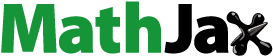
Abstract
Companies can gain a competitive edge by managing closed-loop supply chains efficiently. This requires the joint consideration of forward and reverse logistic processes. The acquisition of used products is an important factor within the closed-loop context, as the availability of acquired used cores has a direct effect on the manufacturing–remanufacturing disposition strategy. Thus, the acquisition process and the production disposition are highly interrelated. In further consequence, the linked view on an active acquisition process combined with manufacturing–remanufacturing decisions is essential. In this paper, the optimal strategies concerning the acquisition of used cores, manufacturing of new products, and remanufacturing are studied analytically in a two-period model with stochastic, newsvendor-like demands. Given the closed-loop setting, the quantity acquired and remanufactured in the second period is limited to the sales of new products in the first period. An extensive numerical study provides insights into the influence of an active acquisition process on quantities and profit.
1. Introduction and motivation
An observable trend in supply chain management is the change from a view on forward logistic activities including disposal logistics to reverse logistics (RL) and closed-loop supply chains (CLSC) integrating coordinated forward and reverse processes. Hewlett-Packard (HP) and IBM exemplify this, as both established various RL activities. In 2011, HP reused 26,700 tons of electronic products directly, and additionally collected 133,900 tons of used electronic products for recycling (HP, Citation2011). Similar to HP, IBM reprocessed around 37,950 tons of used electronic equipment in 2011. In detail, 6.6% of the collected products could be reused, 38.6% were sold to reproducers, and 52.4% were recycled to obtain reusable raw materials (IBM, Citation2011). This evolution of CLSCs is driven by both exogenous and endogenous factors: competition, resource scarcity, and legislation – like the well-known WEEE (WEEE, Citation2014) – force companies to act sustainably and resource-efficiently. On the other hand, various case studies indicate that the implementation of reusing and reprocessing concepts in companies often leads to an increased profitability (e.g. de Brito, Dekker, & Flapper, Citation2004 or Guide, Teunter, & Van Wassenhove, Citation2003).
Following some significant research work (Guide & Van Wassenhove, Citation2006, Citation2009; Thierry, Salomon, Van Nunen, & Van Wassenhove, Citation1995), the activities related to RL and CLSCs are acquisition of used products, testing, sorting, and quality grading of the acquired products, product disposition, reprocessing with different recovery options (e.g. remanufacturing, refurbishing, recycling, etc.), and remarketing. The optimization of inventory systems (Toktay, Wein, & Zenios, Citation2000; Van Der Laan, Salomon, Dekker, & Van Wassenhove, Citation1999), the design of closed-loop supply networks (Fleischmann, Citation2001), or production lot sizing (Tang & Teunter, Citation2006) are examples of applying methods of operations research to related fields of research. However, considering isolated activities or processes does not allow a holistic view on CLSCs. Besides many more interrelated processes in a CLSC, the link between acquisition of used products and decisions concerning manufacturing/remanufacturing exemplifies this issue. The acquisition of used products can often be controlled by the effort spent for, e.g. offering incentives like discounts or costly measures like establishing and operating a collection network. Subsequently, the acquired items are the base for the recovery activities in terms of both quantity and quality. Naturally, the amount of recovered goods is limited by the acquired quantity, while the quality of the returns determines potential profitable recovery options. In order to explore the resultant effects of such links, activities in a closed-loop context can be combined to integrated models with interdependent processes.
In this paper, we present a two-period model with a joint consideration of active effort-dependent acquisition of used products and a manufacturing–remanufacturing disposition process. Furthermore, stochastic, newsvendor-like demand occurs in both periods. While in the first period new products can be manufactured to supply the market, the demand in the second period can be satisfied either by producing new items or remanufacturing used products. In order to ensure the supply with used cores for remanufacturing, new products sold in the first period can be acquired by spending some costly effort. In an extension of the model, the option to store excess production of the first period to offer it in the second period is included. The optimal strategies with respect to manufacturing, remanufacturing, and potential inventory decisions can be determined for both models. Numerical analyses provide insights into the profitability, the optimal acquisition effort, and the quantity decisions in the models.
The main trade-off in the model is the interplay between sales in the first period and the spent acquisition effort to obtain an optimal pool of used products to acquire for remanufacturing in the second period. There are two options to control the supply with used products: the producer can either stimulate expected sales by excess production in the first period or the acquisition quantity is raised by spending an increased acquisition effort. In the extended model, another trade-off concerning costs for storing excess production in the first period, and costs for acquisition and remanufacturing of used products in the second period occurs.
The practical motivation for the model is the fact that many products are limited to two usage phases, one life cycle as a new and one as a second-life product. Toner and ink cartridges (InfoTrends, Citation2011) and white goods like washing machines (Sepp Eisenriegler, head of R.U.S.Z, personal communication, April 24, 2013) are examples for this. The approach using two periods allows to model essential intertemporal dependencies while keeping the system analytically tractable. This technique is widely used in the area of newsvendor models and stochastic programming (see, for instance, Cheaitou, van Delft, Dallery, & Jemai, Citation2009; Chen & Xiao, Citation2011; Linh & Hong, Citation2009; Ravi, Citation2005).
Concerning potential fields of application, the first model without inventory represents two similar but not equal generations of one product. The sold products of the first generation can be collected and remanufactured after their end-of-use, like in the case of gambling machines or washing machines. Regarding the model with the option to store excess production, the practical application is operational (re)manufacturing-planning (e.g. refilling toner cartridges) induced by shorter periods.
The remainder of the paper is organized as follows. Section 2 presents the literature related to our model and the research gap which we work on with this article. The formal model is introduced and related analytical results are shown in Section 3. Section 4 provides insights into the extension of the model to store excess production and the according analytical results. Numerical analyses regarding the model without and with the option to store first-period production are presented in Section 5. Finally, Section 6 includes a summary and an outlook on further research opportunities.
2. Related work
Over the last years, scientists paid high attention on exploring the field of RL and CLSC. In the following, we present scientific work and relate our model to existing literature. Particularly, we analyze the academic work with respect to active acquisition of used products for recovery – often in combination with different processes like grading and/or disposition of acquired used products, demand type, and intertemporal dependencies.
