Abstract
Molecular and functional mechanisms of bone metastases were poorly understood. This study was to screen out differentially expressed genes (DEGs) and functional proteins in bone metastases from lung for better understanding of the molecular and functional mechanisms. Our results suggested CTSS, CTSD, MX1, NKX2-1 might play a decisive role in bone metastasis. Collectively, these results demonstrated that bone metastasis from lung cancer would lead to changes in lysosome function, which may affect the decomposition and elimination of old bone matrix, thus affecting bone turnover. In addition, our findings provided new insights into the prediction and treatment of bone metastases.
Introduction
Lung cancer was one of the most common and malignant cancers among both men and women worldwide (Parkin et al. Citation2005). The skeleton was the most common site of cancer metastasis (Brown et al. Citation2005). Bone metastases from lung cancer was defined as lung cancer cells metastasizing and invading bones. And the incidence of bone metastases was highly associated with the tumor histological type of primary location. Adenocarcinoma was recognized as the cancer with the highest incidence of bone metastasis, followed by small cell lung cancer and squamous cell carcinoma. It’s reported that in patients with bone metastasis, 86% were characterized by osteolytic destruction, 6.9% by the osteogenic destruction, and the remainder showed mixed destruction. Many factors were closely connected with facilitating metastases of tumors to bone, including blood flow in red bone marrow, immobilized growth factors in the bone matrix, and adhesive molecules on the tumor cells (Coleman Citation1997, James et al. Citation2003, Koenders et al. Citation1991, Roodman Citation2004).
Surprisingly, 30% to 40% of the patients with advanced lung cancer would develop bone metastasis, which severely diminished quality of life and dramatically increased mortality rates (Rove and Crawford Citation2009, Weiss and Wedin Citation2011). Osteolytic bone metastasis induced by lung cancer usually was associated with enhancing osteoclast activity and decreasing osteoblast capacity, finally leading to bone resorption (Sims and Gooi Citation2008, Sterling et al. Citation2011). Therefore early detection was crucial for prognosis of the disease. It is important to explore the mechanism of promoting bone metastasis to develop biological marker for prediction and therapy strategies.
The biology of organ-specific metastasis was poorly understood. Most studies were associated with breast cancer, but rarely with lung cancer. Recent reports showed disruption of DDR1 hampered tumor cell survival and led to impaired early tumor–bone, and inhibition of DDR1 crucially altered bone colonization, which suggested that DDR1 might represent a novel therapeutic target involved in bone metastasis (Valencia et al. Citation2012). Research on the identification of differentially gene expression in stage I NSCLC tumors using subtractive hybridization, showed that several genes were significantly associated with metastasis in NSCLC patients (Ji et al. Citation2003). To characterize molecular expression patterns in breast cancer metastasis, study on hypermethylation of five genes (Cyclin D2, Twist, RAR-β, RASSF1A, and HIN-1) in metastasis to four common tissues: lymph node, bone, brain, and lung, indicated that demethylation of gene might be a potiential therapy (Mehrotra et al. Citation2004). However, there was no study on the bioinformatics of skeleton metastasis in lung cancer.
In this study, gene expression of bone marrow negative and bone marrow positive samples were analyzed. Besides, the protein–protein interaction (PPI) network was constructed to figure out the regulation relationship among these differentially expressed genes (DEGs). Functional enrichment biological process and pathway were analyzed by gene ontology (GO) and pathway enrichment analysis. Furthermore, core proteins were screened out from the constructed PPI network. These findings might provide new insights into the signature prediction and the therapeutic strategies of skeleton metastasis from lung cancer.
Material and methods
Date source and processing
Expression data of bone metastasis patients with lung cancer was downloaded from the Gene Expression Omnibus (GEO, http://www.ncbi.nlm.nih.gov/geo/) database (accession NO. GSE10799). A total of 16 patients with lung cancer were selected, including 9 negative and 7 positive bone marrow samples. Normalization was carried out across multiple data sets in common to all data sets. Arrays were scanned by standard Affymetrix protocols in order to make easy access and manipulation of probe intensity data. Expression values were calculated by use of Affymetrix GeneChip analysis software MAS 5.0 (Santa Clara, CA). Each probe set was treated as an individual gene (Gautier et al. Citation2004).
