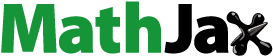
ABSTRACT
Numerous studies have revealed that high-speed railways (HSRs) can efficiently reduce transportation costs for firms and therefore bring more business capacities to firms in regions with HSR services. This paper aims to adopt the difference-in-difference (DID) and PSM (propensity score matching) DID methods to examine the HSR’s causal effects on business costs. The author finds that business costs are significantly reduced due to the operation of HSR lines. Besides, private firms and firms in the secondary and tertiary sectors would experience more reductions of business costs encouraged by the HSR than state-owned and agricultural firms. The placebo test and parallel trend test have proved the feasibility of this study. Finally, this paper provides policy suggestions that the construction of HSR lines needs to be encouraged in order to promote the higher efficiency of business operations for firms and more-balanced spatial business developments in China.
1. INTRODUCTION
The high-speed railway (HSR) is one of the most advanced means of transportation and is typically divided into three categories, based on the minimum speed, i.e., a minimum speed of 250, 200 or 160 km/h (Pyrgidis, Citation2016). The HSR system in China has three speed-based categories: railways with an operational speed ranging from 300–380 km/h on HSR-only tracks, 200–250 km/h on HSR-only tracks and 160–200 km/h on existing tracks (former Ministry of Railways, 2013).
The modern HSR project in China in the twenty-first century started to operate in 2008 with the 6th campaign to raise the speed of railways and the operation of the 300 km/h Beijing-to-Tianjin high-speed railway, days before the opening of the 2008 Beijing Olympic Games. China has witnessed rapid developments of the HSR system since 2008, which has covered 31 provinces with over 150 million people by 2020 and has become one of the largest HSR networks around the world (Zhang & Jefferson, Citation2020). As a fast and modern transportation system, the HSR system is considered to have tremendously improved the efficiency of business developments and reduced the time cost of business operation, which therefore encouraged a higher frequency of interprovincial trades and business (Liu & Zhang, Citation2018; Niu et al., Citation2020). For example, the total trade volume between Beijing and Shanghai, two economic centres in China, increased by 3.6% from 2010 to 2011 and Li and Wang (Citation2013) suggest that the HSR line connecting these two economic centres of China contributed 56% to this increase. Meanwhile, business costs of local firms in regions through which the HSR passes are proven to decrease more rapidly than those in other regions without HSR lines in China, indicating that the HSR significantly contributed to the decrease in business costs (Wu et al., Citation2022).
Business costs are a significant component of daily operations for all companies and can be categorised into fixed and variable costs (Park et al., Citation2005). It refers to all costs generated during the process of business and the method of categorisation (fixed/variable costs) has been applied in numerous studies (Briciu, Citation2008; Roberts et al., Citation1999; Winters & Martins, Citation2004). Fixed costs include business expenses that are not dependent on extra goods or services produced, including rent and lease costs, insurance and utility bills, while variable costs include expenses depending on the firm’s production or sales volume, such as costs of extra materials for production. It has been widely acknowledged (Fama & French, Citation1999; Thomas, Citation1998) that business operators consider business costs a dominant concern since both fixed and variable costs have critical impacts on business behaviours and expectations of corporate growth. Among all kinds of variable costs, the cost of transporting labour resources is one of the most fundamental. For example, Anderson et al. (Citation2008) found that 20% of total business costs for a manufacturing company and 45% of those for a financial company result from costs of business-related labour exchange which belong to variable costs. Current literature reveals that there are two major channels through which a HSR can have positive impacts on reducing business costs. First, the HSR could encourage agglomeration effects of industries and attract firms in the supply chain to be relocated to areas with HSR lines or strengthen the cooperation of firms in areas that are connected by HSR lines so that business costs of supply chain cooperation can be significantly reduced (Ahlfeldt & Feddersen, Citation2018). Such agglomeration effects can be implemented through mechanisms of reducing costs of worker mobility (Heuermann & Schmieder, Citation2019), reducing innovation costs by creating inter-regional innovation cooperations (Komikado et al., Citation2021), and reducing investment costs by benefitting from service-oriented local governments which have stronger intentions of utilising the HSR for business developments (Sun et al., Citation2023). Second, even though the HSR barely serves as cargo trains, the direct reduction of communication costs such as costs of labour and capital exchange can play an important role in the overall reduction of business costs (Blum et al., Citation1997).
To contribute to the current literature, this paper is intended to include some distinctive features. First, most existing papers implemented the macro-level analysis of the correlations between the HSR and corporate performance (Guo et al., Citation2021; Yilmaz & Ari, Citation2017) while this paper adopts a firm-level lens to analyse the impacts of the HSR systems on reductions of business costs, an important determinant of corporate developments. To the best of my knowledge, this research for the first time applied the massive amount of Chinese firm-level data to analysing impacts of HSR on business costs. Second, few papers empirically analysed impacts of the HSR on business costs for firms in regions with HSR lines, especially from a firm-level perspective with a comprehensive firm dataset and the categorisation of dividing business costs into fixed and variable costs. Third, the operation of two major HSR lines, namely the Shanghai-to-Nanjing line (started in 2010) and Beijing-to-Tianjin line (started in 2008), provided us with a quasi-natural experiment since the construction of HSR lines is purely decided by the central government of China and is hardly impacted by local businesses, making the difference-in-difference (DID) analysis applicable to this topic (Lawrence et al., Citation2019). Finally, to eliminate the selection bias, the author completes the propensity score matching (PSM) DID analysis utilising the data of almost all firms on the A-share listFootnote1 in China.
