ABSTRACT
Industry 4.0 aims to support the factory of the future, involving increased use of information systems and new ways of using automation, such as collaboration where a robot and a human share work on a single task. We propose a classification of collaboration levels for Human-Robot collaboration (HRC) in manufacturing that we call levels of collaboration (LoC), formed to provide a conceptual model conducive to the design of assembly lines incorporating HRC. This paper aims to provide a more theoretical foundation for such a tool based on relevant theories from cognitive science and other perspectives of human-technology interaction, strengthening the validity and scientific rigour of the envisioned LoC tool. The main contributions consist of a theoretical grounding to motivate the transition from automation to collaboration, which are intended to facilitate expanding the LoC classification to support HRC, as well as an initial visualization of the LoC approach. Future work includes fully defining the LoC classification as well as operationalizing functionally different cooperation types. We conclude that collaboration is a means to an end, so collaboration is not entered for its own sake, and that collaboration differs fundamentally from more commonly used views where automation is the focus.
1. Introduction
Historically, automation in industry has been kept separate from human workers for safety reasons, but Industry 4.0 aims to support the factory of the future, increasing effectivity and satisfaction (Bundesministerium für Wirtschaft und Energie, Citation2016; Hermann, Pentek, & Otto, Citation2015; Kalpakjian, Schmid, & Sekar, Citation2014) and recent development in collaborative robotics are leading to robots being incorporated into assembly lines in close proximity to human workers, sharing workspace and tasks. However, introducing human-robot collaboration (HRC) into assembly lines is complicated. For this, theoretically grounded tools are required to help assembly line designers better understand the requirements of both the human and the robot in multiple scenarios. A first step in that direction is to provide a tool for designers to build useful mental models of collaboration when it comes to HRC. Such tools exist for automation, notably levels of automation (LoA) (Frohm, Lindström, Winroth, & Stahre, Citation2008; Shi, Jimmerson, Pearson, & Menassa, Citation2012). LoA exist for, e.g. self-driving vehicles (SAE, Citation2016). Collaboration with automation does not fit easily into the existing levels of automation, as the focus is on achieving full automation, while in HRC the goal is that the human and the robot each perform the parts of the task that they are good at, thus complementing one another (Krüger, Wiebel, & Wersing, Citation2017). We, therefore, suggest levels of collaboration (LoC) that are built in a parallel fashion, instead of the linear construction of existing levels of automation. The purpose with the proposed LoC is to provide a tool that helps with identifying and supporting the appropriate level of collaboration between human and robot, which should not be confused with the idea of maximising collaboration. The underlying idea with the LoC tool is to provide guidance of how to distribute the division of labour and task-allocation in an efficient way; humans and robots have different properties and skills, resulting in various pros and cons, and those should determine the type of interaction, not the other way around. The aim of this paper is to provide a more theoretical foundation for such a LoC tool, which is based on relevant theories from cognitive science and other perspectives of human-technology interaction, strengthening the validity and scientific rigour of the envisioned LoC tool.
The rest of this paper is structured as follows. First, in the background section, the notions of robots in human-robot collaboration and humans in human-robot collaboration are clarified. Next, the shift from levels of automation to levels of collaboration are identified and presented. The arguments for, and the initial visualisation of, the proposed classification of levels of collaboration (LoC) for human robot-collaboration are then presented. Finally, the paper ends with a summary of the main contributions, future work, and some conclusions.
2. Background
Industry 4.0 is a term for an approach to create the next generation of manufacturing (Bundesministerium für Wirtschaft und Energie, Citation2016; Hermann et al., Citation2015) and advocates the use of sensors, ICT, and advanced automation throughout manufacturing facilities. The goal is to usher in the creation of ‘the factory of the future’ (Bundesministerium für Wirtschaft und Energie, Citation2016; Hermann et al., Citation2015). Many tools are required to support the creation of the factory of the future, and work is ongoing to identify and answer the myriad challenges facing engineers and designers (Bundesministerium für Wirtschaft und Energie, Citation2016; Hermann et al., Citation2015). Today’s industrial robots are contained within safety cells, being kept away from human workers for safety reasons. They are installed in a fixed manner, and reconfiguration is both costly and time-consuming. Projects, such as the Horizon 2020 project Manuwork, exist to explore the feasibility of flexible automation that can be added or removed from a manufacturing line, with the automation further supporting workers in their assembly through cooperation and collaboration. The development from industrial robots that acted less autonomously to robots operating in the same physical and social spaces as humans puts higher demands on the quality of the interaction between the human and the robot. In the past, issues related to the autonomy of robots have focused on safe interaction with the material environment, but the growth in the area of collaborative robots that function as partners with workers in industry result in robots that to a larger extent need to consider the human actor perspective.
2.1. Robots in human-robot collaboration
The purpose of robotic technology is to make it possible for a person to conduct something that he or she could not do earlier, or facilitate a certain task. The boundaries for how robots can be constituted, and the settings in which they can act, are continually expanding. Hence, robots can vary along multiple dimensions, e.g. the types of task it is intended to support, its morphology, interaction roles, human-robot physical proximity, and autonomy level (Alenljung, Lindblom, Andreasson, & Ziemke, Citation2017; Thrun, Citation2004). Moreover, the role of humans in relation to robots can vary; the human can be a supervisor, operator, mechanic, teammate, bystander, mentor, or information consumer (Goodrich & Schultz, Citation2007). The interaction can be indirect – which means that the user operates the robot by commanding it – or direct when the communication is bi-directional between the user and robot and the robot can act on its own (Thrun, Citation2004).
It should be emphasised that, the concept of ‘robot’ is constantly changing (Alenljung et al., Citation2017; Dautenhahn, Citation2013). In this paper, the robot is denoted as a tool whose configuration of sensors, actuators and integrated control system provides a significant level of flexible, independent and autonomous action (Montebelli, Messina Dahlberg, Billing, & Lindblom, Citation2017). Accordingly, a traditional computer is not viewed as a robot, although it is functionally flexible but it does not provide many action facilities, and neither is a traditional car although it provides action, yet it lacks satisfactory levels of flexibility and independence. Indeed, a robotic vacuum cleaner scores low in flexibility, but is providing some level of independent action (Montebelli et al., Citation2017; Powers, Citation2008). A robot has to some extent a physical instantiation, i.e. a physical form, being embodied. Accordingly, the physical body of the user is, often in a natural way, directly involved in the interaction with the technology in human-robot interaction (HRI) and HRC, instead of indirect ways performed with the help of commands, symbols, and icons on a computer screen via input tools such as keyboards or computer mice (although these activities also require some kind of embodied interaction and could be part of the robot’s interface) (Lindblom & Alenljung, Citation2015). As Hartson and Pyla (Citation2012) highlight, embodied interaction means bringing interaction into the humans’ real physical world to involve the human’s own physical being in the world, moving the interaction off the screen and into the real world. This means that the activities of the robot and human need to be coordinated ‘here and now’ and are taking place in shared physical as well as social space (Dautenhahn & Sanders, Citation2011).
The more mature and advanced technology that is being developed allows for more interactive robots, having the functional capabilities that are necessary but not sufficient for high-quality technology use. The varying degrees of humanlike morphology is well-suited to function in human environments, and thus, it can be more efficient, effective, and satisfying to interact with a robot than other kinds of interactive technology. One of the major goals of the field of HRI is to find the ‘natural’ means by which humans can use to interact and communicate with robots (see e.g.Benyon, Citation2019; Dautenhahn, Citation2013; Lindblom & Alenljung, Citation2015).
