ABSTRACT
Today’s global supply chain (SC) is grappling with unprecedented challenges, which rendered conventional SC resilience (SCR) inadequate. In the wake of the Industry 4.0 (I4.0) age, investment in digital SCR (DSCR) relying on I4.0 technologies can potentially enhance organizations abilities to detect, avoid, respond to, and recover from disruptions promptly and efficiently. Literature most focuses on traditional SCR, while DSCR remains incipient. From this, this paper conducts a comprehensive literature review on the SCR, I4.0, and investment (INV) interplays to identify potential research gaps and avenues. It is revealed the integration of SCR-I4.0-INV as a complex and multifaceted process that requires holistic approaches. Some research gaps include the need for empirical studies on DSCR impacts, the role of organizational culture in supporting digital transformation, and investment and resilience trade-offs. This research provides insights for decision-makers and policymakers seeking to develop strategies for promoting resilient SCs in the digital transformation era.
1. Introduction
In the wake of interdependent global markets and amplified international trade, today’s businesses require additional ingredients than mere human and financial resources and their supporting eco-system. In the current augmented information age, within the so-called Industry 4.0 (I4.0) mandate, digital transformation encompasses the digitization or digitalization of the business in a manner that captures the current states of the systems by the installation and operation of pervasive sensing and communication capabilities. That in consonance with advanced analytics allows the utilization of near past inputs to predict and prescribe near to further future outcomes (Menezes et al., Citation2019). It provides the necessary scalability, traceability, and evolution into optimized and automated operations and controls.
Given the unprecedented rates of opportunities and threats, supply chain resilience (SCR) continues to play an increasing role in an organization’s survival and growth. SCR is believed to encompass critical roles that far exceed responding to an ad-hoc crisis, albeit it is about continuously matching and optimizing a series of interconnected supply chain and logistics processes to enhance the situational awareness of the present and future statuses of the networks (Al-Banna et al., Citation2022, Citation2023). Therefore, the organization’s abilities of early anticipation and adjustment to risks and disruptions are critical to its resilience and its two distinct stages of resistance and recovery as proposed in Melnyk et al. (Citation2014), where each one stems into two more sub-stages. Resistance into avoidance and containment, while recovery into stabilization and return, as shown in .
Figure 1. Tree of supply chain resilience, adapted from (Melnyk et al., Citation2014).
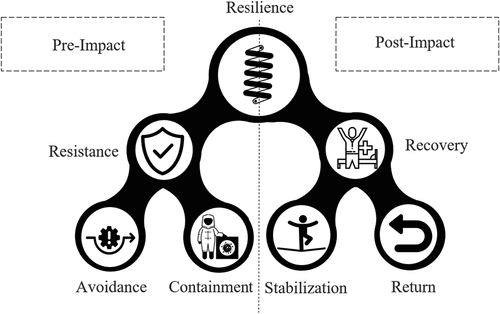
Inopportunely, some organizations appear to be myopic about the fact that building resilience starts simultaneously with laying the first bricks of the business and that it requires continuous innovation and evolution in parallel with the organization’s vision, strategic planning, and operations. In this paper, the subject of supply chain resilience shall be discussed in a different approach from previous research. The notion of digital supply chain resilience (DSCR) is represented by the optimum adoption and utilization of the relevant I4.0 technology enabler that yields optimum results for the organization. For example, blockchain is among the most innovative I4.0 enablers. Nevertheless, its utilization will be hindered in an environment where blockchain and cryptocurrencies are not regulated yet. In other words, the optimum I4.0 technology enabler identification needs to be carefully evaluated and selected based on a robust blueprint and a number of verifications criteria (AlBanna et al., Citation2022).
This paper aims to cover the interconnectedness between supply chain resilience, I4.0, and investment. The paper first addresses the generic definitions of SCR in conjunction with the increasing varieties of risks and uncertainties and their associated mitigation and control strategies. The review methodology is explored in section 2. Next, the literature review gaps and discussions are discussed in section 3, with a focus on SCR definitions, development, the role of I4.0 in SCR, investment in I4.0 and the cost of cybersecurity vs. cyberattacks. Finally, the conclusion is drawn in section 4.