This paper consists of two empirical studies. First, the DID analysis on how the HSR impacts business costs for firms in Beijing and Shanghai is conducted. Then, to eliminate the regional heterogeneity of business development and economic growth and to remove selection bias due to Beijing and Shanghai being two economic centres in China, the PSM-DID method is applied in the second study to analyse correlations between the HSR and business costs for firms in all prefecture-level cities in China in order to generate patterns and mechanisms of business impacts of the HSR.
The remainder of this paper is organised as follows. Section 2 includes the literature review of the HSR system, business costs and current findings of correlations between them. Section 3 outlines the research design and main methodologies. Section 4 introduces data sources and variable specifications. Section 5 shows results of DID and PSM-DID estimations and Section 6 contributes to these results by providing robustness tests. Section 7 concludes the paper.
2. HIGH-SPEED RAIL AND BUSINESS COSTS
The HSR system can be linked to improved business efficiency and regional economic growth. Some macro-level studies have revealed that newly built HSR projects can have positive impacts on regional economic developments and better business environments. More specially, there are several mechanisms through which the HSR could have impacts on business and the economy. First, the HSR is believed to improve the business environment by creating the agglomeration effect. For instance, numerous papers adopt this mechanism and demonstrate how Japanese HSR lines, i.e., Shinkansen, improved business environments in Japan (Komikado et al., Citation2021), raised business profits by encouraging corporate innovations (Miwa et al., Citation2022) and lowered the unemployment rate by creating more jobs (Hiramatsu, Citation2018). Second, the HSR can efficiently reduce transportation costs, which is an important branch of variable costs, so that the business profits can be maximised. Chen et al. (Citation2016) claim that the Chinese economy and firms in multiple industries have benefited from the reduction of rail transportation costs, and firms in regions with HSR lines experienced a significant reduction of business costs due to lower transportation costs. Charnoz et al. (Citation2018) argue that the expansion of HSR lines in France led to decreasing communication costs for firms and higher specialisation of business operations.
However, relevant studies on impacts of the HSR on the business cost reduction in China are still far from adequate. Meanwhile, to the best of my knowledge, the majority of existing literature on analysing firm-level impacts of the HSR on business costs use survey data that account for a small proportion of the data of all firms in the sample regions, and few studies have adopted the panel data of all A-share listed firms in China to conduct research on correlations between the HSR and firm-level business costs for almost all firms in the country, which could become an area where this paper can make contributions. In accordance with large HSR networks and dynamic business environments, it might be essential to concentrate on firm-level analysis on impacts of the HSR on business costs for firms in China.
In China, the HSR lines as a significant means of infrastructure have been an important public agenda that is highly valued by the central and local governments (Lawrence et al., Citation2019). As noted by Sahoo et al. (Citation2010), rapid developments in public transportation in China such as the HSR have considerably contributed to increases in regional economic output and domestic interprovincial trade, which inspired the central and local governments to pay more attention to quantified firm-level impacts of new HSR lines. With regard to the macro-level benefits of the HSR in a wider background of railway acceleration in the past few decades in China, Zhou and Zheng (Citation2012) also found that rounds of railway acceleration, including the operation of several HSR lines in China, have significantly encouraged regional economic growth and higher business efficiency in Beijing, Shanghai and Canton in the past three decades. The eye-catching development of the HSR system in China has also encouraged papers that conduct firm-level analysis on its influences on firms. Liu and Hu (Citation2011) find that the HSR system helped to improve the efficiency of interprovincial trade in South China and narrowed the gap in corporate benefits between relatively wealthy regions and relatively poor regions. However, since most of the micro-level databases have not been published until recently, HSR-related firm-level research projects on this issue are still far from adequate in China.
Regarding how the HSR impacts business costs, relevant literature is far from sufficient while business costs are a significant measurement of business operations. Most of the current literature considers business costs as a branch of models for a broader analysis of impacts of the HSR on business developments while few papers discuss how business costs will respond to the opening of HSR lines specifically based on firm-level databases. Chi and Han (Citation2023) claim that the HSR has significant impacts on industrial business movements by reducing the costs of business operations. Wang et al. (Citation2014) analyse changes in travelling modes after the HSR was introduced in China and find that positive impacts of the HSR on the reduction of business costs are significant due to lower costs of business trips. The mechanism of how the HSR will have impacts on business costs might have been most straightforwardly illustrated by Ahlfeldt and Feddersen (Citation2018) who claim that the HSR can lead to agglomeration effects of labour and capital so that exchange activities of both factors will induce lower business costs than without the HSR. Business costs are a significant measurement which reveals the efficiency of business operations for individual firms (Jiang et al., Citation2006), and it is extremely valuable to analyse the potential impacts of the HSR on reducing business costs given the growing requirements for business cost reduction amid the current era of uncertainty.
In conclusion, how the HSR can have impacts on overall regional economic development and narrowing gaps in spatial economic growth has been thoroughly discussed from macro-level perspectives of economics. However, it should also be noted that multiple studies reveal that the HSR might cause wider regional economic gaps and discourage the development of local businesses, especially in left-behind regions, through multiple mechanisms such as the agglomeration effect which pulls labour and capital from these regions to economic centres (Kim & Sultana, Citation2015; Pagliara et al., Citation2022). As such, the author expects that impacts of the HSR on the reduction of business costs in China might demonstrate regional heterogeneity, i.e., firms in several regions might benefit from lower business costs due to the HSR while those in certain other regions might not. Meanwhile, it remains to be answered how it will affect business costs for firms among regions and cities with or without HSR lines in China, which requires firm-level information on this issue. Thus, it is necessary to design an empirical firm-level study on the potential causal effects of HSR on business costs for firms in China, and the first case will focus on business costs for firms in Beijing and Shanghai before and after 2008, the year of the first HSR line operation in China which connects Beijing and Tianjin.
