Abstract
We intended to identify favourable metabolite(s) and pharmacological mechanism(s) of gut microbiota (GM) for liver regeneration (LR) through network pharmacology. We utilized the gutMGene database to obtain metabolites of GM, and targets associated with metabolites as well as LR-related targets were identified using public databases. Furthermore, we performed a molecular docking assay on the active metabolite(s) and target(s) to verify the network pharmacological concept. We mined a total of 208 metabolites in the gutMGene database and selected 668 targets from the SEA (1,256 targets) and STP (947 targets) databases. Finally, 13 targets were identified between 61 targets and the gutMGene database (243 targets). Protein–protein interaction network analysis showed that AKT1 is a hub target correlated with 12 additional targets. In this study, we describe the potential microbe from the microbiota (E. coli), chemokine signalling pathway, AKT1 and myricetin that accelerate LR, providing scientific evidence for further clinical trials.
Graphical Abstract
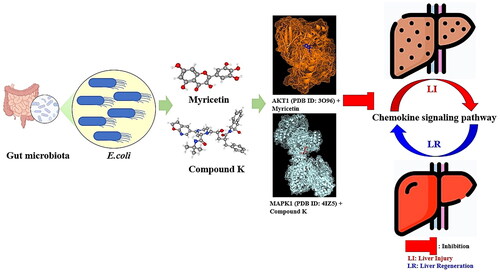
Introduction
Liver regeneration (LR) is a critical process to reorganize tissue and is the most significant process associated with relapse after liver injury [Citation1]. The risk attributed to loss of hepatocytes results in liver fibrosis, liver cirrhosis, liver failure and even liver cancer [Citation2,Citation3]. These stages are causative factors that increase the mortality rate, which leads to the diverse pathological spectrum ranging from atherosclerosis and diabetes mellitus [Citation4]. This finding suggests that regeneration in liver injury is the uppermost curative process to dampen the development of other unexpected complications. Additionally, a report showed that the main markers of LR include hepatocyte growth factor (HGF), interleukin-6 (IL-6) and vascular endothelial growth factor (VEGF), which are crucial components influencing on hepatocyte proliferation [Citation5].
These factors are representative endogenous components in liver injury as well as main elements in the recovery of function, suggesting that the endogenous targets might represent key regulators that accelerate LR.
In probiotics, a report showed that the diversity of gut microbiota (GM) produces dynamic effects on its recovery rate during LR after surgical operation of liver as well as GM regulation under antibiotics or probiotics [Citation6]. In particular, optimal colonization of the GM is essential to maintain healthy liver conditions, whereas gut dysbiosis leads to chronic liver diseases [Citation7]. In detail, gut microbial dysbiosis has negative effects on hepatic functionality; therefore, metabolites detected in serum have been altered due to biased gut microbial reserves [Citation8]. Dysbacteriosis results in a lack of natural killer (NK) T cells, which are vulnerable to acute liver injury [Citation9]. An animal study demonstrated that a reduced percentage of Firmicutes to Bacteroidetes was correlated with improved cell proliferation, and significant changes in observational results were noted 12 h after partial hepatectomy (PH) [Citation10].
In prebiotics, the supply of growth supplements for GM to drive different composites or metabolic changes can be an alleviative strategy. For instance, fructose well-known as prebiotics protect hepatocytes from apoptosis induced by tumour necrosis factor (TNF) via activating JNK pathway [Citation11]. Lactulose accelerates H2 production intrinsically and promotes LR after 70% partial PH in rats [Citation12]. Presumably, it elicits that key prebiotics digested by GM converted into key metabolites which can exert biological activity.
In metabolites, indoles and its derivatives as the representative metabolites produced by GM intaking tryptophan in the gut play a significant role in enhancing LR through stabilizing the gut mucosal epithelial barrier [Citation13]. More recently, endogenous microbiota-derived components, such as proteins and compounds produced from living microbiota communities, have exerted favourable effects on hosts [Citation14]. Metabolites derived from GM can reach the liver via the portal vein, which can ameliorate liver diseases related to immunity [Citation15].
The metabolites produced by GM and exogenous substrate (ES) affect via gut-liver axis, which might manage the expression of cytokines in intestinal epithelial cells and hepatocytes [Citation13]. Accordingly, they play important roles to promote LR [Citation16,Citation17]. Furthermore, many liver diseases have been implicated in inhibiting LR [Citation18]. The metabolites (postbiotics) and immune system are significant factors influencing on LR via GM. The diversity of GM is a critical element to control above the two factors (metabolites and prebiotics) and thus acts on LR.
Hence, the multiple components as in GM (probiotics), ES (prebiotics) and metabolites (postbiotics) can be utilized to accelerate the rate of LR. However, the definite mechanisms of these inter-networking remain unclear. In the context of incomplete project, we employed the network pharmacology to unravel the conundrum in the complex microbiome for LR.
Network pharmacology is a systemic perspective to elucidate the effector mechanism of targets and ligands (metabolites) in complex biological pathways [Citation19]. Recently, a report showed that network pharmacology was utilized to uncover the effects of the infectious GM against ulcerative colitis [Citation20]. A study suggested that expounding the promising pharmacological mechanisms connected with microbiota diversity affects the host pathological response directly or indirectly [Citation21]. Therefore, the use of an integrated concept to decode the complex scenario in the microbiome, as such relationships between GM, signalling pathways, targets and metabolites, is imperative.
