ABSTRACT
We assess the consequences of sustainable farming practices (“Best Management Practices”) on oil palm fruit production on 40 smallholder farms in Sabah, Malaysian Borneo, and whether maintaining vegetation cover, which is linked to environmental sustainability and higher biodiversity, results in a trade-off with oil palm production. We found considerable variation in yield among farms (6.9–37.4 t ha−1 yr−1), but little evidence for any trade-offs between yield and farming practices. This finding has important implications for livelihoods if farmers can minimize agricultural inputs, reducing costs and supporting the environmental sustainability of oil palm smallholder farms whilst maintaining crop yields.
Graphical Abstract

Introduction
Since the beginning of the 21st century, agricultural cultivation has been the main driver of forest conversion in Southeast Asia (Zeng et al. Citation2018). For example, in Malaysian Borneo, the oil palm industry has been a major contributor to deforestation and land-use change in the past four decades, mainly driven by the expansion of industrial plantations and smallholder farms (Gaveau et al. Citation2018). This agricultural expansion has resulted in widespread species declines across most taxa (Edwards et al. Citation2014; Kwatrina et al. Citation2018; Savilaakso et al. Citation2014). However, with careful management, agricultural landscapes could have the potential to conserve biodiversity and valuable ecosystem services (Tscharntke et al. Citation2005), thereby helping to ensure the environmental sustainability of oil palm landscapes, whilst also supporting high yields and farmer livelihoods.
Smallholder producers (<50 hectares; RSPO 2021) are estimated to contribute up to 33% of global palm oil production (Descals et al. Citation2020), but the average smallholder yield reported by the Roundtable on Sustainable Palm Oil (RSPO) is only 18.9 tonnes of fresh fruit bunches (FFB; ha−1 yr−1), which is almost half of the estimated potential average yield for smallholders at 33.2 t ha−1 yr−1 (Euler et al. Citation2016), and half of the estimated potential average yield for large commercial plantations (35–40 t ha−1 yr−1; Hoffmann et al. Citation2015). Studies of smallholder agriculture have been increasing (Euler et al. Citation2016; Kurniawan et al. Citation2018; Rhebergen et al. Citation2020), and the capacity to boost yields on current smallholdings has been highlighted as a potential solution to sustainably intensify palm oil production and increase farmer incomes (Hoffmann et al. Citation2017; Varkkey, Tyson, and Choiruzzad Citation2018). This intensification may also reduce further conversion of natural habitat to agriculture, conserving biodiversity (Edwards et al. Citation2010; Lee et al. Citation2014). Variations in yield can occur due to climate (Oettli, Behera, and Yamagata Citation2018), soil characteristics and topography (Corley and Tinker Citation2016), and agricultural management practices (Euler et al. Citation2016; Hoffmann et al. Citation2017; Woittiez et al. Citation2017), which may explain the wide variations in smallholder yields that have been reported. The effects of certain management practices on FFB yields have been well studied, such as optimal palm planting density (Bonneau, Impens, and Buabeng Citation2018; Corley Citation1973), fertilizer inputs (Tao et al. Citation2017; Tengoua et al. Citation2015), frond pruning (Marcelino and Diaz Citation2016; Soliman et al. Citation2016), FFB harvesting frequency (Lee et al. Citation2014; Rhebergen et al. Citation2020), and weeding and ground cover management (Samedani et al. Citation2014; Soliman et al. Citation2016). However, the overall impact of farm management intensity on smallholder oil palm yields remains unclear.
There is a need to identify management practices that result in win–win scenarios for yield and environmental sustainability (Fischer et al. Citation2017; Tamburini et al. Citation2020). For example, ‘Best Management Practices’ (BMPs) are recommended by the RSPO as a way to sustainably intensify palm oil production by improving soil fertility and preventing soil degradation (RSPO P&C Citation2018). These BMPs include practices that minimize the use of inorganic pesticides and fertilizers (Sundram, Angel, and Sirajuddin Citation2019; Yi et al. Citation2019), techniques that reduce soil erosion and nutrient leaching by managing ground cover vegetation (Darras et al. Citation2019; Formaglio et al. Citation2020), and maintaining soil organic matter through the application of crop residues such as Empty Fruit Bunches (EFBs) and palm oil mill effluent (Tao et al. Citation2018b; Yi et al. Citation2019). The uptake of BMPs substantially increases FFB yields on some smallholdings (Pauli et al. Citation2014; Rahman et al. Citation2021; Rhebergen et al. Citation2020), as well as replenishing soil organic carbon (SOC) and nitrogen content (Rahman et al. Citation2021; Tao et al. Citation2016), but BMPs can be labor intensive. Hence, it is important to assess whether application of BMPs affects yield and soil quality, and whether BMPs offer more environmentally sustainable management options.
Supporting greater structural complexity of ground vegetation could maintain soil fertility, a crucial factor for healthy palm growth and fruit production (Corley and Tinker Citation2016), and also reduce soil erosion and run-off (García-Orenes et al. Citation2012; Sahat et al. Citation2016; Zheng Citation2006). Understory vegetation could also support biodiversity that improves the functioning of soil processes (Barnes et al. Citation2014). Thus, increasing understory vegetation cover could improve yields, as well as improving the environmental sustainability of farms. However, high-intensity management practices such as high herbicide inputs suppress understory vegetation (Luke et al. Citation2019), with consequent negative effects on soil fauna (Ashton-Butt et al. Citation2018; Darras et al. Citation2019), which contributes to the degradation of SOC, a valuable indicator of soil fertility (Guillaume et al. Citation2016a). These findings imply trade-offs between management practices to support ground vegetation cover and soil fertility, versus management to increase yields. Hence, improved environmental sustainability might incur costs to farmers if it reduces yields, and so it is important to examine relationships between yield, management practices, and understory vegetation cover on farms.
In this study, we examined variation in yield on smallholder oil palm farms (Fig S1), and the role of management intensity, understory vegetation cover, climate, and soil characteristics (soil type, SOC, total N, total P, and available P) on yields. We used responses from face-to-face questionnaires of 40 smallholder farmers located across six governance zones in Sabah, Malaysian Borneo, to collect information about their management practices, including BMPs, and reported FFB yields. We also carried out field surveys on these farms to quantify understory vegetation cover and soil characteristics (Fig S2). We used this information to examine variation in reported FFB yields among farms, how yields varied in relation to management intensity and use of BMPs, and the effects of management intensity on understory vegetation cover and soil chemical properties. We investigated whether there are trade-offs between increasing yields versus enhancing environmental sustainability on farms through increased understory vegetation cover and improved soil chemical properties.
Materials and methods
Study sites
We collected data between August and November 2019 from 40 smallholdings (defined as farms <50 ha) across six governance areas in Sabah, Malaysia (Fig S2a-c) of which 35 smallholders were part of a Smallholder Group Scheme organized by a local social NGO (WildAsia), and the remaining five were independent smallholders. In our analyses, we allocated smallholders to governance areas (n = 6) according to the village community the smallholder belonged to, on the assumption that smallholders within the same governance area would manage their farms in a similar way by following the guidance of the local community leader and WildAsia staff. Governance areas differed slightly in maximum temperature (°C) and minimum rainfall (mm month−1), as well as soil type (Table S1). Farms had been established between 8 and 27 years ago, ranged between 0.81 and 7.73 ha in size, and had either been established after conversion from commercial selectively logged forest (n = 18 farms) or from agriculture other than palm oil (such as coffee, rice, vegetables, or fruit crops; 22 farms) (Table S1). Smallholders were either oil palm monocultures (n = 19 farms) or mixed agriculture systems that included other crops (n = 21 farms), although non-palm tree crops grown on mixed agriculture farms were at very low densities because farmers were not relying on non-palm trees for income. Planting densities of palms were the same on monoculture farms (median = 136 trees ha−1, n = 19) and mixed farms (median = 136 trees ha−1, n = 21). Smallholder selection criteria for inclusion within our study were (i) crop age (to minimize variation in yields as a result of crop age, trees had to be mature fruiting trees, that is, >8 years) (Hoffmann et al. Citation2017), and (ii) farm size (to be large enough to conduct ecological surveys using our standardized plot design, that is, >0.5 ha). When farms had mature oil palm trees of multiple ages >8 years old, we computed the average age of the oil palm crop according to the proportion of the farm area planted with each age group.