Differentially expressed gene screening
Firstly, based on the chip GSE10799 data platform (GPL570), gene names were listed according to the probe number of the pre-processing microarray data. Average expression value was used as the gene expression values, when multiple probes correspond to the same gene. DEGs from seven positive bone marrow samples were analyzed compared with the nine negative bone marrow samples through Limma package in R software application (P < 0.05, |logFC| > 1) (Smyth Citation2005).
Functional enrichment analysis of DEGs
Gene Ontology (GO) enrichment analysis of DEGs was further conducted (FDR <0.05) using online DAVID software (http://david.abcc.ncifcrf.gov/summary.jsp) (Dennis et al. Citation2003). KEGG pathway analysis of DEGs was carried out using cluster data (Yu et al. Citation2012) package in R (P < 0.05). Pathview software (Luo and Brouwer Citation2013), a new kind of software used to integrate and visualize data, was used to map the most significant enrichment pathway.
PPI network analysis
PPI network of the DEGs was constructed with string online software (http://string-db.org/) (combined score >0.4) (Franceschini et al. Citation2013). Score of relationship, including neighborhood, fusion, co-occurrence, homology, co-expression, experimental, knowledge, text-mining, and combined score were assessed. To visualize the interaction network, map was drawn to show the relationship among differentially expressed genes.
Results
Data source and DEGs screening
Black line of the box, median of each set of data, was almost in the same line, which indicated that pre-processing dates were standardized (), compared to the original data in which median of each set of data were not in a line (). Based on their bone marrow status, patients were divided into two groups: bone marrow-negative (n = 9) and bone marrow-positive (n = 7).
Figure 1. Expression cassette value of gene microarray before and after standardization. The horizontal axis shows the sample name, and longitudinal axis shows expression values. Black line is the median of each set of data.
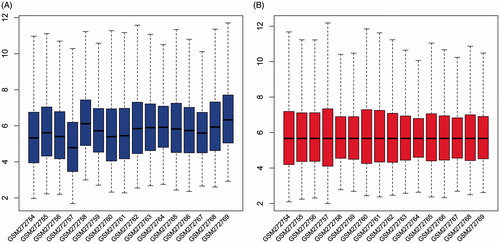
A total of 390 DEGs were obtained from bone marrow positive samples, compared with bone marrow negative samples through Limma package in R software application (P < 0.05, |logFC| > 1), including 77 up-regulated and 313 downregulated genes (, Supplementary Table 1). Besides, the volcano figure () and heatmap () were obtained.
Figure 2. Volcano plot of differentially expressed genes. Ordinate stand for logFC and abscissa for -log10 (P value). Red dot represents the significant differentially expressed gene, and black represents the non-significant differentially expressed genes.

Figure 3. Heat map of significant differentially expressed genes. Horizontal axis stands for the sample name, and the vertical axis for gene name. Blue represents downregulated expression, and red represents up-regulated expression. Color of rectangular on the top represents sample properties, namely, dark blue for bone marrow negative samples and light blue for the bone marrow positive samples.
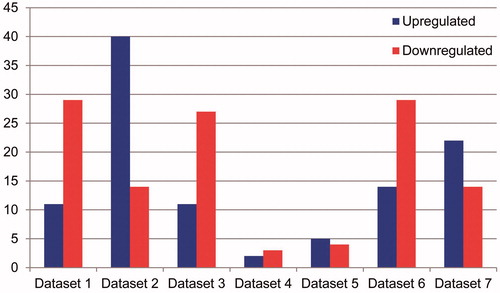
Table 1. Top ten of differentially expressed genes.
GO and pathway enrichment analysis
To determine the function of significant DEGs in bone metastases of lung cancer, the DEGs were mapped to the GO database. It was displayed that lysosome and lytic vacuole were the most enriched ones in GO term (). For further insights into the changes of the biological pathways, the significant pathways related with the DEGs were analyzed, including lysosome, antigen processing and presentation (, ). Lysosome were significantly enriched in both GO and KEGG pathway enrichment analysis. To integrate and visualize the enrichment pathway, the most significant enrichment pathway hsa04142 was mapped (). Some lysosomal enzymes were significantly downregulated, such as lysosomal acid hydrolase proteases (including cathepsins, napsin and LGMN), and ceramidase (including ASAH1). Furthermore, not only major lysosomal membrane proteins, LAMP and LIMP, but some minor lysosomal membrane proteins, NPC for example, were also significantly downregulated.
