in our context cities become more or less integrated into the intra-firm networks of APS.
5. RESULTS AND DISCUSSION
In the following section we analyse and discuss the key findings. In the first subsection we compare the territorial core–periphery pattern as defined by the BBSR (urban, semi-urban and rural) with the relational connectivity changes of FUAs. In the second subsection we compare the territorial core–periphery pattern to the relational changes in globalism and localism.
5.1. Connectivity changes in the core–periphery pattern of German FUAs
In this subsection we analyse changes in the global connectivity of German FUAs and how the ranking of these FUAs has changed accordingly. Our first research question asks how the connectivity development in Germany differs between urban and rural regions between 2009 and 2019.
We start by displaying the 10 FUAs with the highest increase in the ranking from 2009 to 2019 in the left column of . The centre column shows the then FUAs with the highest decrease in the ranking and the right column shows all seven FUAs that had the same rank in both time points (for the complete data, see Appendix A in the supplemental data online).
Table 2. Rank changes in global interlock connectivity in German functional urban areas (FUAs), 2009–19.
We first analyse the top 10 increase. Here, half of the FUAs are (semi-)urban and the other half are rural. We check if the FUAs are part of a German mega-city region. In this context, we are talking about functionally polycentric mega-city regions, not the political concept of metropolitan regions (Lüthi, Citation2011). They are neither based on administrative units nor on spatial development policies, but in functional terms. They are polycentric to different degrees, including patterns of interaction between urban centres and secondary or tertiary agglomerations (ESPON, Citation2005). Eight FUAs are secondary cities within mega-city regions, for example, Lueneburg belongs to the mega-city region of Hamburg, Cottbus and Brandenburg to Berlin–Brandenburg and Rosenheim to Munich. Only two FUAs, Bad Hersfeld and Iserlohn, are located outside of a mega-city region. We cannot interpret an increase in connectivity in urban regions. Rather, there is a tendency that the connectivity increases in secondary cities within mega-city regions, no matter how they are classified according to the BBSR.
We then analyse the top 10 decrease. Here, all FUAs are (semi-)urban. Half of the FUAs are secondary cities within mega-city regions (e.g., Kleve and Gummersbach belong to the mega-city region of Rhine-Ruhr and Dessau to the Saxony Triangle). The other half of the FUAs are located outside of mega-city regions. This means that being located inside a mega-city region does not automatically indicate that the FUAs connectivity increases over time; being classified as rural does not automatically indicate a decreasing connectivity. Analysing the FUAs with unchanged ranks, we see that all of them are classified as urban.
In addition, all are primary cities within mega-city regions. This descriptive analysis shows that for the top 10 FUAs, their territorial categorization and whether they are part of a mega-city region does not talk much about their connectivity development relative to other FUAs. There might be a tendency that FUAs classified as urban do not increase their connectivity; however, this is not the whole picture, and we lack the geographical information.
We now move on to our visualization and beyond the top 10 FUAs. We start with the map from and add a new layer to combine the territorial data with our relational data, comparing temporal relational changes with the federal three-level spatial categorization.
shows the rank changes of FUAs based on global interlock connectivity between 2009 and 2019. A (+) indicates that the FUAs has moved up in the ranking, a (–) indicates a decrease in the ranking and a (0) indicates an unchanged position. The borders of the individual FUAs are not displayed, to keep the map readable.
Figure 3. Interlock connectivity rank changes between 2009 and 2019 in Germany. Source: Authors’ own map.
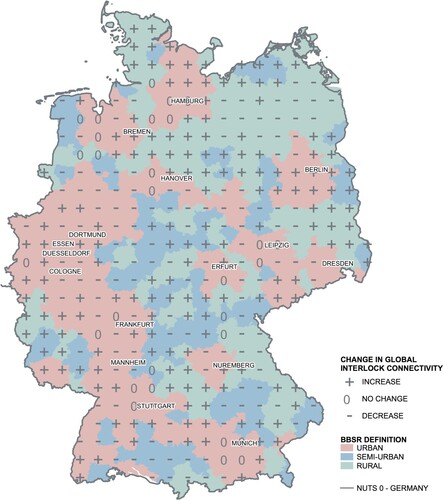
When we look at the structural features of German settlements according to the BBSR, we see that there is an East–West divide: there are more rural areas in the east and more urban areas in the west of Germany. However, when we add the information about the change in connectivity, this East–West divide is no longer visible. Here our findings contrast Growe and Volgmann (Citation2016), who still find an East–West divide in terms of a lower performance of East Germany and increasing connectivity in western regions. However, the authors analysed connectivity changes inside the German urban system from 2002 to 2009.
shows that the highest decreases in connectivity are in urban and semi-urban regions, whilst the highest increases in connectivity can be found in all three spatial categories.