Smallholder questionnaires to assess management intensity and fruit bunch yields
Information about farm management practices was collected from interviews with smallholder farmers, following a standardized questionnaire of 29 questions, including questions on farm history and physical characteristics, palm fruit harvesting practices, and management inputs (for questions see SI3). The questionnaire was developed based on information about practices known to influence oil palm yields (Corley and Tinker Citation2016; Woittiez et al. Citation2017). Questionnaires with farmers were conducted in Bahasa Malay by ABS, SGA, and a translator from WildAsia.
We developed an index of management intensity by synthesizing the information on management practices that we collected from the interviews (applying multiple imputation methods for a very small number of incomplete responses; see SI4 for details). From the responses to the questionnaires, we derived nine management parameters per farm, and graded each parameter a binary score for intensity of either 0 (less intensive) or 1 (more intensive) (). Each management practice was graded as more intensive if it required more production inputs, either in labor or agricultural inputs. For example, there were two questions about fertilizer application: a score of 1 was assigned if the smallholder used fertilizers and 0 if they did not, and for those farms applying fertilizer, an input score of 0 if fertilizer inputs were below the median input level across farms (ha−1 yr−1) and a score of 1 if inputs were above the median value. To calculate the overall management intensity score for each farm, we computed the management intensity index (with a value between 0–1) by summing the intensity scores for all nine parameters and dividing by 9. To obtain a measure of yield for each farm, we used the reported monthly Fresh Fruit Bunch (FFB) harvest by each farmer or calculated yield from the reported monthly income and price per tonne of FFB issued by the mill. Depending on the information provided by farmers, data on reported average yields were either the most recently obtained single month or harvest yield, or an average yield per month or per harvest. We report the monthly FFB yield as annual yield in tonnes per hectare per year (t ha−1 yr−1) for comparison with published information, as well as yield in kilograms per tree per year (kg tree−1 yr−1) to account for variation in planting densities on farms.
Table 1. Description of the management practices carried out by smallholder farmers, and how information on management practices was used to produce a management intensity index score for each farm. Information about the implementation of these management practices was collected from interviews with the smallholder farmers (n = 40 farms; see SI for details of questionnaires).
Identifying RSPO best management practices
From the farmer questionnaires, we extracted information on the application of ‘Best Management Practices’ as outlined by the Roundtable on Sustainable Palm Oil (RSPO). The RSPO lists in its Principles and Criteria (P&C) number 7.4 (RSPO P&C Citation2018) that ‘long-term fertility depends on maintaining the structure, organic matter content, nutrient status and microbiological health of the soil’ and P&C 7.5 that ‘techniques that minimise soil erosion are well known and should be adopted, where appropriate. These should include practices such as ground cover management, biomass recycling, terracing, and natural regeneration or restoration instead of replanting.’ We concluded that RSPO ‘Best Management Practices’ taken up by farmers in our study related primarily to nutrient recycling and ground cover management, including (i) application of crop residues, (ii) minimizing use of herbicides, and (iii) retaining areas of ground vegetation cover, and (iv) clearing a contour of vegetation around palms (). We assigned smallholders into four BMP groups according to the number of BMPs the smallholder farmer employed: 0, 1, 2, or 3 BMPs (no farmer did all four BMPs).
Table 2. Description of ‘Best Management Practices’ (BMPs) as defined by the Roundtable on Sustainable Palm Oil. Information about uptake of BMPs by farmers was collected from interviews with the smallholder farmers (n = 40 farms; see SI for details of questionnaires).
Field surveys of understory vegetation structure
Surveys of understory vegetation were conducted on each farm, measuring 18 vegetation parameters within a 0.28 ha circular plot (i.e. 30 m radius plot; Fig SI2d) within each smallholding (see SI5 for further details). To quantify differences in understory vegetation cover among farms, we then used principal component analysis (PCA) to identify which vegetation parameters were the most important for explaining variability in understory vegetation structure across the smallholder farms (Table S4 and S5). The first principal component (PC1) accounted for 25% of the total variance, which was positively related to vegetation height and cover (>10 cm height) and palm contour vegetation cover, and was negatively related to leaf litter depth (Table S5). The second principal component (PC2) accounted for a further 15% of the total variance, and was positively associated with the occurrence of non-palm large trees and saplings, leaf-litter depth, and cover, and was negatively associated with bare ground cover (Table S5). We included PC1 and PC2 in our analyses as measures of understory vegetation cover and structure.
Measuring soil chemical properties
In each 0.28 ha survey plot, we also collected soil samples (20 cm depth) to quantify soil carbon and nutrients (see SI6). In subsequent analyses, we omitted soil variables that were highly correlated (Pearson’s r > 0.9; SI5; Table S6), and included soil organic carbon (%; SOC), total nitrogen, total phosphorus, and available phosphorus, which are important for the growth and yield of oil palm trees (Corley and Tinker Citation2016; Guillaume et al. Citation2016a; Webb et al. Citation2013).
Oil palm yields are also affected by soil type, for example, soils that are saline, acid sulfate, or poorly drained are less suitable for oil palm cultivation (Corley and Tinker Citation2016). We classified smallholdings according to soil type using ‘The Soils of Sabah’ base map (Panagos et al. Citation2011; see SI6), with most farms on mudstone, sandstone, and miscellaneous rocks (soil type 2; n = 24 farms), nine farms on mudstone and alluvium (soil type 3), and five farms on alluvium (soil type 1; Table S1).
Effects of climatic conditions (rainfall and temperature)
To control for possible time-lagged effects of climatic variables on yield, we analyzed rainfall and temperature variables from CRU TS 4.05 dataset (0.5° × 0.5° grid cells; 50 km resolution; Harris et al. Citation2020), averaged across 36 months prior to the study (i.e. between November 2016 and December 2019, covering the period of inflorescence development; see SI7 for more details). Farms from our six governance regions occurred within four different CRU climate data grids (Table S1). We included monthly minimum rainfall (mm; a measure of drought and water stress, which is an important limiting factor to FFB yields in Sabah) and maximum monthly temperature (°C; linked to solar radiation, which is important for oil palm growth (Carr Citation2011; Corley and Tinker Citation2016; Oettli, Behera, and Yamagata Citation2018) (see SI7).
Statistical analyses
All data and statistical analyses were performed in the R statistical programming (R Core Team Citation2019). Prior to fitting all GAMM models, we standardized explanatory variables by transforming every value as the ratio of that value to the maximum value for that variable.