There are three hypotheses that need to be specified in this paper.
HSR services contribute to the reduction of business costs for firms in China.
The operation of HSR lines has been proven to contribute to the reduction of business costs for firms through the mechanism of the agglomeration effects in many countries. Ahlfeldt and Feddersen (Citation2015) show that the HSR project connecting Frankfurt and Cologne in Germany has contributed to a higher economic agglomeration coefficient in cities along the line by 8 percent and reduction of business costs of local firms in these cities by 10 percent. Tian et al. (Citation2019) constructed the agglomeration coefficient for urban service sectors in China by combining the location factor of the service sector and the density of employment and service providers. They found that larger agglomeration coefficients are highly correlated with lower-level business costs of firms in urban service sectors in China. More specifically, the reduction of fixed and variable costs due to the HSR might be varied since the transportation costs were reduced due to the HSR belonging to variable costs while fixed costs can also be negatively impacted by the HSR due to lower labour costs (Yu et al., Citation2018).
(2) Such reduction has spatial heterogeneity patterns, indicating that business costs for firms in a more developed city might be reduced more than those in a less developed city even though both cities have HSR passing through.
New economic geography (NEG) focuses on the emergence of agglomeration effects which rely on increasing returns of scale and transportation costs (Krugman, Citation1991). Elements of the current literature have applied this theory to analysing gaps in the reduction of business costs driven by the agglomeration of business due to the operation of HSR lines among cities that benefit from the HSR. Vickerman (Citation2015) demonstrates that the reduction of business costs in mid-level and small cities is significantly less pronounced than that in major cities, although all of them benefit from HSR lines in Europe which argues that HSR lines failed to meet the primary objective of reducing regional disparities of corporate growth. Ke et al. (Citation2017) focus on the diversified impacts of the HSR on business developments among cities with HSR services in China by constructing hypothetical counterfactuals for cities in the absence of HSR services using outcomes in selected non-HSR cities and find that the reduction of business costs tends to be greater in cities with HSR services and higher-level industrialisation, greater ability of the service sector and better-supporting infrastructure.
(3) Such reduction also presents heterogeneity across firms with different ownerships. For example, private firms and those companies in service sectors might benefit more from the reduction of business costs.
A great deal of attention has been paid to gaps in business growth and costs between state-owned and private enterprises in China and how they respond to increases in productivity differently. Lin et al. (Citation2020) compare corporate structures and innovation-provoking schemes between state-owned and private enterprises in China and claim that private enterprises benefit more from innovations than state-owned enterprises due to more flexibility in absorbing new technologies and talents. Guo and Wu (Citation2020) demonstrate that the reduction of the cost of debt financing as an important category of business costs varies between state-owned and private firms in China when these firms are located in cities with HSR services. More specifically, they find that the increase in productivity encouraged by HSR services is vulnerable to the ownership of the firm and state-owned firms present a lower-level transition of such an increase in productivity in the form of cost reduction.
3. METHODOLOGY
3.1. DID analysis on firms in Shanghai and Beijing
See the Appendix in the supplemental data online.
3.2. PSM-DID analysis on firms in all prefecture-level cities in China
The author applies the PSM-DID method to examine whether results from the prior analysis are still feasible for firms in other prefecture-level cities of China to eliminate the sampling bias. The propensity score method (PSM) is a widely used quasi-experimental method that allows non-experimental or observational data to conduct interventional effect analysis on projects (Rosenbaum & Rubin, Citation1983) and can convert raw data into constructed data that follows the prerequisite of parallel trends embedded in the DID approach by matching each treated unit with a non-treated unit of similar characteristics. Reasons for choosing the PSM-DID method are the following. First, whether a city or a region is planned to have HSR lines is not randomly determined and this might lead to correlations between explanatory variables and residuals in the traditional DID model and thus endogeneity problems (Huang & Wang, Citation2020). Second, removing the self-selection bias entirely with the DID estimation strategy proves challenging due to the significant heterogeneity in business costs among firms in different cities. Economically developed central cities typically boast a superior business environment, resulting in lower business costs. Additionally, cities situated along the HSR routes are more likely to experience a substantial reduction in business costs compared to those not on HSR lines. Consequently, the initial disparity in business costs between the treatment group cities and the control group cities before the implementation of HSR could introduce self-selection bias. Last, but not least, the DID method meets the requirement of the panel data model based on a quasi-experimental setting since the operation dates of HSR lines in different cities in China are varied and several time-invariant variables such as the distance between two cities connected by the HSR need to be controlled.
According to Rosenbaum (Citation2023), the propensity score is the probability that a unit will enrol in a programme or be affected by a policy or a shock based on observed characteristics. Then, PSM matches treated units to untreated units based on the propensity score. The implementation of the PSM method in this study includes the following steps. First, it is essential to select several covariates such as GDP per capita and the population density to define the similarity between city individuals. A logit model of binary choice was established to estimate the similarity. Second, linearised propensity scores are calculated to define similarity between different cities to ensure the consistency between propensity score similarity and covariate similarity. Finally, results of the PSM process can provide the effectiveness of the matching, as shown in .