Looking at the urban regions in Germany, there is no clear answer for our first question. The urban regions of Berlin, Hamburg and Bremen, for example, have increased their positioning in the ranking of connectivity relative to the other FUAs. In other words, they have become more integrated into the APS intra-firm networks over time. In contrast, the urban regions of Dresden and Nuremberg have decreased their positioning in the ranking of connectivity relative to the other FUAs. They have become less integrated into the APS intra-firm networks over time and are therefore getting more peripheral in relational terms. Some urban regions show a heterogenous pattern with both an increase and decrease of connectivity, for example, Rhine-Ruhr (Dortmund, Essen, Duesseldorf, Cologne), Frankfurt/Rhine-Main, Stuttgart, Hannover and Erfurt. There are also some urban regions that remain unchanged, for example, Leipzig and Munich. We also see that some border regions have intensified their intra-firm exchange, for example, FUAs at the border to Poland, Czech Republic, Denmark, the Netherlands and Luxembourg. We can interpret this increasing connectivity with a process of centralization at these borders. Based on the dynamic locational behaviour of APS, these geographically peripheral regions go through a process of centralization.
Rural regions also show a quite heterogeneous pattern. Large rural regions in Mecklenburg-Vorpommern in the north of Berlin, north-east of Nuremberg and in the south of Germany along Lake Constance show a decrease in connectivity. In contrast, the rural regions between Hannover and Hamburg, between Stuttgart and Nuremberg and in the south of Erfurt show increasing connectivity values.
We now focus in two interesting examples with contrasting developments. First, the metropolitan region of Munich in the south of Germany. In the city of Munich itself, the connectivity remained stable during the last 10 years. In the urban areas outside the central city, the connectivity increased. This centralization of Munich’s hinterland through APS intra-firm networks illustrates the spillover effect of an economically powerful city into the semi-urban and even rural hinterland. For example, Deggendorf hosts one of the main systems suppliers for BMW. It is located 1.5 hours north-east of Munich and rural according to the BBSR. Deggendorf has gained 34 ranks from 2009 to 2019. This is consistent with Wagner and Growe (Citation2022) who find that the surrounding areas of large German cities, especially in the south of Germany, are attractive locations for knowledge-intensive occupations, combining supply and innovation functions and strengthening the supply infrastructures in the surrounding areas. In Bavaria, the historically grown structure of small and medium towns is supported by funding structures, which increases their attractiveness (Wagner & Growe, Citation2022). However, since Munich itself remained unchanged in the ranking over time, we cannot confirm the relative economic re-urbanization in the Munich region found by Wagner and Growe (Citation2022), where the entire region develops well but the core even better.
A second example is Dresden in the east of Germany. Here, the connectivity in the urban core has decreased during the last 10 years. Dresden’s economy is dominated by service sectors, which employ 80% of the inhabitants and generate 80% of the city’s gross value added (Kluge, Citation2016). It appears that APS firms have moved to the smaller but still urban region of Chemnitz/Zwickau (plus 17 ranks) in the south-west of Dresden and to the north into rural regions. These secondary cities have increased their connectivity. In line, Wagner and Growe (Citation2022) find that the knowledge-intensive employment for Zwickau rose between 2012 and 2019. Zwickau is about one hour by car from Dresden. However, we cannot confirm their finding of a complete or predominant relative decrease of importance in the regions of eastern Germany. Rather, we see network effects as a possible interpretation of our map. Network economies describe a spatial spread, where firms move to smaller cities surrounding an urban core. Still maintaining a certain connection to the core and being geographically close allows firms to profit from more beneficial conditions outside of the centre regarding availability of office spaces and lower rents. As Hoyler (Citation2008) remarks, there is a limit to the amount of cities in one region that can become important hubs for global service activities and this limit might be reached in some German regions.