During the years, the role of the robot has been changing, from machine or tool in industrial robots to roles such as assistant, companion, and partner, to a teammate in HRI and HRC (Michalos et al., Citation2015; Thrun, Citation2004). The embodied nature of collaborative robots has several implications on the social interactions between humans and robots (Alenljung et al., Citation2017; Lindblom & Alenljung, Citation2015; Lindblom & Andreasson, Citation2016). However, from a cognitive science perspective, embodiment means much more than having a physical body that, roughly speaking, occupies some physical space. Sometimes embodied interaction is used as a kind of buzzword in HRI and HRC, but there are more aspects than moving and occupying some shared space. This issue is, however, beyond the topic of this paper (but see e.g. Lindblom, Citation2015; Lindblom & Alenljung, Citation2015; Vernon, Citation2014; Ziemke, Citation2016 for some detailed discussions of embodiment in robots from an embodied cognitive science perspective).
Although autonomous action is crucial for many types of robots, autonomy remains a problematic concept, receiving dramatically different interpretations in different communities (Lodwich, Citation2016; Maturana & Varela, Citation1987; Vernon, Citation2014). For example, in industrial robotic automation, high autonomy implies that the human operator can specify the robot’s behaviour. In contrast, following the notion of autonomy present in biology, cognitive science, and to some extent also in HRI, an artificial agent is autonomous if its behaviour cannot be fully controlled by an operator (Montebelli et al., Citation2017). Furthermore, Mindell (Citation2015) addresses three myths of today’s robots; 1) linear progress, 2) replacement, and 3) full autonomy. The myth of linear progress involves full autonomy where the human is no longer in the loop as being the ultimate goal, but is dismissed by Mindell (Citation2015) as being both unrealistic and based on policy, not on natural evolution of technology. The myth of replacement involves machines replacing humans at most tasks, taking over one task at a time. Mindell (Citation2015) likewise dismisses this as being inaccurate, as experience with mechanical substation suggests that new technologies solve new tasks and do not fully replace what has gone before. An example of this is autonomous aircraft, which are useful for dangerous missions but are limited in their response to conditions as well as their decision-making ability. Mindell (Citation2015) argues that full autonomy is a myth since the robot or other kinds of autonomous technology always is wrapped by human control. It is the human designer’s assumptions, plans and intentions that are built into the robot, which means that every person operating or interacting with the robot actually is cooperating with the programmer who still is present in the robot. This means that how a robot acts, although not only predicted in advance, it still acts within the constraints imposed by its designer. As phrased by Mindell (Citation2015, p. 10): ‘How a system [robot] is designed, by whom, and for what purpose shapes its abilities and its relationships with the people who use it’. Using the less strict term for various machines has been argued to lead to a vicious cycle of reduced concept richness, and reduced human authority and autonomy (Stensson & Jansson, Citation2014).
2.2. Humans in human-robot collaboration
Although robots that interact exactly like humans do are not likely to be developed in the foreseeable future, it is still necessary to examine how humans interact and collaborate so that HRC can take these factors into account. Examining human social interaction also requires a basic understanding of human cognition, as this gives valuable insights into the limitations and strengths of humans when interacting with either other humans or with robots.
2.2.1. Human social interaction and cognition
There is a lot of literature focusing on this area of research in several research communities, including cognitive psychology, communication, philosophy of mind, and cognitive science. Most generally, and without reviewing the large amount of literature on human social interaction and cognition, there are many proposals for organizing the various levels and kinds of social interaction but the differences lie principally in terminology and the sizes and numbers of parts, and not in where the major ‘cuts’ are made (see, e.g. Lindblom, Citation2015 for more details). Two main categorization of human social interactions are dyadic and triadic interaction. Various kinds of dyadic interaction exist, which could be characterised the mutual and direct sharing of behaviour and emotions, usually through facial expression, vocalization (not involving symbolic language), gaze, and turn-taking. During these face-to-face interactions, the interactants attend and attune to each others’ movements as well as emotional and facial expressions/signals, creating modes of mutual immediacy. A central feature of dyadic interactions is its turn-taking structure, in which emotional displays seem to be the glue that holds the interaction together, emphasizing that this kind of interaction is merely a form of ‘attentional-sharing’ and not ‘intentional-sharing’ (i.e. being about something or a shared goal) (Lindblom, Citation2015).
Triadic interaction is characterised by various kinds of shared interaction, entailing joint attention to objects or states of shared social referencing. Beyond turn-taking, gaze-following is an essential prerequisite for triadic interaction, which is the rapid shift between looking at the eyes of another person, following their gaze, and focusing the look at the same distal object or person. As a result, a referential triangle of ‘shared’ or ‘joint’ attention, between a person, another person and the object or event to which they focus their attention emerges. Typical joint activity behaviours are the giving and taking of objects, as well as conventional pointing and naming games. These abilities are consequently commonly termed joint (or shared) attention, which involves a whole complex range of social skills and interactions, such as gaze-following, joint engagement, social referencing, and imitative learning. In triadic engagements, the interactions are mostly individual activities in the form of mutual responsiveness to sharing goals and perceptions of some external object in a triadic fashion. Different kinds of joint attention are building blocks of more sophisticated and higher-order coordination and collaboration towards a shared goal in a joint action. Joint action can be described as a form of triadic interaction where two or more persons coordinate their actions in space and time in order to make a change in the environment, e.g., together moving a table. In so doing, a goal-directed joint action could be necessary, which means that rather than imitating the other person’s actions one must sometimes perform complementary actions to reach the common goal, i.e. tilting the table in order to pass through a narrow door opening (Lindblom, Citation2015).
It should be pointed out that cooperation and collaboration are different kinds of triadic interactions that often are used interchangeably in HRC, but there are conceptual differences between them. Cooperation, on the one hand, is described as a sequence of actions towards a shared goal, that each person is doing independently via subtasks towards the shared goal. This means that the actions are rather independent of each other (compare students that write an assignment by splitting up the writing process in individual parts, which then is put together into one shared document). Collaboration, on the other hand, is described as a sequence of shared actions towards a shared goal (students that write the assignments by adapting to each other, not only doing one’s own part, but for students to actively engage in the task of one’s peers, from the beginning to the end). The ability of social learning and variants of imitation adds a unique aspect to social interactions among humans (Lindblom, Citation2015). Without reviewing the vast social cognition literature in detail, one could say that what all these variants of learning and imitation have in common is that the differences concern how aware or conscious the learner is of its particular ‘imitating’ act. This can range from no awareness at all to being conscious of others as intentional beings, from whom they imitate a particular set of activities to reach a certain goal or end result (Lindblom, Citation2015).
When humans interact with each other, all of these aspects unfold relatively fluently and effortlessly, and for the most part it happens subconsciously, i.e. each of the human interactants has some level of understanding of the other human’s mental and emotional states (Dennett, Citation2009). The humans are thus able to recognise each others’ actions and intentions during various forms of triadic interaction. This ability is often referred to as mindreading. It should be emphasised that there is a great challenge to achieve a similar mutual and fluent dyadic and triadic interaction between humans and collaborative robots, because of the obvious differences between the underlying biological versus technological mechanisms (Lindblom, Citation2015; Ziemke, Citation2016). Therefore, the interaction between humans and robots needs to develop to a degree that is sufficient to enable similarly smooth interactions as between humans. A central part in order to succeed in this matter is to make it possible for humans to easily perceive, understand, and predict the intentions and actions of robots (Kiesler & Goodrich, Citation2018).