Figure 1. Bar chart of propensity scores.
Note: The propensity score represents the probability that each city individual will be affected by the HSR, i.e., the probability of being selected to the treatment group. The vertical axis represents the nuclear density. On support means that samples are successfully matched after the PSM method, i.e., propensity scores of both treatment and control groups are in the range of common support, and off support means that samples are not successfully matched after the PSM method, indicating data of these samples ought to be omitted (Abadie & Imbens, Citation2016).
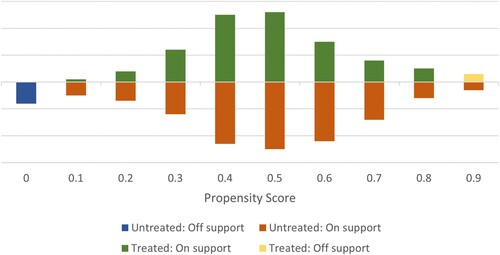
Thus, the experimental group consists of firms in prefecture-level cities with HSR services and the control group includes firms in other prefecture-level cities without HSR services. After the PSM process that completes the transformation of datasets, it is possible to estimate the expected impacts of HSR services on a reduction of business costs in major cities in China. Instead of considering firm-level control variables in the previous DID analysis, the PSM-DID analysis chooses macroeconomic variables of cities where firms are located as control variables in order to specifically control for regional heterogeneity. The panel data model is constructed as follows:
(1)
(1) In this model,
represents the
th firm in a designated prefecture-level city and
represents time.
is a dummy variable which equals 0 if the city where the
th firm is located did not have HSR services at year
and equals 1 if it had HSR services at year
.
is another dummy variable which equals to 0 when the year of the data of business costs is before the year of starting HSR operation in the city where the firm is located and equals to 1 when the year of the data is after the year of starting HSR operation.
is the core independent variable and
is the coefficient that measures impacts of HSR services on the reduction of business costs.
represents all control variables with the number of
. shows definitions of all variables in Equation (1).
Table 1. Variable definitions of PSM-DID analysis.
It is important to note that, regarding the year of starting the operation of HSR services, some HSR lines started the service in the second half of the year. For example, the HSR line between Guangzhou and Wuhan began to operate on 26th December 2009. According to Nash (Citation2015) and Chen (Citation2019), impacts of HSR services on the business operations of local firms and regional economic growth will have lagged due to the process of transforming improved efficiency of public transportation into economic impacts. Thus, in following empirical analysis, for all cities that had HSR services during the second half of the year (e.g., December 2009) the year of starting HSR operation will have lagged by one year (e.g., 2010).
The control variable set originates from previous macro-level models of analysing correlations between public transportation and business costs (Litman, Citation2017), which includes the GDP per capita of a city (to measure economic growth), the proportion of the secondary industry in the regional economy of a city (to measure the level of industrialisation), the proportion of output created by FDI in the aggregate output of the city (to measure investment contribution), the Internet penetration rate of the city (to measure the level of digital technology) and the ratio of population to the total area of the city (the population density). All of these variables will have impacts on decisions regarding the location of business operation for firms.
4. DATA
There is a two-step data identification for this study. First, to conduct the DID analysis on impacts of HSR services on business costs of firms in Beijing and Shanghai, the firm-level panel data of business costs for firms in both cities is utilised. Second, to measure how HSR lines affect business costs of firms in different cities with HSR services, the firm-level panel data of firms in all prefecture-level cities in China is adopted. Regarding the dependent variable, the firm-level panel data of business costs comes from the China Industrial Business Database, which has been completed yearly by Peking University since 1998 and includes almost all business-related survey data of variables for each firm in China’s prefecture-level cities. Considering the adequacy of the sample data, the author selected the sample period to be from 2004 to 2012 with two critical time points, namely 2008 when Beijing started to have HSR services and 2010 when Shanghai connected the HSR network.
Data of control variables for the Beijing-Shanghai DID analysis is from the China Industrial Business Database, China Statistical Yearbook (2004–2012)Footnote2 and China City Statistical Yearbook (2004–2012).Footnote3 Data of control variables for the PSM-DID analysis comes from the same databases but during the period from 2012 to 2020. To further reduce statistical bias, data of firms in several cities such as Chaohu City and Sansha City is deleted due to changes in administrative areas of these cities during this period. Note that firms selected for this study are all A-share listed firms in China due to the availability of data. The final sample contains 4588 firms with 36,704 firm-year observations for the section of analysing firms in all prefecture-level cities in China and 768 firms with 6144 firm-year observations for the section of analysing firms in Beijing and Shanghai.
5. EMPIRICAL RESULTS
5.1. DID analysis on firms in Shanghai and Beijing
See the Appendix in the supplemental data online.
5.2. PSM-DID analysis on firms in all prefecture-level cities in China
Beijing and Shanghai are two economic centres in China with higher densities of firms and better-than-average business environments compared to other cities, which might lead to lower business costs than average and bring sample selection bias to this study. To eliminate such bias, the PSM-DID analysis is performed on the data of business costs of all A-shared listed firms in all prefecture-level cities of China.