In this study, network pharmacology was utilized to reveal key components for improving LR and to determine how the microbiota-signalling pathways-targets-metabolites (MSTM) relationship induces positive effects on LR. First, we utilized the gutMGene database to retrieve targets and metabolites related to the microbiota. Then, metabolite-associated targets and LR-related targets were identified by two public databases. Second, the overlapping targets between the two public databases were obtained via a Venn diagram, in which the intersecting targets were determined between LR-related targets. The final overlapping targets were identified by comparison with the intersecting targets and reported targets in the gutMGene database. Third, the final overlapping targets were chosen to construct a protein–protein interaction (PPI) network and a bubble plot to identify the key signalling pathways in LR. After identifying the key signalling pathways, we performed a molecular docking assay (MDA) to verify the potential metabolites on key targets. Finally, we integrated the relationships of MSTM networks to reveal how the four components interact with one another in LR. Therefore, this analysis might provide critical hints for further experimental studies and the utilization of metabolites for LR. The workflow of this study is displayed in . Additionally, pioneering clinical intervention of various liver diseases directing to facilitate LR associated with metabolism by adjusting to GM is a promising therapeutic strategy in the future research area.
Figure 1. (A) A workflow of this study. (B) The number of 668 overlapping targets between SEA (1256) and STP (947). (C) The number of 61 targets between 668 targets and liver regeneration-related targets (365). (D) The identification of 13 core targets between 61 targets and Gut human targets (223 targets) from gutMGene database. SEA: Similarity Ensemble Approach; STP: SwissTargetPrediction; PPI: protein–protein interaction; GO: gene ontology; MSTM: microbiota-signalling pathways-targets-metabolite.
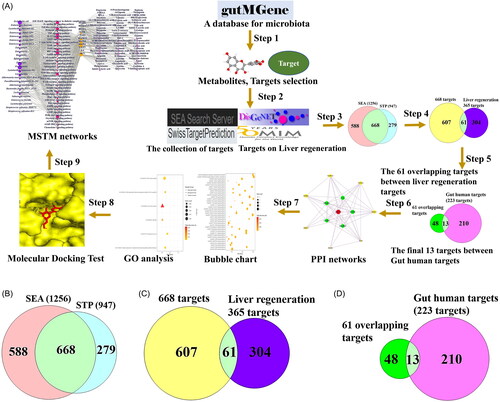
Materials and methods
Database platforms and data analysis associated with network pharmacology
Biologically significant databases that give a great amount of data associated with the relationship between compounds and proteins enable the researcher to employ network pharmacology as a powerful way for drug discovery (Supplementary Table S1). All these web-based databases are accessible freely to users who pursue to retrieve valuable information, moreover, which is functional methodology to be applicable to microbiome and network pharmacology principle at once. In this study, we tried new approach to explore the complex microbiome and its network with phytochemicals, via public databases.
Table 1. The degree value of core targets.
The mining of metabolites and targets from the gut microbiota
The gutMGene v1.0 (http://bioannotation.cn/gutmgene/) (accessed on 15 June 2022) was employed to retrieve the metabolites and targets for further analysis. The metabolites converted by GM were identified by a sub-folder of downloads section (http://bio-annotation.cn/gutmgene/public/res/gutMGene-human.xlsx) in gutMGene v1.0, which was considered as key metabolites reported to date. The Simplified Molecular Input Line Entry System (SMILES) formats of metabolites were adopted by PubChem (https://pubchem.ncbi.nlm.nih.gov/) (accessed on 15 June 2022).
The acquisition of crucial targets for regeneration
Using the SMILES format, the related targets on metabolites were browsed by both the Similarity Ensemble Approach (SEA) as a cheminformatics database to identify potential targets from repository of compounds (http://sea.bkslab.org/) (Accessed on 16 June 2022) and SwissTargetPrediction (STP) as a target-selecting database platform from reservoir of ligands (http://www.swisstargetprediction.ch/) (Accessed on 16 June 2022) on ‘Homo Sapiens’ mode. Specifically, Dr Shoichet’s group assembled the ‘Similarity Ensemble Approach (SEA)’ to identify the ligand-target affinity and eventually uncover its interaction [Citation22]. In addition, STP is a web-based searching tool developed by SIB (Swiss Institute of Bioinformatics), accessing approved 376,342 compounds and 3068 targets since 2014 [Citation23].
We obtained the intersecting targets between the overlapping targets selected by the SEA and STP databases and LR-related targets obtained by DisGeNET as a platform of target-disease correlation (https://www.disgenet.org/) (accessed on 16 June 2022) and OMIM as a database of target-etiology association (https://www.omim.org/) (Accessed on 16 June 2022) on a Venn diagram. Crucial targets between the intersecting targets and targets reported in the gutMGene database were obtained. Thus, we considered these targets to be the most significant targets for LR.
The protein–protein interaction network
The PPI network was identified by STRING (https://string-db.org/) analysis (accessed on 20 June 2022), the network of which was imported into R Package software. The middlemost target with the greatest degree value in the PPI network was considered the uppermost target.
Gene ontology and Kyoto Encyclopaedia of Genes and Genomes analysis
Gene ontology (GO) molecular function (MF) analysis was mainly utilized to represent the functions of targets, including biological processes (BPs) and cellular components (CCs). This information was displayed on a bubble plot utilizing the R Package. The signalling pathways on the KEGG (Kyoto Encyclopaedia of Genes and Genomes) database were analysed based on the crucial targets, which allows the identification of the signalling pathways related to metabolites from the GM.