2.2.2. Role and relevance of human social interaction and cognition in HRC
It should be acknowledged that there is an ongoing debate whether non-biological robots could possess intrinsic intentionality and mindreading abilities (e.g., Dennett, Citation2009; Lindblom, Citation2015; Lindblom & Ziemke, Citation2003; Ziemke, Citation2016). However, it should be mentioned that the line between a human action and a robot action sometimes are a bit blurred. Some recent and ongoing research in (social) neuroscience has observed that when a biological agent (human or ape) carries out an action or observes the same action there is a correspondence in the so-called mirror (neuron) system, which highlights the importance of embodiment and morphological similarities in social interactions (e.g. Lindblom, Citation2015; Rizzolatti & Craighero, Citation2004; Ziemke, Citation2016). Some prior work on action and action recognition has focused on how some social robots could be used as an ‘interactive probe’ to investigate and assess the embodiment mechanisms underlying human–human interaction (e.g., Sandini & Sciutti, Citation2018; Sciutti, Ansuini, Becchio, & Sandini, Citation2015; Sciutti & Sandini, Citation2017; Vignolo et al., Citation2017). Furthermore, related work has been conducted to investigate whether a similar understanding also could occur beyond human–human interaction to encompass human-robot interaction, i.e. whether robots could be perceived as goal-oriented agents by humans. The obtained experimental results indicate a similar implicit processing of humans’ and robots’ actions. The authors suggested to use anticipatory gaze behaviour as a tool for evaluating human-robot interaction (Sciutti et al., Citation2013). This indicates that humans might be able to more or less easily grasp the actions and intentions of very human-like robots, but not necessarily the behaviour of, for example, autonomous lawn mowers or automated vehicles.
Dennett (Citation2009), among others, points out that humans often tend to use several mentalistic terms to interpret, predict, and explain the behaviour of other humans, animals, and sometimes even technical artefacts, although there are a lot of questions whether in particular robots could be said to truly have a mind. According to Dennett (Citation2009) the intentional systems theory offers some tentative answers to these above questions, by analysing the preconditions and the practice of making attributions when humans adopt an intentional stance towards an entity (human, animal, artefact). The intentional stance is the human strategy to interpret the behaviour of an entity by treating it as if it has a rational mind or intrinsic intentionality which, in turn, controls its selection of ‘actions’ depending on its ‘beliefs’, ‘intentions’ or ‘motives’. The central issue here is to study their role in practical reasoning and the prediction of the behaviour of this kind of reasoning (for further details, see Dennett, Citation2009). In order to develop a level of collaboration between humans and robots in HRC, we adopt the intentional stance in this paper.
3. Shift from automation to collaboration in HRC
Automation and collaboration fundamentally differ in that automation actively seeks to remove the human from the task being performed, whereas collaboration in HRC aims to maximise the capabilities of both human and robot through collaboration. An example of this can be seen in how self-driving cars are being approached in terms of how the system’s capabilities are defined. The goal state in self-driving cars is to eliminate the need for a driver, while the intermediate states suggest the need for a driver as being the result of current technology lacking the capability to function in a fully autonomous fashion (SAE, Citation2016). This can be contrasted with HRC where the goal is that the human and the robot collaborate, each agent contributing strengths as well as making up for each other’s weaknesses, as opposed to negating the need for one agent or the other (Michalos et al., Citation2015).
3.1. Collaboration in human-robot collaboration
Cooperation between humans and robots has been discussed from many perspectives, and HRC can thus mean many things. To address this, it is necessary to keep in mind the intended context, and specify three things; which humans are involved, what kind of robots, and what kind of collaboration. Within the manufacturing domain and Industry 4.0, the relevant humans to consider are the people working alongside the machines, however, Industry 4.0 points out that other staff on the assembly line can approach and work with the robots. As for the robots, it is more relevant to define them in terms of how humans perceive them than in terms of their actual capabilities (Vollmer, Wrede, Rohlfing, & Cangelosi, Citation2013). The actual capabilities can be derived from the particulars of the task, such as requirements in dexterity or strength, but safety issues are also important and common reasons for the specific properties of the used robots (Michalos et al., Citation2015). Collaboration also has many meanings and interpretations. From a cognitive systems perspective, as addressed in sub-section 2.2.1, collaboration has a more specific meaning than cooperation, which is more of an umbrella term for interacting agents. To collaborate in this sense is to partake in joint cooperative action or, in other words, shared cooperative activity (Vernon, Citation2014). For this to be possible in an intrinsic way, the involved agents need to have joint action, shared intentions, shared goals, and joint attention, each of which is a complicated phenomenon to handle in their own right. Joint action, for example, demands mutual responsiveness, commitment to the joint activity and commitment to mutual support (Bratman, Citation1992). When it comes to robots in manufacturing it might thus often suffice with robots that can provide instrumental helping (Vernon, Citation2014, p. 206) to the human, that is, to without any reward offered to help the humans perform something they cannot do by themselves.
The International Organization for Standardization (ISO) has defined collaborative operation as a ‘state in which a purposely designed robot system and an operator work within a collaborative workspace’, which in turn is a ‘space within the operating space where the robot system (including the workpiece) and a human can perform tasks concurrently during production operation’ (Technical committee: ISO/TC 299 Robotics, Citation2016). These definitions are specifically made for HRC in the industry, and from this the definition of collaboration can be inferred to be a kind of work performed simultaneously and co-located by a robot and an operator during production. An important phrase to highlight is ‘purposely designed robot system’; reminding that design decisions need to be informed. To, for example, talk about such systems in terms of ‘teams’ can be tempting, as much research has shown how powerful such constellations can be, however, to be a teammate comes with high demands and if they cannot be met, the foundation for the cooperation can break down (Groom & Nass, Citation2007). This is thus another example showing the necessity for the human’s attitude towards the robot to be taken into consideration. One specific aspect that can make or break interactions is trust, which is central also for interaction with automated intelligent systems (Lee & See, Citation2004).
A simple definition of trust consistent with the requirements of human-robot collaboration is that trust is the attitude that an agent will help achieve an individual’s goals in a situation characterised by uncertainty and vulnerability (Lee & See, Citation2004, p. 51). When it comes HRC then Maurtua, Ibarguren, Kildal, Susperregi, and Sierra (Citation2017) point to trust in automation as being essential for worker acceptance of collaborative robots, which is in line with research on other forms of automation, such as decision support systems (Helldin, Citation2012). Importantly, trust is not an intention or a behaviour, which is a common mischaracterisation of trust that has the potential to confuse the effect of trust with the effects of other factors that can influence behaviour, such as workload, situation awareness, and self-confidence of the operator (Lee & Moray, Citation1994). In cases of uncertainty and vulnerability then trust can be partly rational, but sometimes trust is largely irrational, especially when it comes to non-dangerous situations (Lee & See, Citation2004). This irrational aspect of trust needs to be taken into consideration when it comes to HRC in manufacturing, as the robots can be perceived as dangerous although they are designed in a way as to be safe to work with. This does mean that during deployment of HRC systems it is important to take in feedback from workers, and to take seriously ‘soft’ issues such as irrational fear, uncertainty, and feelings of vulnerability, as they are likely to have a large impact on all aspects of work, including worker satisfaction and productivity.