In a couple of scientific papers (Bakal & Akcali, Citation2006; Galbreth & Blackburn, Citation2006, Citation2010; Guide et al., Citation2003; Pokharel & Liang, Citation2012; Robotis, Bhattacharya, & Van Wassenhove, Citation2005; Teunter & Flapper, Citation2011; Vadde, Kamarthi, & Gupta, Citation2007), RL models including an active, controllable acquisition process are presented. Bakal and Akcali explore the effect of stochastic recovery yield on optimal acquisition and sales prices for an end-of-life product when both supply of used cores and demand for recovered products are price-sensitive and therefore controllable. Similar to this setting, Guide et al. provide optimal pricing decisions with respect to acquisition prices of used products and the sales price of remanufactured goods when multiple quality levels are available for acquisition. In two papers, Galbreth and Blackburn derive the optimal acquisition quantities for satisfying a deterministic demand under heterogeneous quality of returns. While in the first paper (Galbreth & Blackburn, Citation2006) the quality distribution of acquired goods is known, this assumption is relaxed in Galbreth and Blackburn (Citation2010). The authors find that the optimal acquired quantity of used products may exceed the consumer demand, as the possible stricter selectivity induced by an increased acquisition quantity allows to remanufacture only items in a good condition. Pokharel and Liang consider a consolidation center which acquires used products with different quality levels from the collection centers to satisfy a deterministic demand of a remanufacturer. The authors provide optimal pricing decisions under uncertain return quantities. In the model presented by Robotis et al., acquired used high- or low-quality products can be sold directly or remanufactured to increase the price of sale. Assuming newsvendor-like demand, the authors show that potential remanufacturing raises the profitability of the company. Teunter and Flapper determine optimal acquisition and production strategies for a model with uncertain quality of returned products and stochastic demand to tackle the problem of the value of quality information. The results show that quality information coming from a grading process always raises profits compared to the case without information. A model including a product recovery facility facing deterministic demand is analyzed by Vadde et al. The comparison of the results of several scenarios with and without a controllable acquisition process shows that an active acquisition process allows reducing costs.
Additionally to active acquisition, Jung and Hwang (Citation2011) and Shi, Zhang, and Sha (Citation2011) introduce CLSC with joint decision-making concerning newly manufactured and recovered products. Optimal acquisition and sales prices are determined in the model studied by Jung and Hwang, where an original equipment manufacturer and a remanufacturer are either competitors or cooperate. Shi et al. consider a production planning-model incurring a joint capacity constraint for a product portfolio. The authors derive optimal production and remanufacturing quantities and optimal prices for the acquisition of used items when both supply and demand are stochastic.
However, all of the articles discussed above are single-period settings without intertemporal dependencies. Approaches linking more than one period can be found in Liang, Pokharel, and Lim (Citation2009), Nenes and Nikolaidis (Citation2012), and Tagaras and Zikopoulos (Citation2008). Liang et al. derive the optimal product acquisition prices considering a stochastic sales price. In order to control the return quantity, the acquisition rate can be influenced by setting incentives. A model to obtain optimal acquisition, remanufacturing, inventory, and salvaging decisions is presented by Nenes and Nikolaidis. The proposed mixed integer linear program can cope with heterogeneous quality levels of acquired products, several suppliers of used products, and multiple periods. Tagaras and Zikopoulos study the design of a supply chain with centralized/decentralized grading processes. The multi-period model includes uncertain demands and deterministic yield in the grading process. Considering settings without, with centralized, or with decentralized sorting, the optimal supply chain design and the value of a sorting procedure is explored.
While these papers deal with RL approaches, the remaining works target at CLSC. A single-period and a finite multi-period manufacturing/remanufacturing model with deterministic demand are studied in the article of Minner and Kiesmüller. Next to the optimal strategy concerning acquisition price and (re)manufacturing quantities, optimal decisions for potential storing of acquired used cores are determined. Toktay et al. present a closed queuing network including the assumption that the sales in past periods are the base for stochastic return flows of used products. The authors explore the impact of several model characteristics like demand rate, return delay, or return probability of used cores.
Finally, a sales-dependent acquisition processes can also be found in a work of Kelle and Silver (Citation1989). The authors consider stochastic returns of reusable containers with assumed loss of products over time. Due to the fact that the returned quantity for reusing is restricted by the sales in previous periods, new production may appear to replace these losses.
In summary, to the best of our knowledge, the presented model is the first approach which integrates the link between acquisition and manufacturing–remanufacturing disposition, stochastic demands, intertemporal dependencies, and a restriction of the maximum acquisition quantity by previous sales. It extends the current literature by following properties: Jung and Hwang (Citation2011), Minner and Kiesmüller (Citation2012), Nenes and Nikolaidis (Citation2012), and Vadde et al. (Citation2007) assume deterministic demands, while we consider stochastic demands. In contrast to the single-period approach of Shi et al. (Citation2011), we use a time horizon of two periods to explore intertemporal effects. Kelle and Silver (Citation1989) and Toktay et al. (Citation2000) assume that the returns are received passively, without the option to control the acquisition process. The restriction of the maximum acquisition quantity to sales in previous periods does not appear in any of the mentioned papers, except for the work of Jung and Hwang (Citation2011), Kelle and Silver (Citation1989), and Toktay et al. (Citation2000).
3. The model
The work in this paper was motivated by models presented by Reimann and Lechner (Citation2012) and Ferrer and Swaminathan (Citation2006) and deals with the optimal planning decisions of a risk-neutral original equipment manufacturer (OEM). Besides production of new products, the company’s activities also cover acquisition of used cores and remanufacturing. We assume a two-period environment with stochastic, newsvendor-like market demands. The demand in the first period is satisfied by new production; as the OEM is considered as a price-taker, overproduction is possible in the first period to stimulate the expected sales. In the second period, the market can be supplied by two kinds of goods which are assumed to be perfect substitutes: newly produced items or remanufactured products in an as-new condition. In order to enable remanufacturing of used items, goods sold in the first period can be acquired with some costly effort. In contrast to the work of Reimann and Lechner (Citation2012) where the return rate is exogenously given and therefore fixed, the core collection rate can be controlled actively by spending a certain acquisition effort. In this way, an increased per-unit acquisition effort leads to an increased return rate, and consequently to a raised acquisition quantity. The core collection rate increases in the average acquisition effort due to efficiency reasons, but at a decreasing rate. Naturally, the expected sales of new products in period one restrict the quantity that can be acquired in the second period. Summing up, the acquired quantity depends on both the effort-dependent core collection rate and the sales in the first period.
In periods , the new production quantities
, the acquisition effort
, and the remanufacturing quantity
are optimized in order to maximize the expected profit
over both periods. The time value of money is implemented by using a discount factor
, which represents the discounted cash flow for profits in the second period; this discount may also be caused by, e.g. technological obsolescence. Parameters
are the per-unit selling prices and production costs for a new unit, respectively. In the case that a product is remanufactured instead of newly manufactured,
is the saving per remanufactured item, i.e. remanufacturing is more efficient than new production
. The acquisition effort
directly influences the strictly increasing, concave, and continuous core collection yield function
. Without loss of generality, we assume that
. Additionally, the resulting cost of the acquisition may not exceed the savings from remanufacturing, as then new production would be more efficient than remanufacturing (therefore,
).
are uncertain demands, following known probability density functions
and cumulative distribution functions
, respectively. At last,
are the expected sales in period t. The offered quantities are
in the first period and
in the second period. In summary, the following Table lists the components of the model.
Table 1. Notation list of model parameters.
In the following Figure a short summary of the contents and highlights of Section 3 is given. We start with the formulation of the model in Section 3.1 and present the related analytical results (Section 3.2). Finally, we complete this part in Section 3.3 with some numerical examples to exemplify the analytical results and to demonstrate some occurring effects.
Figure 1. Overview of the contents of Section 3.
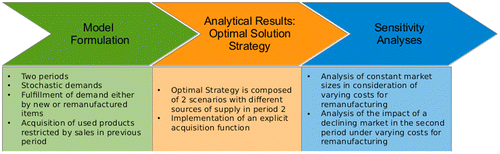
3.1. Formulation of the model
We can formulate the optimization model including the defined requirements as follows:
(1)
(1)
s.t.
