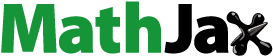
Abstract
Evaluation of the proper and appropriate consultants can play an important role in successful total quality management (TQM) program implementation and helps the manufacturing organizations to attain competitive advantage. In general, many conflicting factors affect the appropriate consultant selection problem which adheres to uncertain and imprecise data. In this paper, a simple, systematic and logical scientific approach is structured to evaluate TQM consultant through integrating Fuzzy Analytical Hierarchy Process with the Preference Ranking Organization Method for Enrichment Evaluations. The proposed decision-making approach takes advantage of the synergy between these two well-known multi-criteria decision-making methods in which their strong and weak points are detected and a ranking is provided which facilitates the final selection for the decision-maker. To accredit the proposed model, it is implemented in a furniture industry in Bangladesh. The results indicate that technical/administrative is the most significant criteria whereas work experience in related field is the most important sub-criteria.
1. Introduction
Due to rapid globalization, manufacturing organizations that can compete in today’s competitive market will be those that can win in global competition of both domestic and international markets. Due to the dynamic business environment and changing customers’ preferences, total quality management (TQM) becomes an essential management philosophy for manufacturing organizations to remain competitive (Khan, Citation2011). Today, a number of manufacturing organizations implement TQM to generate a competitive advantage (Shenawy, Baker, & Lemak, Citation2007), improve organizational effectiveness (Macinati, Citation2008), productivity (Kumar, Choisne, Grosbois, & Kumar, Citation2009), achieve customer satisfaction (Hur, Citation2009), improve the organization’s overall business process (Zehir & Sadikoglu, Citation2010), generate improved products and services (Munhurrun & Munhurrun, Citation2008), and overall reputation (Jung, Wang, & Wu, Citation2009; Psychogios & Priporas, Citation2007).
In the rush of this rising tide of TQM popularity, however, many organizations miss one important point – TQM is not a ‘quick-fix’, ‘off-the-shelf’ solution to competitiveness for any organization. TQM is a long-term improvement process which must be an integrated, continuous, professional system based on the commitment of top management and employees, working together with customers so that the needs of all are met (Ahire & Rana, Citation1995; Kabir, Citation2014; Saremi, Mousavi, & Sanayei, Citation2009; Tan, Citation1997). Thus, it takes more than just knowing all the right slogans or some statistical techniques, and investing in some training sessions to make TQM work (Kabir, Citation2014; Salaheldin, Citation2009).
It is broadly agreed that the key element to the long-term success of TQM within an organization is the implementation process (Shenawy et al., Citation2007; Wali & Boujelbene, Citation2010). The effectiveness of TQM implementation involves defining and development of several key elements. For the development and implementation of a successful TQM program in manufacturing organizations, Al-Khalifa and Aspinwall (Citation2008), Salaheldin (Citation2009), Shenawy et al. (Citation2007) and Siddiqui and Rahman (Citation2007) attempted to identify these key elements as critical success factors.
According to these literatures, TQM program fails due to lack of management commitment and management understanding on ‘Quality’, lack of awareness on the benefits of TQM implementation in the organization, lack of clarity in the guideline, implementation plan and implementation methods, inadequate knowledge of TQM and improper understanding of the measurement technique, lack of understanding about the positive results of continuous improvement and ignoring the importance of customers (Kabir, Citation2014; Saremi et al., Citation2009). Small and medium manufacturing firms typically face problem for implementation of successful TQM program due to the lack of adequate financial, internal and external resources. Meditating entities such as consultants can play an important role in successful TQM program implementation in manufacturing organization’s through removing knowledge gap and other constraints.
Selection of consultant depends on organization’s purposes, resources and preferences. This problem is affected by multiple factors which may be in conflict and adhere with uncertain and imprecise data (Kabir, Citation2014). In this complex and conflicting situation, multi-criteria decision-making (MCDM) is inevitable for the selection of appropriate TQM consultant for manufacturing organizations where both quantitative and qualitative objectives can be considered (Kabir, Citation2014). Moreover, involving several people from functional areas in the selection process increased the complexity of this process. MCDM refers to making preference decision (e.g. evaluation, prioritization, and selection) over the available alternatives that are characterized by multiple, usually conflicting criteria (Kabir & Hasin, Citation2012; Padhi & Mohapatra, Citation2009). Real-life applications of MCDM methods require the processing of imprecise, uncertain, qualitative or vague data (Chu, Citation2011; Omar, Trigunarsyah, & Wong, Citation2009).
Thus, a logical and systematic decision support tool is needed to guide manufacturing organizations for selecting appropriate TQM consultant. Therefore, the main objective of this study is to formulate a consultant selection decision support tool, which would determine influential and conflicting criteria and sub-criteria, and evaluate appropriate consultant. Fuzzy Analytical Hierarchy Process (FAHP) is being used more and more frequently in consultant selection (Cebeci & Ruan, Citation2007; Tsai, Lin, Chen, & Hung, Citation2007; Vayvay, Ozcan, & Cruz-Cunha, Citation2012) because of its similarity to human reasoning, dealing with ambiguous, vague, and uncertain characteristics, ideas and judgments (Kabir & Sumi, Citation2014). Moreover, FAHP allows group decision-making to derive priorities based on sets of pairwise comparisons (Kabir & Hasin, Citation2012; Kahraman, Cebeci, & Ulukan, Citation2003). But FAHP approach has some major limitations that compensation between good scores on some criteria and bad scores on other criteria can occur and the number of pairwise comparisons to be made, may become very large (more specifically: n(n − 1)/2) (Kabir & Sumi, Citation2014; Macharis, Springael, Brucker, & Verbeke, Citation2004; Wang & Yang, Citation2007).