Trust must be built, and requires learning that the system’s indications of its intentions actually match what then happens. As a practical example of what can be done to support trust in collaborative robots, Maurtua et al. (Citation2017) suggest the use of status lights, sounds, and changes in movement speed to indicate intentions, as well as to indicate that the built-in safety mechanisms are active. Existing research on usability suggests that the design and execution of any such indications is critical to success, and that this needs to take into account the full social and physical context surrounding the whole system, including robot and worker, as well as other workers, machines, lighting, sound, etc. (Benyon, Citation2019; Cooper et al. Citation2014; Hartson & Pyla, Citation2012). Previous research in HRI and HRC reveals that safety is a necessary but not sufficient condition for avoiding incidents between humans and robots (Frohm et al., Citation2008; Kalpakjian et al., Citation2014). It has further been acknowledged that acceptance of and trust towards the robot companion also is needed for a credible and reliable HRC (Frohm et al., Citation2008; Krüger et al., Citation2017; Michalos et al., Citation2015; Shi, Jimmerson, Pearson, & Menassa, Citation2012; SAE, Citation2016; Shi et al., Citation2012). De Graaf and Allouch (Citation2013), among others, have shown that users’ subjective experience of the interaction quality with a humanoid robot have the same impact on the robot acceptance and trust as more performance-related aspects. A human-centred evaluation investigated to what extent operators experience trust when collaborating with a robot on a common workspace but with separate tasks in manual assembly. The findings demonstrated that the operators’ experienced limited trust while cooperating with the robot. It was only five out of 12 predefined goals that passed the target levels. The two major-identified reasons for the limited confidence of trust are: communication problems during the collaboration, which then resulted in participants’ uncertainty of their own ability to collaborate with the robot. The communication problems were strongly linked to the design of robot’s interface, which mixed several kinds of interaction modes (Nordqvist & Lindblom, Citation2018).
Worth noting is that trust should not be maximised. When overtrust occurs, where the operator’s trust in the system exceeds its capabilities, the operator is inclined to delegate inappropriate tasks for the robot. On the other hand, if the operator does not trust the robot enough the robot will not be used to its fullest potential. Another complicating factor is that trust is a dynamic attitude that can change based on how the system performs, is hard to gain, easy to lose, and even harder to rebuild (Atoyan & Shahbazian, Citation2009). In the domain of automated vehicle systems – where humans interact with highly automated machines for long periods of time – several factors have been identified regarding trust-management, and broken down into different distinct events where they are relevant (Ekman, Johansson, & Sochor, Citation2018). Factors of relevance are, for example, what training the operator has and what feedback the machine provides, and among the events are first encounter, hand-over situations, and incidents. The different factors can thus be used to tweak the operator’s trust, and make sure that it remains at an appropriate level. Although it is framed in a traffic context, much of the analysis can be generalised for other kinds of human-machine interaction. Moreover, appropriate levels of trust can vary based on the intended type of cooperation or collaboration. This effectively means that working in proximity with a robot requires a base level of trust, while basic cooperation requires more trust and full collaboration involving fully joint action makes much different requirements as to the trust that must be built and maintained. Each of these kinds of cooperation/collaboration requires all the trust from the simpler levels, in a way similar to how Maslow’s hierarchy of needs (Maslow, Citation1943) works. This is visualised in .
Figure 1. Eachmore involved type of cooperation/collaboration adds to what is required of the robot, the human, and the interaction between the two.
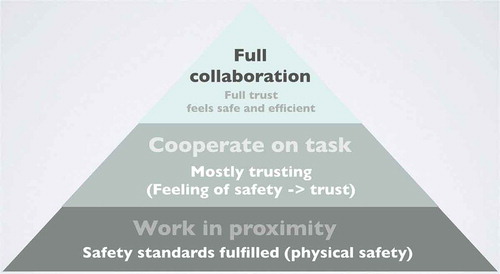
Savioja, Liinasuo and Koskinen (Citation2014) stress that the common practice in safety-critical domains is to focus on performance-related issues, which are highly influenced by human factors and ergonomics (HF&E). Because of the prevailing orientation towards HF&E in manufacturing, HRC research runs the risk of not considering the modern understandings of human cognition and technology-mediated activity, as embodiment (Lindblom, Citation2015) and activity theory (Kuutti, Citation1996), in which humans are considered as meaning-making actors (not factors) in a socio-cultural and material context.
3.2. From levels of automation to levels of collaboration
Supporting joint action requires an understanding of aspects of collaboration and cooperation. This has led to domain-specific definitions of various aspects of collaboration/cooperation, in some cases in the form of levels of automation (e.g. SAE, Citation2016; Sheridan & Verplank, Citation1978). Krüger et al. (Citation2017) examine how the task responsibility is distributed through different levels of human-machine interaction, visually illustrating how the changes in the distribution of task responsibility does not simply involve handing off a task between the human and the machine, but rather that there is a degree of mixing of the task responsibility, with possible lack of clarity as to who is responsible for a particular operation within the shared activity (see ).
Figure 2. Distribution of task responsibilities for different levels of human-machine interaction. (Adapted from Krüger et al., Citation2017).
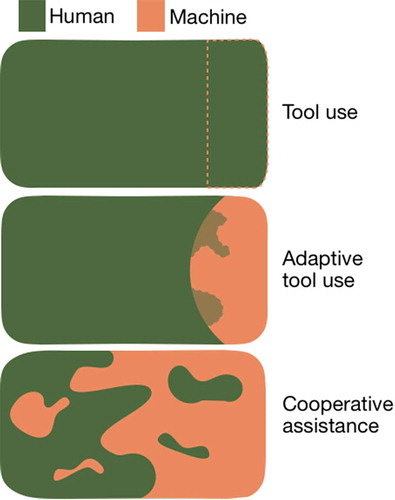
The SAE levels of automated driving (SAE, Citation2016) are a well-known example of domain-specific levels of automation, and are interesting as these levels range from complete human control of a vehicle, to fully autonomous operation in all conditions. The SAE levels can be seen in . An interesting attribute of these levels of automation is that they are portrayed as a single dimension, i.e. how much of the work is performed by each of the two envisioned partners in the activity (the human driver and the vehicle).
Table 1. SAE automation levels (adapted from the Department of Transportation, 2017).
The levels of automation proposed by Sheridan and Verplank (Citation1978) are similar in that they go from the activity being completely controlled by the human worker to being completely performed by the automation, with the automation determining what information needs to be communicated. What these levels of automation have in common is a presented one-dimensionality, they do not explicitly support viewing collaboration, examining trust in the automation, or inspecting other elements of joint action such as whether the task or space are shared or separate, with collaboration placing higher requirements on both partners than cooperation. One way of approaching some of these issues have been proposed by Lagerstedt, Riveiro, and Thill (2017), by mapping different kinds of robots with respect to their levels of automation and the degree of responsibility they are intended to handle in interactions. Trust has, as mentioned above, been shown to be a critical factor in working with collaborative robots, as is having an understanding of both the task to be performed and the workspace in which the task is to be performed. From this it becomes clear that a way of understanding levels of automation specific to collaboration that can assist decision-makers or designers of manufacturing lines in considering relevant factors would be useful, and this is what will now be shown.
3.3. Approaches for describing and visualising collaboration
Levels of collaboration (LoC) for industrial robots have been explored before, e.g. by Shi et al. (Citation2012) who looked at low, medium, and high LoC in both a current state of the industry and in a future state. Shi et al. (Citation2012) mostly focus on the technological aspects of collaboration, i.e. the limitations of then current automation, explaining that in a high LoC in the future state the robot is active, and in automatic mode. This is taken as a given now; collaboration with an inactive robot is not particularly useful. Shi et al. (Citation2012) also mention that the goal of collaborative robots in manufacturing is for a human worker and robot to perform tasks together, and that this is challenging. All the collaboration explored here happens at what Shi et al. (Citation2012) refer to as a High level of human-robot collaboration.