(2)
(2)
(3)
(3)
(4)
(4)
The first two terms represent cost for manufacturing and expected revenue in the first period, while the remaining part of the function includes discounted manufacturing- and remanufacturing-related terms of the second period. Constraint (2) ensures that the remanufactured quantity does not exceed the number of acquired used items, while constraint (3) restricts the core collection rate to a maximum of 1. The concavity of the objective function and the convexity of the constraints allow the application of Karush–Kuhn–Tucker conditions (KKT) to the model. All related proofs are available from the authors upon request.
3.2. Analytical results
Concerning the analytical insights, note that the remanufacturing constraint (2) is always binding: . The explanation for this is a basic one: in the hypothetical case that the acquired quantity would be greater than the remanufactured quantity, an adjustment of the acquisition quantity to the remanufacturing quantity would always lead to higher profits due to acquisition cost savings.
The unconstrained single-period newsvendor quantity serves as a reference value to measure a possible quantity deviation in the first period. Therefore, excess production occurs in the case when qt exceeds
.
Depending on the shadow price related to Equation (Equation2
(2)
(2) ), the optimal acquisition and manufacturing–remanufacturing strategy is characterized by the following two scenarios. In both scenarios, excess production in the first period, and acquisition and remanufacturing of used products in the second period takes place.
Scenario M1.1: Excess production in period 1, acquisition & remanufacturing of used products in period 2, and no new production in period 2.
If , the following equations define the optimal production scenario:
(5)
(5)
(6)
(6)
(7)
(7)
(8)
(8)
Scenario M1.2: Excess production in period 1, acquisition & remanufacturing of used products in period 2, and new production in period 2.
If , the following equations define the optimal production scenario:
(9)
(9)
(10)
(10)
(11)
(11)
(12)
(12)
Basically, in scenario M1.2, new production appears in the second period, and in both scenarios remanufacturing of used acquired cores occurs. Naturally, only one of the scenarios can be optimal. In order to obtain the profit-maximizing scenario, the following procedure was applied. First of all, derive the values of scenario M1.2 by using the defined equations. The crucial point is to solve Equation (Equation12(12)
(12) ) numerically to get the optimal value for cr and subsequently, to obtain a solution for the second scenario M1.2. If this computation turns out to be valid with respect to all constraints, scenario M1.2 is the optimal one. Otherwise, proceed with the computation of scenario M1.1. Equation (Equation8
(8)
(8) ) shows that cr increases/decreases with
and vice versa. Furthermore, this property can also be detected concerning the first-period new production q1, as it rises with cr and
(see Equation (Equation5
(5)
(5) )). In consideration of these properties, the optimal profit-maximizing value for
can be found by a numerical search method, e.g. the bisection method.
As mentioned above, an intuitive and cost-minimizing result is that all acquired used products are remanufactured, as constraint (2) is binding in any case. Furthermore, there is always excess production in period 1 due to the fact that . This can be explained by the trade-off between excessive first-period production and acquisition/remanufacturing savings in period 2: raised new production in the first period induces increased expected first-period sales. The improved availability of used products for acquisition in the second period results in increased second-period profits due to less acquisition/remanufacturing costs. Thus, the overall optimal profit is based upon increased gaining in the second period and reduced profit compared with the profit of the single-period newsvendor quantity in the first period.
As the analytical findings shown above are based on a general core collection function, using a specific acquisition function leads to more explicit analytical results. Therefore, a base case acquisition function is introduced:
(13)
(13)
The parameter x allows to control the slope of the acquisition cost functions, and consequently, to increase the variability of potential cost functions. Joint consideration of constraint (3), γ(cr) ≤ 1, acquisition function (13) leads to the requirement . Two further assumptions, cr < δ < c2 and x ≥ 1, lead to the fact that constraint (3) holds for this acquisition function.
In consideration of acquisition function (13), ,
, and
(see Equation (Equation8
(8)
(8) )), or cr = δ/3 (see Equation (Equation12
(12)
(12) )) the optimal scenarios M1.1/M1.2 can be further specified.
Scenario M1S.1: Excess production in period 1, acquisition & remanufacturing of used products in period 2, no new production.
If and
, the following equations define the optimal production scenario:
(14)
(14)
(15)
(15)
(16)
(16)
(17)
(17)
Scenario M1S.2: Excess production in period 1, acquisition & remanufacturing of used products and new production in period 2.
If and
, the following equations define the optimal production scenario:
(18)
(18)
(19)
(19)
(20)
(20)
(21)
(21)
An intuitive illustration why excess production in the first period always occurs can be found: both variables cr and q1 are optimized simultaneously in period 1, but not only the acquisition effort or the new production. This effect can be explained by analyzing Equations (Equation14(14)
(14) ) and (Equation18
(18)
(18) ), respectively. In both cases, an increase/decrease of cr directly leads to a raised/reduced first-period production quantity q1 and vice versa. This ensures the optimal balance of the trade-off between increased costs in the first period and gainings from acquisition/remanufacturing of used products in the second period.
In scenario M1S.1, the acquisition effort cr is below the maximum acquisition effort . A rise in cr directly increases the shadow price
up to a specific maximum value. In the case that this maximum value is reached, the optimal scenario switches to M1S.2. As it is determined by the acquisition effort function, the specific maximum effort is δ/3 when
. Interestingly, this is far below the limit of cr < δ.
3.3. Numerical analysis
In this section, we present some numerical analyses to support the insights obtained from the analytical results. First of all, a numerical base case is introduced. The first-period demand D1 ∼ U(a1, b1), a1 = 25, b1 = 75 is the same as the second-period demand D2 ∼ U(a2, b2), a2 = 25, b2 = 75. The discount factor is β = .9 and both prices p1 = p2 = 10 and costs c1 = c2 = 8 are the same in the first and second period. Remanufacturing savings amount to δ = 4 and the base case includes a value for x of 1. Regarding the effort-dependent acquisition function, Equation (Equation13(13)
(13) ) is implemented. Insights into the sensitivity of the model can be gained by varying savings from remanufacturing δ, which alters remanufacturing conditions for each numerical setup. Thus, δ is increased by .5 in the range of .5 ≤ δ ≤ 7.5 to obtain the results in the whole range from cost-intensive remanufacturing process to high savings from remanufacturing used items.
The analyses considering different savings from remanufacturing (δ) are presented in Figure , covering a case with constant demand on the left and declining demand on the right of the figure. In the case D2 ∼ U(25, 75) on the left side, new production (q2) always occurs in the second period (optimal scenario M1.2). Rising cost savings from remanufacturing lead to a higher possible acquisition effort (), an increased excess production in the first, and higher remanufacturing rates in the second period, as acquisition and remanufacturing of used products becomes more attractive. The remanufacturing cost savings have a strong positive impact on the profits.