On the contrary, Preference Ranking Organization METHod of Enrichment Evaluation (PROMETHEE), one of the most efficient outranking methods (Herva & Roca, Citation2013; Kalogeras, Baourakis, Zopounidis, & Dijk, Citation2005) is closely coinciding with the human perspectives and it determines the preferences among multiple decisions (Brans & Mareschal, Citation2005; Kabir, Sadiq, & Solomon Tesfamariam, Citation2013). For an integrated assessment analysis, PROMETHEE is a suitable approach as its flexible algorithm can enable tailor-made enhancements to meet specific requirements (Kabir et al., Citation2013). PROMETHEE also has some limitations like it does not yet provide any formal guidelines on how weights can be elicited (Gervasio & da Silva, Citation2012; Turcksin, Bernardinia, & Macharisa, Citation2011). It assumes that the decision-maker is able to weigh the criteria appropriately, no specific guidance is provided for its determination (Kabir & Sumi, Citation2014). Therefore, to eliminate these limitations and to develop an improved and systematic decision support tool for TQM consultant selection, the proposed decision-making approach takes advantage of the synergy between these two well-known multi-criteria methods where FAHP will apply to determine the weights of the criteria and sub-criteria, and PROMETHEE will be used for the aggregation of the criteria and ranking of the alternatives.
The remainder of this paper is organized as follows. In the next section, a brief literature review is presented. In the following section, the proposed methodology has been described with an overview and the concepts of the Fuzzy AHP, and PROMETHEE methods. In the next section, the proposed methodology is applied to evaluate and select the appropriate consultant for a furniture manufacturing company in Bangladesh. Finally, the last section presents the conclusion and discusses the limitations and scope for future research.
2. Literature review
Different MCDM methods have been proposed for the selection or evaluation of consultant for the quality improvement. Cheung, Kuen, and Skitmore (Citation2002) implemented analytic hierarchy process (AHP) for the architectural consultant selection for the design and construction of the projects in Hong Kong. Tsai, Shen, Lee, and Kuo (Citation2007) also applied AHP to set priority weights for consultant alternatives in order to solve the enterprise resource planning (ERP) consultant selection problems. In another study, Tsai et al. (Citation2009) applied ANOVA and regression analysis to examine the impact of ERP consultant selection factors on ERP project management and the impact of ERP project management on the performance improvement on ERP systems. Ling (Citation2003) presented a conceptual model for the selection of architects by project managers using multi-attribute value technique (MAVT). Ng and Chow (Citation2004) also used MAVT for engineering consultant’s performance evaluation. The fuzzy gap analysis model is proposed to select and evaluate performance of engineering consultants by Chow and Ng (Citation2007). Saremi et al. (Citation2009) proposed nominal group technique (NGT) with technique for order preference by similarity to ideal solution (TOPSIS) under fuzzy environment for the consultant selection of an Iranian auto part manufacturer. NGT is used for selecting the criteria and fuzzy TOPSIS is used to rank the consultant firms considering qualitative and quantitative criteria. But the authors considered all the criteria as qualitative including implementation cost. Omar et al. (Citation2009) presented design science research method (DSRM) and hierarchical fuzzy TOPSIS method for consultant selection for Malaysian Department of Irrigation and Drainage infrastructure projects. Alencar and Almeida (Citation2010) applied PROMETHEE VI to select consultants for a construction project considering three subjective (culture, design, and quality) and three objective (cost, time, and experience) criteria. Chu (Citation2011) suggested centroid ranking approach-based fuzzy MCDM method to evaluate consulting firm. The ranking approach of Euclidean distance based on centroid points was applied to determine the ranking order of the alternatives. El-Santawy and El-Dean (Citation2012) combined multi-objective optimization on the basis of ratio analysis (MOORA) method with standard deviation weight method to select a consulting firm for a multi-national manufacturing company to determine the price for its new product. The authors considered three benefit criteria (company size, potential profit, and expected growth) and two cost criteria (cost of the initial consultation and the monthly current installments paid to the firm as its monthly fees). In another study, El-Santawy and Ahmed (Citation2012) presented VIKOR to select the consulting firm considering three benefit criteria (company size, potential profit, and expected growth) and one cost criterion (cost of the consulting). In both studies, the authors did not consider any qualitative criteria. FAHP was applied to select the best Turkish quality consultant for the implementation of ISO 9000 projects considering 3 main attributes and 11 sub-attributes by Cebeci and Ruan (Citation2007). But the authors considered only the qualitative criteria in their analysis. Moreover, due to limitation of Chang (Citation1996) extent analysis, the weights of willingness to help clients, communication and interpersonal skills of the consultant, professional knowledge, expertise of consultants in client’s sector, and problem-solving ability are considered zero. According to Wang, Luo, and Hua (Citation2007), Chang (Citation1996) extent analysis method might assign an irrational zero weight to some useful decision criteria and sub-criteria, leading to them not to be considered in decision analysis. In this study, modified Chang extent analysis is proposed to resolve the limitations of Chang extent analysis. Vayvay et al. (Citation2012) compared AHP, Fuzzy AHP and analytic network process (ANP) for ERP consultant’s selection. However, the authors considered transportation cost, consultancy cost, companies employed, and projects completed as qualitative criteria during their evaluation. Kabir (Citation2014) proposed VIKOR method with fuzzy set theory for consultant selection for automotive battery manufacturing company. But implementation cost and experience in relevant field are also evaluated as qualitative criteria by the decision-makers (DMs).