All in all, we cannot see a general trend of urban areas increasing their connectivity during the last 10 years as we would expect to happen through processes of agglomeration economies and centralization processes. Not all urban FUAs have moved up in the German functional urban hierarchy. Accordingly, not all rural FUAs have lost relative position in the German core–periphery pattern. We cannot see a general trend of peripheralization in rural areas. A possible explanation for an increase in connectivity in peripheral regions could be the spillover of economic activity into the urban hinterlands when firms move to secondary or tertiary cities. This process might be reinforced by improved rail and road access (Wenner et al., Citation2019).
Generalizing our findings for our first question, we observe a tendency for a connectivity increase if the FUA is a secondary city within a mega-city region. FUAs remain rather constant if they are both classified as urban and are primary cities within mega-city regions. The patterns for a decrease in connectivity remain unclear except that the highest decrease can be found in FUAs classified as (semi-)urban. Other than that, the BBSR classification of urban, semi-urban and rural is so far not a helpful indicator for connectivity changes over time.
5.2. Changes of globalism and localism in the core–periphery pattern of German FUAs
In this section we analyse changes in globalism and localism in the core–periphery pattern of German FUAs. Our second research question asks in what way the connectivity development differs if we differentiate between connectivity to locations outside of Germany (globalism) and connectivity to locations inside Germany (localism). We start by displaying the 10 FUAs with the highest increase in the ranking of globalism from 2009 to 2019 in the left column of . The right column shows the 10 FUAs with the highest increase in the ranking of localism (for complete data, see Appendix B in the supplemental data online).
Table 3. Rank changes in globalism and localism in German functional urban areas (FUAs), 2009–19.
We see that Friedrichsdorf has a high increase in both globalism and localism. The FUA is located 20 km from Frankfurt (Main). Analysing the increase in globalism, we see that all FUAs are urban. Searching for other possible patterns, we check if the FUAs are part of a German mega-city region. Here, we see that four FUAs are secondary cities within mega-city regions: Friedrichsdorf belongs to the mega-city region of Rhine-Main, Potsdam to Berlin–Brandenburg, and Essen and Aachen to Rhine-Ruhr. The other six FUAs are located outside of a mega-city region. For these 10 most extreme cases, the first part of our hypothesis seems to fit well: these urban regions have increased their connectivity from 2009 to 2019.
Analysing the increase in localism, we see that all except one FUA are classified as (semi-)urban. Here, we see that four FUAs are secondary cities within mega-city regions (Friedrichsdorf belongs to the mega-city region of Rhine-Main, Wuelfrath to Rhine-Ruhr, Naumburg to the Saxony Triangle and Brandenburg to Berlin–Brandenburg). The other six FUAs are located outside of mega-city regions. It is not the predominant development that rural regions have increased their connectivity to locations within Germany, at least for the 10 most extreme cases. In addition, whether a FUA is part of a mega-city region does not tell us anything about its dynamics in localism.
We now move on to our visualization to get an overview beyond the top 10 FUAs. compares globalism and localism with the territorial core–periphery pattern defined by the BBSR. Shaded areas show FUAs with increasing localism, dotted areas show FUAs with increasing globalism between 2009 and 2019.
Some urban regions such as Hanover, Dresden, Leipzig, Frankfurt, Cologne, Dortmund and Bremen have increased in globalism and hence strengthened their positioning in the ranking of global connectivity relative to the other regions. The higher integration into the network of contemporary globalization can be interpreted as a process of centralization. In contrast, there are urban regions that have increased in localism, for example, Munich, Berlin, Erfurt and Essen. These FUAs have strengthened their positioning in the ranking of national connectivity relative to the other German regions.
This is surprising given the negative description of Dresden’s development by Growe and Volgmann (Citation2016) between 2002 and 2009. In their study, Dresden was among the four regions with the highest negative relative change. Here we see the importance of our differentiation between localism and globalism. Dresden was able to integrate more into the global connectivity by hosting more firms that have global locations. It rose from rank 86 to rank 47, which is a plus of 39 ranks. Also, for Berlin, their negative result cannot be confirmed. In the core of Berlin, we observe an increase in localism and no shrinking of its hinterlands. In contrast, in the north of Berlin there is an increase in globalism.