Next, we consider the case of a market decline for the product in the second period (D2 ∼ (5, 55)), shown in the right part of Figure . This decline can be driven either by macro-economical factors as observed in the semiconductor industry (see, e.g. King, Citation2012) or by micro-economical impact factors. For example, innovation in high-technology industries leads to substitution of first-generation products by products of the second generation, and subsequently to reduced market potential for first-generation products (e.g. smartphones and microchips). Clearly, an OEM tries to find the optimal manufacturing–remanufacturing strategy under these conditions. Hence, the question when new production in the second period should take place and under which conditions a pure remanufacturing strategy suffices can be answered: for the sufficiently low-expected demand in the second period and a relatively high δ ≥ 4, remanufacturing is the only source of supply in the second period. The reason for this characteristic is the rising profitability of remanufacturing with a more efficient remanufacturing process. In detail, for δ ≥ 4, the acquisition efforts are below the maximum limits compared to the base case () and the second-period new production is zero. Even acquisition efforts which are lower than the potential maximum acquisition efforts ensure a sufficient supply with used products. Thus, no new production is necessary. With respect to the analytical results presented in Section 3.2, this can be explained by the transition from scenario M1.2 to scenario M1.1 due to increased savings from remanufacturing. Additionally, following the analytical results excess first-period production appears in all scenarios (
).
4. The model with inventory carry-over
In this section, the model presented in Section 3 is extended by the option to store excess first-period new production. Due to demand uncertainty, there may remain some unsold items in the first period, which can be stored and sold in the second period. Consequently, inventory can act as a third source of supply, next to new production and remanufacturing. This model represents a production planning problem, considering the production/remanufacturing of the same product types in two sequenced periods. The inventory I causes costs of hI in the first period, while it can be used for sales in period 2 at no charge. A new constraint is introduced in Equation (Equation25(25)
(25) ): Naturally, the stored production quantity I cannot exceed the expected remaining inventory
. Another assumption concerns holding cost: h > βc2 can never be optimal, because in this case new production in period 2 would always be favored over holding inventory due to lower costs. Thus, holding cost is limited by 0 ≤ h ≤ βc2. In order to ensure that producing a quantity greater than the maximum demand in period 1 to store these products cannot occur, we assume c1 + h ≥ βc2. Clearly, the supply quantity for the second-period demand is given by
, so in consequence the expected sales are
.
In analogy to the previous part, we present an overview of the following sections in Figure . First, the model formulation is presented (Section 4.1). Thereafter, analytical results including eight different solution scenarios are shown in Section 4.2. Finally, some numerical examples in Section 4.3 illustrate the results of the analytical section.
Figure 3. Overview of the contents of Section 4.
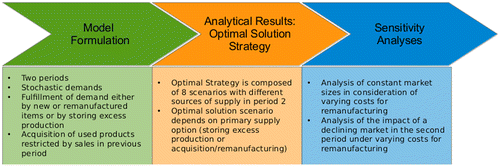
4.1. Formulation of the model
Again, the concave objective function and the convex constraints allow the application of Karush–Kuhn–Tucker conditions. Considering the requirements mentioned above, the model is defined by:
(22)
(22)
s.t.
(23)
(23)
(24)
(24)
(25)
(25)
(26)
(26)
In analogy to the first model, the first three terms of the objective function represent the costs and expected revenue in the first period. The remaining terms are the discounted profit of the second period, including production, acquisition, and remanufacturing costs and expected revenue.
4.2. Analytical results
Depending both on the costs for acquisition/remanufacturing of used products as well as storing first-period production, either remanufacturing (β(c2 − δ) < h) or holding (β(c2 − δ) ≥ h) is economically more efficient and therefore the primary supply option for the second period. In both cases, four different production scenarios define the optimal manufacturing–remanufacturing–inventory strategy. The strategy is presented below: in the left column, storing excess production is the preferred primary supply (h ≤ β(c2 − δ)). On the right-hand side, the case when remanufacturing acquired used products is the preferred primary supply is shown (h > β(c2 − δ)).
The optimal manufacturing–remanufacturing–inventory strategy is determined by the shadow prices related to remanufacturing constraint (23)) and
related to inventory constraint (25)). Naturally, for all under-determined scenarios the shadow prices
and
have to be found by applying a search method.
Table
Some main properties of the solution strategy can be identified. First of all, compared to the analytical results of the model without the possibility to store first period, excess production, acquisition, and remanufacturing of used products does not occur in each of the scenarios. Next, manufacturing of new products in the second period only occurs if full use of the – by definition economically more efficient – options of acquisition/remanufacturing and storing excess production is made but does not suffice to satisfy the demand. Thus, it can never be optimal to start manufacturing in period 2 when the options of storing excess production or acquisition/remanufacturing are not used. Furthermore, similar to the model without inventory, acquisition/remanufacturing of used products always involves excessive first-period production. This is caused by the simultaneous optimization of first-period new production and acquisition effort. Finally, scenario M.2.3A nearly equals M.2.3B and scenarios M.2.4A/M.2.4B are identical. Thus, we discuss them in pairs below.
Concerning the scenarios M2.1.A and M2.2.A of the first sub-strategy, storing first-period production is the primary supply option. In these cases, inventory is sufficient to cover the second-period demand. In the first scenario M2.1.A, the new production in period 1 equals the optimal unconstrained single-period newsvendor quantity. The second-period demand can be fulfilled by the difference between the production quantity in period 1 and the related expected sales. Starting from scenario M2.2.A, excess production occurs in period 1 driven by shadow price . The second-period demand can be fulfilled by the stored first-period production
; thus, excess production in the first period is exclusively used for inventory. In the case that the usage of increased excess production for inventory becomes more costly than acquiring and remanufacturing used products, the optimal strategy is the third scenario M2.3.A; as mentioned above, the third and fourth scenarios are akin/equal in both sub-strategies and therefore discussed in pairs below.
The second sub-strategy, when remanufacturing acquired used products is the preferred first supply option, starts with excess production in period 1 (M2.1.B). This is triggered by the fact that the first-period new production and the acquisition effort are optimized simultaneously. Acquisition and remanufacturing of used products is the only supply for the expected demand in the second period in scenario M2.1.B. With rising acquisition effort cr to acquire more products increases, and storing excess production becomes economically more attractive as a second supply option. When
reaches β(δ − c2) + h, a further raised acquisition effort is more costly than storing excess production. At this stage, additional demand in period 2 is satisfied by inventory
. The optimal scenario is M2.2.B, including acquisition and remanufacturing and storing first-period production quantity.
In the third scenarios M2.3.A/M2.3.B, both inventory and acquisition/remanufacturing of used items occur. The shadow prices as well as
rise with the acquisition effort cr, so the inventory and the acquisition/remanufactured quantity increase simultaneously. Additionally, both shadow prices impact the excessive first-period new production.
Finally, in case that the limits and
are reached (when
) the optimal scenarios switch to the fourth, in which new production q2 appears in the second period due to high costs for acquisition/remanufacturing and inventory, respectively (M2.4.A/M2.4.B).
As only one of the eight scenarios can deliver the optimal solution, a procedure to determine the optimal scenario is presented in the following Figure . The first decision concerning the preferred supply option – holding or acquisition and remanufacturing – is determined by the given parameters. The further process is analogical for both possibilities: starting with scenarios M.2.4.A/M.2.4.B, the feasibility of the solution is checked. In case that the derived solution does not violate any restriction, this is the optimal scenario; otherwise, the results of scenarios M.2.3.A/M.2.3.B are checked and so forth. The same procedure is applied to scenarios M.2.2.A/M.2.2.B. However, if scenarios M.2.2.A/M.2.2.B do not provide any valid results, M.2.1.A/M.2.1.B delivers the optimal solution.