3. An overview of the Fuzzy AHP and PROMETHEE approaches
In this study, FAHP will apply to structure the decision-making problem and to determine the weights of the criteria and sub-criteria, and PROMETHEE will be used for the aggregation of the criteria, ranking of the alternatives, and sensitivity analysis (Figure ). FAHP and PROMETHEE are detailed theoretically in the following sub-sections.
3.1. Fuzzy AHP
Fuzziness and vagueness are common characteristics in many real-life decision-making problems. Therefore, an effective decision-making model needs to consider vagueness or ambiguity (Kabir & Hasin, Citation2012). The transformation of qualitative preferences to point estimates may not be sensible as DMs often provide uncertain answers rather than precise values (Kabir & Sumi, Citation2014). Conventional AHP that requires the selection of subjective values in pairwise comparison may not be sufficient and uncertainty should be considered in some or all pairwise comparison values (Hofmann & Knébel, Citation2013; Kabir & Hasin, Citation2012; Kahraman et al., Citation2003). The application of Saaty’s AHP has some shortcomings as follows: (1) the AHP method is mainly used in nearly crisp decision applications, (2) the AHP method does not take into account the uncertainty associated with the mapping of one’s judgment to a number, (3) the subjective judgment, selection, and preference of DMs have great influence on the AHP results, (4) ranking of the AHP method is rather imprecise, (5) the AHP method creates and deals with a very unbalanced scale of judgment. Therefore, conventional AHP seems inadequate to capture DMs requirements explicitly (Kabir & Hasin, Citation2012; Kabir & Sumi, Citation2014; Padhi & Mohapatra, Citation2009). FAHP should be more appropriate and effective than conventional AHP as it can take the pessimism/optimism rating attitude of DMs into account, linguistic values, whose membership functions are usually characterized by triangular or trapezoidal fuzzy numbers (Kabir & Hasin, Citation2012; Kutlu & Ekmekçioglu, Citation2012; Sen & Çinar, Citation2010).
Researchers proposed several methods like geometric mean method, direct fuzzification method, synthetic extent analysis, fuzzy least square method, fuzzy modification of the logarithmic least squares method, fuzzy preference programming, and two-stage logarithmic programming to attain the priorities in FAHP (Kabir & Hasin, Citation2012). Modified Chang’s extent analysis is utilized in this research to evaluate the focusing problem. Chang (Citation1996) develops a new approach for handling Fuzzy AHP, using triangular fuzzy numbers (TFN) for the pairwise comparison scale of Fuzzy AHP, and using the extent analysis method for the synthetic extent values of the pairwise comparisons. A TFN denoted as = (l,m,u) where l < m < u, has the following triangular type membership function:
(1)
(1)
In this methodology, decision-makers are asked to indicate the relative importance of two evaluation criteria in the same level. Linguistic pairwise comparisons are transformed to corresponding TFNs illustrated in Table .
Table 1. Linguistic variables and fuzzy scales (Kabir & Hasin, Citation2012; Kabir & Sumi, Citation2014).
Geometric mean operations have been used to combine pairwise comparisons of DMs or aggregating group decisions (Davies, Citation1994; Kahraman et al., Citation2003):
(2)
(2)
It is necessary to check the consistency ratio of the comparison after aggregating group decisions. In this study, graded mean integration approach is utilized for defuzzifying the matrix (Kabir & Sumi, Citation2014). According to the graded mean integration approach, a fuzzy number = (l,m,u) can be transformed into a crisp number by employing Equation (Equation3
(3)
(3) ) (Kutlu & Ekmekçioglu, Citation2012):
(3)
(3)
After the defuzzification of each value in the matrix, ‘Consistency Ratio’ (CR) of the matrix has been calculated and checked whether CR is smaller than .10 or not (Hofmann & Knébel, Citation2013; Kabir & Hasin, Citation2012; Kahraman et al., Citation2003).
In this study, modified Chang extent analysis method is used to derive the importance weights of criteria from pairwise comparisons (Kabir & Sumi, Citation2014). Let W be the normalized weight vector of triangular fuzzy comparison matrix A, which includes the importance weights of criteria in the crisp form. The steps for calculating this vector are as follows.
The first step is to calculate the fuzzy synthetic extent value of each pairwise comparison. Denote as the TFN related to the pairwise comparison of criterion i over criterion j, which is represented as (lij, mij, uij). According to Chang (Citation1996), the value of fuzzy synthetic extent with respect to the criterion i, denoted as
= (li, mi, ui), can be obtained via Equation (Equation4
(4)
(4) ):
(4)
(4)
where n is the size of the fuzzy judgment matrix A.