Drivers of yield
To examine whether smallholder yields were influenced by management intensity (our management intensity index score, 0–1), understory vegetation cover (PC1 and PC2 scores), soil type and chemical properties (SOC, total N, total P, and available P), climate (average monthly maximum temperature and minimum monthly rainfall in the 36 months prior to the study), and farming system (monoculture or mixed agriculture), we fitted a Generalized Additive Mixed effect Model (GAMM) via restricted maximum likelihood (REML) using the gamm4 package (Wood and Scheipl Citation2017). GAMMs model both linear and non-linear relationships by using smoothing functions (i.e. splines) (Lin and Zhang Citation1999). We applied penalized cubic regression splines as the smoothing basis function, to remove non-influential explanatory variables from the model (Marra and Wood Citation2011). We applied the GAMM to the smallholder dataset excluding two yield outliers (n = 38 farms; see SI9), and included smoothing parameters for management intensity score, understory vegetation cover (PC1 and PC2 scores), soil chemical properties (SOC, total N, total P, and available P), minimum rainfall (mm month−1), and monthly maximum temperature (oC). We also included farm area with productive palms (ha; to control for differences in yield as a result of farm size), crop age (to control for possible variations in yield as a consequence of palm tree age; Hoffmann et al. Citation2017; Woittiez et al. Citation2017), and soil type. We fitted models with a Gaussian identity link function to obtain homoscedasticity and normality of residuals (see SI9 for further details of GAMMs).
To assess whether the use of BMPs influenced yields on farms, we examined the differences in yield across the four BMP groups (0, 1, 2, or 3 BMPs taken up by farmers). We fitted a GAMM with yield as a response variable, the number of BMPs employed, farming system (monoculture or mixed) and previous land-use as linear terms, and smoothing parameters (penalized cubic regression splines) for crop age, farm size, and monthly minimum rainfall. Our main analyses used FFB yield per farm (t ha−1 yr−1), but we repeated analyses using mean data for yield in kilograms per tree per year (kg tree−1 yr−1), which did not alter our main conclusions (see SI9).
Consequences of management intensity and BMPs
To examine the consequences of management intensity on understory vegetation cover and soil chemical properties (SOC, total N, total P, and available P) on farms, we fitted GAMMs similar to those for identifying drivers of yield. We fitted smoothing parameters to management intensity index, crop age, monthly minimum rainfall, and maximum temperature, and included previous land-use (forested or agriculture) to control for the effect that land-use history may have on soil chemical properties on farms (Guillaume et al. Citation2016b). We fitted the models using the full smallholder dataset (n = 40) to model the effects of management intensity on understory vegetation cover (PC1 and PC2 scores), SOC, total soil N, and total P. When modeling the effects of management intensity on available soil P, we used a reduced dataset excluding one outlier datapoint for available P (n = 39 farms) (see SI11). The models were fitted with an inverse Gaussian log link function to the SOC, a Gaussian log link function to the total N data, and a Gaussian identity link function to the PC1, PC2, total P, and available P data. Differences in soil chemical properties across the four BMP groups (0, 1, 2, or 3 BMPs taken up by farmers) are given in SI8 (Table S10).
Results
Summary of yield and management variability across smallholder oil palm farms
Across the 40 farms, we found that the reported yields (measured as FFB t ha−1 yr−1) varied widely, ranging from 6.9 to 37.4 t ha−1 yr−1 (median = 15.6 t ha−1 yr−1, SD = 7.3). This variation arose because smallholder yields varied among the six governance areas (median = 13.2–20.8 t ha−1 yr−1; ), and also among smallholders within the same governance area (Table S1).
Figure 1. Boxplots detailing variation in (a) fresh fruit bunch yield (FFB t ha−1 yr−1), (b) management intensity, (c) understory vegetation cover, yellow boxplots correspond to PC1 scores and green boxplots to PC2 scores, and (d) total phosphorus content (mg/kg) on farms in six governance areas (n = 40 farms; governance area number corresponds to those shown in figure S2b). Data show the median (horizontal bar) and interquartile range (box), as well as the range of the largest and smallest values within 1.5 times the interquartile range (vertical line).
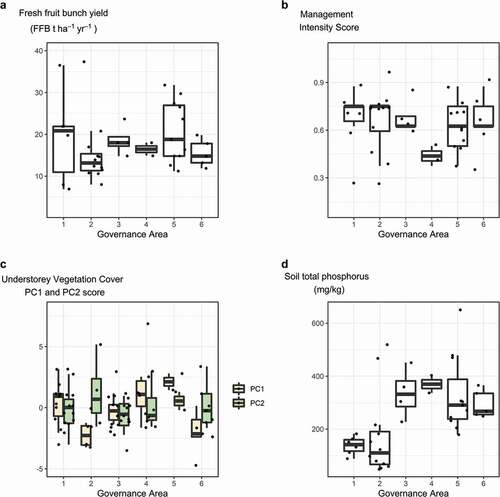
Smallholders also varied considerably in the way that they managed their farms, and the management intensity scores (synthesized from nine management practices, index max/min score range = 0–1; ) ranged from 0.25 to 1.00 (median = 0.69; ). The study farms were either oil palm monocultures (n = 19 farms) or mixed-crop farms (n = 21) cultivating fruit crops such as coconut (Cocos nucifera) or rambutan (Nephelium lappaceum) for household consumption. Some management practices were relatively consistent across farms, for example, most farms carried out palm frond pruning and there was little variation in FFB harvesting frequency (either once or twice a month). However, herbicide application rates (varying from 0 to 40 L ha−1 yr−1) and fertilizer inputs (varying from 0 to 1456 kg ha−1 yr−1, equivalent to 0–10 kg tree−1 yr−1) did vary widely (Table S1 and S9), as well as the types of fertilizer (e.g. borate, murate of potash, as well as relative composition of MPK and NPK) and herbicides applied (e.g. glyphosate, metsulphuron). The planting density of productive fruit-bearing palm trees (86–165 trees ha−1) also varied, but the median value across the study farms (136 trees ha−1) was within the recommended optimal planting density (130–145; Corley and Tinker Citation2016). We obtained planting stock information from eight farmers: one of whom planted dura, one planted pisifera, and six farmers planted tenera seed types. Smallholder farmers differed in the number of ‘Best Management Practices’ (BMPs) they employed (median = 2; ranging from two farms using no BMPs, to five farms using three BMPs, with the majority of farmers applying two BMPS, but no farmer using all four). Of the four types of BMPs, more than half of the farms cleared a contour of vegetation around palm trees (n = 23 farms), and about a third applied crop residues such as empty fruit bunches (n = 12 farms) or retained areas of ground vegetation cover (i.e. did not carry out blanket spraying) (n = 12); applying minimal amounts of herbicide was the least popular BMP (<2 L ha−1 yr−1; n = 9 farms) (Table S9).
Drivers of variation in yields across smallholder farms
Despite wide variation in yields (t ha−1 yr−1) among smallholder farms, only 3% of this variation in yield was explained by management intensity, understory vegetation cover (PC1 and PC2 scores), productive farm area (ha), oil palm tree age (years), soil chemical properties, or rainfall (adjusted R2 = 0.007; ; see Table S12 and Fig S3). Yield was not significantly influenced by farm productive area (n = 38 farms, F = 0.00, p = 1.00), crop age (F = 0.00, p = 1.00), or soil type (t = 0.80, p = .43; t = 1.89, p = .07). Our results show that 38/40 farmers employed at least one Best Management Practice (BMP) on their farm, but yields did not vary with the number of BMPs employed by a farmer (0–3 BMPs; n = 40, Adjusted-R squared = 0.028; ; see Table S14).
Figure 2. Boxplots show fresh fruit bunch yields (FFB t ha−1 yr−1) across smallholders employing 0, 1, 2, and 3 ‘Best Management Practices’ (BMPs; n = 40 farms). Data show the median (horizontal bar) and interquartile range (box), as well as the range of the largest and smallest values within 1.5 times the interquartile range (vertical line).