Metabolite-target preparation for molecular docking assay
The metabolites linked to the key targets were translated into .sdf files from PubChem to the .pdb format utilizing PyMOL. Then, files in the .pdbqt format were obtained through AutoDock software and used to perform MDT. The main targets were retrieved from the STRING database via RCSB (https://www.rcsb.org/) (accessed on 18 June 2022). Files in the .pdb format extracted from RCSB were converted into the .pdbqt format for incorporation into AutoDock −1.5.6 for MDT.
Molecular docking assay
AutoDockTools-1.5.6 was adopted to generate the target centre and the grid box dimensions of the active site. With the default 10 different poses of the metabolites, AutoDock4 software was set up with 4 energy ranges and 8 exhaustiveness to identify the greatest score. For docking, a cubic box was created with solid dimensions based on the active site, which was set to 40 Å × 40 Å × 40 Å. The tangible centres to bind the metabolites on the two main targets were X: 6.313, Y: −7.926, Z: 17.198 and X: −20.844, Y: 40.173, Z: 21.018. The hydrophobic and hydrophilic residues of the complex were obtained by LigPlot+ 2.2 (https://www.ebi.ac.uk/thornton-srv/software/LigPlus/download.html) (Accessed on 19 June 2022)) [Citation24]. The cut-off value of MDA was −6.0 kcal/mol [Citation25], and the key ligand with the lowest docking score on a specific target was considered the uppermost metabolite for LR.
Drug-likeness properties and toxicological evaluation
The drug-likeness properties of the two metabolites were evaluated using the SwissADME database [Citation26]. Conventionally, the metabolite is prone to exhibit hydrophilic properties for easy excretion from the body, indicating that these metabolites have low bioavailability. Thus, it is necessary to profile their physicochemical characteristics using an in silico assay.
In addition, the level of toxicity is the primary factor that fails during the development of new agents. Toxicity is assessed using the following agents and assays: hERG blockers [Citation27], Human Hepato-Toxicity (H-HT) [Citation28], Ames mutagenicity [Citation29], Skin sensitization [Citation30], Lethal Dose 50 (LD50) of acute toxicity [Citation31] and Drug Induced Liver Injury (DILI) [Citation32]. The toxicity findings are confirmed utilizing the ADMETlab platform [Citation33].
Construction of the microbiota-signalling pathways-targets-metabolite network
The crucial targets were input into the STRING database to examine the proteins under the Homo sapiens setting. We subsequently identified the main signalling pathways for LR. Then, microbiota and metabolites related directly to targets were selected via the gutMgene database. MSTM networks were visualized using R Package. The microbiota, signalling pathways, targets and metabolites are described as nodes in the network, and the relationships between the four components are depicted as edges [Citation34]. The MSTM network was constructed on a size plot based on the number of edges for each node. In the network plot, purple circles (nodes) represent the GM; red circles (nodes) represent the signalling pathways; orange circles (nodes) describe the targets; and pink circles (nodes) indicate the metabolites. The size of the purple circles describes the number of connectivity to signalling pathways, metabolites and targets; the size of the red circles represents the number of interactions with GM; the size of orange circles represents the number of associations with signalling pathways; and the size of pink circles depicts the number of correlations with targets. The integrated network was incorporated using R Package.
Results
The promising targets and metabolites from gut microbiota
A total of 208 metabolites obtained by a sub-folder in gutMGene v1.0 converted by the GM were identified through the gutMGene database (Supplementary Table S2).
Table 2. The number of 36 signalling pathways and targets related to LR.
The targets related to the collected metabolites were identified using the SEA (1256 targets) and STP (947 targets) databases () (Supplementary Table S3), and the two databases revealed 668 overlapping targets () (Supplementary Table S3). In total, 61 targets overlapped between 668 targets and 365 LR-related targets (Supplementary Table S3). Finally, 13 core targets overlapping between 61 targets and 223 targets from the gutMGene database were selected to analyse the PPI network () (Supplementary Table S3).
Table 3. The evaluation of drug-likeness properties on two key metabolites.
Protein–protein interaction network
The 13 core targets were incorporated into PPI network analysis, highlighting the most essential proteins in the network. The PPI network consisted of 13 nodes and 58 edges. AKT1 had the highest degree of value (12), showing that AKT1 might be a significant target to accelerate LR () (). Up to date, many of the research indicated that AKT1 might be a hub target to relieve LR. Accordingly, our research lines up with the implication demonstrated by the survey. The targets with the next highest degrees of value included EGFR (11), IL-6 (11), PPARG (11), CASP3 (10) and MAPK8 (10).
Gene ontology and signalling pathway enrichment analysis
To further facilitate the therapeutic application of the metabolites from the GM in the pharmacological concept of LR, the 13 core targets were assessed by signalling pathway enrichment () and GO enrichment analysis () using the STRING bioinformatics database. The 13 core targets were directly associated with 36 signalling pathways in LR (), and the circle size represents the number of targets connected to the pathway. As an observational result, the chemokine signalling pathway with the lowest rich factor (gene ratio) represents a potent inhibitive mechanism for LR. In KEGG pathway, the lowest rich factor is, the more inhibitive signalling pathway is [Citation35]. Additionally, the GO enrichment analysis comprised three modules: MF, BP and CC.