Each of the levels of automation that have been introduced shows each of the collaborators (the human and the automation) performing the task by themselves at the extremes of the scale, with the middle of the scale requiring some work from both partners. That middle section represents a problem for the automation, as these levels tend to denote an area wherein the automation is not always capable of completing the task, even though full autonomy is the state for which these systems strive. An example of this is seen in the SAE levels of automation for self-driving vehicles, where the description for the middle levels explain that the automation may need to disengage and the human may need to take over in certain circumstances (SAE, Citation2016).
Flemisch, Kelsch, Löper, Schieben, and Schindler (Citation2008) view this way of showing levels of automation along a scale from fully human controlled to fully automated as limited, and instead they propose a spectrum of automation that takes into account multiple factors. Although that spectrum includes more factors than most, it still uses one-dimensional visualisations to highlight certain aspects (Flemisch et al., Citation2008). This is useful for practitioners, as simpler visualisations can be designed to focus on one aspect at a time. Moreover, Flemisch et al. (Citation2008) use a design metaphor called the H-metaphor in their model, which offers a view on highly automated vehicles that shares more in common with how the driver and horse of a horse and carriage would interact than what is customary for machines (see ). This kind of analogy for safety and trust issues provides some benefits, particularly for providing a new way of thinking about collaboration with industrial robots in order to establish a relevant safety culture among the operators of HRC. Horses are rather big and strong, and being a social prey species, and the human handler always needs to consider some relevant safety issues depending of the nature on horse behaviour (Mills & McDonnell, Citation2005; Mills & Nankervis, Citation2013). Horses could, from a HRC perspective, be described as autonomous animals with their own intelligence, having a large amount of power that are used in various situations, both with other horses and humans. Although horses have an expected behavioural repertoire, they are not fully predictable. Similarly, autonomous robots used in HRC, with artificial intelligence (AI) and a large amount of power, are handling various components, for various products. These robots have an expected behavioural repertoire, but are not fully predictable. The analogy we want to address if whether, or to what extent, line managers and operators and related staff involved in HRC could apply some of the common sense and safety practices used in human-horse interaction when cultivating a safety culture and making the risk assessment of workplaces with collaborative robots?
Figure 3. Automation and role spectrum as defined by the H-metaphor. This illustrates that automation having the capability to collaborate can be thought of as a strong animal that should be guided by a ‘driver’ who may provide an overall motive to the activity, while the automated system may provide valuable input in the form of lower goals and may be providing fine elements of control. (Adapted from Flemisch et al., Citation2008, p. 13).
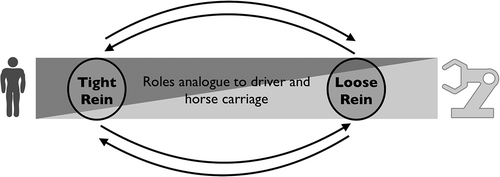
Furthermore, Phillips, Schaefer, Billings, Jentsch, and Hancock (Citation2016) suggest that human-animal teams, preferably human-dog teams, may serve as an analogue for future human-robot teams by influencing the design of these teams as well as enabling trust. They focus mostly on creating future human-robot teams in the military and industrial domains where the human-dog teams serve a convenient analogue for the nearby design of HRC/HRI. Human-animal teams, e.g. officer/patrol dog relationships and animal-assisted therapy, are able of accomplishing a wide variety of physical, emotional, and cognitive tasks by leveraging the distinctive capabilities of each team member (human or animal) without implying the necessity for a full replica/simulation of human social interaction and communication for an effective outcome. Their key points are to provide insights into the design of future HRI/HRC where (1) the human-animal relationships can often directly promote the human partner physically, emotionally, and cognitively. (2) The human-animal relationships range in extent of complexity and interdependence regarding how they interact with each other to accomplish the tasks. Modelling the division of labour in these teams can represent and provide the progression in the development of better HRI/HRC. (3) The development of trust is of utmost importance in HRI/HRC, and the means by which trust is cultivated in analogous human-animal teams serves as a prototype for developing trusting relationships in human-robot teams/collaborations (Phillips et al., Citation2016). It should be noted, however, that although some people with limited experience of interacting with dogs or horses are able to handle and interpret their socially embodied actions and personalities, the role of learning to correctly interpret and handle animals, and in the case of robots, should not be underestimated. While this human-animal role model approach may not solve many aspects of HRC, especially on how to categorise collaboration and how to support cooperation and collaboration of various types, it may be seen to offer an innovative view when interacting in highly collaborative systems.
Michalos et al. (Citation2015) particularly examine aspects of human-robot collaboration, but approach it from a different angle, with more focus on the kinds of cooperation/collaboration they can envision. This involves classifying a shared cooperative activity into whether the task is shared or separately performed by the robot and the human, and whether the space in which the task is performed is shared by the human and the robot or whether they each have their own space. This can be seen in , with a matrix of the possibilities shown in .
Table 2. A matrix that breaks down the characteristics of the taxonomy of Human-Robot collaborative tasks and workspaces shown in .
Figure 4. Taxonomy of human-robot collaborative tasks and workspaces (Adapted from Michalos et al., Citation2015, p. 249).
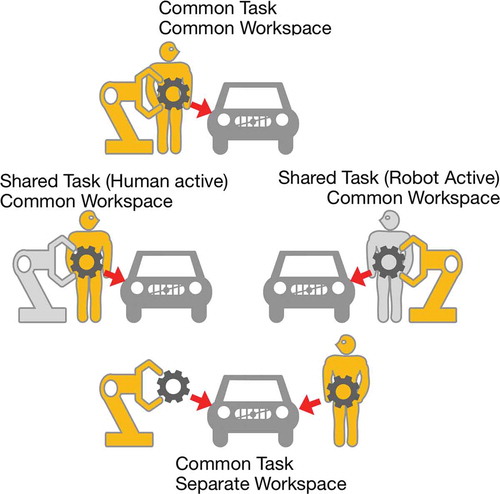
Michalos et al. (Citation2015) point out that in many cases these new human-robot collaboration systems should be able to share the workspace with a human coworker and that this includes having physical contact. These HRC systems are being categorised as either ‘workspace sharing’ or ‘workspace and time sharing’, depending on their functionality (Michalos et al., Citation2015). It should be pointed out, however, that in both cases, the human operators and robots are being able to perform either single, cooperative, or collaborative tasks. This way of working has implications for the human worker, giving the human operator multiple roles, including to act as a supervisor, operator, teammate, mechanic/programmer, but also as a bystander (Michalos et al., Citation2015). Michalos et al. (Citation2015) also point out that HRI/HRC systems could be further categorised, depending on the level of interaction between the human and the robot. As depicted in (adapted from Michalos et al., Citation2015), the robot and the human operator could have a common task and workspace, a shared task and workspace, or a common task and a separate workspace. In the case when the human operator and the robot collaborate in common tasks and workspace, the relation between them could potentially be described as a joint action task (Technical committee: ISO/TC 299 Robotics, Citation2016).
One detail worth pointing out is that Michalos et al. (Citation2015) classify a shared common task in a shared space by which one of the partners (the robot or the human) is active, but not simultaneously This can be seen in the centre row of , where the left part shows the human performing his/her task, and the right side shows the robot active performing its task. In this case, the task is shared, and the space is shared, but the task requires non-concurrent action from the partners (i.e. they take turns). Thus, they do not include any strict joint action as described in the human social interaction and cognition literature.