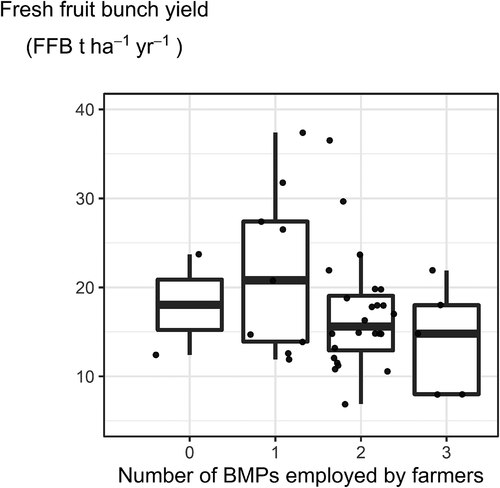
Figure 3. Partial effect plots of the generalized additive mixed effects models, showing (a) the component effect of the smoothed term (fitted with a penalized cubic regression spline as the smooth basis function) for management intensity on smallholder oil palm fresh fruit bunch yield (FFB t ha−1 yr−1; p = .41; n = 38) and (b) the component effect of the smoothed term (fitted with a penalized cubic regression spline as the smooth basis function) for management intensity on understory vegetation cover (PC1 score; F = 0.00, p = 1.00; n = 40). The points show the partial residuals, which are the difference between the partial effect and the data after all partial effects in the model have been accounted for. Management intensity was a non-influential explanatory variable in both models, and so the smoother parameter for has been penalized toward a straight line and shrunk the line toward zero. The blue shaded areas denote the uncertainty within the model, measured as the standard errors of the partial effect term combined with the standard errors of the model intercept. Explanatory variables were scaled prior to fitting the model.
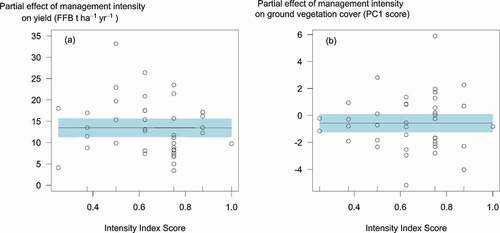
Impacts of management intensity
Our results showed that management intensity did not affect understory vegetation cover (; PC1 score, n = 40, F = 0.00, p = 1.00; PC2 score, F = 0.32, p = .13), SOC (n = 40, F = 0.00, p = 1.00), total N (n = 40, F = 0.00, p = 1.00), total P (n = 40, F = 0.00, p = 1.00), or available P (n = 39, F = 0.05, p = 1.00; Table S15). However, farms on previously cultivated agricultural land (n = 22 farms) had soil containing 28% more total N (t = −2.86, p = .005, adjusted R-squared = 0.14) and 139% more total P (t = −4.83, p < .001, adjusted R-squared = 0.491) and 21% more organic C (t = −2.30, p = .03, adjusted R-squared = 0.05) compared with farms on sites that had previously been forest (n = 18 farms), implying that previous land-use contributes substantially to current soil characteristics.
Discussion
Wide variation in reported yields across smallholder farms
Oil palm yields varied widely across the smallholder farms in our study, ranging from 6.9 to 37.4 FFB t ha−1 yr−1 (equivalent to 50–275 kg tree−1 yr−1), with an average farm yield of 15.6 t ha−1 yr−1 (117 kg tree−1 yr−1). Thus, 35% of the smallholders in our study exceeded the average smallholder production reported by the RSPO (18 t ha−1 yr−1) (RSPO 2020) and 20% exceeded the production of larger-scale industrial companies (23 t ha−1 yr−1; Fairhurst and Griffiths Citation2014). Whilst atypical, such high yields have been reported previously (Jelsma, Giller, and Fairhurst Citation2009), and a few of our study farms had reported yields similar to the estimated potential yields of large-scale plantations (35–40 t ha−1 yr−1; (Hoffmann et al. Citation2015)). However, despite these large variations in reported yields, our results show that management intensity did not influence yield across farms. Increasing the intensity of management practices such as cropping density, fertilization, and harvesting frequency can directly increase oil palm yields (Euler et al. Citation2016; Lee et al. Citation2014; Marcelino and Diaz Citation2016; Rhebergen et al. Citation2020; Woittiez et al. Citation2017), but reported yields may not necessarily increase with management intensity. For example, increasing cropping density may result in trees being planted too closely, which can reduce yields as trees start to compete for soil nutrients and light, although most (11 out 40) farms in our study had planting densities within recommended values of 130–145 (Corley and Tinker Citation2016; Rafii et al. Citation2013), because of advice from WildAsia. Our findings support those of Darras et al. (Citation2019) and Tao et al. (Citation2018a) who also found no effect of herbicide and fertilizer intensification on palm plantation yields or nutrient-use efficiency by palms. Thus, increased use of chemical inputs may not be necessary for closing yield gaps on smallholder farms (Soliman et al. Citation2016).
Our study analyzed data on yields and management practices from questionnaires and interviews, which can have potential biases. For example, respondents may be wary when providing responses to questions that involve sensitive information (Hilborn, Parrish, and Litle Citation2005), such as yield or use of herbicides and pesticides, given the negative perception of chemical products on the environment (Lazaroiu et al. Citation2019; Obiri et al. Citation2021). Moreover, social desirability bias can occur when respondents may want to ‘project a favourable image of themselves and so may provide a more socially desirable response’ (Krumpal Citation2013). Whilst these issues are difficult to eliminate, we mitigated these potential biases by conducting the interviews with WildAsia staff who have a well-established working rapport with the respondents and regularly share this kind of information (Bergen and Labonté Citation2020).
Additional drivers of variation in oil palm yield
We found no associations between yield and management practices. There could be time lags between implementing a particular management practice and observing its effects on yield (Rhebergen et al. Citation2020), but it is unlikely that lags would result in the large yield differences between the farms that we observed (6.9–37.4 FFB t ha−1 yr−1). Yields differed greatly among farms within the same governance area (<10 km apart), thus geographic factors that may affect nutrient uptake by the palm trees are also unlikely to be the main causes of yield variation. We also found no effect of climatic factors on yield. It is likely that rainfall was not an important factor in our analysis as the mean monthly rainfall in our study regions, which ranged between 228 and 289 (mm month−1), met the rainfall requirements for optimum FFB production (above 167 mm month−1; Oettli, Behera, and Yamagata Citation2018). We did not include temperature in our analyses because it co-varied with rainfall (see SI7), but the minimum (21.1–23.4°C) and maximum (28.9–31.3°C) temperatures at our study regions fall within the suitable temperature ranges for high FFB yields (22–24°C and 29–33°C, respectively) (Corley and Tinker Citation2016; Oettli, Behera, and Yamagata Citation2018). However, site-specific factors may nonetheless cause yield variation, such as topography which can influence water availability and drainage (although topography was fairly similar across our study farms) (Balasundram et al. Citation2006), as well as local disease and pest outbreaks (Corley and Tinker Citation2016).