Molecular docking assay analysis
The binding affinities of a total of 20 metabolites and two targets (AKT1 and MAPK1) related to the chemokine signalling pathway at the cocrystalized complex were assessed. Regarding AKT1 (PDB ID: 3O96), myricetin exhibited the highest binding energy of −8.6 kcal/mol followed by kampferol (−8.5 kcal), apigenin (−8.4 kcal/mol) and diosmetin (−8.2 kcal/mol). Compared with three positive controls (miransertib: −7.7 kcal/mol; uprosertib: −7.7 kcal/mol; and afuresertib: −7.6 kcal/mol) (Supplementary Table S4), myricetin could bind stably to the active sites of AKT1. The hydrogen bond interactions of Asn199, Trp80 and Asn53 and the hydrophobic interactions of Gln59, Leu78, Ala58, Ser56, Gln79 and Val201 facilitated myricetin-AKT1 (PDB ID: 3O96) complex formation ().
Figure 3. (A) The docking result of myricetin-AKT1. (B) The docking result of Compound K-MAPK1. (C) Microbiota-signalling pathways-targets-metabolite (MSTM) networks (144 nodes, 499 edges).
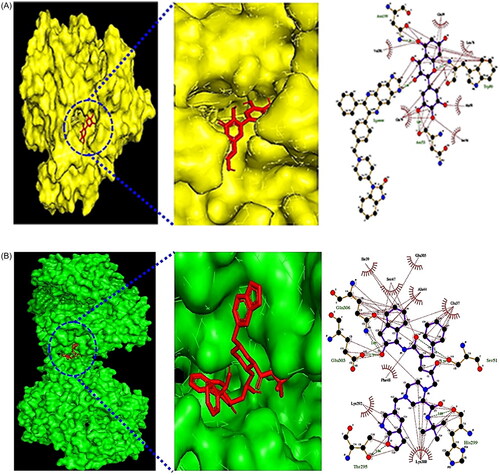
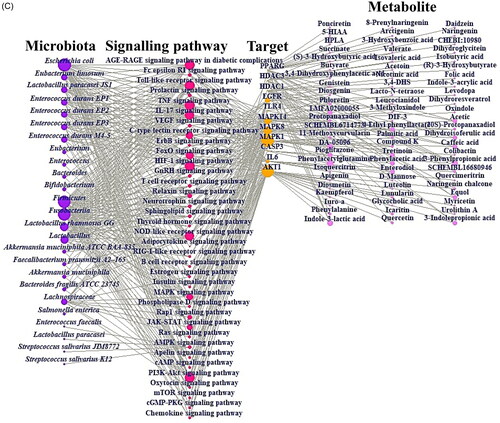
Table 4. The evaluation of toxicity on two key metabolites.
Notably, with a strong binding affinity of −14.0 kcal/mol, colibactin harboured a significant binding pocket for MAPK1 (PDB ID: 5IZ5). However, previous studies have demonstrated that colibactin is a potent genotoxin that is secreted by some bacteria, including the human gut microbiome [Citation36,Citation37]. Thus, we did not consider it as a potential agent in this study. Instead, Compound K, with the second-best binding affinity of −8.4 kcal/mol, was regarded as a promising effector for LR. Moreover, the binding stability of Compound K-MAPK1 (PDB ID: 5IZ5) had a higher affinity than that of the positive controls DH-6-OH-rotenone (−7.8 kcal/mol) and Cu-CPT22 (−6.0 kcal/mol) (Supplementary Table S5). The hydrogen bond interactions of Gln306, Ser51, His299, Thr295 and Glu303 and the hydrophobic interactions of Ile39, Ser47, Glu305, Ala44, Glu37, Lys300, Lys292 and Phe48 facilitated Compound K-MAPK1 (PDB ID: 5IZ5) complex formation ().
Drug-likeness and toxicity evaluation
The two metabolites (myricetin and Compound K) were verified via in silico methodologies, including assessment of drug-likeness properties, such as Lipinski’s rule and Topological Polar Surface Area (TPSA) (Cut-off value: <140 Å2) (). Hence, it is suggested that the two metabolites from the GM can be administered orally to facilitate LR. The toxicity evaluation of Compound K and myricetin was performed using the ADMETlab online tool (). The result shows that the two metabolites did not exhibit obvious hazardous properties that would serve as hurdles if these metabolites were utilized as drugs.
Analysis of MSTM networks
The MSTM network represented the relationships between microbiota, signalling pathways, targets and metabolites, consisting of 144 nodes (25 microbiota, 36 signalling pathways, 11 targets and 72 metabolites) and 499 edges (). The purple circles represent the GM, the red circles indicate the signalling pathways, the orange circles represent the targets, and the pink circles describe the metabolites. The size of each circle indicates the number of relationships between the two. The analysis was performed using R Package. We found that Escherichia coli is the uppermost microbiota with 271 degrees of value, and the AGE-RAGE signalling pathway in diabetic complications, Toll-like receptor signalling pathway, TNF signalling pathway, HIF-1 signalling pathway, and PI3K-Akt signalling pathway were identified as significant mechanisms involved in LR. We also observed that the most significant targets are MAPK1 (32) and AKT1 (31), which exhibit higher degrees of value than other targets. In parallel, phenylacetylglutamine is the most notable metabolite due to the highest degree of value for LR. The suggested 4 elements represent underlying hallmarks of LR, indicating that these factors might orchestrate favourable effects on LR.