The PSM requires a balance test on the samples of the control group and the intervention group to detect and eliminate sample selection bias (Zang et al., Citation2020). The ideal statistical features after the balance test include the reduction of the difference between two groups, the absolute value of the deviation of the sample data on each covariate not exceeding 20 and the p-value with regard to the t-statistic higher than 0.05. shows the result of the balance test, and it is apparent that all requirements are met in accordance with ideal statistical features, which indicates that there is no significant sample selection bias after the test. The histogram of propensity scores is shown in , which indicates that the distribution of propensity scores of control variables in the treatment group approximates an ideal normal distribution in a symmetrical form. The horizontal axis represents propensity scores and the vertical axis represents the nuclear density which shows the probability density on different levels of propensity scores. The probability and density of the post-matching propensity scores of the treatment and control groups are close, so the control variables used in this study are appropriate. Thus, the PSM matching improves the comparability of the impacts of the HSR on business costs for firms in cities with and without HSR lines.
Table 2. Balance test results of PSM.
The results of DID regression based on the PSM are shown in . Both fixed and variable costs for firms in all sectors are estimated to be reduced due to HSR services since the coefficients of HSR (cross-sectional dummy) are all significantly negative. This finding is slightly different from several studies on the impacts of HSR services on the business developments of firms in China by showing that HSR lines could contribute to the reduction of business costs regardless of industries or sectors. For firms in the secondary and tertiary sectors, impacts of the HSR on the reduction of business costs are inclined to be significantly negative, while such impacts are not significant for firms in the agricultural sector (Guo et al., Citation2021; Salzberg et al., Citation2013). By providing the estimation of such impacts on categorised business costs based on extremely large datasets, this study could hopefully provide additional insights into this issue.
Table 3. Impacts of HSR on fixed and variable costs.
According to data from the China Statistical Yearbook (2012–2020), gaps in the GDP per capita across eastern, central, and western China are significantly large. To further test whether the heterogeneity of spatial economic growth in China contributes to varied impacts of the HSR on the reduction of business costs, the same regression process is repeated three times and shows the regression results that exclude coefficients of all control variables to focus more on impacts of the HSR. Note that data from central and western China are combined due to too few HSR lines during the sampling period. Columns (1), (2), and (3) summarise the results for firms in the agricultural, secondary and tertiary sectors, respectively, with regard to fixed costs in eastern China. Columns (4), (5) and (6) summarise the results for all sectors with regard to variable costs in eastern China. Columns (7), (8) and (9) show the results for all sectors with regard to fixed costs in central and western China and the remaining three columns show the results for all sectors with regard to variable costs in central and western China.
Table 4. Test of spatial heterogeneity.
It is interesting to note the positive impacts of HSR services on the reduction of both fixed and variable costs are significantly imposed on firms in all sectors in eastern China while such impacts are not significant for firms in central and western China. For example, firms in the agricultural sector received no benefits of fixed and variable cost reductions from the HSR in central and western China, and manufacturing firms in this region also did not witness any significant impacts of HSR services on the reduction of fixed costs. Several reasons can explain this finding. First, the relatively lower level of economic growth in central and western China becomes a push factor for firms and the HSR might exacerbate this problem (Fleisher et al., Citation2010). Second, during the sampling period from 2012 to 2020, the total number of HSR lines in central and western China is still much smaller than that in eastern China, although waves of HSR construction started during this period. The impacts of HSR services on business costs might not be reflected in this study due to the lagging economic impacts of the infrastructure. Third, it is also important to note that the substitution effect of HSR services might be mild in western China since the distances between economic centres in western and eastern China can be so long that flights might be superior to the HSR for business purposes.
6. ROBUSTNESS TEST
See the Appendix in the supplemental data online.
7. CONCLUSIONS
By 2021, the total operating high-speed rail network reached 40,000 kilometres in China, making this extraordinary network over three times larger than the HSR system in the EU (Sun et al., Citation2022). This study could give initial inspiration about how the HSR system will have influences on business operations for firms in China with a concentration on business costs, an important aspect in the field of corporate management. To the best of the author’s knowledge, this study is the first firm-level DID-based research on such impacts given the background of rapid development of the HSR network in China in the past decade.
This study has three main findings corresponding to three hypotheses. First, HSR services have significant positive effects on the reduction of business costs for most firms in China if they are located in cities with HSR lines. Second, such impacts have spatial patterns based on regional heterogeneity, indicating that business costs for firms in a more developed region might be reduced more than those in a less developed region even though both regions have HSR lines. Third, such impacts also vary across firms with different features, namely the ownership and the industry. For example, firms in manufacturing and service sectors are likely to benefit more from business cost reductions from HSR services than agricultural firms.
Several policy implications can be drawn from these findings. First, due to the insignificant impacts of the HSR on the reduction in business costs in central and western China, where economic growth is significantly lower than the more developed eastern part of the country, these two parts, along with other remote and undeveloped regions, ought to more effectively receive positive stimulation from the reduction in business costs driven by the HSR in the future. Reasons for insignificant impacts of HSR services on business costs for firms in these regions revealed in this study might be the lack of agglomeration of firms and the lagged effects on business operations. Further analysis on such impacts for firms in central and western China is required and might demonstrate significant impacts in the future. Results of the PSM-DID analysis in this study also, interestingly, reveal that more FDI is significantly correlated with lower business costs, indicating that a transparent and business-oriented local administration could boost the impacts of the HSR on the reduction of business costs. For local governments in central and western China, the openness and transparency of local administrations are still extremely absent. China is currently conducting political reforms of transforming dictatorship-oriented governments to service-oriented governments for healthier business developments in remote provinces, which might stimulate the business cost reductions due to the HSR in undeveloped areas.