An illustrative example concerning the optimal strategy is presented in Figure , based on acquisition function (13). All of the solution scenarios appear with rising second-period demand on the x-axis and increasing holding costs (0 < h < βc2) on the y-axis. In the area with holding costs between 0 < h ≤ β(c2 − δ) storing is the preferred supply option. While all of the demand in period 2 can be satisfied by storing some or all of the items remaining from producing the newsvendor quantity in area A (M2.1.A), excess production in period 1 is necessary in area B (M2.2.A) to cope with the increased demand. Still, inventory is the exclusive supply option satisfying the second-period demand, but with rising holding costs acquisition/remanufacturing becomes more attractive compared to holding new production. On that account, the area B narrows with increasing holding costs.
Clearly, when costs for holding exceed the cost for remanufacturing, h > β(c2 − δ), the preferred supply option is acquisition and remanufacturing of used products. Relatively low demand in the second period can be satisfied by exclusive acquisition and remanufacturing of used products (area C: M2.1.B). With rising demand, the shadow price increases up to a level of
. In this case, the optimal strategy switches to area D (M2.2.B), and the second-period demand is fulfilled both by acquisition/remanufacturing and some inventory. Increased holding costs make remanufacturing more attractive compared to storing excess production. Consequently, the area C of second-period demands, when M2.1.B is optimal, enlarges with rising holding costs. The same can be observed for area D/M2.2.B: as better conditions for remanufacturing increase first-period new production, the potential inventory quantity increases, too. Therefore, more excess production can be stored to be used in the second period.
According to the analytical results, with a further increase of the second-period demand at first area E (M2.3.A/M2.3.B) and afterwards area F (M2.4.A/M2.4.B) become optimal in all cases. The decrease of area E (M2.3.A/M2.3.B) with rising holding costs can be related to the worsening conditions of holding excess production. Clearly, in the case that the conditions for inventory deteriorate, stored first-period new production is substituted by new production in period 2, and a transition to the area F (M2.4.A/M2.4.B) takes place at a lesser second-period demand.
4.3. Numerical analysis
A similar setting as presented in Section 3.3 is used for the numerical study, extended by the possibility to store first-period production. In order to perform a sensitivity analysis over different costs for storing, the holding costs are varied with values of h = 1, 2, ..., 6, 7 whereby h = 2 is considered as the base case value. Certainly, the proposed holding costs comply with the constraints defined in the analytical section, 0 ≤ h ≤ βc2 and c1 + h ≥ βc2.
Table contains the comparison of different scenarios: one without the possibility to store excess production and two scenarios with low-cost (h = 2) or high-cost (h = 7) inventory. Additionally, both a base-demand and a low-demand scenario are presented. Manufacturing–remanufacturing quantities (), acquisition effort cr, stored first-period production I and the profit π are shown in the table.
Table 2. Sensitivity analyses with varying δ, different h, and different demand.
Regarding the base-demand scenarios, one obvious difference between the presented scenarios is the higher first-period production quantity in the setting with low-cost inventory: despite the risen costs in period 1 due to increased excess production and inventory, the higher production quantity in period 1 increases the profits. This effect occurs because of the more favorable conditions in the second period, in detail, the additional supply option with stored products combined with the raised pool of used products for acquisition. In the case of δ = 7.5, second-period new production still occurs in the settings without storing and with high-cost inventory, but not in the model with possible low-cost inventory. The supply with stored and remanufactured products suffices to cover the second-period expected demand, and the least cost-efficient new production does not occur. Concerning the high-cost inventory case, the difference to the scenario without holding new production is smaller compared to low-cost inventory. Still, the first-period production quantities and the remanufacturing quantities are higher, but due to the rather cost-intensive holding less of the second-period new production quantity can be substituted by inventory. Nevertheless, both the low as well as the high-cost inventory increase the profits in all cases.
The lower part of Table includes the settings with low demand in the second period. All different types of scenarios (M2.1.B, M2.2.B, M2.3.A/M2.3.B, M2.4.A/M2.4.B) of an optimal strategy can be observed with rising δ under holding cost of h = 7 and low demand. Up to δ = 2.5, all three possible supply options occur. Beginning from δ = 3.5, new production in the second period disappears, and the optimal scenario switches from M2.4.A/M2.4.B to M2.3.A/M2.3.B. A further increase of remanufacturing cost savings to δ = 4.5 leads to another transition of the optimal scenario (M2.3.A/M2.3.B to M2.2.B), in which the potential inventory is only partly used. Another raise of δ () results in the reduction of the used supply options to a single one, in particular acquisition and remanufacturing of used products (scenario M2.1.B). Clearly, this case of using only remanufacturing as second-period supply yields the same quantities, acquisition effort, and profit as the model without inventory.
Another interesting effect can be found in Table when the cost for inventory is relatively low (h = 2). With rising remanufacturing cost savings δ both the first-period production quantity q1 and the inventory I firstly increase, then decrease, and finally increase again. This non-trivial result can be explained by the different sources of supply and the structure of the acquisition effort function. The first increase is caused by three properties: firstly, remanufacturing becomes more profitable with a rising δ and consequently, a higher acquisition effort can be chosen. Secondly, storing first-period excess production is rather cheap. Thirdly, increasing q1 raises both the possibly stored first-period production and the pool for acquisition and remanufacturing of used products. Therefore, the better conditions for remanufacturing and the rather beneficial increased stored first-period new production supersede new production in the second period. In detail, a transition within the optimal strategy from scenario M2.4.A/M2.4.B, including all three possible sources of supply, to scenario M2.3.A/M2.3.B without new production in period 2, takes place. At this transition point, changes from βδ to
and accordingly,
due to the fact that the acquisition effort cr deviates from the maximum possible value
. The following decrease of q1 and I is initiated by the acquisition effort function: as the acquisition effort is still at a rather low level, a small increase in cr raises the return rate. The final increase is triggered by the excellent conditions for acquisition and remanufacturing when δ ≥ 4.5: in these numerical settings, the gainings from primarily the increased remanufacturing rate in the second period exceed the cost of excessive production in the first period. Thus, with rising savings from remanufacturing the first-period new production, the acquisition, and remanufacturing quantities, and also the expected inventory rise.
5. Comparative numerical analyses for studying impact
In this section, further numerical analyses and insights are provided. Firstly, the consequences of different acquisition functions on both the models with and without inventory are analyzed in Section 5.1. Furthermore, as the analytical results suggest a joint optimization of the acquisition effort cr as well as the first-period new production q1, the implications of a fixation of one of these variables are studied in Section 5.2.
5.1. The impact of different acquisition functions on decision-making and performance of an OEM
In order to study the consequences of the acquisition process efficiency on the system, we use different explicit acquisition cost functions. Next to the function used in the base case (, see Equation (Equation13
(13)
(13) )), the following equations represent two additional types of cost structures regarding the acquisition of used products:
(27)
(27)
(28)
(28)
In Figure , exemplary return rates of the effort-dependent acquisition functions (13), (27), (28) with varying acquisition efforts are presented (x = 1, cr = .0, .1, ..., 3.9, 4.0).