Farms within the same governance regions were sometimes on different soil types (see SI6), which can influence nutrient loss and retention (Fujii et al. Citation2018), although we found only a weak non-significant effect of soil type on yield. Nutrient deficiencies are known to limit yields (Woittiez et al. Citation2018), but despite some farms having high total N, available P, and total P soil concentrations (the latter of which increased with smallholder management intensity), we found no relationship between soil nutrients and yield. Seed planting material influences yield (Barcelos et al. Citation2015; Woittiez et al. Citation2017), with tenera being the most productive, followed by dura and psifera (Corley and Lee et al. 1992). There were insufficient data to include type of planting material in our analyses of yield, but farmers differed in their sourcing of planting materials and seed varieties (e.g. wild harvesting, supply by government agricultural sectors or company nurseries). Different suppliers will provide different types of seeds, which will affect yield (Durand-Gasselin and Cochard Citation2005; De Vos et al. Citation2021), and planting material likely contributes to the variation in yields that we observed in our study. Using improved seed materials could help farmers increase productivity without increasing the intensity of other management practices, and should be an area of future research as planting material will be of crucial importance for farmers when replanting crops. Another important factor driving oil palm yield is pruning intensity, as the maintenance of an optimum number of palm fronds affects oil palm fruit production (Marcelino and Diaz Citation2016). These factors deserve more study to further develop best practices for smallholders, alongside investigating associations with soil arbuscular mycorrhizal fungal (AMF) (Auliana and Kaonongbua Citation2018) as they have been illustrated to improve yields in other types of crops (Gao et al. Citation2020; Kobae Citation2019).
Causes and consequences of varying management intensity
Smallholders varied considerably in how they managed their farms, even within the same governance area (e.g. intensity score 0.25–1.00, and 0–3 BMPs in Site 2, Reka Halus). Thus, local village governance is not the only factor affecting management decisions of smallholder farmers, which may be influenced by available capital (Jelsma et al. Citation2019; Krishna et al. Citation2015; Lee et al. Citation2014), farmer experience (Pandey and Diwan Citation2020), their desire to make environmentally conscious management choices (such as uptake of BMPs; Romero et al. Citation2019), or wishing to make risk-averse decisions (Chen et al. Citation2018; Martey and Kuwornu Citation2021).
Farmers are encouraged to take-up BMPs as a way to improve environmental sustainability on farms, but our results indicate no relationship between the application of BMPs and yield, or with soil chemical properties (SOC, total N, total P, and available P). Low total C and SOC levels (<2%) were observed across the majority of the study farms (see SI6), and we found no evidence that BMPs improved soil fertility or soil nutrient content, in contrast to other studies (Tao et al. Citation2017; Che Ku Hafeez et al. Citation2020). The implementation of BMPs generally requires more labor inputs (Rhebergen et al. Citation2020); therefore, a better understanding of the sustainability benefits of BMPs, alongside the improved profitability for smallholders, is required if farmers are to benefit from their wider take-up. In addition, clear standardized guidance for employing BMPs is needed if BMPs are to improve yields, soil fertility, and farmer incomes.
Implications of no trade-offs for smallholder farmers
We found little evidence for any strong inter-relationship between yield, vegetation cover, and management intensity. Trade-offs have been shown to occur between smallholder profitability, biodiversity, and ecosystem processes within tropical agricultural systems (Clough et al. Citation2016; Grass et al. Citation2020), but those studies spanned a much greater range of land-use intensities than in our study. We find little evidence that maintaining greater vegetation cover on farms resulted in any reduction in FFB yields, or that increasing intensity of management resulted in higher oil palm yields. By contrast, we found that high yields occurred on some farms with low-intensity management. This finding has potentially important implications for smallholder profitability because any reduction in the reliance on expensive agricultural inputs could result in savings in labor costs and chemicals, such as fertilizers and herbicides (Darras et al. Citation2019). Secondly, high yield from low-intensity management is a potential positive inducement for farmers to enhance the environmental sustainability of their smallholdings, given the benefits of retaining vegetation cover with oil palm plantations for promoting biodiversity and ecosystem functions (Ashton-Butt et al. Citation2018; Darras et al. Citation2019; Luke et al. Citation2019).
Most smallholders that we sampled were part of a Smallholder Group Scheme organized by a local social enterprise (WildAsia), who provide support to farmers for the uptake of sustainable agricultural practices, as well as the uptake of RSPO and MSPO certification. Although we found none, trade-offs with yield could occur at much lower or higher intensity management than was observed in our study, for example, non-certified smallholders have differing management practices and lower oil palm yields compared to sustainably certified farmers (De Vos et al. Citation2021). Smallholders in other regions may have different management strategies, and this should be considered when assessing the generality of our findings, and further studies examining the consequences of different management strategies across a wide range of smallholder landscapes will be important for understanding the ubiquity of our findings.
Conclusion
The results of our study showed that applying sustainable management practices does not reduce yields, and we found no evidence for inter-relationships between yield and management practices among oil palm smallholdings. Yields varied about five-fold across farms, but smallholder management, understory vegetation cover, soil chemical properties, rainfall, and farming system explained only a small amount of this variation, and farms with high understory vegetation cover did not have reduced yield. These findings have important implications for smallholder livelihoods if farmers can minimize their reliance on chemical inputs, which may also help the conservation of biodiversity and the environmental sustainability of oil palm farms. A better understanding of how some farmers achieve yields as high as those on industrial plantations could be shared among farmer groups to help reduce yield variation across smallholder farms, and improve farmer incomes.
Authors’ contributions
JKH, CJM, MJMS, and SGA conceived the project, which was designed by SGA, TRH, ABS, JKH, and CJM, in discussion with MJMS and RA. ABS, SGA, and TRH collected the data. SGA analyzed the data and led the writing of the manuscript, with supervision from CJM and JKH. All authors contributed critically to revising the manuscript.
Consent to participate
Informed consent was obtained from all individual participants included in the study prior to conducting interviews for questionnaires used in this study. Written consent was obtained from each participant.
Declaration
We thank Agnes Lee Agama, Johnny Larenus, and Peter Chang for logistical support; Jenner Jasper, Ted Kelvin Sudin, Trenner Ensim, Sabri, Azrul Matnawi, and Zakri Bakri (WildAsia) for their assistance with conducting smallholder interviews; Rolando Robert and Noreen Majalap (Forest Research Centre, Sabah) for soil analysis; and anonymous reviewers for suggestions to improve the paper. Most of all, we thank the farmers who took part in this study.
Ethical approval
The project received ethical approval from the Biology Ethics Committee, University of York (Ref. SGA201906), and permission from the Sabah Biodiversity Council (Ref. JKM/MBS.1000-2/2 JLD.8), Danum Valley Management Committee (Ref. YS/DVMC/2019/27), and South East Asia Rainforest Research Partnership (project number 18033) for permission to conduct our research in Sabah, Malaysia.
Availability of data and material
The data that support the findings of this study are openly available in “NERC Environmental Data Service” at https://doi.org/10.5285/38487932-b32a-4b15-9fda-ea812c463466.
Supplemental Material
Download MS Word (4.1 MB)Disclosure statement
We do not report any conflicts of interest or competing interests.
Supplementary material
Supplemental data for this article can be accessed online at https://doi.org/10.1080/21683565.2022.2131691
Additional information
Funding
References
- Ashton-Butt, A., A. K. Anak, A. S. C. Aryawan, M. N. Hood, D. Purnomo, R. W. Suhardi, R. Wahyuningsih, S. Willcock, G. M. Poppy, J.-P. Caliman, et al. 2018. Understory vegetation in oil palm plantations benefits soil biodiversity and decomposition rates. Frontiers in Forests and Global Change. 1. doi:10.3389/ffgc.2018.00010.
- Auliana, S, and W. Kaonongbua. 2018. “Preliminary study on biodiversity of arbuscular mycorrhizal fungi (AMF) in oil palm (Elaeis Guineensis Jacq.) plantations in Thailand.” In IOP Conference Series: Earth and Environmental Science, Samarinda, East Kalimantan, Indonesia. Vol. 144. doi:10.1088/1755-1315/144/1/012010.
