Abstract
The outcomes of Lung squamous cell carcinoma (LUSC) is still challenging to evaluate or predict. We aimed to screen prognostic lncRNAs and to mine their roles in LUSC. RNA-Seq data of primary lung cancer were extracted from the Cancer Genome Atlas. Generally, changed lncRNAs in cancer samples were screened and analyzed in univariate survival analysis for identification of prognostic lncRNAs. Robust likelihood-based survival model was generated and random sampling iterations were performed 1000 times to calculate the frequency of feature key lncRNAs. Clustering and multivariate survival analysis of these lncRNAs was used to evaluate their functions and impacts on prognosis. Finally, the stability and validity of the optimal clustering model were verified. In total, we obtained 5664 generally changed lncRNAs among primary lung cancer samples, including 289 identified relating to prognosis in univariate survival analysis. Robust likelihood-based survival modelling for 1000 iterations generated 11 feature lncRNAs with frequency larger than 300. Their interacting proteins were found participating in DNA repairing and cell proliferation. Among stable assembly of 11 lncRNAs, a 4-lncRNA model was selected finally with high stability and feasibility. The ideal 4-lncRNA model can cluster patient samples with significant difference, providing new avenues for the prognostic predication of LUSC.
Introduction
Lung and bronchus cancer leads the death amount of all kinds of cancers (27% death in male and 22% in female). Annually, the estimated new cases are more than 25,000 all over the world, ranking the second in cancer [Citation1,Citation2]. Based on data from SEER 18 2007–2013 [Citation3], the 5-year surviving percent is only 18.1%. Lung squamous cell carcinoma (LUSC) is a type of cancer that begins in squamous cells which generally exist in the tissue that forms the lining of the respiratory, accounting for about 30% of non-small cell lung cancer (NSCLC) [Citation4]. Thus, LUSC is as well one of the common pathologic subtypes of lung cancer, constituting a vast amount of diagnosed cases [Citation5,Citation6].
Patients with LUSC are always diagnosed in late stage when current available treatment cannot be implemented in time [Citation7]. Moreover, to the treatments by radiotherapy and chemotherapy, LUSC patients are not as sensitive as small cell cancer patients. And carcinoma cells, in variable shapes in LUSC, often penetrate into the adjacent tissue without definite range. In a way, this contributes to a high reoccurrence of LUSC, making the prognostic outcomes more difficult to evaluate. Taken together, essential prediction to prognosis and timely surgical resection are still essential to improve the survival rate of LUSC.
Based on the principle of “early diagnosis and early treatment”, a lot of research is focusing on the methods and/or markers for early diagnosis or even prediction. In recent years, it has been confirmed that the occurrence of LUSC is closely related to the expressions of many oncogenes and tumour suppressor genes, or structural abnormality of their products. Specifically, the subtypes being studied by TCGA researchers are called lung adenocarcinoma and lung squamous cell carcinoma [Citation8].
Several genes and proteins have already been identified related to squamous cell lung carcinoma. Thereof, some related genes were used to distinguish LUAD from LUSC, where the expression of these genes (e.g. MLPH, TMC5, SFTA3 and CALML3) in lung cancer sections were used with immunohisto-chemical staining [Citation9]. In addition, research focused on biomarker identification reported some genes (e.g. c-erbB-2) for prognostic indicator [Citation10]; as well as squamous differentiation genes and pathways (e.g. NFE2L2 and KEAP1) as potential therapeutic target [Citation11]. The study of the development and progression of LUSC has been studied deeply at the molecular level. In particular, except for the genes involved in the diagnosis and treatment of LUSC, it is still important to study genes and/or pathways that can be referred to evaluate or predict the prognostic outcomes of LUSC.
Recently, more research tends to explore the interacting regulatory RNAs. Although the definition of long non-coding RNAs (lncRNA) is non-protein coding transcripts longer than 200 nucleotides [Citation12], some lncRNAs do encode proteins and play roles in transcriptional and epigenetic gene regulation, and cancer [Citation13,Citation14].
In the present study, RNA-Seq data of primary lung cancer were downloaded on the TCGA to screen out feature lncRNAs related to prognosis. Robust likelihood-based survival model was generated and the frequency of feature lncRNAs was calculated in random sampling iterations. Finally, 11 feature lncRNAs with frequency larger than 300 were selected, of which a 4-lncRNA model was selected finally with high stability and feasibility. With this model, patients with at least four of these lncRNAs differentially expressed can be predicted as high risk with LUSC. The ideal 4-lncRNA model can cluster patient samples with significant difference. It might provide new reference to evaluate the outcomes of patients with LUSC, providing avenues for the prognostic predication of LUSC.