Constraint (3), γ(cr) ≤ 1, combined with the acquisition functions (13) and (27) leads to the requirement cr/(c2x) ≤ 1. δ is assumed to be greater than cr but less than c2; therefore, cr < c2. x is greater or equal than 1. The exponential function (28) can never violate the restriction as 1 − exp(−cr/x) < 1. Taking all the presented properties into account, constraint (3) holds for all acquisition functions.
The chosen acquisition functions cover a wide range of possible scenarios. For example, the linear function represents an acquisition process where rather high effort is needed to acquire used items, but still may lead to low return rates. An exemplary case is the market of car batteries. Used batteries are collected by several companies, for example, OEM’s, companies doing car battery refurbishing, and scrap dealers. In order to ensure the supply with raw materials or reusable parts, an OEM has to take costly measures like establishing transportation networks or rebuying from scrap dealers to collect/acquire them. Oppositely, the root and the exponential acquisition functions allow acquiring a rather high quantity of used products by spending a reasonable effort. This may occur in, e.g. the market for business copiers. Business copiers cannot only be bought but also rented and leased. Consequently, this ensures the availability of used products for acquisition, as they are returned after use (see, e.g. Chesbrough, Citation2007).
5.1.1. The impact of different acquisition functions on the model without possible inventory
In Table , the results of using different acquisition functions (13), (27), (28) in the model without holding inventory in consideration of varying savings from remanufacturing δ are presented. Here, the quantities, acquisition efforts, and profits strongly differ between the functions. Nearly all of the shown acquisition efforts are at a maximum value. Consequently, new production in period 2 arises in most of the presented optimal results due to the fact that scenario M1.2 is optimal. The only scenario in which new production in the second period does not occur (optimal scenario: M1.1) is the setting including γ(cr) = 1 − exp(−cr/x) and δ = 7.5. This relates to the fact that this acquisition function is the most efficient one, and therefore, excess production in period 1, remanufacturing quantities, and profits are the highest. Furthermore, new production in the second period is rather low in this case, because the bigger part or even the entire demand can be satisfied by remanufacturing. Clearly, the more efficient the acquisition process is the more profitable remanufacturing becomes; consequently, increased excess production and remanufacturing quantities occur. Compared to the profit of two aggregated single-period newsvendor problems of πNV = 114.00, even the least efficient acquisition process γ(cr) = cr/(c2x) yields higher profits.
Table 3. Comparison of different acquisition functions γ(cr) (D2 ∼ U(25, 75), δ = 4).
5.1.2. The impact of different acquisition functions on the model with possible inventory
Similar to above, the analyses are applied to the model with the option of storing excess production and presented in Table . In the base case in the column on the right, the 4th scenario of the optimal strategy, M2.4.A/M2.4.B, is optimal in all cases but the one when δ = 7.5. All three supply sources are used to fulfill the demand (see Section 4.3).
Table 4. Sensitivity analyses over δ for different acquisition functions.
The two additional acquisition functions are shown in the first two columns, again for holding cost of h = 2 and h = 7 and varying δ. Concerning the linear acquisition function on the left, the optimal scenario is M2.4.A/M2.4.B for all values of δ. Compared to the base case function, the linear acquisition function is less efficient. This leads to reduced excess production and acquisition/remanufacturing of used products due to the increased costs. Clearly, the reduced q1 also results in a decreased expected inventory. The missing supply from remanufacturing and storing products is substituted by new production q2, and all three sources of supply are used. In detail, the acquisition effort cr is at the function-specific maximum limit in all cases, and in the sequel,
are at their maximum values. Due to the improving conditions for remanufacturing,
increase and q2 decreases with rising δ.
In the mid-column of the table containing an exponential acquisition function, an interesting transition occurs: up to δ = 2.5 in the base case and δ = 4.5 in the low demand scenario, respectively, new production appears in period 2. In the case of a further increase of δ, the production quantity q2 becomes zero. This effect is based on a switch of the optimal scenario from M2.4.A/M2.4.B to M2.3.A/M2.3.B ( and
) due to the high efficiency of the exponential acquisition function. Exactly at the point where cr falls below the critical limit
the new production in period 2 disappears. When cr is below this maximum value, remanufacturing and storing products are the exclusive supply options, and therefore, q2 = 0. Regarding the profits, the same observation as in the model without the possibility to store first-period excess production can be made: the more efficient the acquisition process is, the more profit can be obtained.
Furthermore, an interesting effect concerning the first-period new production quantities occurs when h = 2. While q1 is higher in the setting with the exponential acquisition function when δ ≤ 4.5 than in the one with the base root function, this flips with increasing savings from remanufacturing. These results can be explained as follows: up to δ = 2.5, both settings use all three possible sources of supply. As the exponential acquisition function is more efficient, only acquisition/remanufacturing and holding excess production appear from δ > 2.5, while the root acquisition function remains in M2.4.A/M2.4.B up to δ = 6.5. As the two supply options are sufficient to fulfill the second-period demand in the case with the exponential acquisition function, the values of both q1 and cr are not at their maximum possible values but below. Consequently, the increase of q1 is decelerated. In the root function case, q1 and cr still are at their maximum values up to δ = 6.5 to gain from high increases. Nevertheless, the profits are less than in the exponential function setting due to the decreased acquisition efficiency and consequently, lower remanufacturing quantities.
Finally, another transition from scenario M2.3.A/M2.3.B to M2.2.B can be observed in the scenario with high inventory cost and the exponential acquisition function: the expected inventory is reduced when δ > 5.5. As acquisition and remanufacturing becomes more and more attractive with a rising δ but holding is still rather expensive, the stored quantity I is decreased and substituted by remanufactured used products.
5.2. Evaluating the importance of a joint optimization of first-period new production and acquisition effort
In Section 3.2, we found that a simultaneous optimization of the first-period new production and the acquisition effort is necessary to obtain the optimal profit. Based on these analytical insights, we present some more numerical experiments where we focus on the influence of the trade-off between first-period new production q1 and the acquisition effort on quantities and profits. Either q1 or cr are not optimized but fixed, so the impact of considering only one variable instead of both can be shown. Therefore, two different scenarios are created to obtain the effects of fixed acquisition efforts and a fixed first-period production quantity on profits and quantities in the systems.
The first scenario contains a fixed first-period new production quantity. The OEM wants to optimize the first-period profit and sets the first-period production quantity to the optimal newsvendor quantity of the unconstrained single-period newsvendor model, in this case q1 = 35. As no excess production is possible, the profit is optimal for a single period, but the supply with acquired used cores for remanufacturing is restricted to this quantity. Thus, the acquisition can only be controlled by the acquisition effort cr. A practical situation where such a behavior can occur is the goal incongruence of different departments within a company. While a production department wants to optimize the new production, a remanufacturing department may suffer from this sub-optimized small production quantity due to reduced returns in the following periods. Related to this, another typical example is the short-term production strategy of companies listed on stock markets to optimize the profit in the financial year by reducing production costs.