Second, the central government as the decision maker of HSR lines in China needs to focus more on the input-output ratio of new HSR lines, given that local and national debts in China have reached greater limits since 2022. Empirical results also indicate that several prefecture-level cities, such as Kunming and Guiyang, barely benefited from the HSR with regard to the reduction of business costs while these cities were recently reported to experience a debt default in the near future. The HSR construction relies on partial funds from local governments, which becomes an alert for the central government.
Third, the mechanism of having impacts of HSR services on business costs requires that barriers to the improvement of corporate management and business operation should be removed. The HSR network accelerates the flow of business factors such as capital and labour so that local firms can benefit from the improvement of these factors. However, it will be impossible for firms to benefit from such improvements if the city where firms are located has too many obstacles, such as high administrative costs, lack of business transparency and market disorders, or if firms suffer from low business operation efficiency and depressed innovations due to the ownership (Liang et al., Citation2022; Murakami & Cervero, Citation2017). This study reveals that the reduction in business costs in state-owned enterprises seem to be less significantly boosted by the HSR than privately-owned firms, which echoes numerous studies claiming low efficiency of state-owned firms in China (Lin et al., Citation2020; Stone et al., Citation2022). For the time being, the best strategy for firms is to locate their sites in regions not only with convenient public transport (such as the HSR) but also with acceptable business environments, such as investment-friendly administrative policies.
Finally, this study can motivate further research in the following aspects. The negative correlations between FDI and business costs require detailed analysis, such as the examination of whether the HSR accelerates the original impacts of more FDI on lower business costs or the overall business environment attracts both more FDI and lower business costs despite the HSR even though FDI is considered as a control variable in this study. Meanwhile, the regional heterogeneity of impacts of the HSR on the reduction of business costs that is found in this study needs to be further specified. For example, it is worthwhile to note that western China has much more complicated landscapes, which technically makes it more difficult to construct HSR lines than in central and eastern China. Last, but not least, the assumption at the very beginning of this paper, which is the top-down HSR decision-making process, might be more thoroughly discussed since several local administrators might have closer relationships with members of the standing committee of CPC, which increases the possibility of HSR construction in regions where these administrators govern. It is an extraordinarily complex issue that involves political competition and conflicts among local governments in China, which requires more effort on the perspectives of Chinese politics.
Supplemental Material
Download PDF (375.7 KB)DISCLOSURE STATEMENT
No potential conflict of interest was reported by the author(s).
Additional information
Funding
Notes
1 A-shares are shares of companies based in mainland China that are listed on either the Shanghai or Shenzhen stock exchanges (see the official website of China Securities Regulatory Commission: http://www.csrc.gov.cn/).
2 See the official website of National Bureau of Statistics of China: http://www.stats.gov.cn/sj/ndsj/ (Chinese version).
3 See the official website of China City Statistical Yearbooks: http://www.shujuku.org/china-city-statistical-yearbook.html (Chinese version).
4 It is important to note some difficulties in respect of the year of HSR starting operations. Some HSR lines started services in the second half of a calendar year. For example, the HSR line between Guangzhou and Wuhan began to operate on 26th December 2009. According to Nash (Citation2015) and Chen (Citation2019) the impacts of HSR services on business operations of local firms and regional economic growth will need a lag structure due to the process of transforming improved efficiency of public transportation into economic impacts. Then in the following empirical analysis, for all cities that had HSR services during the second half of the year (e.g., December 2009) the year of starting HSR operation lagged by one year.
5 http://www.stats.gov.cn/sj/ndsj/(Chinese version).
6 http://www.shujuku.org/china-city-statistical-yearbook.html (Chinese version).
REFERENCES
- Abadie, A., & Imbens, G. W. (2016). Matching on the estimated propensity score. Econometrica, 84(2), 781–807. https://doi.org/10.3982/ECTA11293
- Ahlfeldt, G. M., & Feddersen, A. (2015). From periphery to core: Economic adjustments to high-speed rail. SERC Discussion Papers (Vol. 172). London.
- Ahlfeldt, G. M., & Feddersen, A. (2018). From periphery to core: Measuring agglomeration effects using high-speed rail. Journal of Economic Geography, 18(2), 355–390. https://doi.org/10.1093/jeg/lbx005
- Anderson, B., Turan, M., & Thompson, D. (2008). Public transportation: A revolution on business cooperation. Journal of Logistics and Business, 2(3), 12–25.