Materials and methods
Data source and preprocessing
The database Cancer Genome Atlas (TCGA, https://tcga-data.nci.nih.gov/tcga/), generating multimodal genomics data for thousands of tumour samples covering more than 30 types of cancer, was used in the present study [Citation15]. All the RNA-Seq data of lung cancer by the time of 5th April 2017 were downloaded from TCGA. There were 502 lung squamous carcinoma samples in total, including 450 primary lung squamous carcinoma samples. The long non-coding RNAs (lncRNAs) of these primary samples were applied for analysis. Standardization of the level of lncRNA expression for each sample was normalized with median quantification. Besides, as for the numerical distribution of Reads per Kilo-base per Million mapped (RPKM) reads are too wide to be used in model analysis, we formulated each RPKM value in log2 (X + 1). X is the RPKM value.
Preliminary screening of generally changed lncRNAs
The different levels of lncRNA expressions lead to the various prognostic risks and outcomes from the same cancer type of different patients. Therefore, we firstly screened the lncRNAs with distinctive expressions among different patients of primary lung cancer. A, representing a generally changed lncRNA, was selected basing on the following rules:
The median expression level of A in every sample was more than 20% of the total median expressions of all lncRNAs in every sample;
The variance of expression level of A in every sample was higher than 20% of the total expression variances of all lncRNAs in every sample.
Selection of seed lncRNAs
Survival analysis is an inference to study the survival time and outcome, as well as the relationship and extent between the numbers of influencing factors. All the selected lncRNAs with significant changes among patient samples were analyzed in univariate survival analysis by using Survival in R [Citation16]. lncRNAs with expressing significance p values <.05 were selected as seed lncRNAs.
Identification of key lncRNAs relating to prognosis
The seed lncRNAs in primary selection are not suitable for clinical diagnosis. Therefore, we constructed Robust likelihood-based survival models to identify the key lncRNAs influencing the prognosis of this cancer by using rbsurv in R language [Citation17–19]. The procedure was as followings:
The samples were randomly divided into the training set with N*(1 − p) samples and the validation set with N*p samples, with p = 1/3. We fitted a gene to the training set of patient samples and then the parameter estimate for this gene was obtained. Then we evaluated log likelihood with the parameter estimate and the validation set of samples. This evaluation was repeated for each gene.
We repeated the above procedure 10 times, obtaining 10 log likelihoods for each gene. The best gene, g (1), with the largest mean log likelihood was selected. Subsequently, we searched the next best gene by evaluating every two-gene model and selected an optimal one with the largest mean log likelihood.
We continued this forward gene selection procedure, resulting in a series of models. Akaike’s information criterions (AICs) for all the candidate models were computed and an optimal model with the smallest AIC was selected finally.
We randomly selected more than half of the samples for a thousand times, the Robust likelihood-based survival model was applied for each time of the survival analysis. The lncRNAs with highest frequency were selected the final feature lncRNA.
Interdependency of key prognostic lncRNAs
Via searching the database starbase2.0, the proteins interacting with these key prognostic lncRNAs was identified. The lncRNA-protein net-work was constructed and analyzed. Then these interacting proteins and lncRNAs were annotated by using the Database for Annotation, Visualization and Integrated Discovery (DAVID) [Citation20] on both KEGG (Kyoto Encyclopaedia of Genes and Genomes) pathways and Gene Ontology (GO) terms. The threshold was p < .05.
Multivariate survival analysis and the hazard ratio of the feature lncRNAs
To figure out how the whole lncRNAs impacting the prognosis of patient samples, multivariate survival analysis was performed on the seed lncRNAs by using survivalROC in R Language [Citation21]. The area under the curve (AUC) values for seed lncRNA was generated then.
According to the expression profile of feature lncRNAs, the hazard ratio (HR) of each lncRNA was obtained in univariate survival analysis. The HR of each lncRNA was calculated in the Cox-model (Cox semiparametric proportional hazards model) [Citation22,Citation23] with the following formula: HR = h1(t)/h2(t); h1 is the hazard ratio of exposure group, h2 is the hazard ratio of non-exposure group, t represents the same time point.
Clustering analysis of feature lncRNAs
The hazard ratios (HR) of each lncRNA, together with the expression profile of the feature lncRNA were considered as references to assort the patient samples with poor prognosis.