In the remaining additional scenario, the acquisition effort is specified as a certain value (cr = .25). As a consequence, the level of acquisition effort is determined and kept constantly. Thus, the only option for the OEM to control the return flow is to change the first-period new production quantity q1. The optimized results of the base case with full optimization of q1, , q2 and cr including acquisition function (13) serves as a reference solution. This scenario can be considered as a decision of a company to limit the acquisition effort to a certain, rather low level.
As can be found in Table , fixing q1 results in a reduction of the remanufacturing quantity compared to the fully optimized case. Contrary, the spent acquisition effort is also at the maximum limit. Consequently, the only cause for this decrease is the reduction of q1. Furthermore, the difference between the profits of the optimal and the fixed q1 scenarios raises with increasing remanufacturing cost savings δ: keeping in mind that cr is already at its maximum value, the missing supply with used products has to be substituted with the increased second-period new production q2. New production is less efficient than remanufacturing, and in the sequel, profits are lower. This effect increases with a raising δ due to the increasing loss of efficiency.
Table 5. Analysis for different values of δ (base case, fixed q1 = 35, fixed cr = .25).
Furthermore, the results of considering a static acquisition effort cr are shown on the right of the table. Fixing the acquisition effort has a huge impact on the variables. In the setting cr = .25, the first-period production quantity q1 is less than q1 in the fully optimized base case. As the losses of further excess production in period 1 would exceed the gainings of an increased pool of used products for acquisition and remanufacturing, q1 is at a rather low level. Naturally, the remanufacturing quantity is also lower, as instead of remanufacturing items the new production quantity in the second period is raised compared to the fully optimized case.
Concerning the profits of the presented scenarios, Figure demonstrates the relative profit decline (RPD) over different remanufacturing cost savings. The optimized base case (optimized q1 and cr) serves as the reference profit value; thus, the deviation of the resulting profits considering the scenarios and cr = .25 compared to the optimal value is presented. In the case that the acquisition effort is fixed to a rather small value (cr = .25), the decline in profit can reach around -25%. The static amount spent for acquisition impedes the profitable acquisition of more used products. This effect worsens with an increasing δ as even more acquired used products could be remanufactured profitably.
Figure 7. Relative profit deviation of settings q1 = 35, cr = .25 compared to fully optimized model.
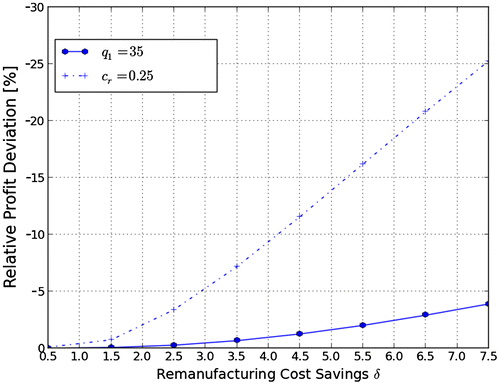
The profit deviation in the case of a fixed first-period quantity q1 = 35 is less dramatic. Nevertheless, it still reaches up to -3.90% in our example. As explained above, the second-period new production substitutes the lack of remanufactured products due to less acquired used products. However, this results in lower profits and consequently, in a higher profit deviation.
Structurally identical results can be observed in the case with the possibility to store first-period excess production. The detrimental effect of a sub-optimization increases in both cases: while there is only a marginal increase of the RPD in the case of cr = .25, the RPD changes dramatically when the first-period new production is fixed to q1 = 35. The explanation for this is rather straightforward: the fixed new production in period 1 restricts the expected inventory to . Therefore, the potential profits of excessive first-period production cannot be realized, neither by inventory nor by acquisition and remanufacturing.
6. Conclusion and further research
In this paper, we presented a two-period model considering a closed-loop supply chain with an actively controlled process for acquiring used products for remanufacturing. Depending on the spent acquisition effort, the return rate can be controlled. The demand is uncertain in both periods, and the expected sales in the first period determine the availability of used products for remanufacturing in the second period. Additionally, an extension to store excess production in the first period and offer the inventory in the second period was included. Depending on the company’s cost structure, we analytically derived the optimal acquisition–manufacturing–remanufacturing (inventory) strategies for both models.
Moreover, we performed some numerical studies to gain insights into the sensitivity of the models. Particularly, we explored and quantified the effects of different numerical settings on manufacturing, remanufacturing, and holding quantities, the acquisition effort, and profits. This was performed for the model without and with the option to store excess first-period production.
Some of our key findings lead to interesting managerial insights. First of all, the option to remanufacture may increase profits compared to a simple new production strategy. Another main result concerns the optimization of the first-period new production quantity and the acquisition effort: as they are linked, a partial optimization of only one variable decreases the possible profits. Therefore, both variables must be optimized simultaneously. Consequently, both the pool of available products for remanufacturing and the effort spent for acquiring those items have to be taken into account to obtain an optimal profit. Related to this result, one difference can be discovered between the model without and the model with the option to store excess production. While in the model without inventory the increase (decrease) of savings from remanufacturing leads to an increase (decrease) of both the first-period new production quantity and the acquisition effort, this is not necessarily the case in the model with inventory. Actually, we presented some numerical scenarios where new production in period 1 first increases, then decreases, and finally, increases again with rising savings from remanufacturing, while the acquisition effort increases in all cases. Furthermore, we found that a declining market may be covered by a pure acquisition/remanufacturing strategy or acquisition/remanufacturing/inventory, respectively. One of the main influencing factors for this is the efficiency of the acquisition process, as a sufficient supply with used products to remanufacture is a basic requirement to satisfy the occurring demands.
Clearly, there exist some limitations concerning the model. First of all, the newly produced and remanufactured items are assumed to be perfect substitutes. Related to this, there exists a lack of potential disposition possibilities, as solely as-new products are demanded. Another critical point is the missing consideration of quality, which would have high impact on acquisition and disposition decisions. Concerning the design of the closed-loop supply chain, we considered a manufacturer which also acquires and remanufactures used products. However, a closed-loop supply chain often involves more players with different business areas. Finally, the presented model is a heuristic concerning the timing of the decisions, as in the current model all variables for the first and second period are optimized at the beginning of the first period.
Nevertheless, these limitations allow many opportunities for further research: besides manufacturing and remanufacturing, multiple recovery options (e.g. refurbishing, recycling) can be considered. Additionally, cannibalizational effects may be explored by coupling the markets for new and recovered products. The assumption of perfect substitutes can be eliminated easily for these extensions. As mentioned above, an interesting extension is the inclusion of heterogeneous quality of used products to study its influence on acquisition and production disposition decisions. The implementation of a grading process to determine the quality of acquired cores with unknown quality would enlarge the field of application of the model. Also extensions with respect to the design of CLSCs are worth to study: for example, the product acquisition or the recovery activities could be performed by dependent or independent, single or multiple firms. Finally, to better reflect the fact that in practice second-period decisions can be taken on the basis of information about first-period sales, the model could be altered to a two-stage stochastic optimization problem.
Acknowledgment
The authors would like to thank Michael Galbreth for his valuable comments.