The same complex and uneven development like in the urban regions can be observed for rural regions. There are rural regions, which have increased in globalism, like the large areas in Mecklenburg–West Pomerania (north of Berlin) and between Hamburg and Hannover. An example is the global logistics firm Rhenus which has opened a new location in Uelzen in the FUA of Lueneburg (overall plus 19 ranks in globalism).
In contrast, the rural regions north of Hamburg, north-west of Berlin and Bautzen (north-east of Dresden) have increased in localism. A reason for the increasing localism in these FUAs could be the large size of the German domestic market, which provides a critical mass of business opportunities and customers for nationally oriented firms. An example is the firm Vodafone from the Information & Communication Services sample. The firm has opened a new location in Bautzen (overall +51 ranks in localism) as being close to customers for individual service is important.
All in all, the classification in urban, semi-urban and rural areas according to the BBSR does not match with the functional orientation of the FUAs. In contrast to Lüthi et al. (Citation2011) – who noted increasing globalism especially in urban regions – we also find increasing globalism in several rural regions. A possible explanation for this phenomenon is provided by Berman et al. (Citation2020), explaining that it is important for innovative firms in peripheral regions to maintain widespread networks to facilitate the flow of knowledge from different parts of the world. They argue that this compensates peripheral disadvantages of resource thinness and weaker local infrastructure (Berman et al., Citation2020). Also, Bathelt et al. (Citation2004) and Eder (Citation2018) argue that peripheral regions can compensate proximity through channels of communication called ‘pipelines’ outside of the region or country. However, not all regions are capable to do this. The FUA of Nordhorn located halfway between Dortmund and Bremen on the German border is classified as rural and had the most dramatic rank decrease: minus 154 ranks in globalism and minus 51 ranks in localism.
To round off the results section, we reflect about our own results. First, our data show how German FUAs are connected through firm locations of large APS firms. We do not consider smaller firms or external suppliers. This has some implications in terms of uncaptured firm dynamics since we cannot generalize our finding to the whole knowledge economy. Second, during our period of analysis, 2009–19, the world is not standing still and several influences such as digitalization, structural economic changes and increased remote work impact firms, which could possibly mask an actual tightening or loosening of firm networks. The connectivity development of Germany was sometimes analysed in combination with employment increase. Today, employment might be less informative as knowledge-intensive jobs become less stationary and current research is concerned with multilocal knowledge workers (Bürgin et al., Citation2022; Reuschke & Ekinsmyth, Citation2021).
6. CONCLUSIONS
The goal of this paper has been to find empirical evidence and theoretical explanations for the changing core–periphery patterns in German functional regions. Network science questions the dichotomy between centre and periphery as the interplay between agglomeration economies and network economies leads to a complex core–periphery pattern in space and time. Peripheralization and centralization are relational processes with dynamic, overlapping, and trans-scalar networks, challenging the traditional perspective of a nested core–periphery pattern as an organizing principle of space. According to Sassen (Citation2010), transnational networks of APS firms lead to a new geography of centrality cutting across existing core–periphery patterns.
To reveal the complexities of the core–periphery patterns in Germany, we analysed the changing intra-firm networks of APS firms between 2009 and 2019 and compared the findings with the territorial classification of urban, semi-urban and rural regions based on the definition of the BBSR (Citation2020a). Our approach is novel in the sense that we consider the entire country, include the international connectivity, examine the relationship between global and local relations and visualize trans-scalar network dynamics. The results show that the territorial classification of urban, semi-urban and rural areas does not match with the functional connectivity of the FUAs. Whilst the BBSR classification and territorial data show a clear East–West divide, this divide is no longer visible when we add relational data on intra-firm exchange between regions.
We do not find a general trend of urban areas increasing their connectivity. In contrast, some urban regions have become less integrated into the APS intra-firm networks and have gotten more peripheral over time in relational terms. We also do not find a general trend of peripheralization in rural areas. Especially some border regions have gone through a process of centralization by intensifying their intra-firm exchange with other regions. Furthermore, we find increasing globalism and localism in both urban and rural regions. However, an increasing connectivity can often be observed in the hinterland of core cities in mega-city regions.