One risk factor for poor prognosis would be counted when patient was identified with the expression >80th percentile (HR >1) or/and the expression <20th percentile (HR <1). Calculating the numbers of the risk factors of every patient, the patients were then classified according to the risk factors (≥1, ≥2, ≥3…).
Prognostic impacts of the models in univariate analysis
Kaplan–Meier univariate survival analysis on the clustering algorithm from each regression model was performed to calculate the impacts to the prognosis of primary lung cancer. Repetitive training of random samples in each model (samples contain at least a death event) to verify the model’s stability. Random samples were analyzed in stepwise multivariate and univariate survival analysis for one thousand times to calculate statistical stability of each regression model.
According to each regression model, patients were clustered into different classifications basing on the expression of the linkage model. Univariate survival analysis with Kaplan–Meier method of each regression model was performed to calculate the significance of the impacts to the prognosis of primary lung cancer. Finally, the ideal clustering model was selected to predict the prognosis of lung cancer.
Stability and validity verification
Patient samples (samples contain at least one death event) were randomly drew to perform survival and clustering analysis. The significances of the prognostic cluster models were tested for 1000 iterations. Statistical stability under each cluster model was evaluated.
To test validity of the cluster models on other classification methods, these, patient samples were classified as well according to tumour node metastasis (TNM). Comparing with the outcomes of these two classifications, the validity of the clustering algorithm was verified and figured out.
Results
Data source and preprocessing
A total of 11907 RNA-Seq data profiles were obtained from 450 patient samples with lung squamous carcinoma.
Screening of significantly changed lncRNAs
Totally, 5664 generally changed lncRNAs were screened out of 11907 lncRNAs. The significance of the prognostic impact of every lncRNA was evaluated in the univariate survival analysis. Following the threshold of p < .05, there were 289 significantly changed lncRNAs (S1). The top 20 significantly changed lncRNAs were selected as well for further analysis ().
Table 1. The top 20 differentially expressed lncRNAs.
Identification of key lncRNAs relating to prognosis
Random data analysis was performed by using Robust likelihood-based modelling for 1000 times. Statistic frequency analysis of the significantly changed lncRNAs demonstrated that all the selected lncRNAs had a high frequency (). In further analysis, differentially expressed lncRNAs with frequency more than 300 were selected. And there were 11 lncRNAs identified as prognostic feature ().
Table 2. Significantly changed lncRNAs with frequency more than 300.
Interdependency of key prognostic lncRNAs
Proteins interacting with these 11 lncRNAs were searched in starbase2.0 (). In this lncRNA-protein net-work, five lncRNAs interacted with seven proteins. Hereinto, most of the proteins associated with CTD-3099C6.9. These proteins were further annotated by using DAVID. In GO term, we obtained annotations of four proteins, including DNA repairing and cell proliferation. This indicated that the identified lncRNAs, especially CTD-3099C6.9, related with the occurrence and development of primary lung squamous carcinoma.
Multivariate survival analysis and the hazard ratio of the feature lncRNAs
In the multivariate survival analysis, AUC values for eleven seed lncRNAs were all above 0.7 (). The whole lncRNAs impacting the prognosis could cluster these patient samples well in the multivariate survival analysis.
Clustering analysis of feature lncRNAs
The significance analysis and Risk Hazard results of 11 lncRNAs in univariate survival analysis were listed in . It is obvious that all these significances were all significant with p < .01. Based on the clustering model, lncRNAs would be marked with 1 when evaluated as risk factor; otherwise, the lncRNA would be marked with 0.
Table 3. Significant analysis and HR of seed lncRNAs.
Prognostic impacts of the models in univariate analysis
Then, according to the scores of risk factors, patient samples were clustered as lncRNA ≥1, ≥2, ≥3, ≥4… Meanwhile, Kaplan–Meier univariate survival analysis was used to evaluate the significant p values of every clustering method (). It was indicated that clustering method of ≥4-lncRNAs was able to classify samples with significant prognostic difference.
Random samples were analyzed in repeating univariate survival analysis for one thousand times to calculate statistical stability of each regression model. In , significant p values of all regression model in clustering were less than .01. Finally, the ideal clustering model was ≥4-LncRNA with good stability to predict the prognosis of lung cancer.