Additional information
Funding
References
- Bakal, I. S., & Akcali, E. (2006). Effects of random yield in remanufacturing with price-sensitive supply and demand. Production and Operations Management, 15, 407–420.
- de Brito, M. P., Dekker, R., & Flapper, S. D. P. (2004). Reverse logistics: A review of case studies. In B. Fleischmann & A. Klose (Eds.), Distribution logistics: Advanced solutions to practical problems. Lecture notes in economics and mathematical systems (Vol. 544, pp. 243–281). Berlin: Springer.
- Cheaitou, A., van Delft, C., Dallery, Y., & Jemai, Z. (2009). Two-period production planning and inventory control. International Journal of Production Economics, 118, 118–130.10.1016/j.ijpe.2008.08.031
- Chen, K., & Xiao, T. (2011). Ordering policy and coordination of a supply chain with two-period demand uncertainty. European Journal of Operational Research, 215, 347–357.
- Chesbrough, H. (2007). Business model innovation: It’s not just about technology anymore. Strategy Leadership, 35, 12–17.10.1108/10878570710833714
- Ferrer, G., & Swaminathan, J. M. (2006). Managing new and remanufactured products. Management Science, 52, 15–26.10.1287/mnsc.1050.0465
- Fleischmann, M. (2001). Quantitative models for reverse logistics. Lecture notes in economics and mathematical systems (1st ed., Vol. 501). Berlin: Springer.10.1007/978-3-642-56691-2
- Galbreth, M. R., & Blackburn, J. D. (2006). Optimal acquisition and sorting policies for remanufacturing. Production and Operations Management, 15, 384–392.
- Galbreth, M. R., & Blackburn, J. D. (2010). Optimal acquisition quantities in remanufacturing with condition uncertainty. Production and Operations Management, 19, 61–69.10.1111/poms.2010.19.issue-1
- Guide, V. D. R., Teunter, R. H., & Van Wassenhove, L. N. (2003). Matching demand and supply to maximize profits from remanufacturing. Manufacturing and Service Operations Management, 5, 303–316.10.1287/msom.5.4.303.24883
- Guide, V. D. R., & Van Wassenhove, L. N. (2006). Closed-loop supply chains: An introduction to the feature issue (part 1). Production and Operations Management, 15, 345–350.
- Guide, V. D. R., & Van Wassenhove, L. N. (2009). OR FORUM—The evolution of closed-loop supply chain research. Operations Research, 57, 10–18.10.1287/opre.1080.0628
- HP. (2011). HP Global Citizenship: Product reuse and recycling. Retrieved August 21, 2014, from http://www.hp.com/hpinfo/globalcitizenship/environment/reuse/index.html
- IBM. (2011). Product stewardship – IBM 2011 corporate responsibility report. Retrieved August 21, 2014, from http://www.ibm.com/ibm/responsibility/2011/environment/product-stewardship.html
- InfoTrends. (2011). 2011 Western Europe supplies recycling study. Retrieved August 21, 2014, from http://www.hp.com/go/suppliesstudy-emea
- Jung, K. S., & Hwang, H. (2011). Competition and cooperation in a remanufacturing system with take-back requirement. Journal of Intelligent Manufacturing, 22, 427–433.10.1007/s10845-009-0300-z
- Kelle, P., & Silver, E. A. (1989). Purchasing policy of new containers considering the random returns of previously issued containers. IIE Transactions, 21, 349–354.10.1080/07408178908966241
- King, R. (2012). Semiconductor market revenue projected to decline for first time in 3 years. Retrieved August 21, 2014, from http://www.zdnet.com/semiconductor-market-revenue-projected-to-decline-for-first-time-in-3-years-7000003153/
- Liang, Y., Pokharel, S., & Lim, G. H. (2009). Pricing used products for remanufacturing. European Journal of Operational Research, 193, 390–395.10.1016/j.ejor.2007.11.029
- Linh, C., & Hong, Y. (2009). Channel coordination through a revenue sharing contract in a two-period newsboy problem. European Journal of Operational Research, 198, 822–829.10.1016/j.ejor.2008.10.019
- Minner, S., & Kiesmüller, G. (2012). Dynamic product acquisition in closed loop supply chains. International Journal of Production Research, 50, 2836–2851.10.1080/00207543.2010.539280
- Nenes, G., & Nikolaidis, Y. (2012). A multi–period model for managing used product returns. International Journal of Production Research, 50, 1360–1376.10.1080/00207543.2011.609650
- Pokharel, S., & Liang, Y. (2012). A model to evaluate acquisition price and quantity of used products for remanufacturing. International Journal of Production Economics, 138, 170–176.10.1016/j.ijpe.2012.03.019
- Ravi, P. S. (2005). A two-period newsvendor model with concave capacity acquisition costs. Journal of Statistics and Management Systems, 8, 639–657.10.1080/09720510.2005.10701184
- Reimann, M., & Lechner, G. (2012). Production and remanufacturing strategies in a closed loop supply chain: A two-period newsvendor problem. In T.-M. Choi (Ed.), Handbook of newsvendor problems: Models, extensions and applications, international series in operations research and management science (pp. 219–247). New York, NY: Springer.
- Robotis, A., Bhattacharya, S., & Van Wassenhove, L. N. (2005). The effect of remanufacturing on procurement decisions for resellers in secondary markets. European Journal of Operational Research, 163, 688–705.10.1016/j.ejor.2004.01.013
- Shi, J., Zhang, G., & Sha, J. (2011). Optimal production planning for a multi-product closed-loop system with uncertain demand and return. Computers & Operations Research, 38, 641–650.
- Tagaras, G., & Zikopoulos, C. (2008). Optimal location and value of timely sorting of used items in a remanufacturing supply chain with multiple collection sites. International Journal of Production Economics, 115, 424–432.10.1016/j.ijpe.2007.10.026
- Tang, O., & Teunter, R. H. (2006). Economic lot scheduling problem with returns. Production and Operations Management, 15, 488–497.
- Teunter, R. H., & Flapper, S. D. P. (2011). Optimal core acquisition and remanufacturing policies under uncertain core quality fractions. European Journal of Operational Research, 210, 241–248.10.1016/j.ejor.2010.06.015
- Thierry, M., Salomon, M., Van Nunen, J., & Van Wassenhove, L. N. (1995). Strategie issues in product recovery management. California Management Review, 37, 114–135.10.2307/41165792
- Toktay, L. B., Wein, L. M., & Zenios, S. A. (2000). Inventory management of remanufacturable products. Management Science, 46, 1412–1426.10.1287/mnsc.46.11.1412.12082
- Vadde, S., Kamarthi, S. V., & Gupta, S. M. (2007). Optimal pricing of reusable and recyclable components under alternative product acquisition mechanisms. International Journal of Production Research, 45, 4621–4652.10.1080/00207540701449973
- van der Laan, E., Salomon, M., Dekker, R., & Van Wassenhove, L. N. (1999). Inventory control in hybrid systems with remanufacturing. Management Science, 45, 733–747.10.1287/mnsc.45.5.733
- WEEE. (2014). Recast of the WEEE directive – Environment – European Commission. Retrieved August 21, 2014, from http://ec.europa.eu/environment/waste/weee/index_en.htm