Discussion
Our study provides significant perspectives into systemic relationships between the microbiome and LR, which is an important and robust methodology to clarify the function of potential bioactive metabolites at the integrated level. Additionally, our study demonstrated that the 12 metabolites related to a key target (AKT1) were classified into 5 categories: flavonoids (6 compounds), coumarins (2 compounds), indoles (2 compounds), steroids (1 compound) and carboxylic acids (1 compound).
Flavonoids represented 50% of the 12 metabolites, suggesting that flavonoids are significant compounds that exert more pharmacological effects in LR compared with other metabolites. According to the physicochemical properties of drug-likeness, the 90th percentile of agents accepted by Lipinski’s rule are entered into phase II [Citation38]. Additionally, myricetin bound stably to AKT1 and is a flavonoid compound that inhibits the PI3K/AKT signalling pathway [Citation39]. Notably, myricitrin can be converted into myricetin via Escherichia coli sp. 12 and Escherichia coli sp. 33 [Citation40]. The result is consistent with our observational result, indicating that Escherichia sp. could positively affect LR after liver injury. In parallel, the myricetin dampens the activation of hepatic stellate cell (HSC) and alleviates liver fibrosis induced by carbon tetrachloride (CCl4) as well as diminishes TNF-α expression [Citation41]. Besides, proinflammatory cytokines as in TNF-α and IL-6 expression level in hepatocytes were dramatically reduced (p value < .001) by myricetin [Citation42]. The hepatectomy is a mainstay for treatment of the hepatocellular carcinoma (HCC); however, some subjects are exposed to critical post-hepatectomy complications such as subphrenic infection and urinary tract infection related to inflammatory responses [Citation43,Citation44]. An animal test demonstrated the pharmacological efficacy of flavonoid silymarin, suggesting that silymarin promoted LR by activating cell cycle in PH liver [Citation45]. It implies that flavonoids might be potential agents to accelerate LR after PH.
The Lycium chinense combined to myricetin has favourable efficacy on antioxidant activity and the regenerative ratio of residue liver tissue after 70% PH in rats [Citation46]. A report showed that human hepatocytes grow gradually its size for 3, 6 and 10 weeks after transplanting of myricetin-treated hepatocytes [Citation47]. Collectively, myricetin might be an effective agent to relieve inflammation and to accelerate cell proliferation during LR.
The metabolites from the GM had no connectivity to MAPK1. Compound K is a potential metabolite derived from the microbiota. Recently, a report demonstrated that Compound K has potent protective effect on high fat diet (HFD)-induced hepatocyte injury [Citation48]. Still, therapeutic value of Compound K is under consideration including LR.
Reportedly, both myricetin and Compound K enhance wound healing at the cellular level, demonstrating that they have potent anti-inflammatory efficacy [Citation39,Citation49,Citation50]. Other reports have shown that AKT1 and MAPK inhibitors play a crucial role in ameliorating specific injury correlated with inflammasomes in cardiomyocytes [Citation51,Citation52]. In particular, the relative low levels of CXC chemokine are linked to LR and repair, whereas its overexpression levels were related to hepatotoxicity [Citation53–56]. It can thus be hypothesized that the two metabolites (myricetin and Compound K) from GM can dampen inflammation with synergistic effects on each target by inactivating the chemokine signalling pathway.
GO enrichment analysis indicated that metabolites from GM mainly play a role in MAP kinase activity and NAD-dependent histone deacetylase activity (H3-K14 specific) in the MF category; host cell nucleus and spindle in the CC category; and response to ultraviolet-A (UV-A) and cellular response to oxidized low-density lipoprotein particle stimulus in the BP category.
The associations of the top 10 pathways with LR were concisely described as follows, and these findings are based on rich factors. Activation of the AGE-RAGE signalling pathway can enhance intestinal permeability. Thus, lipopolysaccharides (LPS) can cross the intestinal epithelial barrier [Citation57]. This finding implies that the AGE-RAGE signalling pathway induces systemic inflammation and exacerbates liver injury. The Fc epsilon RI signalling pathway is activated by immunoglobulin E (IgE), which has negative effects on LR [Citation57,Citation58]. The Toll-like receptor signalling pathway has two functions. On one hand, the pathway results in favourable effects that improve LR. On the other hand, the pathway leads to delayed LR [Citation59]. From this perspective, studies on TLR in LR might reveal an important mechanism for developing new therapeutic strategies. Similarly, the prolactin signalling pathway is also a double-edged sword with respect to cellular inflammation under some conditions [Citation60].
Treatment with IL-17 promoted proliferation in a liver progenitor cell (LPC) line through the IL-17 signalling pathway [Citation61]. It has been suggested that IL-17 might represent a potential agent for LR via further studies. TNF is a considered trigger for the development of LR, which simultaneously induces an inflammatory cascade via the TNF signalling pathway [Citation62,Citation63]. In addition, an animal test demonstrated that TNF does not promote LR [Citation64]. Still, the exact function of TNF was not exposed clearly to LR. An animal test showed that VEGF plays a significant role in healing injured tissue and enhancing regenerative capacity after PH [Citation65].
A previous report indicated that the deletion of the C-type lectin receptor has detrimental effects on LR after hepatectomy [Citation66]. It can be postulated that the activation of the C-type lectin receptor might be attributed to LR. The ErbB signalling pathway exhibits different expression patterns between foetal and adult hepatocytes but remains poorly understood [Citation50]. FoxO3 suppresses LR by upregulating Nox4 and downregulating Nr4a1 in the Forkhead Box Transcription Factors (FoxO) signalling pathway [Citation67]. This finding indicated that FoxO3 regulation might represent a crucial target against liver damage and for LR. The systemic network is a method used to predict the biological relationships and potential components to reveal the metabolites from complex microbiome interactions [Citation68].