To obtain , fuzzy addition operation can be performed as
(5)
(5)
and to obtain , fuzzy addition operation of
values can be performed as
(6)
(6)
The inverse vector of the Equation (Equation6(6)
(6) ) can be determined using Equation (Equation7
(7)
(7) ):
(7)
(7)
Wang et al. (Citation2007) corrected the normalization formula (Equation (Equation7(7)
(7) )) as Equation (Equation8
(8)
(8) )
(8)
(8)
The second step is to derive fuzzy ranking value of . In this step,
is compared to other synthetic extent values of A,
= (lj, mj, uj). According to Chang Citation(1996), the degree of possibility of
is obtained by the following equation:
(9)
(9)
where d is the ordinate of the highest intersection point between and
. To compare
and
, both the values of V (
) and V (
) are needed.
The degree of possibility defined by the extent analysis method is an index for comparing two TFNs rather than an index for calculating their relative importance (Kabir & Hasin, Citation2012). Therefore, normalized degrees of possibility can only show to what degree a TFN is greater than all the others, but cannot be used to represent their relative importance (Kabir & Sumi, Citation2014; Wang et al., Citation2007). To resolve this problem, Liou and Wang (Citation1992) proposed total integral value with index of optimism, which derives the priorities of the synthetic extent values of A by the following equation:
(10)
(10)
where α is the index of optimism which represents degree of optimism for decision-makers. If α approaches 1 in [0, 1], the decision makers are more optimistic and otherwise they are more pessimistic (Kabir & Hasin, Citation2012; Kabir & Sumi, Citation2014; Sen & Çinar, Citation2010).
Finally, the normalized importance weight vector W = (w1, w2, … , wn)T of the fuzzy judgment matrix A is determined using following equation:
(11)
(11)
where wi is a non-fuzzy number.
3.2. The PROMETHEE approach
The PROMETHEE is developed by Brans (Citation1982) and further extended by Brans and Vincke (Citation1985) and Brans and Mareschal (Citation1995). It belongs to the methods of partial aggregation, or also called outranking methods (Brans & Vincke, Citation1985). The actions are first pairwise compared with each criterion according to the DMs preferences to generate the local scores. These local scores are then aggregated to a global score, which lead to the PROMETHEE I or II ranking (Brans, Citation1982; Brans & Vincke, Citation1985; Kabir & Sumi, Citation2014).
A number of versions of the PROMETHEE method have been developed like PROMETHEE I (partial ranking; Brans, Citation1982), PROMETHEE II (complete ranking; Brans, Citation1982), PROMETHEE III (ranking based on intervals; Brans & Vincke, Citation1985), PROMETHEE IV (continuous case; Brans & Vincke, Citation1985), PROMETHEE GAIA (geometrical analysis for interactive assistance; Mareschal & Brans, Citation1988), PROMETHEE V (MCDA including segmentation constraints; Brans & Mareschal, Citation1992), and PROMETHEE VI (representation of the human brain, Brans & Mareschal, Citation1995; Kabir & Sumi, Citation2014; Kabir et al., Citation2013). In this section, PROMETHEE I, PROMETHEE II, and GAIA methods are described briefly. For more detailed information about these methods, interested readers are referred to Brans and Mareschal (Citation1994, 2005).
3.2.1. Theoretical basis
In PROMETHEE, the preference structure for each criterion is based on pairwise comparisons (Brans & Mareschal, Citation2005). The deviation between the evaluations of two alternatives on a particular criterion is considered. For larger deviations, the decision-maker allocates a larger preference to the best alternative or possibly no preference if the deviation is negligible. The larger the deviation, the larger the preference will be (Kabir & Sumi, Citation2014). These preferences may vary between 0 and 1.
Thus, function for each criterion will be:
(12)
(12)
For which,
(13)
(13)
Equation (Equation12(12)
(12) ) indicates the preference of x over y for observed deviations between their evaluations on criterion gj(.), in case of a criterion to be maximized. For criteria to be minimized, the preference function should be reversed:
(14)
(14)
A generalized criterion {gj(.), Pj(x,y)} has to be defined for each criterion. Table shows six types of particular preference functions which are used in this study (Brans & Mareschal, Citation2005; Gervasio & da Silva, Citation2012; Kabir & Sumi, Citation2014). However, other types of generalized criteria may also be considered.
Table 2. Types of generalized criteria (Kabir & Sumi, Citation2014).
3.2.2. PROMETHEE I methodology
For the application of PROMETHEE I, two major actions like the calculation of aggregated preference indices and outranking flows are needed. The aggregate preference indices π(x,y) expresses with which degree x is preferred to y over all the criteria, while π(y,x) indicates how y is preferred to x. Assuming :
(15)
(15)
π(x,y) ≈ 1, implies a strong global preference of x over y, while, π(x,y) ≈ 0, implies a weak global preference of x over y (Brans & Mareschal, Citation2005). Each alternative x faces (n − 1) other alternatives in A. Two outranking flows can be defined:
(i) The positive outranking flow:
(16)
(16)
expressing how an alternative x is outranking all the others. The higher , the better the alternative (Kabir & Sumi, Citation2014).
(ii) The negative outranking flow:
(17)
(17)
representing how an alternative x is outranked by all the others. The lower , the better the alternative (Kabir & Sumi, Citation2014).
The PROMETHEE I partial ranking is obtained from the positive and negative outranking flows:(18)
(18)
where PRI, IDI, and ICI represent the preference, indifference, and incomparability, respectively. In the case of xICIy, a higher power of one alternative is associated to a lower weakness of the other. In this case, the information provided by both flows is not consistent and both alternatives are considered incomparable (Gervasio & da Silva, Citation2012; Kabir & Sumi, Citation2014).