Figure 4. Top ten pathways by KEGG enrichment analysis. The abscissa represents the number of enrichment genes, and ordinate represents the enrichment pathway name. The color of the pillar represents the significance through KEGG enrichment analysis.
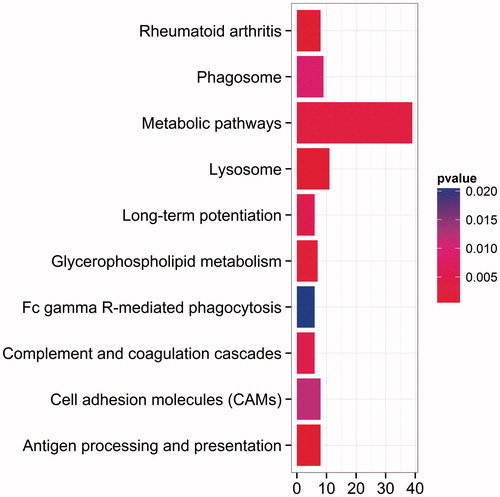
Figure 5. Pathway of hsa04142. Genes with color significant differentially expressed through functional enrichment analysis. Green box represents up-expressed gene, and red for down-expressed genes. log2 (FC) is shown in the upper right corner.
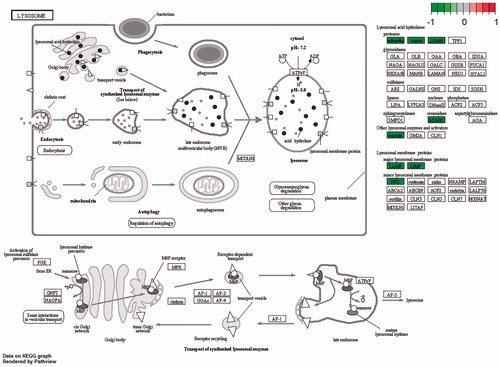
Table 2. GO enrichment analysis.
Table 3. Top ten pathways by KEGG enrichment analysis.
PPI network analysis
Score of interaction among different proteins was assessed and the results showed high relationship between proteins, including PPP3CA and CALML3, CTSS, SERPINB4, FGF13 and NKX2–1 (). Interactions among different proteins were complicated and crossed with each other (). Thereinto, cathepsin S (CTSS) had the most interaction proteins, which was followed by CTSD, MX1, and NKX2–1 (), which indicated that these proteins might be potential molecular markers of prediction and treatment on bone metastases in lung cancer.
Figure 6. Protein–protein interaction network. Balls represent proteins and line is on behalf of the interaction between genes. Different shape of line inside the balls represents the protein structures. Thread color represents different evidence to prove protein interactions. A red line stands for the presence of fusion evidence; a green line for neighborhood evidence; a blue line for co-occurrence evidence; a purple line for experimental evidence; a yellow line for text mining evidence; a light blue line for database evidence; a black line for co-expression evidence.
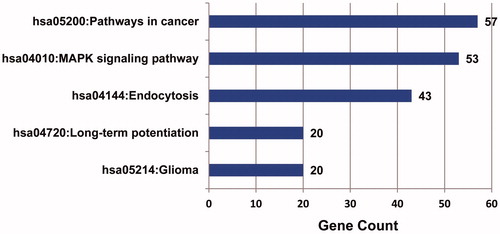
Figure 7. Number of proteins interaction with node protein. The abscissa represents the number of interaction protein, and ordinate represents the core gene name.
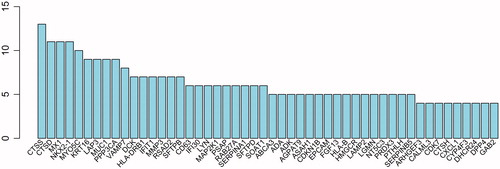
Table 4. Score of protein–protein interaction.