2. Literature review methodology
This review paper surveys research articles published in the past 20 years, from 2002 to 2022, in the area of SCR with a focus on the interplay of I4.0 in SCR and possible investment opportunities therein. Literature retrieval is performed by performing keyword searches primarily in the Scopus and Web of Science (WOS) databases. In the next step, citation lists from the databases are compiled into a single repository, and duplicates are removed. Next, with the objective of creating a comprehensive repository of relevant papers, other relevant ad-hoc articles are considered and added. We adopt a structured funnel approach starting from a macro level (generic theme), diving deeper into the micro-level (focused theme), and then zooming on the intersections between SCR, I4.0, and INV.
The literature review structure, which comprises of multilevel keyword search based on a funnel approach in Scopus and WOS is detailed in illustrated in . In both Scopus and WOS, keyword search is performed in the TITLE-ABS-KEY (Title, Abstract, Keywords, and Keywords Plus). In Level 1, the search is performed by querying ‘supply chain’ AND ‘resili*’, where the logical operator ‘AND’ returns results having both the keywords in title, abstract or keywords. Another logical operator ‘OR’ is used in Level 2 and above, which finds articles containing any of the keywords separated by the operator. Furthermore, an asterisk (*) is used to perform a wild card search, which finds multiple characters. For example, ‘resil*’ finds resilience, resilient, and resiliency. Other wild cards such as question mark (?) and dollar sign ($), available in WOS, are not used.
Figure 2. Literature review process – funnel approach.
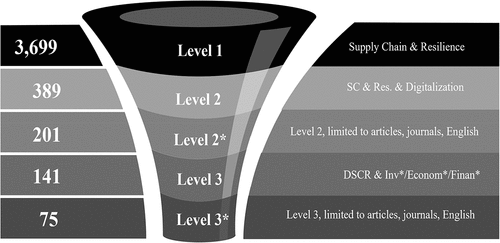
Generic keyword searches such as ‘Supply Chain’, ‘Resilience’, and ‘Investment’ are avoided because they generate a large number of irrelevant results. Cited references of shortlisted articles from the keyword search are also analyzed. In the next step, conference papers were filtered out, and only journal papers published in the English language are retained. The shortlisted articles from the keyword searches are exported to EndNote (version X9) library where the citations are removed. A total of 167 articles are collected from the databases by performing a Level 3* search. In the next step, 47 duplicates are removed by using EndNote’s built-in feature. The remaining 110 articles are then evaluated for their relevance to the review paper’s scope by studying their abstracts, methodology, conclusion, and in some cases results and discussion sections. This step leads to the shortlisting of 67 articles. However, 8 more articles that do not appear in the keyword search are later added ad-hoc during the review process making the final list of 75 papers. Moreover, several review papers and articles presenting SCR definitions in Section 3 are excluded from this count. Both Scopus and WOS have the feature of creating a list of citations. For Scopus, it is simply called ‘Lists’ while for WOS it is called ‘Marked Lists’. This feature is used in both databases to collect references however, judging with respect to user-friendliness, we find Scopus lists more intuitive. However, after consolidating results from both the databases in EndNote X9 and removing the duplicate references, we find that WOS was able to retrieve around 5% more references. These additional WOS references are individually checked by running their DOI, and title as search queries in Scopus however, they are not found.
3. Literature review gaps and discussion
In the first part of this section, the supply chain resilience (SCR) definitions are addressed. SCR has been defined in several ways in the literature because the concept of resilience is multidisciplinary and multidimensional (Ponomarov & Holcomb, Citation2009). Among the selected 75 articles, the SCR definitions found in each one are summarized in this section. Subsequently, in the second part of this section, the literature concerning the interplay of SCR and digital transformation from an I4.0 perspective are summarized. First, articles discussing current and future applications of I4.0 technologies such as AI, blockchain, IoT, big data, and deep learning are presented. Next, articles are broadly distributed under different categories as per their area of concern.
3.1. Supply chain resilience definition
A chronological literature survey of selected publications is performed presenting various SCR definitions proposed by the authors among the selected 75 articles. We first study the literature reviews that discuss SCR by compiling definitions from previous research, presenting a new one, and/or endorsing an already published definition.