The findings in this paper show that more empirical and conceptual research is needed to reveal the changing core–periphery relationships in the German space economy. The relational approach provides valuable insights into the logic of core–periphery patterns, complementing the classical territorial reading that relies on attributional or point data. Networks encourage us to understand socio-economic phenomena as intertwined and mutually interdependent processes, putting economic actors, their spatial organisation of labour in value chains and thus their spatial locational behaviour at the core of the conceptual framework. By including the time dimension into the relational analysis of core–periphery patterns, further information on the drivers of change in spatial development can be revealed. For example: which consequences has the current COVID-19 crisis on the core–periphery relationships in regional economies? To answer this question for further research, we need a nuanced reading of core–periphery from a network perspective of knowledge-intensive firms and a long-term analytical approach to understand the spatial impact of such external shocks on regional and urban development.
Supplemental Material
Download MS Word (124.7 KB)DISCLOSURE STATEMENT
No potential conflict of interest was reported by the authors.
Additional information
Funding
REFERENCES
- Bathelt, H., & Glückler, J. (2011). The relational economy: Geographies of knowing and learning. Oxford University Press.
- Bathelt, H., Malmberg, A., & Maskell, P. (2004). Clusters and knowledge: Local buzz, global pipelines and the process of knowledge creation. Progress in Human Geography, 28(1), 31–56. https://doi.org/10.1191/0309132504ph469oa
- BBSR. (2010). Metropolräume in Europa. Bonn: Federal Office for Building and Regional Planning https://www.bbsr.bund.de/BBSR/DE/veroeffentlichungen/berichte-kompakt/2010/DL_4_2010.pdf;jsessionid=5D6535092F7D9F9D52D1F5D64996E420.live11291?__blob=publicationFile&v=1
- BBSR. (2022). Atlas der Stadt- und Regionalentwicklung. https://www.bbsr.bund.de/BBSR/DE/veroeffentlichungen/sonderveroeffentlichungen/2022/atlas-der-stadt-und-regionalentwicklung.html
- BBSR. (2020a). Laufende Raumbeobachtung – Raumabgrenzungen: Stadt-Land-Regionen – Siedlungsstrukturtyp. https://www.bbsr.bund.de/BBSR/DE/forschung/raumbeobachtung/Raumabgrenzungen/deutschland/regionen/StadtLandRegionen_Typen/StadtLandRegionen_Typen.html?nn=2544954
- BBSR. (2020b). Städtebauförderung außerhalb von Stadtregionen. https://www.bbsr.bund.de/BBSR/DE/forschung/programme/refo/staedtebau/2010/StaedtefoerderungStadtregionen/01_Start.html?nn=2541684&cms_pos=2
- BBSR. (2021a). Kleinstädte in Deutschland: die wichtigsten Fakten Bonn: Federal Office for Building and Regional Planning https://www.bbsr.bund.de/BBSR/DE/startseite/topmeldungen/downloads/kleinstaedte-bericht-fakten.pdf;jsessionid=0C75A2880CEE2DECFAA2B4A82632BF03.live21303?__blob=publicationFile&v=3
- BBSR. (2021b). Raumordnungsbericht 2021. Bonn: Federal Institute for Research on Building, Urban Affairs and Spatial Development https://www.bbsr.bund.de/BBSR/DE/veroeffentlichungen/sonderveroeffentlichungen/2021/rob-2021-dl.pdf;jsessionid=A9A5F7B731D4FE15BEF157E8501D9677.live21321?__blob=publicationFile&v=4
- Benton-Short, L., Price, M. D., & Friedman, S. (2005). Globalization from below: The ranking of global immigrant cities. International Journal of Urban and Regional Research, 29(4), 945–959. https://doi.org/10.1111/j.1468-2427.2005.00630.x
- Berman, A., Marino, A., & Mudambi, R. (2020). The global connectivity of regional innovation systems in Italy: A core–periphery perspective. Regional Studies, 54(5), 677–691. https://doi.org/10.1080/00343404.2019.1672865
- Bürgin, R., Mayer, H., Kashev, A., & Haug, S. (2022). Far away and yet so close: Urban–rural linkages in the context of multilocal work arrangements. Regional Studies, Regional Science, 9(1), 110–131. https://doi.org/10.1080/21681376.2022.2042370
- Castells, M. (2000). Toward a sociology of the network society. Contemporary Sociology, 29(5), 693–699. https://doi.org/10.2307/2655234
- Copus, A. K. (2001). From core–periphery to polycentric development: Concepts of spatial and aspatial peripherality. European Planning Studies, 9(4), 539–552. https://doi.org/10.1080/713666491
- Csermely, P., London, A., Wu, L.-Y., & Uzzi, B. (2013). Structure and dynamics of core/periphery networks. Journal of Complex Networks, 1(2), 93–123. https://doi.org/10.1093/comnet/cnt016
- Derudder, B., Hoyler, M., Taylor, P., & Witlox, F. (2008). Postscript: Trends and change. In P. Taylor, N. Pengfei, B. Derudder, M. Hoyler, J. Huang, & F. Witlox (Eds.), Global urban analysis (pp. 337–350). Earthscan.