Discussion
Bone metastasis in lung cancer mostly resulted in osteolytic lesion, but small-cell undifferentiated carcinoma and adenocarcinoma lung cancer could lead to osteogenic destruction. Osteolytic bone metastasis induced by lung cancer was usually associated with enhancing osteoclast activity and decreasing osteoblast capacity, finally leading to bone resorption (Sims and Gooi Citation2008, Sterling et al. Citation2011). However, the biology of organ-specific metastasis was poorly understood, particularly in lung cancer bone metastases. To further understand the mechanism of bone metastases in lung cancer, expression data of patients with lung cancer were downloaded and analyzed, which might prove new insights into signature prediction and treatment of bone metastases in lung cancer.
Lysosomal alterations were common in cancer cells. In acute cell death, lysosomal hydrolases were released into the cytosol and digested endocytosed and autophagocytosed material inside the lysosomal/autolysosomal compartment, but in cancer progression following their release into the extracellular space. The upregulated expression and altered trafficking of lysosomal enzymes contributed to tissue invasion, angiogenesis and sensitization to the lysosomal death pathway (Kroemer and Jäättelä Citation2005). Reports showed that lysosomal enzymes in osteoclast could be released into the extracellular to decompose and eliminate the old bone matrix, which was an important step in the bone updates (Weber and Schilling Citation2014). In this study, pre-processed data were analyzed by GO and pathway enrichment analysis, and both the results pointed to lysosome. These results indicated that bone metastasis from lung cancer would lead to changes of lysosomal pathway, which might influence the old bone matrix decomposition and elimination, and finally affect the progression of bone updation. It was speculated that lysosome played an important role in the update of bone during the process of bone growth and reconstruction. These pathways might be a novel signature of prediction and strong evidence in bone metastasis study from lung cancer.
Core genes (CTSS, CTSD, MX1, NKX2–1) were obtained from the PPI network, which may be biological markers of bone metastases lung cancer. Cathepsin S (CTSS) was a cellular cysteine protease, which was frequently observed over-expressed in human cancer cells. Study revealed that CTSS interfered with the EGFR–ERK/MAPK-signaling pathway and significantly affected tumor metastasis, and regulated cell autophagy (Chen et al. Citation2012). Research on MUC1-CD-induced tumorigenesis showed that CTSS was associated with the activation of genes that functionally contributed to the growth of tumors in vivo (Khodarev et al. Citation2009). CTSS were also reported to play a critical role in gastric cancer cell migration and invasion, potentially by regulating the expression of a series of extracellular proteins involvement in cellular movement. And CTSS target genes were potential drug targets and might prove a combinatorial approach to adjuvant therapy and treatment of metastatic gastric cancers (Taleb et al. Citation2006). Herein, CTSS was significant differentially expressed in bone metastases in lung cancer. It was speculated that CTSS also played an important role in the lung cancer cell migration and invasion by regulating the expression of a series of extracellular proteins involvement in cellular movement. Therefore, CTSS possessing completed interaction with other proteins indicated that it might be a potential drug target and might prove a new approach to the therapy and treatment of bone metastasis in lung cancers.
Gene cathepsin D (CTSD) coding ubiquitous lysosomal aspartyl protease was over-expressed in aggressive human breast cancers. The high association of CTSD with other proteins was consistent with results of functional enrichment analysis in which lysosome was the most differentially expressed. Transcription of CTSD was induced by estrogens in hormone-responsive breast cancer cells (Cavailles et al. Citation1993). To investigate the role of this protease in breast cancer growth and progression to metastasis, transfecting a highly metastatic human breast cancer cell line demonstrated that cathepsin-D facilitates tumorigenesis and metastasis of breast cancer cells (Glondu et al. Citation2002). The demethylation incidence in CTSD enhancer was highly related to metastasis of breast cancer (Li and Tang Citation2011). In present research, CTSD acting as a core protein demonstrated its important role in bone metastasis from lung cancer. It is speculated that CTSD facilitated tumorigenesis and metastasis of lung cancer cells in bone, indicating CTSD gene a prognostic indicator of metastases bone lung cancer.