- Balasundram, S., P. Robert, D. Mulla, and D. Allan. 2006. Relationship between oil palm yield and soil fertility as affected by topography in an Indonesian plantation. Communications in Soil Science and Plant Analysis 37 (9–10):1321–37. doi:10.1080/00103620600626817.
- Barcelos, E., S. D. A. Rios, R. N. V. Cunha, R. Lopes, S. Y. Motoike, E. Babiychuk, A. Skirycz, and S. Kushnir. 2015. Oil palm natural diversity and the potential for yield improvement. Frontiers in Plant Science 6 (MAR). doi: 10.3389/fpls.2015.00190.
- Barnes, A. D., M. Jochum, S. Mumme, N. F. Haneda, A. Farajallah, T. H. Widarto, and U. Brose. 2014. Consequences of tropical land use for multitrophic biodiversity and ecosystem functioning. Nature Communications 5. doi:10.1038/ncomms6351.
- Bergen, N., and Ronald Labonté. 2020. ‘Everything is perfect, and we have no problems’: detecting and limiting social desirability bias in qualitative research. Qualitative Health Research 30 (5):783–92. doi:10.1177/1049732319889354.
- Bonneau, X., R. Impens, and M. Buabeng. 2018. Optimum oil palm planting density in West Africa. OCL - Oilseeds and Fats, Crops and Lipids 25 (2). doi: 10.1051/ocl/2017060.
- Carr, M. K. V. 2011. The water relations and irrigation requirements of oil palm (Elaeis Guineensis): a review. Experimental Agriculture. doi:10.1017/S0014479711000494.
- Chen, X., D. Zeng, X. Ying, and X. Fan. 2018. Perceptions, risk attitude and organic fertilizer investment: evidence from rice and banana farmers in Guangxi, China. Sustainability (Switzerland) 10 (10). doi: 10.3390/su10103715.
- Clough, Y., V. V. Krishna, M. D. Corre, L. H. Kevin Darras, A. M. Denmead, S. Moser, S. Moser, O. Musshoff, S. Steinebach, E. Veldkamp, et al. 2016. Land-use choices follow profitability at the expense of ecological functions in Indonesian smallholder landscapes. Nature Communications. 7. doi:10.1038/ncomms13137.
- Corley, R. H. V. 1973. Effects of plant density on growth and yield of oil palm. Experimental Agriculture 9 (2):169–80. doi:10.1017/S0014479700005639.
- Corley, R. H. V., and P. B. Tinker. 2016. The oil palm. Fifth. Vol. 1. Hoboken, New Jersey, United States: Wiley. ISBN: 9781405189392. doi:10.1002/9781118953297.
- Darras, K. F. A., M. D. Corre, G. Formaglio, A. Tjoa, A. Potapov, F. Brambach, K. T. Sibhatu, I. Grass, A. A. Rubiano, D. Buchori, et al. 2019. Reducing fertilizer and avoiding herbicides in oil palm plantations—ecological and economic valuations. Frontiers in Forests and Global Change. 2. doi:10.3389/ffgc.2019.00065.
- Descals, A., S. Wich, E. Meijaard, D. Gaveau, S. Peedell, and Z. Szantoi. 2020. High-resolution global map of smallholder and industrial closed-canopy oil palm plantations. Earth System Science Data Discussions. doi:10.5194/essd-2020-159.
- Durand-Gasselin, T., and B. Cochard. 2005. Oil palm seed distribution. OCL - Oleagineux Corps Gras Lipides 12:148–53. doi:10.1051/ocl.2005.0148.
- Edwards, D. P., J. A. Hodgson, K. C. Hamer, S. L. Mitchell, A. H. Ahmad, S. J. Cornell, and D. S. Wilcove. 2010. Wildlife-friendly oil palm plantations fail to protect biodiversity effectively. Conservation Letters 3:236–42. doi:10.1111/j.1755-263X.2010.00107.x.
- Edwards, D. P. , Magrach, A. , Woodcock, P. , Lim, N. T.-L. , Edwards, F. A. , Larsen, T. H. Hsu, W. W. et al. (2014). Selective-logging and oil palm: Multitaxon impacts, biodiversity indicators, and trade-offs for conservation planning. Ecological Applications 24(8): 2029–2049. doi: 10.1890/14-0010.110.1890/14-0010.1.sm
- Euler, M., M. P. Hoffmann, Z. Fathoni, and S. Schwarze. 2016. Exploring yield gaps in smallholder oil palm production systems in Eastern Sumatra, Indonesia. Agricultural Systems 146:111–19. doi:10.1016/j.agsy.2016.04.007.
- Fairhurst, T., and W. Griffiths. 2014. Oil palm: best management practices for yield intensification. Singapore: International Plant Nutrition Institute (IPNI).
- Fischer, J., D. J. Abson, A. Bergsten, N. F. Collier, I. Dorresteijn, J. Hanspach, K. Hylander, J. Schultner, and F. Senbeta. 2017. Reframing the food–biodiversity challenge. Trends in Ecology & Evolution 32:335–45. doi:10.1016/j.tree.2017.02.009.
- Formaglio, G., E. Veldkamp, X. Duan, A. Tjoa, and M. D. Corre. 2020. Herbicide weed control increases nutrient leaching compared to mechanical weeding in a large-scale oil palm plantation. Biogeosciences 17 (21):5243–62. doi:10.5194/bg-17-5243-2020.
- Fujii, K., M. Shibata, K. Kitajima, T. Ichie, K. Kitayama, and B. L. Turner. 2018. Plant–soil interactions maintain biodiversity and functions of tropical forest ecosystems. Ecological Research 33 (1):149–60. doi:10.1007/s11284-017-1511-y.
- Gao, X., H. Guo, Q. Zhang, H. Guo, L. Zhang, C. Zhang, Z. Gou, Y. Liu, J. Wei, A. Chen, et al. 2020. Arbuscular Mycorrhizal Fungi (AMF) enhanced the growth, yield, fiber quality and phosphorus regulation in upland cotton (Gossypium Hirsutum L.). Scientific Reports. 10(1). doi:10.1038/s41598-020-59180-3.
- García-Orenes, F., A. Roldán, J. Mataix-Solera, A. Cerdà, M. Campoy, V. Arcenegui, and F. Caravaca. 2012. Soil structural stability and erosion rates influenced by agricultural management practices in a semi-arid Mediterranean agro-ecosystem. Soil Use and Management 28 (4):571–79. doi:10.1111/j.1475-2743.2012.00451.x.
- Gaveau, D. L. A., M. A. Bruno Locatelli, H. Y. Salim, P. Pacheco, and D. Sheil. 2018. Rise and fall of forest loss and industrial plantations in Borneo (2000–2017). Conservation Letters. doi:10.1111/conl.12622.
- Grass, I., V. V. Christoph Kubitza, M. D. Krishna, O. M. Corre, P. Pütz, J. Drescher, J. Drescher, K. Rembold, E. S. Ariyanti, A. D. Barnes, et al. 2020. Trade-offs between multifunctionality and profit in tropical smallholder landscapes. Nature Communications. 11(1). doi:10.1038/s41467-020-15013-5.
- Guillaume, T., A. M. Holtkamp, M. Damris, B. Brümmer, and Y. Kuzyakov. 2016a. Soil degradation in oil palm and rubber plantations under land resource scarcity. Agriculture, Ecosystems & Environment. 232:110–18. doi:10.1016/j.agee.2016.07.002.