4. Towards a classification of collaboration levels for human-robot cooperation
When aiming for collaboration the argument needs to be slightly different to when the goal is merely to view how much automation is involved. There is still a need for showing how much work each partner needs to contribute, but also a need for examining the depth and complexity of the collaboration between the partners. This is why instead of a scale from one extreme (human only) to another (automation only) we propose the use of a description and a visualisation where the level of collaboration (LoC) becomes the main (horizontal) axis, with ‘human only’ and ‘automation only’ being placed on one side of the scale, gradually intertwining towards the other side of the scale. This can be seen in the lower visualisation in , where the levels go from describing a task fully performed by either a human or robot, goes to cooperation where both the human and robot may contribute to the task in some way, and finally full collaboration where the task responsibility is fully shared by both partners (see Krüger et al., Citation2017).
Figure 5. Top: the common view of LoA, going from full human control to full automation, with an area in the middle where the automation is not sufficient to complete the task (e.g., SAE, Citation2016). Bottom: Levels of Collaboration (LoC) as a parallel process that builds from a task being completed either by only a human or only by automation at the left, towards full collaboration at the right.
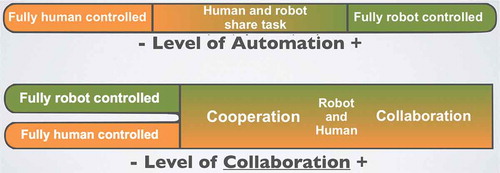
How the scale is visualised affects what is prioritised by the user, which is why the LoC needs to be in focus. This is the primary difference between the LoC being developed here and the various different LoA that have been introduced, the focus is on collaboration, not on degree/level of automation and the highest level should involve full collaboration where the task space and task responsibility are shared equally between human and automation.
The requirements of each of the collaboration partners can then be highlighted, with the distribution of task responsibility being interpreted as increasing and mixing along the scale. The distribution of task responsibility was visualised by Krüger et al. (Citation2017) illustrating how the task responsibility of the human and the robot mix with increased collaboration. This serves as a reminder that a clear task separation or responsibility is not always possible (or even desirable) as in the case of one partner holding an object and the other partner guiding and fastening the object to other objects. Indeed, as well as the simple LoA previously described going from fully manual to fully automatic, LoA have also been described in terms of a parallel control continuum (Billings, Citation2018). This is useful when the focus does not lie on how much automation there is, but rather on the collaboration between manual work and automation.
Viewing the LoA as a parallel control continuum makes it simple to view the distribution of work as not going from fully human control to fully automatic control with some sort of problematic ‘in-between’ state, but rather that both the fully human control and the fully automatic control build towards a ‘fully collaborative’ state, see . This difference between the LoC visualisation and the LoA lifts the difference into focus; when it comes to HRC the goal can be to have the automation assume full control and the human to take over when needed, but when it comes to collaborating on a task, such as in a factory, then the goal is to use the best aspects of the human and the robot together, not to have automation take over the task.
Figure 6. Visualisation of collaboration level. Lower image is adapted and modified from Krüger et al. (Citation2017) to fit with the main visualisation, showing collaboration as uniting the effort of the human and the robot.
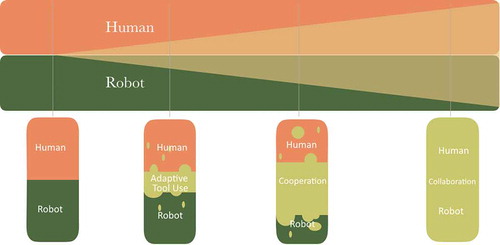
Importantly, collaboration makes requirements of the automation hardware that full automation does not, and makes psychological requirements of the human worker that working alone does not. The automation must fulfill legal requirements for collaborative robots, that is simply the most basic requirement to even consider collaborative automation, while the human worker must have some level of trust in the automation to even consider starting working with it, and when full collaboration is achieved then these requirements become more complex. This is somewhat similar to the classic Maslow’s hierarchy of needs, where basic needs must be met before more complex needs can even be considered (Maslow, Citation1943). An illustration of how Maslow’s hierarchy of needs can be adapted to a sort of ‘hierarchy of needs for HRC can be seen in .
shows how the parallel concept of LoC can be visualised, combining the concept shown in with elements from the figures developed by Krüger et al. (Citation2017). In here, those visualisations (Krüger et al., Citation2017) are imagined as ‘slices’ (or into the third dimension) of the parallel concept, and show how cooperation and collaboration gradually start between the human and the robot, and then gradually take over to become a full collaboration between human and robot. The visualisations made by Krüger et al. (Citation2017) used two colours, one for each partner in the collaboration, and showed how those gradually interleave. We add a third colour (see ), which denotes the collaboration itself, suggesting that the collaboration itself can be viewed as an emergent property of the sharing of task responsibility. Viewing collaboration in this way also highlights the dyadic and triadic aspects of cooperation/collaboration, with higher cooperation/collaboration requiring a shift from dyadic to triadic forms of interaction and making higher requirements of both agents. The first two colours highlight the requirements and responsibilities of each agent (human and robot) while the third colour is useful to highlight the requirements for supporting the required level of collaboration. The horizontal markings in the ‘slices’ denote which agent is begin referred to, while the vertical markings denote examples of typical cooperation types.
The slice at the far left of visualises tasks where one or the other of the agents have full responsibility of the task, with this either referring to a task where only one of the agents is involved, or where a shared task is performed non-concurrently with each agent with full responsibility for the task at any given.
The second slice from the left highlights adaptive tool use where both agents take part in the task but the amount of cooperation or collaboration may be limited; this slice can have more or less control by each agent, but the important feature is the relatively small amount of cooperation/collaboration. An example of what this slice entails is a robot arm used as a lifting tool for heavy objects. The robot arm is not active, i.e. does not perform actions on its own, but does render the heavy object that should be lifted weightless. This allows the human agent to perform the task, leading the robot, but the robot arm follows rules as to where it can go so as to avoid collisions and ensure that the component is lifted to the correct spot. The human agent performs the motions, and ‘wiggles’ the component into place.
The third slice illustrates a fair level of cooperation. This involves both actors actively working on a task or activity and both actors are active in the task. This can involve a shared action where both actors work on the same part at the same time, but does not involve a fully joint activity where the actors need to share a common goal and be aware of each other’s intentions. A medium to a high degree of trust is required in the human actor to support this level of cooperation.
Fully joint activities can be illustrated by the fourth slice. Collaboration permeates the whole task, and although it is possible to map out which actor is the principal in each action or operation, it is as likely that the actions require the effort of both actors. Task responsibility shift fluidly between the actors as required, and both actors are ‘aware’ of each other’s intentions. A high degree of trust is required to work at this level.
Note that is a support tool for conceptually understanding the concepts surrounding HRC; the slices use the area to denote which agent has more responsibility during any given task as well as how much cooperation/collaboration the task involves. The slices are in effect an abstraction of the task responsibility.
In the future, it is possible that the slices can be coupled to an analysis tool to map out an activity so that the distribution of task responsibility can be better understood within the activity.
5. Contribution and conclusion
This short paper seeks to introduce a more practical way to think about levels of automation when it comes to HRC, focusing on collaboration rather than automation when, and only when appropriate, i.e. when the goal of the use of automation in that context is collaboration. The goal of the collaboration levels is to provide a classification into which legal, technical, and psychological requirements and limitations of both the human and the robot can be inserted. No claim is made that this is complete, but rather that the classification combines useful research in a way that supports future work on collaborative robotics in industry. Although we mainly base the work here on a foundation of cognitive science an important point is that work on HRC requires an interdisciplinary or transdisciplinary approach, as no one discipline will offer answers to all the questions surrounding HRC. Indeed, even creating a starting point such as levels of collaboration requires multiple competences, and an insight into domains such as manufacturing, robotics, human cognition, and HF&E.