NKX2–1, also known as TITF1 and TTF-1, was a lineage-survival oncogene in lung adenocarcinomas. Reports showed that NKX2–1 induced the expression of the receptor tyrosine kinase-like orphan receptor 1 (ROR1), which in turn sustained a favorable balance between prosurvival PI3K-AKT and pro-apoptotic p38 signaling (Yamaguchi et al. Citation2012). What’s more, cross-species analysis identified the transcription factor Nkx2–1 as a candidate suppressor of malignant progression. Nkx2–1 in the same tumor type substantiated its dual function lineage factor, the oncogenic and suppressive functions (Winslow et al. Citation2011). Studies have reported that MX1 played important role in the bone marrow stromal cells (Walkley et al. Citation2007), and closely related with bone growth and differentiation (Park et al. Citation2012). In this study, complex relationship with the other proteins of MX1 and Nkx2–1 manifested that they were in the focus in biology process of suppress and promotion of malignant progression, which indicated that they might be prognostic markers of metastases bone lung cancer.
In summary, to better understand the molecular biological mechanisms of bone metastases in lung cancer, microarray database was downloaded and analyzed. Finally, a total of 390 DEGs were obtained. Functional enrichment analysis showed that lysosome was enriched in both GO and pathway enrichment analysis, indicating its highly correlation with bone metastases in lung cancer. What’s more, PPI network based on differentially expressed genes were constructed and four core proteins were obtained, which might act as potential biological makers and play a critical role in bone metastasis. Collectively, these results proved new insights into the prediction and treatment of bone metastases in lung cancer.
supplement_table_1.xlsx
Download MS Excel (60.5 KB)Disclosure statement
All authors declared no conflict of interest.
References
- Brown JE, Cook RJ, Major P, Lipton A, Saad F, Smith M, et al. 2005. Bone turnover markers as predictors of skeletal complications in prostate cancer, lung cancer, and other solid tumors. J Natl Cancer Inst. 97:59–69.
- Cavailles V, Augereau P, Rochefort H. 1993. Cathepsin D gene is controlled by a mixed promoter, and estrogens stimulate only TATA-dependent transcription in breast cancer cells. Proc Natl Acad Sci. 90:203–207.
- Chen K-L, Chang W-SW, Cheung CHA, Lin C-C, Huang C-C, Yang Y-N, et al. 2012. Targeting cathepsin S induces tumor cell autophagy via the EGFR–ERK signaling pathway. Cancer Lett. 317:89–98.
- Coleman RE. 1997. Skeletal complications of malignancy. Cancer. 80:1588–1594.
- Dennis G Jr, Sherman BT, Hosack DA, Yang J, Gao W, Lane HC, et al. 2003. DAVID: database for annotation, visualization, and integrated discovery. Genome Biol. 4:P3.
- Franceschini A, Szklarczyk D, Frankild S, Kuhn M, Simonovic M, Roth A, et al. 2013. STRING v9. 1: protein-protein interaction networks, with increased coverage and integration. Nucleic Acids Res. 41:D808–DD15.
- Gautier L, Cope L, Bolstad BM, Irizarry RA. 2004. affy—analysis of affymetrix genechip data at the probe level. Bioinformatics. 20:307–315.
- Glondu M, Liaudet-Coopman E, Derocq D, Platet N, Rochefort H, Garcia M. 2002. Down-regulation of cathepsin-D expression by antisense gene transfer inhibits tumor growth and experimental lung metastasis of human breast cancer cells. Oncogene. 21:5127–5134.
- James J, Evans A, Pinder S, Gutteridge E, Cheung K, Chan S, et al. 2003. Bone metastases from breast carcinoma: histopathological - radiological correlations and prognostic features. Br J Cancer. 89:660–665.
- Ji P, Diederichs S, Wang W, Boing S, Metzger R, Schneider PM, et al. 2003. MALAT-1, a novel noncoding RNA, and thymosin beta4 predict metastasis and survival in early-stage non-small cell lung cancer. Oncogene. 22:8031–8041.
- Khodarev NN, Pitroda SP, Beckett MA, MacDermed DM, Huang L, Kufe DW, et al. 2009. MUC1-induced transcriptional programs associated with tumorigenesis predict outcome in breast and lung cancer. Cancer Res. 69:2833–2837.