Collectively, we identify key microbiota, mechanisms and targets core metabolites, and our results suggest that these components play important roles in LR. Based on mechanistic insight into network pharmacology, this study provides an integrated methodology to elucidate the relationships of MSTM networks for LR. Despite limited data on the complex microbiome, the therapeutic mechanisms of metabolites from GM were identified by utilizing multiple data. In subsequent analyses, the network models for microbiome analysis provide scientific-based evidence for further clinical trials.
Conclusion
In summary, this study demonstrated promising effectors (AKT1 and MAPK1) in the treatment of LR using a network pharmacology approach. We revealed that Escherichia sp., AKT1 and myricetin might play significant roles in LR by dampening the chemokine signalling pathway. In addition, our MDA also showed that Compound K can bind stably to MAPK1, positively affecting LR. These results suggest a significant point for further investigation. However, this study has some limitations, and clinical trials should be analysed to further verify our findings.
Author contributions
Ki-Tae Suk, Dong Joon Kim and Ki-Kwang Oh had substantial contributions to the conception or design of the work and interpretation of data for the work. Ki-Tae Suk, Ki-Kwang Oh and Ickwon Choi were involved in the acquisition, analysis or interpretation of data for the work. Ki-Tae Suk, Ki-Kwang Oh, Haripriya Gupta, Raja Ganesan, Satya Priya Sharma, Sung-Min Won and Jin-Ju Jeong drafted the work and revised it critically for important intellectual content and finally approved the version to be published. Su-Been Lee, Min-Gi Cha, Goo-Hyun Kwon, Min-Kyo Jeong, Byeong-Hyun Min, Ji-Ye Hyun, Jung-A Eom, Hee-Jin Park, Sang-Jun Yoon and Mi-Ran Choi agree to be accountable for all aspects of the work in ensuring that questions related to the accuracy or integrity of any part of the work are appropriately investigated and resolved.
Supplemental Material
Download MS Excel (11.6 KB)Supplemental Material
Download MS Excel (13.1 KB)Supplemental Material
Download MS Excel (42.7 KB)Supplemental Material
Download MS Excel (12.7 KB)Supplemental Material
Download MS Excel (11.7 KB)Acknowledgments
This study has been implemented with the support of the Hallym University Research Fund, the Basic Science Research Program through the National Research Foundation of Korea, funded by the Ministry of Education, Science and Technology.
Disclosure statement
No potential conflict of interest was reported by the author(s).
Data availability statement
All data generated or analysed during this study are included in this published article (and its Supplementary Information files).
Correction Statement
This article has been corrected with minor changes. These changes do not impact the academic content of the article.
Additional information
Funding
References
- Tao Y, Wang M, Chen E, et al. Liver regeneration: analysis of the main relevant signaling molecules. Mediators Inflamm. 2017;2017:4256352.
- Dewhurst MR, Ow JR, Zafer G, et al. Loss of hepatocyte cell division leads to liver inflammation and fibrosis. PLoS Genet. 2020;16(11):e1009084.
- Tan Z, Sun H, Xue T, et al. Liver fibrosis: therapeutic targets and advances in drug therapy. Front Cell Dev Biol. 2021;9:2622.
- Dickson JC, Liese AD, Lorenzo C, et al. Associations of coffee consumption with markers of liver injury in the insulin resistance atherosclerosis study. BMC Gastroenterol. 2015;15(1):1–9.
- Hoffmann K, Nagel AJ, Tanabe K, et al. Markers of liver regeneration—the role of growth factors and cytokines: a systematic review. BMC Surg.;2020;20(1):1–15.
- Zheng Z, Wang B. The gut-liver axis in health and disease: the role of gut microbiota-derived signals in liver injury and regeneration. Front Immunol. 2021;12:775526.
- Yu J. The functional role and translational potential of gut microbiota and microbial metabolites in liver diseases. J Gastroenterol Hepatol. 2022;37(1):5–6.
- Wang J, Wang Y, Zhang X, et al. Gut microbial dysbiosis is associated with altered hepatic functions and serum metabolites in chronic hepatitis B patients. Front Microbiol. 2017;8:2222.
- Li F, McClain CJ, Feng W. Microbiome dysbiosis and alcoholic liver disease. Liver Res. 2019;3(3-4):218–226.
- Bao Q, Yu L, Chen D, et al. Variation in the gut microbial community is associated with the progression of liver regeneration. Hepatol Res. 2020;50(1):121–136.
- Alwahsh SM, Gebhardt R. Dietary fructose as a risk factor for non-alcoholic fatty liver disease (NAFLD). Arch Toxicol. 2017;91(4):1545–1563.
- Yu J, Zhang W, Zhang R, et al. Lactulose accelerates liver regeneration in rats by inducing hydrogen. J Surg Res. 2015;195(1):128–135.
- Xu Z, Jiang N, Xiao Y, et al. The role of gut microbiota in liver regeneration. Front Immunol. 2022;13:1003376.
- Howarth GS, Wang H. Role of endogenous microbiota, probiotics and their biological products in human health. Nutrients. 2013;5(1):58–81.