Collaboration in itself is seldom a goal, but rather a means to an end, and HRC is in most cases no different. When it comes to HRC in manufacturing it is important not to lose sight of what the point of collaboration is; to support activities and tasks that make too high requirements of either human or robot when working alone. This can have many sources such as a task being physically demanding for a human, leading to repetitive strain injuries that negatively affects worker health (and may remove skilled workers from the workforce), or it may be that a robot can solve almost the whole task but fitting two parts together may need some ‘wiggling’ for which the robot lacks finesse. The task, or activity, is therefore the focus of collaboration, and cooperation or collaboration should be designed to complete the task. This means that the goal of HRC in manufacturing is not to achieve a maximum collaboration level, but rather that the whole system (robot, procedures, task, human) supports the appropriate level of collaboration to successfully complete that task. This requires understanding the requirements of the task/activity, as well as understanding the requirements and capabilities of both agents (human and robot). User-centred design is an example of an approach useful for this, and highlights the human-centred perspective (Benyon, Citation2019; Hartson & Pyla, Citation2012). Approaches for this may be taken from multiple disciplines, such as HF&E, UXD (user experience design), interaction design, as well as more theoretical approaches from HF&E and cognitive science. An example of the more theoretical approach is Lindblom and Wang (Citation2018) who present an envisioned evaluation framework of HRC, which focuses on safety, trust and operators’ experience when interacting closely with different collaborative robots.
In this paper, we have presented a way of visualising different situations where humans and robots work together. Contrary to many others, we have not framed it as a linear scale along which one agent is in control at each extreme. Instead, we have used the degree of involvement in a common task as the distinguishing feature, and in doing so emphasised the various kinds of cooperation. Under the right circumstances, collaboration could emerge among cooperating agents, and change the nature of the interaction. It is thus important to consider collaboration from a holistic perspective, where it is something fundamentally more than two individual agents working. The goal with this paper is to provide the theoretical tools to support future work on human-robot collaboration, as well as to introduce theories that are relevant but not yet common in technical domains such as manufacturing. Such a theoretical background is necessary to understand the nuances of interaction, human cognitive abilities, and of the whole collaborative system, as well as maintaining appropriate expectations when designing systems that incorporate HRC.
Future work requires clearly defining LoC to operationalise the functionally different cooperation stages as a next step, after which a tool, such as a checklist, would directly benefit practitioners such as assembly line designers in the industry. Such a tool should support the analysis of the task/activity, human requirements and limitations, and robot requirements and limitations in such a way as to support the design or evaluation of an assembly station incorporating HRC. Furthermore, discussions with industry experts have highlighted that being able to figure out what requirements will be made of a robot in such an assembly line would be useful for understanding what capabilities should be considered when purchasing robots for HRC applications. Without such a tool the risk is that any HRC application is seen as requiring all possible sensors and options, making HRC prohibitively expensive and effectively reducing the deployment of HRC even where it would otherwise be appropriate. A practical impact of having such a tool available is that effective use of HRC can support the long-term viability of humans in manufacturing, reducing negative health effects on human workers from performing tasks that can cause injuries. Conversations with industry specialists have suggested that removing humans from manufacturing is not considered viable even in the long-term, although human workers are expensive and time-consuming to train, and that it has become increasingly difficult to attract workers. This suggests that it will become imperative for manufacturing companies to explicitly show that they support their workers and demonstrate in what ways the workers are supported. This is important both to attract personnel and to keep their expensive assembly specialists healthy and contributing to the workforce as long as possible.
Acknowledgments
This work has been supported by funding from the European Union’s Horizon 2020 research and innovation programme and under grant agreement No723711 and the SYMBIO-TIC [637107] sponsored by the European Union. This work was also financially supported by the Knowledge Foundation, Stockholm, under SIDUS grant agreement no. 20140220 (AIR, Action and intention recognition in human interaction with autonomous systems).
Disclosure statement
No potential conflict of interest was reported by the author.
Additional information
Funding
References
- Alenljung, B., Lindblom, J., Andreasson, R., & Ziemke, T. (2017). User experience in social human-robot interaction. International Journal of Ambient Computing and Intelligence, 8(2), 13–32.
- Atoyan, H., & Shahbazian, E. (2009). Analyses of the concept of trust in information fusion and situation assessment. In: Shahbazian E., Rogova G., DeWeert M.J. (Eds.), Harbour protection through data fusion technologies (pp. 161–170). Dordrecht: Springer.
- Benyon, D. (2019). Designing user experience: A guide to HCI, UX and interaction design (4th ed.). Harlow, England: Pearson.
- Billings, C. E. (2018). Aviation automation: The search for a human-centered approach. Mahwah, NJ: CRC Press.
- Bratman, M. E. (1992). Shared cooperative activity. The Philosophical Review, 101(2), 327–341.
- Bundesministerium für Wirtschaft und Energie. (2016). Was Ist industrie4.0? Retrieved from http://www.plattform-i40.de/I40/Navigation/DE/Industrie40/WasIndustrie40/was-ist-industrie-40.html
- Cooper, A., Reimann, R., Cronin, D., & Noessel, C. (2014). About face: the essentials of interaction design. Indianapolis, IN: John Wiley & Sons.
- Dautenhahn, K., & Sanders, J., (Eds.). (2011). Introduction. In K. Dautenhahn & J. Sanders (Eds.), New frontiers in human-robot interaction (pp. 1–5). Amsterdam, Netherlands: John Benjamins.
- Dautenhahn, K. (2013). Human-robot interaction. In M. Soegaard & R. F. Dam (Eds.), The encyclopedia of human-computer interaction (2nd ed.). Aarhus: The Interaction Design Foundation. Retrieved from http://www.interaction-design.org/encyclopedia/human-robot_interaction.html
- De Graaf, M. M., & Allouch, S. B. (2013). Exploring influencing variables for the acceptance of social robots. Robotics and Autonomous Systems, 61(12), 1476–1486.
- Dennett, D. (2009). Intentional systems theory. In B. McLaughlin, A. Beckermann, & S. Walter (Eds.), The Oxford handbook of philosophy of mind (pp. 339–350). Oxford: Oxford University Press.
- Ekman, F., Johansson, M., & Sochor, J. (2018). Creating appropriate trust in automated vehicle systems: A framework for hmi design. IEEE Transactions on Human-Machine Systems, 48(1), 95–101.
- Flemisch, F., Kelsch, J., Löper, C., Schieben, A., & Schindler, J. (2008). Automation spectrum, inner/outer compatibility and other potentially useful human factors concepts for assistance and automation. In de Waard, Flemisch, Lorenz, Oberheid, and Brookhuis (Eds.), Human factors for assistance and automation (pp. 1–16). Maastricht, the Netherlands: Shaker Publishing.
- Frohm, J., Lindström, V., Winroth, M., & Stahre, J. (2008). Levels of automation in manufacturing. Ergonomia 30(3)(3).
- Goodrich, M. A., & Schultz, A. C. (2007). Human-robot interaction: A survey. Foundations and Trends in Human–Computer Interaction, 1(3), 203–275.
- Groom, V., & Nass, C. (2007). Can robots be teammates?: Benchmarks in human–Robot teams. Interaction Studies, 8(3), 483–500.
- Hartson, R., & Pyla, P. S. (2012). The UX book: Process and guidelines for ensuring a quality user experience. Amsterdam: Morgan Kaufmann.
- Helldin, T. (2012). Human-centred automation: with application to the fighter aircraft domain (Doctoral dissertation, Örebro universitet)
- Hermann, M., Pentek, T., & Otto, B. (2015). Design principles for industrie 4.0 scenarios: A literature review. Technische Universität Dortmund. Audi Stiftungslehrstuhl Supply Net Order Management.