- Guillaume, T., D. Maranguit, K. Murtilaksono, and Y. Kuzyakov. 2016b. Sensitivity and resistance of soil fertility indicators to land-use changes: new concept and examples from conversion of Indonesian rainforest to plantations. Ecological Indicators 67:49–57. doi:10.1016/j.ecolind.2016.02.039.
- Hafeez, C. K., C. K. Denang, K. S. D. Singh, A. Arifin, and S. R. Keeren. 2020. Effects of empty fruit bunch (EFB) application on oil palm yield, soil properties and cost-benefits analysis. Journal of Environmental Science and Pollution Research 6 (3). doi: 10.30799/jespr.208.20060301.
- Harris, I., T. J. Osborn, P. Jones, and D. Lister. 2020. Version 4 of the CRU TS monthly high-resolution gridded multivariate climate dataset. Scientific Data 7 (1). doi: 10.1038/s41597-020-0453-3.
- Hilborn, R., J. K. Parrish, and K. Litle. 2005. Fishing rights or fishing wrongs? Reviews in Fish Biology and Fisheries 15 (3):191–99. doi:10.1007/s11160-005-5138-7.
- Hoffmann, M. P., C. R. Donough, S. E. Cook, M. J. Fisher, C. H. Lim, Y. L. Lim, J. Cock, S. P. Kam, S. N. Mohanaraj, K. Indrasuara, et al. 2017. Yield gap analysis in oil palm: framework development and application in commercial operations in Southeast Asia. Agricultural Systems 151:12–19. doi:10.1016/j.agsy.2016.11.005.
- Hoffmann, M. P. , Donough, C. , Oberthur, T. , Castaneda Vera, A. , Van Wijk, M. T. , Lim, C. H. , Asmono, D et al. 2015. Benchmarking yield for sustainable intensification of oil palm production in Indonesia using PALMSIM. . The Planter, Kuala Lumpur. 91(1067).
- Jelsma, I., K. Giller, and T. Fairhurst. 2009. Smallholder oil palm production systems in Indonesia: lessons learned from the NESP Ophir project.
- Jelsma, I., L. S. Woittiez, J. Ollivier, and A. H. Dharmawan. 2019. Do wealthy farmers implement better agricultural practices? An assessment of implementation of good agricultural practices among different types of independent oil palm smallholders in Riau, Indonesia. Agricultural Systems 170:63–76. doi:10.1016/j.agsy.2018.11.004.
- Kobae, Y. 2019. Dynamic phosphate uptake in arbuscular mycorrhizal roots under field conditions. Frontiers in Environmental Science 6. doi:10.3389/fenvs.2018.00159.
- Krishna, V. V., M. Euler, H. Siregar, Z. Fathoni, and M. Qaim. 2015. “Farmer heterogeneity and differential livelihood impacts of oil palm expansion among smallholders.” EFForTS Discussion Paper Series No. 13.
- Krumpal, I. 2013. Determinants of social desirability bias in sensitive surveys: a literature review. Quality & Quantity 47:2025–47. doi:10.1007/s11135-011-9640-9.
- Kurniawan, S., M. D. Corre, S. R. Utami, and E. Veldkamp. 2018. Soil biochemical properties and nutrient leaching from smallholder oil palm plantations, Sumatra-Indonesia. Agrivita 40 (2). doi: 10.17503/agrivita.v40i2.1723.
- Kwatrina, R. T., B. Yanto Santosa, N. Santoso, and N. Santoso. 2018. The impacts of oil palm plantation establishment on the habitat type, species diversity, and feeding guild of mammals and herpetofauna. Biodiversitas 19 (4):1213–19. doi:10.13057/biodiv/d190405.
- Lazaroiu, G., M. Andronie, C. Uţă, and I. Hurloiu. 2019. Trust management in organic agriculture: sustainable consumption behavior, environmentally conscious purchase intention, and healthy food choices. Frontiers in Public Health 7. doi:10.3389/fpubh.2019.00340.
- Lee, J., S. Huay, J. Ghazoul, K. Obidzinski, and L. P. Koh. 2014. Oil palm smallholder yields and incomes constrained by harvesting practices and type of smallholder management in Indonesia. Agronomy for Sustainable Development 34 (2):501–13. doi:10.1007/s13593-013-0159-4.
- Lin, X., and D. Zhang. 1999. Inference in generalized additive mixed models by using smoothing splines. Journal of the Royal Statistical Society. Series B, Statistical Methodology 61 (2):381–400. doi:10.1111/1467-9868.00183.
- Luke, S. H., D. Purnomo, A. D. Advento, A. A. K. Aryawan, M. Naim, R. N. Pikstein, S. Ps, T. D. S. Rambe, J.P. Caliman, Snaddon, J. L., Foster, W. A., Turner, E. C., et al. 2019. Effects of understory vegetation management on plant communities in oil palm plantations in Sumatra, Indonesia. Frontiers in Forests and Global Change. 2. doi:10.3389/ffgc.2019.00033.
- Marcelino, J., and E. Diaz. 2016. Frond pruning enhanced the growth and yield of eight-year-old oil palm (Elaeis Guineensis Jacq.). Annals of Tropical Research 96–105. doi:10.32945/atr3827.2016.
- Marra, G., and S. N. Wood. 2011. Practical variable selection for generalized additive models. Computational Statistics & Data Analysis 55 (7):2372–87. doi:10.1016/j.csda.2011.02.004.
- Martey, E., and J. K. M. Kuwornu. 2021. Perceptions of climate variability and soil fertility management choices among smallholder farmers in Northern Ghana. Ecological Economics 180:106870. doi:10.1016/j.ecolecon.2020.106870.
- Obiri, B. D., E. A. Obeng, K. A. Oduro, M. M. Apetorgbor, T. Peprah, A. Duah-Gyamfi, and J. K. Mensah. 2021. Farmers’ perceptions of herbicide usage in forest landscape restoration programs in Ghana. Scientific African 11:e00672. doi:10.1016/j.sciaf.2020.e00672.
- Oettli, P., S. K. Behera, and T. Yamagata. 2018. Climate based predictability of oil palm tree yield in Malaysia. Scientific Reports 8 (1). doi: 10.1038/s41598-018-20298-0.
- Panagos, P., A. Jones, C. Bosco, and P. S. Senthil Kumar. 2011. European digital archive on soil maps (EuDASM): preserving important soil data for public free access. International Journal of Digital Earth 4 (5):434–43. doi:10.1080/17538947.2011.596580.
- Pandey, C., and H. Diwan. 2020. Assessing fertilizer use behaviour for environmental management and sustainability: a quantitative study in agriculturally intensive regions of Uttar Pradesh, India. Environment, Development and Sustainability. doi:10.1007/s10668-020-00848-1.
- Pauli, N., C. Donough, T. Oberthür, J. Cock, R. Verdooren, R. G. Abdurrohim, G. Abdurrohim, K. Indrasuara, A. Lubis, T. Dolong, et al. 2014. Changes in soil quality indicators under oil palm plantations following application of ‘best management practices’ in a four-year field trial. Agriculture, Ecosystems & Environment 195:98–111. doi:10.1016/j.agee.2014.05.005.
- Rafii, M. Y., Z. A. Isa, A. Kushairi, G. B. Saleh, and M. A. Latif. 2013. Variation in yield components and vegetative traits in Malaysian oil palm (Elaeis Guineensis Jacq.) Dura×pisifera hybrids under various planting densities. Industrial Crops and Products 46:147–57. doi:10.1016/j.indcrop.2012.12.054.