- Derudder, B., Taylor, P., Ni, P., de Vos, A., Hoyler, M., Hanssen, H., … Yang, X. (2010). Pathways of change: Shifting connectivities in the world city network, 2000–08. Urban Studies, 47(9), 1861–1877. https://doi.org/10.1177/0042098010372682
- Dicken, P., & Malmberg, A. (2001). Firms in territories: A relational perspective. Economic Geography, 77(4), 345–363. https://doi.org/10.1111/j.1944-8287.2001.tb00169.x
- Eder, J. (2018). Innovation in the periphery: A critical survey and research agenda. International Regional Science Review, 42(2), 119–146. https://doi.org/10.1177/0160017618764279
- ESPON. (2005). ESPON 1.1.1 Project report. Potentials for polycentric development in Europe. https://www.espon.eu/sites/default/files/attachments/full_revised_version_113.pdf
- Federal Government Commissioner for the New Federal States. (2018). Annual Report of the Federal Government on the Status of German Unity 2018. Berlin: Federal Ministry for Economic Affairs and Energy.
- Frank, A. G. (1967). Capitalism and underdevelopment in Latin America. New York University Press.
- Gallego, J., & Maroto, A. (2015). The specialization in knowledge-intensive business services (KIBS) across Europe: Permanent co-localization to debate. Regional Studies, 49(4), 644–664. https://doi.org/10.1080/00343404.2013.799762
- Garas, A., Rozenblat, C., & Schweitzer, F. (2015). The network structure of city-firm relations. https://arxiv.org/abs/1512.02859.
- Gehrke, B., Frietsch, R., Neuhäusler, P., Rammer, C., & Leidmann, M. (2013). Neuabgrenzung forschungsintensiver Industrien und Güter. http://www.zew.de/publikationen/neuabgrenzung-forschungsintensiver-industrien-und-gueter-niwisizew-listen-2012/
- Glückler, J. (2014). How controversial innovation succeeds in the periphery? A network perspective of BASF Argentina. Journal of Economic Geography, 14(5), 903–927. https://doi.org/10.1093/jeg/lbu016
- Grillitsch, M., Martynovich, M., Fitjar, R. D., & Haus-Reve, S. (2021). The black box of regional growth. Journal of Geographical Systems, 23(3), 425–464. https://doi.org/10.1007/s10109-020-00341-3
- Growe, A. (2012). Emerging polycentric city-regions in Germany. Regionalisation of economic activities in metropolitan regions. Erdkunde, 66(4), 295–311. https://doi.org/10.3112/erdkunde.2012.04.02
- Growe, A. (2013). Regionalisierungsprozesse von wissensintensiven Dienstleistungsaktivitäten: Veränderung des Stadt-Umland-Verhältnisses im deutschen Städtesystem. Raumforschung und Raumordnung | Spatial Research and Planning, 71(2), 129–142. https://doi.org/10.1007/s13147-013-0220-4
- Growe, A., & Blotevogel, H. H. (2011). Knowledge hubs in the German urban system: Identifying hubs by combining network and territorial perspectives. Raumforschung und Raumordnung, 69(3), 175–185. https://doi.org/10.1007/s13147-011-0087-1
- Growe, A., & Volgmann, K. (2016). Exploring cosmopolitanity and connectivity in the polycentric German urban system. Tijdschrift Voor Economische en Sociale Geografie, 107(2), 214–231. https://doi.org/10.1111/tesg.12142
- Hanssens, H., & Derudder, B. (2011). The urban geography of advanced producer service transaction links in Belgium. Belgeo, 1-2, 10–11. http://journals.openedition.org/belgeo/6345
- Heider, B. (2019). What drives urban population growth and shrinkage in postsocialist East Germany? Growth and Change, 50(4), 1460–1486. https://doi.org/10.1111/grow.12337
- Hoyler, M. (2008). German cities. In P. Taylor, N. Pengfei, B. Derudder, M. Hoyler, J. Huang, & F. Witlox (Eds.), Global urban analysis (pp. 224–230). Earthscan.