- Kalpakjian, S., Schmid, S. R., & Sekar, K. S. (2014). Manufacturing engineering and technology. Upper Saddle River, NJ: Pearson.
- Kiesler, S., & Goodrich, M. A. (2018). The science of human-robot interaction. ACM Transaction of Human-Robot Interaction, 7(1), Article 9.
- Krüger, M., Weibel, C. B., & Wersing, H. (2017). From tools towards cooperative assistants. Paper presented at the 5th International Conference on Human Agent Interaction (pp. 287–294). New York: ACM.
- Kuutti, K. (1996). Activity theory as a potential framework for human-computer interaction research. In B. Nardi (Ed.), Context and consciousness: Activity theory and human-computer interaction (pp. 17–44). Cambridge, MA: The MIT Press.
- Lee, J. D., & Moray, N. (1994). Trust, self-confidence, and operators' adaptation to automation. International Journal of Human-computer Studies, 40(1), 153–184.
- Lee, J. D., & See, K. A. (2004). Trust in automation: Designing for appropriate reliance. Human Factors, 46(1), 50–80.
- Lindblom, J. (2015). Embodied social cognition. Berlin: Springer Verlag.
- Lindblom, J., & Alenljung, B. (2015). Socially embodied human-robot interaction: Addressing human emotions with theories of embodied cognition. In J. Vallverdú (Ed.), Synthesizing human emotion in intelligent systems and robotics (pp. 169–190). Hershey, PA: IGI Global.
- Lindblom, J., & Andreasson, R. (2016,. July 27-31) Current challenges for UX evaluation of human-robot interaction. Advances in Ergonomics of Manufacturing: Managing the Enterprise of the Future: Proceedings of the AHFE 2016 International Conference on Human Aspects of Advanced Manufacturing, FL, USA (267–277). Switzerland: Springer.
- Lindblom, J., & Wang, W. (2018). Towards an evaluation framework of safety, trust, and operator experience in different demonstrators of human-robot collaboration. In Thorvald, P. & Case, K. (Eds) Advances in manufacturing technology XXXII (pp. 145–150).
- Lindblom, J., & Ziemke, T. (2003). Social situatedness of natural and artificial intelligence: Vygotsky and beyond. Adaptive Behavior, 11(2), 79–96.
- Lodwich, A. (2016). Differences between industrial models of autonomy and systemic models of autonomy. arXiv.org:1605.07335.
- Maslow, A. H. (1943). A theory of human motivation. Psychological Review, 50(4), 370.
- Maturana, H. R., & Varela, F. J. (1987). The tree of knowledge: The biological roots of human understanding. Boston, MA: New Science Library/Shambhala Publications.
- Maurtua, I., Ibarguren, A., Kildal, J., Susperregi, L., & Sierra, B. (2017). Human–Robot collaboration in industrial applications: Safety, interaction and trust. International Journal of Advanced Robotic Systems, 14(4), 1–10.
- Michalos, G., Makris, S., Tsarouchi, P., Guasch, T., Kontovrakis, D., & Chryssolouris, G. (2015). Design considerations for safe human-robot collaborative workplaces. Procedia CIrP, 37, 248–253.
- Mills, D. S., & McDonnell, S. M. (Eds.). (2005). The domestic horse: The origins, development and management of its behaviour. Cambridge: Cambridge University Press.
- Mills, D. S., & Nankervis, K. J. (2013). Equine behaviour: Principles and practice. Oxford: Blackwell Science.
- Mindell, D. A. (2015). Our robots, ourselves: Robotics and the myths of autonomy. New York: Viking Adult.
- Montebelli, A., Messina Dahlberg, G., Billing, E., & Lindblom, J. (2017). Reframing HRI education: Reformulating HRI educational offers to promote diverse thinking and scientific progress. Journal of Human-Robot Interaction, 6(2), 3–26.
- Nordqvist, M., & Lindblom, J. (2018). Operators’ experience of trust in manual assembly with a collaborative robot. In M. Imai, T. Norman, E. Sklar, & T. Komatsu (Eds.), HAI ‘18 proceedings of the 6th international conference on human-agent interaction (pp. 341–343). New York, NY: ACM Digital Library.
- Phillips, E., Schaefer, K. E., Billings, D. R., Jentsch, F., & Hancock, P. A. (2016). Human-animal teams as an analogue for future human-robot teams: Influencing design and fostering trust. Journal of Human-Robot Interaction, 5(1), 100–125.
- Powers, A. (2008). What robotics can learn from HCI. Interactions, 15(2), 67–69.
- Rizzolatti, G., & Craighero, L. (2004). The mirror-neuron system. Annual Review of Neuroscience, 27, 169–192.
- SAE. (2016). Taxonomy and definitions for terms related to driving automation systems for on-road motor vehicles. Retrieved from https://saemobilus.sae.org/content/J3016_201806 .
- Sandini, G., & Sciutti, A. (2018). Humane robots—from robots with a humanoid body to robots with an anthropomorphic mind. ACM Transactions on Human-Robot Interaction (THRI), 7(1), 7.
- Savioja, P., Liinasuo, M., & Koskinen, H. (2014). User experience: does it matter in complex systems? Cognition, Technology & Work, 16(4), 429–449.
- Sciutti, A., Ansuini, C., Becchio, C., & Sandini, G. (2015). Investigating the ability to read others’ intentions using humanoid robots. Frontiers in Psychology, 6, 1362.
- Sciutti, A., Bisio, A., Nori, F., Metta, G., Fadiga, L., & Sandini, G. (2013). Robots can be perceived as goal-oriented agents. Interaction Studies, 14(3), 329–350.
- Sciutti, A., & Sandini, G. (2017). Interacting with robots to investigate the bases of social interaction. IEEE Transactions on Neural Systems and Rehabilitation Engineering, 25(12), 2295–2304.
- Sheridan, T. B., & Verplank, W. L. (1978). Human and computer control of undersea teleoperators. Cambridge: Massachusetts Inst of Tech Cambridge Man-Machine Systems Lab.
- Shi, J., Jimmerson, G., Pearson, T., & Menassa, R. (2012). Levels of human and robot collaboration for automotive manufacturing. Proceedings of the Workshop on Performance Metrics for Intelligent Systems (pp. 95–100). New York: ACM.
- Stensson, P., & Jansson, A. (2014). Autonomous technology–Sources of confusion: A model for explanation and prediction of conceptual shifts. Ergonomics, 57(3), 455–470.
- Technical committee: ISO/TC 299 Robotics. (2016). Robots and robotic devices – Collaborative robots. ISO 15066:2016(en) (p. 33). Geneva, Switzerland: International Organization for Standardization.
- Thrun, S. (2004). Toward a framework for human-robot interaction. Human-Computer Interaction, 19(1), 9–24.
- Vernon, D. (2014). Artificial cognitive systems: A primer. Cambrige: MIT Press.
- Vignolo, A., Noceti, N., Rea, F., Sciutti, A., Odone, F., & Sandini, G. (2017). Detecting biological motion for human–Robot interaction: A link between perception and action. Frontiers in Robotics and AI, 4, 14.
- Vollmer, A.-L., Wrede, B., Rohlfing, K. J., & Cangelosi, A. (2013). Do beliefs about a robot’s capabilities influence alignment to its actions? Presented at IEEE Third Joint International Conference on Development and Learning and Epigenetic Robotics (ICDL), Osaka (pp. 1–6).IEEE.
- Ziemke, T. (2016). The body of knowledge: On the role of the living body in grounding embodied cognition. Biosystems, 148, 4–11.