3.2.3. PROMETHEE II methodology
PROMETHEE II shows a complete ranking of the alternatives. In this case, the net outranking flow is defined as the balance between the positive and the negative outranking flows:
(19)
(19)
In PROMETHEE II, all the alternatives are comparable; no incomparability’s remain (Brans & Mareschal, Citation2005). When Ø(x) > Ø(y), x outranks y on all the criteria (Kabir & Sumi, Citation2014).
3.2.4. GAIA plane
Geometrical analysis for interactive aid (GAIA) provides a visual guidance for the principal criteria that are used for ranking of the alternatives based on the PROMETHEE II method (Kabir & Sumi, Citation2014). GAIA matrix is constructed from a decomposition of the net outranking flows Ø(x). The matrix data is then processed by a Principal component analysis (PCA) algorithm and displayed on GAIA biplot (Keller, Massart, & Brans, Citation1991). PCA is used to reduce the number of dimensions while minimizing the loss of information. This transformation of a multi-criteria problem to a two-dimensional space and geometrical representation of relations between criteria and alternatives provides a new perspective to the problem with the inevitable loss of some relation characteristics (Kabir & Sumi, Citation2014). It measures the amount of information preserved in the GAIA plane (values larger than 70% correspond to reliable GAIA planes) to control its quality (Herva & Roca, Citation2013; Kabir & Sumi, Citation2014; Visual PROMETHEE, Citation2013).
4. Application of the proposed methodology
The proposed method has been implemented on a furniture manufacturing company situated in the eastern part of Bangladesh and having more than one decade of successful operations. This study was unable to mention the real name of the organization under investigation as a condition of obtaining access for data collection. The company, which began manufacturing, retail, and export in the early 1990s, was one of the largest furniture manufacturing plants in Bangladesh. The area of the factory is more than 350,000 square feet and total number of employees is almost 2500. The company has more than 60 outlets and showrooms for sales. Currently, the company has joint venture with Bangladesh–Denmark and is exporting to Denmark, Netherland, India, Ukraine, Sweden, Germany, Switzerland, Singapore, Thailand, and Japan. The organization has decided to increase the overall production and customer satisfaction from 2012. For this, Board of Directors wants to apply TQM for getting better competitive advantages. That is why the company was willing to select appropriate TQM consultants.
To select the most appropriate TQM consultant, the company adopted the proposed method and set up a consultant selection decision panel. Twelve specialists with average work experiences of 13 years were chosen to form the decision team. Table shows the profiles of the experts.
Table 3. Experts profiles.
Initially, a literature review was conducted to identify various criteria and sub-criteria considered by the researchers and decision-makers (Cebeci & Ruan, Citation2007; Cheung et al., Citation2002; Chow & Ng, Citation2007; Chu, Citation2011; Kabir, Citation2014; Ling, Citation2003; Ng & Chow, Citation2004; Omar et al., Citation2009; Saremi et al., Citation2009; Tsai et al., Citation2007, 2009; Vayvay et al., Citation2012). Thereafter, these criteria were placed in front of the expert panel to elicit the most appropriate and widely considered criteria from their experience and judgment for selection of TQM consultant. The expert panel selected six TQM consultants (further to be named as Con A to Con F) for further evaluation. The expert panel generate a decision hierarchy model which is shown in Figure .
After the decision hierarchy model was defined, the proposed approach in the earlier section was applied. To determine the importance weights of the criteria and sub-criteria, the expert panel were asked to make pairwise comparisons for the main and sub-criteria using fuzzy scales shown in Table . The geometric mean of the individual assignments has been determined to obtain overall or group results using Equation (Equation2(1)
(1) ). Table indicates the comparison matrix for the criteria with respect to the overall goal or objective.
Table 4. Pairwise comparison matrix and relative weights with respect to goal.
The consistency ratio of the defuzzified comparison matrix (Table ) is .032. As CR < .1, the level of inconsistency present in the information stored in comparison matrix is satisfactory. Because the other calculations are similar for each comparison matrix, those are not given here and can be done simply according the computations below. The value of fuzzy synthetic extent with respect to the ith object (i = 1, … ,4) is calculated as
The integral values of these values were calculated as below:
ITA = .366; IER = .345; IEC = .142; IKR = .171.
After obtaining the total integral values using Equation (Equation10(10)
(10) ), the importance weights of the main criteria were obtained via normalization (Equation (Equation11
(9)
(9) )): W = (.357, .337, .139, .167).
Similarly, comparison matrix for the sub-criteria was determined and their weights were calculated, which are given in Table .
Table 5. Overall synthesized weighted scores.
The input details (i.e. it has to be minimized or maximized, quantitative and qualitative data, and unit for each criterion) of six consultants are presented in Table . Among all the criteria or attributes, average project completion time (PC, months), work experience in related field (WE, number of years), and implementation cost (IC, Tk. × 1000) are considered as quantitative criteria and rest of the attributes are considered as qualitative criteria. For this purpose, a 7-point qualitative scale ranging from very low, low, below average, average, above average, high to very high has been applied in this study. Among them average project completion time and implementation cost are considered as non-beneficial attributes and lower values are desirable.
Table 6. Criterion details.