Stability and validity
To test the validity of the model used in different subtypes of LUSC, clinic samples were classified again basing on TNM classification system. In , patient samples were classified into different subtypes T1, T2, T3 + 4, N0, N1, N2 + 3, M0, Stage I, Stage II and Stage III. Then every clustering model was applied to verify their influence and association with prognosis (). Each model has a good classification result for most of the subtypes, especially for Stage I, Stage II, T2, N0 and M0. As to the stability, the ≥4-LncRNA was selected as ideal clustering model due to its good stability and robustness.
Figure 6. Kaplan–Meier survival analysis of different clustering algorithm. Each square is the risk score of clustering algorithm of 11 lncRNAs. Domains in red (marked with stars) represent the significant influences on the prognoses with p value < .05. The right ordinate is the gene model of each gene group; the horizontal ordinate is the classification according to the TNM.
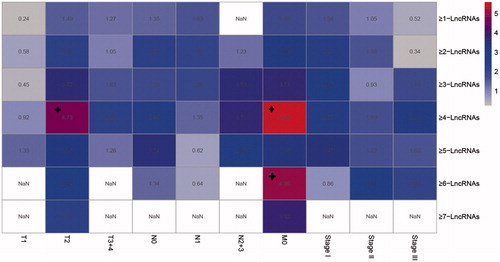
Table 4. Sample counting of TNM.
Discussion
Lung cancer, with the highest mortality rate, has been hot spots and difficulties in the field of cancer research. According to pathology or histology, lung cancer is divided into non-small cell lung cancer (NSCLC) and small cell lung cancer. NSCLC accounts for about 85% of the total number of lung cancer patients [Citation24], while squamous cell carcinoma (LUSC) accounts for more than 30% of NSCLC. It has been a problem not only in the early diagnosis but also in the prognostic evaluation, which leads to a low 5-year survival rate of patients with LUSC [Citation25].
To provide prognostic genes or models to predict the outcomes of LUSC patients, this study leveraged recently available RNA-seq data of primary NSCLC tumours from database TCGA. A total of 11 lncRNAs (AC064853.2, AC090044.2, CTD-3099C6.9, KB-1836B5.4, OTX2-AS1, RP11–108M12.3, RP11–506H20.1, RP11–535M15.1, RP11–547C5.1, RP11–739N20.2 and RP11–76C10.5) were identified with significant impacts on the prognosis of LUSC. Meanwhile, proteins interacting with theses lncRNAs were mainly in cell nucleus. Herein, most of the proteins (FMRP, LIN28B, IGF2BP2, eIF4AIII, UPF1, PTB) were found interacted with lncRNA CTD-3099C6.9, participating in DNA repair and cell proliferation. In a previous study on the expression of lncRNA in human primary lung fibroblasts (IMR-90), CTD-3099C6.9 was also identified interacting with RNA binding proteins [Citation26], consistent with our research. This indicated that this lncRNA might be related to the occurrence and development of this tumour as well.
Among these 11 lncRNAs, except for OTX2-AS1, all other lncRNAs were the first time to be discovered differentially expressed in cancer samples. In previous study, orthodenticle homeobox 2 antisense RNA 1 (OTX2-AS1) was reported as novel lncRNAs enriched in urine from patients with urothelial bladder cancer [Citation27] and cervical cancer [Citation28]. Because the correlation study of lncRNA and tumours are burgeoning field, research for lncRNAs as biomarkers of lung cancer are still in the initial stage, let alone those for LUSC. To date, key oncogenic alterations have been identified in numerous small subsets of NSCLC, either for pathway-based systemic therapy or for molecular-based approaches [Citation29,Citation30]. There is still a shortage of biomarker resource which can be used to analyze the prognosis of LUSC. Many reports have revealed lncRNA signature related with the survival of patients with oesophageal [Citation31,Citation32], tongue [Citation33] and oral [Citation34] squamous cell carcinoma rather than lung squamous cell carcinoma. Therefore, the present study yields novel insight into the prognostic lncRNAs in LUSC.
To evaluat the outcomes of a treatment or the prognosis of cancer patients, the TNM (tumour-node-metastasis) classification system has been a vital guide since 1978 [Citation35,Citation36]. This system can give a good indication of prognosis, however, more accurate classification within one stage of lung cancer remains a challenging task [Citation37]. Thus, this research aims to figure out prognosis associating lncRNAs with significant expressing difference. Clustering with these key lncRNAs might be more sensitive than basing on the size and extension of the primary tumour, its lymphatic involvement and the presence of metastases [Citation38]. In the present study, we constructed models to classify the patients with LUSC.