- Blum, U., Haynes, K. E., & Karlsson, C. (1997). Introduction to the special issue: The regional and urban effects of high-speed trains. Annals of Regional Science, 31(1), 1–20. https://doi.org/10.1007/s001680050036
- Briciu, S. (2008). Variable and fixed costs in company management. Annales Universitatis Apulensis Series Oeconomica, 1(10), 1–14. https://doi.org/10.29302/oeconomica.2008.10.1.14
- Charnoz, P., Lelarge, C., & Trevien, C. (2018). Communication costs and the internal organisation of multi-plant businesses: Evidence from the impact of the French high-speed rail. The Economic Journal, 128(610), 949–994. https://doi.org/10.1111/ecoj.12592
- Chen, Z. (2019). Measuring the regional economic impacts of high-speed rail using a dynamic SCGE model: The case of China. European Planning Studies, 27(3), 483–512. https://doi.org/10.1080/09654313.2018.1562655
- Chen, Z., Xue, J., Rose, A. Z., & Haynes, K. E. (2016). The impact of high-speed rail investment on economic and environmental change in China: A dynamic CGE analysis. Transportation Research Part A: Policy and Practice, 92, 232–245. https://doi.org/10.1016/j.tra.2016.08.006
- Chi, F., & Han, H. (2023). The impact of high-speed rail on economic development: A county-level analysis. Land, 12(4), 874–898. https://doi.org/10.3390/land12040874
- Fama, E. F., & French, K. R. (1999). The business cost of capital and the return on business investment. The Journal of Finance, 54(6), 1939–1967. https://doi.org/10.1111/0022-1082.00178
- Fleisher, B., Li, H., & Zhao, M. Q. (2010). Human capital, economic growth, and regional inequality in China. Journal of Development Economics, 92(2), 215–231. https://doi.org/10.1016/j.jdeveco.2009.01.010
- Guo, H., & Wu, K. (2020). Does opening high-speed railways affect the cost of debt financing? A quasi-natural experiment. China Finance Review International, 10(4), 473–496. https://doi.org/10.1108/CFRI-06-2019-0083
- Guo, Z., Chan, K. C., & Huang, J. (2021). The impact of executive diversity on corporate innovation: Evidence from the natural experiment of high-speed rail in China. Managerial and Decision Economics, 42(1), 219–234. https://doi.org/10.1002/mde.3227
- Heuermann, D. F., & Schmieder, J. F. (2019). The effect of infrastructure on worker mobility: Evidence from high-speed rail expansion in Germany. Journal of Economic Geography, 19(2), 335–372. https://doi.org/10.1093/jeg/lby019
- Hiramatsu, T. (2018). Job and population location choices and economic scale as effects of high speed rail: Simulation analysis of Shinkansen in Kyushu, Japan. Research in Transportation Economics, 72, 15–26. https://doi.org/10.1016/j.retrec.2018.06.007
- Huang, Y., & Wang, Y. (2020). How does high-speed railway affect green innovation efficiency? A perspective of innovation factor mobility. Journal of Cleaner Production, 265, 121623. https://doi.org/10.1016/j.jclepro.2020.121623
- Jiang, B., Frazier, G. V., & Prater, E. L. (2006). Outsourcing effects on firms’ operational performance: An empirical study. International Journal of Operations & Production Management, 26(12), 1280–1300. https://doi.org/10.1108/01443570610710551
- Ke, X., Chen, H., Hong, Y., & Hsiao, C. (2017). Do China’s high-speed-rail projects promote local economy? – New evidence from a panel data approach. China Economic Review, 44, 203–226. https://doi.org/10.1016/j.chieco.2017.02.008
- Kim, H., & Sultana, S. (2015). The impacts of high-speed rail extensions on accessibility and spatial equity changes in South Korea from 2004 to 2018. Journal of Transport Geography, 45, 48–61. https://doi.org/10.1016/j.jtrangeo.2015.04.007
- Komikado, H., Morikawa, S., Bhatt, A., & Kato, H. (2021). High-speed rail, inter-regional accessibility, and regional innovation: Evidence from Japan. Technological Forecasting and Social Change, 167, 120697. https://doi.org/10.1016/j.techfore.2021.120697
- Krugman, P. (1991). Increasing returns and economic geography. Journal of Political Economy, 99(3), 483–499. https://doi.org/10.1086/261763
- Lawrence, M., Bullock, R., & Liu, Z. (2019). China’s high-speed rail development. World Bank Publications.
- Li, C., & Wang, X. (2013). The economic effects of Beijing-Shanghai HSR on mutual trades. China Railway and Economics Studies, 10(2), 23–42.
- Liang, P., Cui, X., Lin, M., Yang, T., & Wu, B. (2022). High-speed rail effects on station area-level business commercial agglomeration: Evidence from 110 stations in China. Frontiers in Environmental Science, 10, 2334. https://doi.org/10.3389/fenvs.2022.1045959
- Lin, K. J., Lu, X., Zhang, J., & Zheng, Y. (2020). State-owned enterprises in China: A review of 40 years of research and practice. China Journal of Accounting Research, 13(1), 31–55. https://doi.org/10.1016/j.cjar.2019.12.001
- Litman, T. (2017). Evaluating transportation economic development impacts. Victoria Transport Policy Institute.
- Liu, L., & Zhang, M. (2018). High-speed rail impacts on travel times, accessibility, and economic productivity: A benchmarking analysis in city-cluster regions of China. Journal of Transport Geography, 73, 25–40. https://doi.org/10.1016/j.jtrangeo.2018.09.013
- Liu, S., & Hu, A. G. (2011). Public transportation and China’s regional economic cooperation. Journal of Economic Research (China), 3(2), 68–90.
- Miwa, N., Bhatt, A., Morikawa, S., & Kato, H. (2022). High-Speed rail and the knowledge economy: Evidence from Japan. Transportation Research Part A: Policy and Practice, 159, 398–416. https://doi.org/10.1016/j.tra.2022.01.019
- Murakami, J., & Cervero, R. (2017). High-speed rail and economic development: Business agglomerations and policy implications. In B. L. P. Henríquez & E. Deakin (Eds.), High-Speed rail and sustainability (pp. 244–271). Routledge.