- Hoyler, M. (2011). External relations of German cities through intra-firm networks – A global perspective. Raumforschung und Raumordnung, 69(3), 147–159. https://doi.org/10.1007/s13147-011-0100-8
- Kluge, J. (2016). Die wirtschaftliche Dynamik in der Stadt Dresden. https://www.ifo.de/DocDL/ifoDD_16-04_34-44_Kluge.pdf
- Kohtamäki, M., & Partanen, J. (2016). Co-creating value from knowledge-intensive business services in manufacturing firms: The moderating role of relationship learning in supplier–customer interactions. Journal of Business Research, 69(7), 2498–2506. https://doi.org/10.1016/j.jbusres.2016.02.019
- Krätke, S., & Brandt, A. (2009). Knowledge networks as a regional development resource: A network analysis of the interlinks between scientific institutions and regional firms in the metropolitan region of Hanover. Germany. European Planning Studies, 17(1), 43–63. https://doi.org/10.1080/09654310802513930
- Kühn, M. (2015). Peripheralization: Theoretical concepts explaining socio-spatial inequalities. European Planning Studies, 23(2), 367–378. https://doi.org/10.1080/09654313.2013.862518
- Legler, H., & Frietsch, R. (2006). Neuabgrenzung der Wissenswirtschaft – forschungsintensive Industrien und wissensintensive Dienstleistungen. Studien zum deutschen Innovationssystem, 22. http://www.niw.de/uploads/pdf/publikationen/StuDIS_2007_22_Neuabgrenzung_Wissenswirtschaft.pdf
- Liu, X., Derudder, B., & Liu, Y. (2015). Regional geographies of intercity corporate networks: The use of exponential random graph models to assess regional network-formation. Papers in Regional Science, 94(1), 109–126. https://doi.org/10.1111/pirs.12060
- Liu, X., Derudder, B., Liu, Y., Witlox, F., & Shen, W. (2013). A stochastic actor-based modelling of the evolution of an intercity corporate network. Environment and Planning A, 45(4), 947–966. https://doi.org/10.1068/a45350
- Lüthi, S.. (2011). Interlocking firm networks and emerging mega-city regions. The relational geography of the knowledge economy in Germany. [Dissertation]. Munich University of Technology, Munich. https://mediatum.ub.tum.de/?id=1321019
- Lüthi, S., Thierstein, A., & Bentlage, M. (2011). Interlocking firm networks in the German knowledge economy. On local networks and global connectivity. Raumforschung und Raumordnung, 69(3), 161–174. https://doi.org/10.1007/s13147-011-0088-0
- Lüthi, S., Thierstein, A., & Hoyler, M. (2018). The world city network: Evaluating top-down versus bottom-up approaches. Cities, 72, 287–294. https://doi.org/10.1016/j.cities.2017.09.006
- Macleod, G., & Jones, M. (2007). Territorial, scalar, networked, connected: In what sense a ‘regional world’? Regional Studies, 41(9), 1177–1191. https://doi.org/10.1080/00343400701646182
- Martinus, K., Suzuki, J., & Bossaghzadeh, S. (2020). Agglomeration economies, interregional commuting and innovation in the peripheries. Regional Studies, 54(6), 776–788. https://doi.org/10.1080/00343404.2019.1641592
- Mudambi, R., & Santangelo, G. D. (2016). From shallow resource pools to emerging clusters: The role of multinational enterprise subsidiaries in peripheral areas. Regional Studies, 50(12), 1965–1979. https://doi.org/10.1080/00343404.2014.985199
- Muller, E., & Doloreux, D. (2009). What we should know about knowledge-intensive business services. Technology in Society, 31(1), 64–72. https://doi.org/10.1016/j.techsoc.2008.10.001
- Nelle, A., Großmann, K., Haase, D., Kabisch, S., Rink, D., & Wolff, M. (2017). Urban shrinkage in Germany: An entangled web of conditions, debates and policies. Cities, 69, 116–123. https://doi.org/10.1016/j.cities.2017.02.006
- Nordlund, C. (2018). Power-relational core–periphery structures: Peripheral dependency and core dominance in binary and valued networks. Network Science, 6(3), 348–369. https://doi.org/10.1017/nws.2018.15
- Opsahl, T., Agneessens, F., & Skvoretz, J. (2010). Node centrality in weighted networks: Generalizing degree and shortest paths. Social Networks, 32(3), 245–251. https://doi.org/10.1016/j.socnet.2010.03.006
- Pain, K., & Hall, P. (2008). Informational quantity versus informational quality: The perils of navigating the space of flows. Regional Studies, 42(8), 1065–1077. https://doi.org/10.1080/00343400701808865
- Pugh, R., & Dubois, A. (2021). Peripheries within economic geography: Four “problems” and the road ahead of us. Journal of Rural Studies, 87, 267–275. https://doi.org/10.1016/j.jrurstud.2021.09.007
- Reuschke, D., & Ekinsmyth, C. (2021). New spatialities of work in the city. Urban Studies, 58(11), 2177–2187. https://doi.org/10.1177/00420980211009174
- Santos, T. D. (1970). The structure of dependence. The American Economic Review, 60(2), 231–236. http://www.jstor.org/stable/1815811
- Sassen, S. (2001). The global city: New York, London, Tokyo. Princeton University Press.