Seven assembly of the 11 lncRNAs (≥1 LncRNA, ≥2 LncRNA, … ≥7 LncRNA) can in different levels cluster patient samples into prognostic classifications basing on high and low risk. Besides, by using TNM system, these patient samples were classified into different subtypes (T1, T2, T3 + 4, N0, N1, N2 + 3, M0, Stage I, Stage II and Stage III). Seven assembly of the 11 lncRNAs (≥1 LncRNA, ≥2 LncRNA, … ≥7 LncRNA) can in different levels cluster patient samples into prognostic classifications basing on high and low risk. It can be seen from the figure that each model has a good classification result for most of the subtypes, especially for Stage I, Stage II, T2, N0 and M0. Herein, the ≥4-LncRNA was selected as ideal clustering model due to its good stability and robustness.
In conclusion, we figured out 289 lncRNAs totally relating to prognosis from 5664 generally changed lncRNAs among primary lung cancer samples. From these lncRNAs, we then selected 11 feature lncRNAs with frequency larger than 300. Their interacting proteins were found participating in DNA repairing and cell proliferation. Among stable assembly of 11 lncRNAs, a 4-lncRNA model was selected finally with high stability and facticity. This is the pioneering research to identify lncRNA influencing the prognosis of LUSC and to model an approach to cluster the patients in different subtypes of TNM system. In addition, the discovery also provides new insights for the prognostic predication of LUSC. However, for clinic utilization and evaluating the prognostic value of our model, an integrative analysis combining the expression data and other variation data will be carried out in further studies.
Disclosure statement
All authors declared no conflict of interest.
References
- DeSantis CE, Siegel RL, Sauer AG, et al. Cancer Statistics for African Americans, 2016: Progress and opportunities in reducing racial disparities. CA: a cancer journal for clinicians; 2016.
- Rahib L, Smith BD, Aizenberg R, et al. Projecting cancer incidence and deaths to 2030: the unexpected burden of thyroid, liver, and pancreas cancers in the United States. Cancer Res. 2014;74:2913–2921.
- Mayer JE, Swetter SM, Fu T, et al. Screening, early detection, education, and trends for melanoma: current status (2007–2013) and future directions: Part I. Epidemiology, high-risk groups, clinical strategies, and diagnostic technology. J Am Acad Dermatol. 2014;71:599.e1–599.e12.
- Choi M, Kadara H, Zhang J, et al. Mutation and immune profiles in early-stage lung squamous cell carcinoma. San Diego (CA): AACR; 2016.
- Travis WD, Rekhtman N, Riley GJ, et al. Pathologic diagnosis of advanced lung cancer based on small biopsies and cytology: a paradigm shift. J Thorac Oncol. 2010;5:411–414.
- Travis WD, Brambilla E, Noguchi M, et al. International association for the study of lung cancer/american thoracic society/european respiratory society international multidisciplinary classification of lung adenocarcinoma. J Thorac Oncol. 2011;6:244–285.
- Schwartz AM, Rezaei MK. Diagnostic surgical pathology in lung cancer: diagnosis and management of lung cancer: American College of Chest Physicians evidence-based clinical practice guidelines. CHEST J. 2013;143(5_suppl):e251S–e262S.
- OHara AJ, Keshavan R, Che Z, et al. An integrated comparative analysis of TCGA lung adenocarcioma and lung squamous cell carcinoma copy number and RNA-Seq expression data. San Diego (CA): AACR; 2015.
- Terry J, Leung S, Laskin J, et al. Optimal immunohistochemical markers for distinguishing lung adenocarcinomas from squamous cell carcinomas in small tumor samples. Am J Surg Pathol. 2010;34:1805–1811.
- Tateishi M, Ishida T, Mitsudomi T, et al. Prognostic value of c-erbB-2 protein expression in human lung adenocarcinoma and squamous cell carcinoma. Eur J Cancer Clin Oncol. 1991;27:1372–1375.
- Network CGAR. Comprehensive genomic characterization of squamous cell lung cancers. Nature. 2012;489:519–525.
- Mercer TR, Dinger ME, Mattick JS. Long non-coding RNAs: insights into functions. Nat Rev Genet. 2009;10:155–159.
- Morris KV, Mattick JS. The rise of regulatory RNA. Nat Rev Genet. 2014;15:423–437.
- Ponting CP, Oliver PL, Reik W. Evolution and functions of long noncoding RNAs. Cell. 2009;136:629–641.