- Li R, Mao Z, Ye X, et al. Human gut microbiome and liver diseases: from correlation to causation. Microorganisms. 2021;9(5):1017.
- Peterson LW, Artis D. Intestinal epithelial cells: regulators of barrier function and immune homeostasis. Nat Rev Immunol. 2014;14(3):141–153.
- Allaire JM, Crowley SM, Law HT, et al. The intestinal epithelium: central coordinator of mucosal immunity. Trends Immunol. 2018;39(9):677–696.
- Forbes SJ, Newsome PN. Liver regeneration - mechanisms and models to clinical application. Nat Rev Gastroenterol Hepatol. 2016;13(8):473–485.
- Sakle NS, More SA, Mokale SN. A network pharmacology-based approach to explore potential targets of Caesalpinia pulcherima: an updated prototype in drug discovery. Sci Rep. 2020;10(1):16.
- Ding Y, Chen M, Wang Q, et al. Integrating pharmacology and microbial network analysis with experimental validation to reveal the mechanism of composite sophora colon-soluble capsule against ulcerative colitis. Evid Based Complement Alternat Med. 2020;2020:9521073.
- Liu HX, Keane R, Sheng L, et al. Implications of microbiota and bile acid in liver injury and regeneration. J Hepatol. 2015;63(6):1502–1510.
- Keiser MJ, Setola V, Irwin JJ, et al. Predicting new molecular targets for known drugs. Nature. 2009;462(7270):175–181.
- Gfeller D, Grosdidier A, Wirth M, et al. SwissTargetPrediction: a web server for target prediction of bioactive small molecules. Nucleic Acids Res. 2014;42(W1):W32–W38.
- Laskowski RA, Swindells MB. LigPlot+: multiple ligand-protein interaction diagrams for drug discovery. J Chem Inf Model. 2011;51(10):2778–2786.
- Shityakov S, Förster C. In silico predictive model to determine vector-mediated transport properties for the blood-brain barrier choline transporter. Adv Appl Bioinform Chem. 2014;7:23–36.
- Daina A, Zoete V. A BOILED-Egg to predict gastrointestinal absorption and brain penetration of small molecules. ChemMedChem. 2016;11(11):1117–1121.
- Lamothe SM, Guo J, Li W, et al. The human ether-a-go-go-related gene (hERG) potassium channel represents an unusual target for protease-mediated damage. J Biol Chem. 2016;291(39):20387–20401.
- Mulliner D, Schmidt F, Stolte M, et al. Computational models for human and animal hepatotoxicity with a global application scope. Chem Res Toxicol. 2016;29(5):757–767.
- Xu C, Cheng F, Chen L, et al. In silico prediction of chemical Ames mutagenicity. J Chem Inf Model. 2012;52(11):2840–2847.
- Alves VM, Muratov E, Fourches D, et al. Predicting chemically-induced skin reactions. Part II: QSAR models of skin permeability and the relationships between skin permeability and skin sensitization. Toxicol Appl Pharmacol. 2015;284(2):273–280.
- Lei T, Li Y, Song Y, et al. ADMET evaluation in drug discovery: 15. Accurate prediction of rat oral acute toxicity using relevance vector machine and consensus modeling. J Cheminform. 2016;8:6.
- Xu Y, Dai Z, Chen F, et al. Deep learning for drug-induced liver injury. J Chem Inf Model. 2015;55(10):2085–2093.
- Dong J, Wang NN, Yao ZJ, et al. ADMETlab: a platform for systematic ADMET evaluation based on a comprehensively collected ADMET database. J Cheminform. 2018;10(1) : 1–11.
- Lee AY, Park W, Kang TW, et al. Network pharmacology-based prediction of active compounds and molecular targets in Yijin-Tang acting on hyperlipidaemia and atherosclerosis. J Ethnopharmacol. 2018;221:151–159.
- Zhang YJ, Sun YZ, Gao XH, et al. Integrated bioinformatic analysis of differentially expressed genes and signaling pathways in plaque psoriasis. Mol Med Rep. 2019;20(1):225–235.
- Nougayrède JP, Homburg S, Taieb F, et al. Escherichia coli induces DNA double-strand breaks in eukaryotic cells. Science. 2006;313(5788):848–851.
- Auvray F, Perrat A, Arimizu Y, et al. Insights into the acquisition of the pks island and production of colibactin in the Escherichia coli population. Microbial Genomics. 2021;7(5) : 1–16.
- Quinn RJ, Carroll AR, Pham NB, et al. Developing a drug-like natural product library. J Nat Prod. 2008;71(3):464–468.
- Rehan M, Mahmoud MM, Tabrez S, et al. Exploring flavonoids for potential inhibitors of a cancer signaling protein PI3Kγ kinase using computational methods. Anticancer Res. 2020;40(8):4547–4556.
- Du LY, Zhao M, Xu J, et al. Identification of the metabolites of myricitrin produced by human intestinal bacteria in vitro using ultra-performance liquid chromatography/quadrupole time-of-flight mass spectrometry. Expert Opin Drug Metab Toxicol. 2014;10(7):921–931.
- Geng Y, Sun Q, Li W, et al. The common dietary flavonoid myricetin attenuates liver fibrosis in carbon tetrachloride treated mice. MNFR. 2017;61(4): 1-9.
- Wang X, Sun Y, Li P, et al. The protective effects of myricetin against acute liver failure via inhibiting inflammation and regulating oxidative stress via Nrf2 signaling. Nat Prod Res. 2022; 36(24): 1–5.