In this study, seven scenarios have been considered. For quantitative and qualitative assessments, the PROMETHEE guidelines advise to apply a linear preference function and Usual or Level preference function, respectively (Turcksin et al., Citation2011). Therefore, for the first scenario, Linear and Usual preference functions has been applied for quantitative and qualitative assessment, respectively. For the remaining six scenarios, Usual, U-shape, V-shape, Level, Linear, and Guassian preference function have been used for both qualitative and quantitative criteria. After the determination of the evaluation matrix and the preference functions, the scenarios are evaluated and ranked by means of the PROMETHEE decision-making software, Visual PROMETHEE (Citation2013). The positive (Ø+) and negative (Ø−) flows (PROMETHEE I) and the net flow (Ø) values (PROMETHEE II) for seven scenarios are displayed in Table . Based on the net preference flow (PROMETHEE II) of the analyzed alternatives, Consultant E outranks all the other alternatives. Table and Figure indicate that Con E is the right choice for the given application under the given conditions. The next choice is Con A and the last choice is Con F.
Table 7. Positive, negative, and net flows for different scenario.
Figure indicates the GAIA plan of the decision problem, where nine-dimensional space of criteria is projected on a two-dimensional plane by means of PCA. The weighing of the criteria and the PROMETHEE II ranking are represented by the decision axis. Alternatives are represented by points and criteria by axes in this plan. Thus, alternatives scoring high on a particular criterion are represented by points located in the direction of the corresponding criterion axis (Brans & Mareschal, Citation1994). To differentiate the different alternatives, the length of the criterion vector is a measure of its power. Its position is related to its evaluations on the set of criteria in such a way that actions with similar profiles will be closer to each other. Criteria expressing similar preferences have axes that are close to each other, whereas conflicting criteria are pointing in opposite directions (Kabir & Sumi, Citation2014). The quality level for visualization is 92.7%, which means that very few information got lost by the projection.
Figure indicates that technical skills, management skills, and responsible & trustworthy are close to each other. Similarly, work experience in related field, reputation, and average project completion time are close to each other. This means that consultants like Con E exhibiting a higher technical skills, management skills and responsible & trustworthy whereas Con A exhibiting a higher work experience in related field, reputation and lower average project completion time. It is thus possible to find consultants that are good on these criteria at the same time. Con F and Con B perform well with respect to communication & interpersonal skills but perform worse for rest of the criteria.
The sensitivity analysis of the proposed model is performed as PROMETHEE I and II rankings are greatly influenced by the weights allocated to the criteria. To modify the weights and to observe the resulting modifications of the PROMETHEE II ranking, a special feature of the Visual PROMETHEE software, called The Walking Weights is applied in this study (Figure ). The lower bar chart is showing the weights of the criteria, whereas the upper bar chart indicate PROMETHEE II complete ranking. The slider allows to change the weight of the selected criteria and to see the impact (Kabir & Sumi, Citation2014). For the following preference function, it can be easily observed that Con E still dominates the other ones followed by Con A.
5. Conclusions
Meditating entities such as consultants can play an important role for successfully implementing TQM program and help the manufacturing organizations to attain competitive advantage and improve organizational effectiveness. However, the TQM consultant selection problem is often influenced by uncertainty in practice and in real-life decision-making process as the decision-makers are unable (or unwilling) to express his/her preferences precisely in numerical values and the evaluations are very often expressed in linguistic terms.
To deal with this kind of situation, fuzzy set theory is an appropriate tool. In this study, FAHP has been proposed to determine the weights of the criteria and sub-criteria as PROMETHEE does not provide any formal guidelines for weighing up to now. On the other hand, PROMETHEE enriches FAHP by associating a preference function to each criterion. For this purpose, an integrated approach of FAHP and PROMETHEE has been proposed, in which the strengths of both methodologies are combined in a single MCDM tool to select the most appropriate TQM consultant.
The proposed decision support tool is very flexible and can handle any number of quantitative and qualitative attributes simultaneously. The proposed method not only enables us to determine the outranking order of the consultants, but also assess and rate them. It is also capable to identify groups of criteria expressing similar preferences and to better understand the conflicts that have to be solved in order to make a decision. The GAIA plane enables a graphical representation of the alternatives and criteria and helps to explore the strong and weak points of the alternatives. As research limitations, to get more effective decision, detail firm evaluation criteria, and sub-criteria can be incorporated. For further research, the results of this study can be integrated and/or compared with that of other MCDM methods like TOPSIS, ELECTRE, VIKOR, and MOORA. Other network-based techniques like ANP, Cognitive mapping can be also implemented to handle to the interdependencies between the criteria.
References
- Ahire, S. L., & Rana, D. S. (1995). Selection of TQM pilot projects using an MCDM approach. International Journal of Quality & Reliability Management, 12, 61–81.
- Alencar, L. H., & Almeida, A. T. (2010). A model for selecting project team members using multicriteria group decision making. Pesquisa Operacional, 30, 221–236.
- Al-Khalifa, K., & Aspinwall, E. (2008). Critical success factors of TQM: A UK study. International Journal of Productivity and Quality Management, 3, 430–443.10.1504/IJPQM.2008.019759
- Brans, J. P. (1982). The engineering decision: Development of instruments to support the decision. The PROMETHEE method. Québec: Université Laval.
- Brans, J. P., & Mareschal, B. (1992). PROMETHEE V-MCDM problems with segmentation constraints. INFOR, 30, 85–96.