- Koenders P, Beex L, Langens R, Kloppenborg P, Smals A, Benraad TJ, et al. 1991. Steroid hormone receptor activity of primary human breast cancer and pattern of first metastasis. The Breast Cancer Study Group. Breast Cancer Res Treat. 18:27–32.
- Kroemer G, Jäättelä M. 2005. Lysosomes and autophagy in cell death control. Nat Rev Cancer. 5:886–897.
- Li L, Tang J-H. 2011. Epigenetic modification of CTSD gene with breast cancer. Acta Universitatis Medicinalis Nanjing (Natural Science). 8:023.
- Luo W, Brouwer C. 2013. Pathview: an R/Bioconductor package for pathway-based data integration and visualization. Bioinformatics. 29:1830–1831.
- Mehrotra J, Vali M, McVeigh M, Kominsky SL, Fackler MJ, Lahti-Domenici J, et al. 2004. Very high frequency of hypermethylated genes in breast cancer metastasis to the bone, brain, and lung. Clin Cancer Res. 10:3104–3109.
- Park D, Spencer JA, Koh BI, Kobayashi T, Fujisaki J, Clemens TL, et al. 2012. Endogenous bone marrow MSCs are dynamic, fate-restricted participants in bone maintenance and regeneration. Cell Stem Cell. 10:259–272.
- Parkin DM, Bray F, Ferlay J, Pisani P. 2005. Global cancer statistics, 2002. CA Cancer J Clin. 55:74–108.
- Roodman GD. 2004. Mechanisms of bone metastasis. N Engl J Med. 350:1655–1664.
- Rove KO, Crawford ED. 2009. Metastatic cancer in solid tumors and clinical outcome: skeletal-related events. Oncology (Williston Park, N.Y.). 23:21.
- Sims NA, Gooi JH. 2008. Bone Remodeling: Multiple Cellular Interactions Required for Coupling of Bone Formation and Resorption. Seminars in Cell & Developmental Biology. Amsterdam, The Netherlands: Elsevier.
- Smyth GK. 2005. Limma: linear models for microarray data. Bioinformatics and Computational Biology Solutions Using R And Bioconductor. London, United Kingdom: Springer. pp. 397–420.
- Sterling JA, Edwards JR, Martin TJ, Mundy GR. 2011. Advances in the biology of bone metastasis: how the skeleton affects tumor behavior. Bone. 48:6–15.
- Taleb S, Cancello R, Poitou C, Rouault C, Sellam P, Levy P, et al. 2006. Weight loss reduces adipose tissue cathepsin S and its circulating levels in morbidly obese women. J Clin Endocrinol Metab. 91:1042–1047.
- Valencia K, Ormazabal C, Zandueta C, Luis-Ravelo D, Anton I, Pajares MJ, et al. 2012. Inhibition of collagen receptor discoidin domain receptor-1 (DDR1) reduces cell survival, homing, and colonization in lung cancer bone metastasis. Clin Cancer Res. 18:969–980.
- Walkley CR, Shea JM, Sims NA, Purton LE, Orkin SH. 2007. Rb regulates interactions between hematopoietic stem cells and their bone marrow microenvironment. Cell. 129:1081–1095.
- Weber K, Schilling JD. 2014. Distinct lysosome phenotypes influence inflammatory function in peritoneal and bone marrow-derived macrophages. Int J Inflam. 2014. doi:10.1155/2014/154936.
- Weiss RJ, Wedin R. 2011. Surgery for skeletal metastases in lung cancer: complications and survival in 98 patients. Acta Orthopaedica. 82:96–101.
- Winslow MM, Dayton TL, Verhaak RG, Kim-Kiselak C, Snyder EL, Feldser DM, et al. 2011. Suppression of lung adenocarcinoma progression by Nkx2-1. Nature. 473:101–104.
- Yamaguchi T, Yanagisawa K, Sugiyama R, Hosono Y, Shimada Y, Arima C, et al. 2012. NKX2-1/TITF1/TTF-1-Induced ROR1 is required to sustain EGFR survival signaling in lung adenocarcinoma. Cancer Cell. 21:348–361.
- Yu G, Wang L-G, Han Y, He Q-Y. 2012. clusterProfiler: an R package for comparing biological themes among gene clusters. OMICS. 16:284–287.