- Rahman, N., K. E. Giller, D. N. Andreas, J. Magid, D. V. Gerrievan, and T. B. Bruunc. 2021. The Effects of Management Practices on Soil Organic Carbon Stocks of Oil Palm Plantations in Sumatra, Indonesia. Journal of Environmental Management 278:111446. doi:10.1016/j.jenvman.2020.111446.
- R Core Team. 2019. R: A language and environment for statistical computing. Vienna, Austria: R Foundation for Statistical Computing.
- Rhebergen, T., S. Zingore, K. E. Giller, C. A. Frimpong, K. Acheampong, F. T. Ohipeni, E. K. Panyin, V. Zutah, and T. Fairhurst. 2020. Closing yield gaps in oil palm production systems in Ghana through best management practices. European Journal of Agronomy 115:126011. doi:10.1016/j.eja.2020.126011.
- Romero, M. M., K. R. Wollni, R. Asnawi, B. Irawan, and B. Irawan. 2019. Promoting biodiversity enrichment in smallholder oil palm monocultures – experimental evidence from Indonesia. World Development 124:104638. doi:10.1016/j.worlddev.2019.104638.
- RSPO P&C. 2018. Principles and criteria for the production of sustainable palm oil. Kuala Lumpur, Malaysia: RSPO P&C.
- Sahat, S., Z. Yusop, M. Askari, and A. D. Ziegler. 2016. “Estimation of soil erosion rates in oil palm plantation with different land cover.” In IOP Conference Series: Materials Science and Engineering, 2015. Langkawi, Malaysia. Vol. 136. doi:10.1088/1757-899X/136/1/012086.
- Samedani, B., A. S. Juraimi, S. A. S. Abdullah, M. Y. Rafii, A. A. Rahim, and M. P. Anwar. 2014. Effect of cover crops on weed community and oil palm yield. International Journal of Agriculture and Biology 16 (1):23–31.
- Savilaakso, S., C. Garcia, J. Garcia-Ulloa, J. Ghazoul, M. R. Martha Groom, Y. L. Guariguata, Y. Laumonier, R. Nasi, G. Petrokofsky, J. Snaddon, et al. 2014. Systematic review of effects on biodiversity from oil palm production. Environmental Evidence. 3. doi:10.1186/2047-2382-3-4.
- Soliman, T., F. K. S. Lim, J. S. H. Lee, and L. R. Carrasco. 2016. Closing oil palm yield gaps among Indonesian smallholders through industry schemes, pruning, weeding and improved seeds. Royal Society Open Science 3 (8):160292. doi:10.1098/rsos.160292.
- Sundram, S., L. P. L. Angel, and S. A. Sirajuddin. 2019. Integrated balanced fertiliser management in soil health rejuvenation for a sustainable oil palm cultivation: a review. Journal of Oil Palm Research 31 (3). doi: 10.21894/jopr.2019.0045.
- Tamburini, G., R. Bommarco, T. C. Wanger, C. Kremen, M. G. A. van der Heijden, M. Liebman, and S. Hallin. 2020. Agricultural diversification promotes multiple ecosystem services without compromising yield. Science Advances 6 (45). doi: 10.1126/SCIADV.ABA1715.
- Tao, H. H., C. Donough, M. P. Joska Gerendas, A. C. Hoffmann, H. Sugianto, R. Wandri, R. Wandri, G. A. Rahim, M. Fisher, R. P. Rötter, et al. 2018a. Fertilizer management effects on oil palm yield and nutrient use efficiency on sandy soils with limited water supply in Central Kalimantan. Nutrient Cycling in Agroecosystems 112 (3):317–33. doi:10.1007/s10705-018-9948-0.
- Tao, H. H., E. M. Slade, K. J. Willis, J. P. Caliman, and J. L. Snaddon. 2016. Effects of soil management practices on soil fauna feeding activity in an Indonesian oil palm plantation. Agriculture, Ecosystems & Environment 218:133–40. doi:10.1016/j.agee.2015.11.012.
- Tao, H. H., J. L. Snaddon, E. M. Slade, J. P. Caliman, R. H. Widodo, Suhardi, K. J. Willis, and J. Kathrine. 2017. Long-term crop residue application maintains oil palm yield and temporal stability of production. Agronomy for Sustainable Development 37 (4). doi: 10.1007/s13593-017-0439-5.
- Tao, H. H., J. L. Snaddon, E. M. Slade, L. Henneron, J. P. Caliman, and K. J. Willis. 2018b. Application of oil palm empty fruit bunch effects on soil biota and functions: A case study in Sumatra, Indonesia. Agriculture, Ecosystems & Environment. doi:10.1016/j.agee.2017.12.012.
- Tengoua, F. F., M. M. Hanafi, A. S. Idris, and S. R. Syed-Omar. 2015. Screening for optimum concentrations of boron, copper and manganese for the growth of three-month old oil palm seedlings in solution culture. Pertanika Journal of Tropical Agricultural Science 38 (1):113–126.
- Tscharntke, T., A. M. Klein, A. Kruess, I. Steffan-Dewenter, and C. Thies. 2005. Landscape perspectives on agricultural intensification and biodiversity - ecosystem service management. Ecology Letters 8:857–74. doi:10.1111/j.1461-0248.2005.00782.x.
- Varkkey, H., A. Tyson, and S. A. B. Choiruzzad. 2018. Palm oil intensification and expansion in Indonesia and Malaysia: environmental and socio-political factors influencing policy. Forest Policy and Economics 92:148–59. doi:10.1016/j.forpol.2018.05.002.
- Vos, R. E., A. S. De, P. J. Maja Slingerland, D. M. Van, and J. M. Lucey. 2021. Independent oil palm smallholder management practices and yields: can RSPO certification make a difference? Environmental Research Letters 16 (6). doi: 10.1088/1748-9326/ac018d.
- Webb, E., J. Michael, P. N. Nelson, C. Bessou, J.-P. Caliman, and E. S. Sutarta. 2013. “Sustainable management of soil in oil palm plantings.” In Proceedings of a Workshop Held in Medan, Indonesia, 2013. Medan, Indonesia.
- Woittiez, L. S., M. Slingerland, R. Rafik, and K. E. Giller. 2018. Nutritional imbalance in smallholder oil palm plantations in Indonesia. Nutrient Cycling in Agroecosystems 111 (1):73–86. doi:10.1007/s10705-018-9919-5.
- Woittiez, L. S., M. T. van Wijk, M. Slingerland, V. N. Meine, and K. E. Giller. 2017. Yield gaps in oil palm: A quantitative review of contributing factors. European Journal of Agronomy. doi:10.1016/j.eja.2016.11.002.
- Wood, S., and F. Scheipl. 2017. “Gamm4: generalized additive mixed models using Mgcv and Lme4.” R Package Version 0.2-5. 2017.
- Yi, L. G., S. A. A. Wahid, P. Tamilarasan, and C. S. Siang. 2019. Enhancing sustainable oil palm cultivation using compost. Journal of Oil Palm Research 31 (3). doi: 10.21894/jopr.2019.0037.
- Zeng, Z., A. D. Lyndon Estes, A. C. Ziegler, T. Searchinger, F. Hua, K. Guan, A. Jintrawet, E. F. Wood, and E. F. Wood. 2018. Highland cropland expansion and forest loss in southeast asia in the twenty-first century. Nature Geoscience 11 (8):556–62. doi:10.1038/s41561-018-0166-9.
- Zheng, F.-L. 2006. Effect of vegetation changes on soil erosion on the loess plateau. Pedosphere 16 (4):420–27. doi:10.1016/s1002-0160(06)60071-4.