- Brans, J. P., & Mareschal, B. (1994). The PROMCALC & GAIA decision support system for multicriteria decision aid. Decision Support Systems, 12, 297–310.10.1016/0167-9236(94)90048-5
- Brans, J. P., & Mareschal, B. (1995). The PROMETHEE VI procedure. How to differentiate hard from soft multicriteria problems. Journal of Decision Systems, 4, 213–223.10.1080/12460125.1995.10511652
- Brans, J. P., & Mareschal, B. (2005). PROMETHEE methods. In J. Figueira, S. Greco, & M. Ehrgott (Eds.), Multiple criteria analysis – State of the art surveys. International series in operations research and management sciences (Vol. 78, pp. 163–195). New York, NY: Springer.
- Brans, J. P., & Vincke, P. (1985). A preference ranking organization method (The PROMETHEE method for multiple criteria decision making). Management Science, 31, 647–656.10.1287/mnsc.31.6.647
- Cebeci, U., & Ruan, D. (2007). A multi-attribute comparison of Turkish quality consultants by fuzzy AHP. International Journal of Information Technology & Decision Making, 6, 191–207.
- Chang, D. Y. (1996). Applications of the extent analysis method on fuzzy AHP. European Journal of Operational Research, 95, 649–655.10.1016/0377-2217(95)00300-2
- Cheung, F., Kuen, J. L. F., & Skitmore, M. (2002). Multicriteria evaluation model for selection of architectural consultants. Construction Management and Economics, 20, 569–580.10.1080/01446190210159818
- Chow, L. K., & Ng, S. T. (2007). A fuzzy gap analysis model for evaluating the performance of engineering consultants. Automation in Construction, 16, 425–435.10.1016/j.autcon.2006.07.010
- Chu, T. C. (2011). Evaluating consulting firms using a centroid ranking approach based fuzzy MCDM method. EUSFLAT-LFA, 1, 112–118.
- Davies, M. A. P. (1994). A multicriteria decision model application for managing group decisions. Journal of the Operational Research Society, 45, 47–58.10.1057/jors.1994.6
- El-Santawy, M. F., & Ahmed, A. N. (2012). Evaluating consulting firms using VIKOR. Life Science Journal, 9, 5872–5874.
- El-Santawy, M. F., & El-Dean, R. A. Z. (2012). Selection of a consulting firm by using SDV-MOORA. Life Science Journal, 9, 171–173.
- Gervasio, H., & da Silva, L. S. (2012). A probabilistic decision-making approach for the sustainable assessment of infrastructures. Expert Systems with Applications, 39, 7121–7131.10.1016/j.eswa.2012.01.032
- Herva, M., & Roca, E. (2013). Ranking municipal solid waste treatment alternatives based on ecological footprint and multi-criteria analysis. Ecological Indicators, 25, 77–84.10.1016/j.ecolind.2012.09.005
- Hofmann, E., & Knébel, S. (2013). Alignment of manufacturing strategies to customer requirements using analytical hierarchy process. Production & Manufacturing Research: An Open Access Journal, 1, 19–43.
- Hur, M. H. (2009). The influence of total quality management practices on the transformation of how organizations work. Total Quality Management, 20, 847–861.10.1080/14783360903128306
- Jung, Y. J., Wang, J. Y., & Wu, S. (2009). Competitive strategy, TQM practice, and continuous improvement of international project management: A contingency study. International Journal of Quality & Reliability Management, 26, 164–183.
- Kabir, G. (2014). Consultant selection for quality management using VIKOR method under fuzzy environment. International Journal of Multicriteria Decision Making, 3, 381–398.
- Kabir, G., & Hasin, M. A. A. (2012). Integrating modified Delphi method with fuzzy AHP for optimal power substation location selection. International Journal of Multicriteria Decision Making, 3, 381–398.
- Kabir, G., Sadiq, R., & Solomon Tesfamariam, S. (2013). A review of multi-criteria decision-making methods for infrastructure management. Structure and Infrastructure Engineering. doi:10.1080/15732479.2013.795978
- Kabir, G., & Sumi, R. S. (2014). Hazardous waste transportation firm selection using fuzzy analytic hierarchy and PROMETHEE methods. International Journal of Shipping and Transport Logistics.
- Kahraman, C., Cebeci, U., & Ulukan, Z. (2003). Multi-criteria supplier selection using fuzzy AHP. Logistics Information Management, 16, 382–394.10.1108/09576050310503367
- Kalogeras, N., Baourakis, G., Zopounidis, K., & Dijk, G. (2005). Evaluating the financial performance of agri-food firms: A multicriteria decision-aid approach. Journal of Food Engineering, 70, 365–371.10.1016/j.jfoodeng.2004.01.039
- Keller, H. R., Massart, D. L., & Brans, J. P. (1991). Multicriteria decision making: A case study. Chemometrics and Intelligent Laboratory Systems, 11, 175–189.10.1016/0169-7439(91)80064-W
- Khan, M. A. (2011). Total quality management and organizational performance-moderating role of managerial competencies. International Journal of Academic Research, 3, 453–458.
- Kumar, V., Choisne, F., Grosbois, D., & Kumar, U. (2009). Impact of TQM on company’s performance. International Journal of Quality & Reliability Management, 26, 23–37.