- Nash, C. (2015). When to invest in high speed rail. Journal of Rail Transport Planning & Management, 5(1), 12–22. https://doi.org/10.1016/j.jrtpm.2015.02.001
- Niu, D., Sun, W., & Zheng, S. (2020). Travel costs, trade, and market segmentation: Evidence from China’s high-speed railway. Papers in Regional Science, 99(6), 1799–1825. https://doi.org/10.1111/pirs.12557
- Pagliara, F., Henke, I., Russo, L., & Guigon, M. (2022). Is high speed rail a geographically inclusive system? Evidence from some European countries. Applied Spatial Analysis and Policy, 1–23. https://doi.org/10.1007/s12061-021-09399-y
- Park, H. K., Han, S. H., & Russell, J. S. (2005). Cash flow forecasting model for general contractors using moving weights of cost categories. Journal of Management in Engineering, 21(4), 164–172. https://doi.org/10.1061/(ASCE)0742-597X(2005)21:4(164)
- Pyrgidis, C. N. (2016). Railway transportation systems: Design, construction and operation. CRC press.
- Roberts, R. R., Frutos, P. W., Ciavarella, G. G., Gussow, L. M., Mensah, E. K., Kampe, L. M., … Rydman, R. J. (1999). Distribution of variable vs fixed costs of hospital care. Jama, 281(7), 644–649. https://doi.org/10.1001/jama.281.7.644
- Rosenbaum, P. R. (2023). Propensity score. In J. Zubizarreta, E. Stuart, D. Small, & P. Rosenbaum (Eds.), Handbook of matching and weighting adjustments for causal inference (pp. 21–38). Chapman and Hall/CRC.
- Rosenbaum, P. R., & Rubin, D. B. (1983). The central role of the propensity score in observational studies for causal effects. Biometrika, 70(1), 41–55. https://doi.org/10.1093/biomet/70.1.41
- Sahoo, P., Dash, R. K., & Nataraj, G. (2010). Infrastructure development and economic growth in China. Institute of Developing Economies Discussion Paper, 261(2), 1–33.
- Salzberg, A., Bullock, R., Ying, J., & Fang, W. (2013). High-speed rail, regional economics, and urban development in China. China Transport Topics; No. 8 World Bank, Beijing.
- Stone, R. W., Wang, Y., & Yu, S. (2022). Chinese power and the state-owned enterprise. International Organization, 76(1), 229–250. https://doi.org/10.1017/S0020818321000308
- Sun, X., Yan, S., Liu, T., & Wang, J. (2023). The impact of high-speed rail on urban economy: Synergy with urban agglomeration policy. Transport Policy, 130, 141–154. https://doi.org/10.1016/j.tranpol.2022.11.004
- Sun, Y., Razzaq, A., Kizys, R., & Bao, Q. (2022). High-speed rail and urban green productivity: The mediating role of climatic conditions in China. Technological Forecasting and Social Change, 185, 122055. https://doi.org/10.1016/j.techfore.2022.122055
- Thomas, C. (1998). Costs and benefits: An empirical revisit on business dilemma. American Economic Review, 12(6), 127–135.
- Tian, M., Li, T., Yang, S., Wang, Y., & Fu, S. (2019). The impact of high-speed rail on the service-sector agglomeration in China. Sustainability, 11(7), 2128. https://doi.org/10.3390/su11072128
- Vickerman, R. (2015). High-speed rail and regional development: The case of intermediate stations. Journal of Transport Geography, 42, 157–165. https://doi.org/10.1016/j.jtrangeo.2014.06.008
- Wang, Y., Li, L., Wang, L., Moore, A., Staley, S., & Li, Z. (2014). Modeling traveller mode choice behavior of a new high-speed rail corridor in China. Transportation Planning and Technology, 37(5), 466–483. https://doi.org/10.1080/03081060.2014.912420
- Winters, L. A., & Martins, P. M. (2004). When comparative advantage is not enough: Business costs in small remote economies. World Trade Review, 3(3), 347–383. https://doi.org/10.1017/S1474745604001922
- Wu, Y., Lee, C. C., Lee, C. C., & Peng, D. (2022). Geographic proximity and business investment efficiency: Evidence from high-speed rail construction in China. Journal of Banking & Finance, 140, 106510. https://doi.org/10.1016/j.jbankfin.2022.106510
- Yilmaz, V., & Ari, E. (2017). The effects of service quality, image, and customer satisfaction on customer complaints and loyalty in high-speed rail service in Turkey: A proposal of the structural equation model. Transportmetrica A: Transport Science, 13(1), 67–90. https://doi.org/10.1080/23249935.2016.1209255
- Yu, X., Lang, M., Gao, Y., Wang, K., Su, C. H., Tsai, S. B., … Li, S. (2018). An empirical study on the design of China high-speed rail express train operation plan – From a sustainable transport perspective. Sustainability, 10(7), 2478. https://doi.org/10.3390/su10072478
- Zang, J., Wan, L., Li, Z., Wang, C., & Wang, S. (2020). Does emission trading scheme have spillover effect on industrial structure upgrading? Evidence from the EU based on a PSM-DID approach. Environmental Science and Pollution Research, 27(11), 12345–12357. https://doi.org/10.1007/s11356-020-07818-0
- Zhang, C., & Jefferson, T. (2020). High-Speed railways’ spill-over effects: How can high-speed railways reshape the business landscape? Journal of Transportation and Economics, 23(3), 156–178. (Chinese version).
- Zhou, H., & Zheng, X. (2012). The quality of public transportation systems and economic growths: Evidence from China railway acceleration. Journal of Global Economics, 12(3), 75–94. (Chinese version).