- Zhao Q, Shi X, Xie Y, et al. Combining multidimensional genomic measurements for predicting cancer prognosis: observations from TCGA. Brief Bioinform. 2015;16(2):291.
- O’Quigley J, Moreau T. Cox’s regression model: computing a goodness of fit statistic. Comput Methods Programs Biomed. 1986;22:253–256.
- Wang JY, Tai J. Robust quantitative trait association tests in the parent‐offspring triad design: conditional likelihood‐based approaches. Ann Hum Genet. 2009;73:231–244.
- Renaud G, Stenzel U, Maricic T, et al. deML: robust demultiplexing of Illumina sequences using a likelihood-based approach. Bioinformatics. 2015;31:770–772.
- Kendall WL, Pollock KH, Brownie C. A likelihood-based approach to capture-recapture estimation of demographic parameters under the robust design. Biometrics. 1995;293–308.
- Dennis G, Sherman BT, Hosack DA, et al. DAVID: database for annotation, visualization, and integrated discovery. Genome Biol. 2003;51(1):293–308.
- Heagerty PJ, Lumley T, Pepe MS. Time-dependent ROC curves for censored survival data and a diagnostic marker. Biometrics. 2000;56:337–344.
- Spruance SL, Reid JE, Grace M, et al. Hazard ratio in clinical trials. Antimicrob Agents Chemother. 2004;48:2787–2792.
- Cox DR. Regression models and life-tables. In: Kotz S, Johnson NL, editors. Breakthroughs in statistics. vol. 34. New York (NY): Springer; 1992. p. 527–541.
- Beland MD, Wasser EJ, Mayo-Smith WW, et al. Primary non-small cell lung cancer: review of frequency, location, and time of recurrence after radiofrequency ablation. Radiology. 2009;254:301–307.
- Torre LA, Bray F, Siegel RL, et al. Global cancer statistics, 2012. CA: Cancer J Clin. 2015;65:87–108.
- Chen M, Zhao H, Lind SB, et al. Data on the expression of cellular lncRNAs in human adenovirus infected cells. Data Brief. 2016;8:1263–1279.
- Berrondo C, Flax J, Kucherov V, et al. Expression of the long non-coding RNA HOTAIR correlates with disease progression in bladder cancer and is contained in bladder cancer patient urinary exosomes. PLoS One. 2016;11:e0147236.
- Iden M, Fye S, Huang Y, et al. HPV integration and methylation patterns in cervical intraepithelial neoplasia and invasive cervical cancer via HPV capture and high-throughput sequencing. Annual Meeting of the American Association for Cancer Research. San Diego (CA): AACR; 2014.
- Besse B, Adjei A, Baas P, et al. 2nd ESMO Consensus Conference on Lung Cancer: non-small-cell lung cancer first-line/second and further lines of treatment in advanced disease. Ann Oncol. 2014;25:1475–1484.
- Reck M, Popat S, Reinmuth N, et al. Metastatic non-small-cell lung cancer (NSCLC): ESMO Clinical Practice Guidelines for diagnosis, treatment and follow-up. Ann Oncol. 2014;23:56–64.
- Li J, Chen Z, Tian L, et al. LncRNA profile study reveals a three-lncRNA signature associated with the survival of patients with oesophageal squamous cell carcinoma. Gut. 2014;63(11):1700.
- Chen FJ, Sun M, Li SQ, et al. Upregulation of the long non‐coding rna hotair promotes esophageal squamous cell carcinoma metastasis and poor prognosis. Mol Carcinog. 2013;52:908–915.
- Jia LF, Wei SB, Gan YH, et al. Expression, regulation and roles of miR‐26a and MEG3 in tongue squamous cell carcinoma. Int J Cancer. 2014;135:2282–2293.
- Tang H, Wu Z, Zhang J, et al. Salivary lncRNA as a potential marker for oral squamous cell carcinoma diagnosis. Mol Med Rep. 2013;7:761–766.
- Sobin LH, Fleming ID. TNM classification of malignant tumors, fifth edition (1997). Cancer. 1997;80:1803–1804.
- Edge SB, Compton CC. The American Joint Committee on Cancer: the 7th edition of the AJCC cancer staging manual and the future of TNM. Ann Surg Oncol. 2010;17:1471–1474.
- Lababede O, Meziane MA, Rice TW. TNM staging of lung cancer: a quick reference chart. Chest. 1999;115:233–235.
- Ménoret M. The genesis of the notion of stages in oncology: the French permanent cancer survey (1943–1952). Soc Hist Med. 2002;15:291–302.