- Kutlu, A. C., & Ekmekçioglu, M. (2012). Fuzzy failure modes and effects analysis by using fuzzy TOPSIS-based fuzzy AHP. Expert Systems with Applications, 39, 61–67.10.1016/j.eswa.2011.06.044
- Ling, Y. Y. (2003). A conceptual model for selection of architects by project managers in Singapore. International Journal of Project Management, 21, 135–144.10.1016/S0263-7863(02)00014-5
- Liou, T. S., & Wang, M. J. (1992). Ranking fuzzy numbers with integral value. Fuzzy Sets and Systems, 50, 247–255.10.1016/0165-0114(92)90223-Q
- Macharis, C., Springael, J., Brucker, K. D., & Verbeke, A. (2004). PROMETHEE and AHP: The design of operational synergies in multicriteria analysis. Strengthening PROMETHEE with ideas of AHP. European Journal of Operational Research, 153, 307–317.10.1016/S0377-2217(03)00153-X
- Macinati, S. M. (2008). The relationship between quality management systems and organizational performance in the Italian National Health Service. Health Policy, 85, 228–241.10.1016/j.healthpol.2007.07.013
- Mareschal, B., & Brans, J. P. (1988). Geometrical representations for MCDA. The GAIA module. European Journal of Operational Research, 34, 69–77.10.1016/0377-2217(88)90456-0
- Munhurrun, R. P., & Munhurrun, V. (2008). TQM sustainability for competitive advantage. The International Journal of Environmental, Cultural, Economic and Social Sustainability, 6, 37–50.
- Ng, S. T., & Chow, L. K. (2004). Framework for evaluating the performance of engineering consultants. Journal of Professional Issues in Engineering Education and Practice, 130, 280–288.
- Omar, M. F., Trigunarsyah, B., & Wong, J. (2009). A design science approach for consultant selection decision support system. Proceedings of Fourth International Conference on Cooperation and Promotion of Information Resources in Science and Technology, Beijing, China, 21–23 November, pp. 90–94.
- Padhi, S. S., & Mohapatra, P. K. J. (2009). Contractor selection in government procurement auctions: A case study. European Journal of Industrial Engineering, 3, 170–186.10.1504/EJIE.2009.023604
- Psychogios, A. G., & Priporas, C. V. (2007). Understanding total quality management in context: Qualitative research on managers’ awareness of TQM aspects in the Greek service industry. The Qualitative Report, 12, 40–66.
- Salaheldin, I. (2009). Critical success factors for TQM implementation and their impact on performance of SMEs. International Journal of Productivity and Performance Management, 58, 215–237.10.1108/17410400910938832
- Saremi, M., Mousavi, S. F., & Sanayei, A. (2009). TQM consultant selection in SMEs with TOPSIS under fuzzy environment. Expert Systems with Applications, 36, 2742–2749.10.1016/j.eswa.2008.01.034
- Sen, C. G., & Çinar, G. (2010). Evaluation and pre-allocation of operators with multiple skills: A combined fuzzy AHP and max–min approach. Expert Systems with Applications, 37, 2043–2053.10.1016/j.eswa.2009.06.075
- Shenawy, E. E., Baker, T., & Lemak, D. J. (2007). A meta-analysis of the effect of TQM on competitive advantage. International Journal of Quality & Reliability Management, 24, 442–471.
- Siddiqui, J., & Rahman, Z. (2007). TQM principles’ applications on information systems for empirical goals: A study of Indian organizations. The TQM Magazine, 19, 76–87.10.1108/09544780710720853
- Tan, P. K. L. (1997). An evaluation of TQM and the techniques for successful implementation. Training for Quality, 5, 150–159.10.1108/09684879710188158
- Tsai, W. H., Lin, T. W., Chen, S. P., & Hung, S. J. (2007). Users’ service quality satisfaction and performance improvement of ERP consultant selections. International Journal of Business and Systems Research, 1, 280–301.10.1504/IJBSR.2007.015830
- Tsai, W. H., Shen, Y. S., Lee, P. L., & Kuo, L. (2009). An empirical investigation of the impacts of ERP consultant selections and project management on ERP is success assessment. IEEE International Conference on Industrial Engineering and Engineering Management. Hong Kong, China, 8–11 December, pp. 568–572.
- Turcksin, L., Bernardinia, A., & Macharisa, C. (2011). A combined AHP-PROMETHEE approach for selecting the most appropriate policy scenario to stimulate a clean vehicle fleet. Procedia Social and Behavioral Sciences, 20, 954–965.10.1016/j.sbspro.2011.08.104
- Vayvay, O., Ozcan, Y., & Cruz-Cunha, M. M. (2012). ERP consultant selection problem using AHP, fuzzy AHP and ANP: A case study in Turkey. E3 Journal of Business Management and Economics, 3, 106–117.
- Visual PROMETHEE. (2013). Retrieved January, from http://www.promethee-gaia.net/software.html
- Wali, S., & Boujelbene, Y. (2010). The effect of TQM implementation on firm performance in the Tunisian context. International Journal of Productivity and Quality Management, 5, 60–74.10.1504/IJPQM.2010.029510
- Wang, J. J., & Yang, D. L. (2007). Using a hybrid multi-criteria decision aid method for information systems outsourcing. Computers & Operations Research, 34, 3691–3700.
- Wang, Y. M., Luo, Y., & Hua, Z. (2007). On the extent analysis method for fuzzy AHP and its applications. European Journal of Operational Research, 186, 735–747.
- Zehir, C., & Sadikoglu, E. (2010). The relationship between total quality management (TQM) practices and organizational performance: An empirical investigation. International Journal of Production Economics, 101, 1–45.