- Oh JH, Sinn DH, Choi GS, et al. Comparison of outcome between liver resection, radiofrequency ablation, and transarterial therapy for multiple small hepatocellular carcinoma within the Milan criteria. Ann Surg Treat Res. 2020;99(4):238–246.
- Jin S, Fu Q, Wuyun G, et al. Management of post-hepatectomy complications. World J Gastroenterol. 2013;19(44):7983–7991.
- Wu JP, Tsai CC, Yeh YL, et al. Silymarin accelerates liver regeneration after partial hepatectomy. Evid Based Complement Alternat Med. 2015;2015:603529.
- Ahn YM, Lee GS, Yang HG, et al. Antioxidant and proliferative activity of the fruit extract of Lycium chinense in regenerating liver after partial hepatectomy in rats. Biomed Res. 2018;29(12):2590–2596.
- Cui C, Enosawa S, Matsunari H, et al. Natural flavonol, myricetin, enhances the function and survival of cryopreserved hepatocytes in vitro and in vivo. IJMS. 2019;20(24):6123.
- Chen XJ, Liu WJ, Wen ML, et al. Ameliorative effects of compound K and ginsenoside Rh1 on non-alcoholic fatty liver disease in rats. Sci Rep. 2017 7:41144.
- Elshamy AI, Ammar NM, Hassan HA, et al. Topical wound healing activity of myricetin isolated from Tecomaria capensis v. aurea. Molecules. 2020;25(21):4870.
- Ding L, Gu S, Zhou B, et al. Ginsenoside compound K enhances fracture healing via promoting osteogenesis and angiogenesis. Front Pharmacol. 2022;13:855393.
- Wang R, Wang Y, Wu J, et al. Resveratrol targets AKT1 to inhibit inflammasome activation in cardiomyocytes under acute sympathetic stress. Front Pharmacol. 2022;13:818127.
- Romero-Becerra R, Santamans AM, Folgueira C, et al. p38 MAPK pathway in the heart: new insights in health and disease. IJMS. 2020;21(19):7412.
- Hogaboam CM, Bone‐Larson CL, Steinhauser ML, et al. Novel CXCR2-dependent liver regenerative qualities of ELR-containing CXC chemokines. FASEB J. 1999;13(12):1565–1574.
- Colletti LM, Green M, Burdick MD, et al. Proliferative effects of CXC chemokines in rat hepatocytes in vitro and in vivo. Undefined. 1998;10:248–257.
- Kuboki S, Shin T, Huber N, et al. Hepatocyte signaling through CXC chemokine receptor-2 is detrimental to liver recovery after ischemia/reperfusion in mice. Hepatology. 2008;48(4):1213–1223.
- Stefanovic L, Stefanovic B. Mechanism of direct hepatotoxic effect of KC chemokine: sequential activation of gene expression and progression from inflammation to necrosis. J Interferon Cytokine Res. 2006;26(10):760–770.
- Kay AM, Simpson CL, Stewart JA. The role of AGE/RAGE signaling in diabetes-mediated vascular calcification. J Diabetes Res. 2016;2016:6809703.
- Lei Y, Huang T, Su M, et al. Expression and distribution of immunoglobulin G in the normal liver, hepatocarcinoma and postpartial hepatectomy liver. Lab Invest. 2014;94(11):1283–1295.
- Seki E, Park E, Fujimoto J. Toll-like receptor signaling in liver regeneration, fibrosis and carcinogenesis. Hepatol Res. 2011;41(7):597–610.
- Ramos-Martinez E, Ramos-Martínez I, Molina-Salinas G, et al. The role of prolactin in central nervous system inflammation. Rev Neurosci. 2021;32(3):323–340.
- Guillot A, Gasmi I, Brouillet A, et al. Interleukins-17 and 27 promote liver regeneration by sequentially inducing progenitor cell expansion and differentiation. Hepatol Commun. 2018;2(3):329–343.
- Kimura T, Sakaida I, Terai S, et al. Inhibition of tumor necrosis factor-α production retards liver regeneration after partial hepatectomy in rats. Biochem Biophys Res Commun. 1997;231(3):557–560.
- Ozkurt M, Hellwig-Bürgel T, Depping R, et al. miR663 prevents Epo inhibition caused by TNF-alpha in normoxia and hypoxia. Int J Endocrinol. 2021;2021:3670499.
- Fujita J, Marino MW, Wada H, et al. Effect of TNF gene depletion on liver regeneration after partial hepatectomy in mice. Surgery. 2001;129(1):48–54.
- Taniguchi E, Sakisaka S, Matsuo K, et al. Expression and role of vascular endothelial growth factor in liver regeneration after partial hepatectomy in rats. J Histochem Cytochem. 2001;49(1):121–130.
- Kono H, Fujii H, Suzuki-Inoue K, et al. The platelet-activating receptor C-type lectin receptor-2 plays an essential role in liver regeneration after partial hepatectomy in mice. J Thromb Haemost. 2017;15(5):998–1008.
- Carver RS, Stevenson MC, Scheving LA, et al. Diverse expression of ErbB receptor proteins during rat liver development and regeneration. Gastroenterology. 2002;123(6):2017–2027.
- Rosario D, Boren J, Uhlen M, et al. Systems biology approaches to understand the host–microbiome interactions in neurodegenerative diseases. Front Neurosci. 2020;14:716.