- Sassen, S. (2010). Global inter-city networks and commodity chains: Any intersections? Global Networks, 10(1), 150–163. https://doi.org/10.1111/j.1471-0374.2010.00279.x
- Sassen, S. (2019). Cities in a world economy (5th ed.). Sage Publications.
- Statista. (2020). Umsätze der wichtigsten Industriebranchen in Deutschland in den Jahren von 2017 bis 2019 https://de.statista.com/statistik/daten/studie/241480/umfrage/umsaetze-der-wichtigsten-industriebranchen-in-deutschland/
- Statista. (2021). Anteil der Beschäftigung in wissensintensiven Dienstleistungsbereichen an der Gesamtbeschäftigung von 2009 bis 2020. https://de.statista.com/statistik/daten/studie/161772/umfrage/anteil-der-beschaeftigung-in-wissensintensiven-dienstleistungsbereichen/
- Taylor, P. (2001). Specification of the world city network. Geographical Analysis, 33(2), 181–194. https://doi.org/10.1111/j.1538-4632.2001.tb00443.x
- Taylor, P. (2012). Extraordinary cities: Millennia of moral syndromes, world-systems and city/state relations. Routledge.
- Taylor, P., & Aranya, R. (2008). A global ‘urban roller coaster’? Connectivity changes in the world city network, 2000–2004. Regional Studies, 42(1), 1–16. https://doi.org/10.1080/00343400601145202
- Taylor, P., Derudder, B., & Liu, X. (2020). NYLON’s Pre-eminence: The permeability of world regions in contemporary globalization. Geographical Review, 1–27. https://doi.org/10.1080/00167428.2020.1728196
- Taylor, P., & Sánchez-Moral, S. (2013). European cities in globalization: A comparative analysis based on the location strategies of advanced producer services. Springer.
- van Meeteren, M. (2021). About being in the middle: Conceptions, models and theories of centrality in urban studies. Handbook of cities and networks. In Z. Neal, & C. Rozenblat (Eds.), Handbook of cities and networks (pp. 252–271). Edward Elgar Publishing.
- van Meeteren, M., Neal, Z., & Derudder, B. (2016). Disentangling agglomeration and network externalities: A conceptual typology. Papers in Regional Science, 95(1), 1–20. https://doi.org/10.1111/pirs.12214
- Wagner, M., & Growe, A. (2022). Patterns of knowledge bases in large city regions in Germany: Comparison of cores and their surrounding areas. Geografiska Annaler: Series B, Human Geography, 1–21. https://doi.org/10.1080/04353684.2022.2141131
- Wenner, F., Bentlage, M., & Thierstein, A. (2019). High-speed rail and interlocking firm networks of the knowledge economy in Germany. Paper presented at the Annual Conference of the Regional Science Association, Santiago de Compostela, Spain.
- Zöllner, S., Lüthi, S., & Thierstein, A. (2020). A longitudinal network analysis of the German knowledge economy from 2009 to 2019: Spatio-temporal dynamics at the city–firm nexus. Journal of Social Structure, 21(1), 1–27. https://doi.org/10.21307/joss-2020-005