Williams et al. (Citation2008) survey literature with respect to supply chain security. The authors delineate SCR as per Caniato and Rice (Citation2003) and consider resilience to be an important contributor to supply chain security. Klibi et al. (Citation2010) critically review optimization models of supply chain network (SCN) design. The authors discuss resilience as an important concept in SCN design and conclude that most stochastic models partially consider resilience and responsiveness. They argue that resilient SCN models should have the capability to recover from failures and respond to disruptive events. Ponis and Koronis (Citation2012) present a review of 134 articles and introduce a novel definition of SCR. Furthermore, the authors also agree with Jüttner and Maklan (Citation2011) and Barroso et al. (Citation2011) on the comprehensiveness of the SCR definition presented by Ponomarov and Holcomb (Citation2009) that is multidisciplinary and includes response, recovery, and control among the several important elements if a supply chain disruption occurs. Mandal (Citation2014) surveys 45 journal papers and identifies key research issues in areas such as supply chain design, risk management, sourcing strategies, green practices, supply chain performance, sustainable competitive advantage, SCR, and supply chain security. These 45 journal paper seems to encompass several definitions of SCR from previous works that concur with the interpretation of Ponomarov and Holcomb (Citation2009), albeit they present in their own versions. Heckmann et al. (Citation2015) focus on explaining supply chain risk and the relevant concepts, whereas the authors consider the SCR definitions by Christopher and Peck (Citation2004) and Sheffi and Rice (Citation2005) to be foundational, dominating in the field, and have a widely recognized understanding in supply chain management field. Hohenstein et al. (Citation2015) stress the need for an all-encompassing definitions of SCR and find that there is a scarcity of quantitative methods of SCR performance measurement. The authors systematically review 67 articles from 2003 to 2013 and conclude that the SCR definition has four distinct dimensions to its structure: readiness, response, recovery, and growth. Accordingly, they present an overarching definition that covers the critical aspects overlooked in previous research.
In the same connection, Tukamuhabwa et al. (Citation2015) arguably endorse the comprehensiveness of SCR definitions by Ponomarov and Holcomb (Citation2009) and Ponis and Koronis (Citation2012). The authors consider the term cost-effectiveness as a missing factor from these definitions and include it in theirs after reviewing 91 articles/sources. Kamalahmadi and Parast (Citation2016) review 100 publications and discuss the evolution of definitions and concepts in SCR and supply chain enterprise from 2000 to 2015, and eventually put forward an SCR explanation that considers anticipation, resistance, recovery and response as four phases of SCR. Lin et al. (Citation2016) present the concept of a holistic supply chain which is a set of interdependent supply chains. The authors review 43 articles to describe resilient supply chain systems and present a comprehensive SCR interpretation, which is compounded by objective, anticipation, estimation, and preparation. Ali et al. (Citation2017) include 103 papers from 2000 to 2015 in their review and classify various SCR features by mapping their key research concepts, which draw interactions and relationships between them. They chronologically study numerous SCR definitions and find three major constructs and five core capabilities for a comprehensive one. Kochan and Nowicki (Citation2018) review 228 articles from 2000 to 2017. The authors develop a typological framework for a better understanding of SCR and identify relevant assessment techniques and measures. The authors list several SCR definitions from the previous works and contribute by providing clearer characterization of SCR constructs. Ribeiro and Barbosa-Povoa (Citation2018) analyze the development of quantitative methods in SCR, list SCR definitions from 27 articles from 2003 to 2016 and propose a comprehensive SCR meaning supported by the identified four SCR elements: focus event, adaptive framing or adaptive response, speed, and performance level. Golgeci and Ponomarov (Citation2013) cover SCR theory by performing a co-occurrence analysis of the articles published between 2003 and 2018 and quote SCR definition by Ponomarov and Holcomb (Citation2009) and regard it as one of the seminal ones in the literature.
Gligor et al. (Citation2019) perform a multidisciplinary literature review to find similarities and differences between agility and resilience, which are evaluated by scrutinizing their definitions from the literature. Common themes among agility and resilience are found as: the ability to speed and accelerate operations, the ability to scan the environment/anticipate, and the ability to adjust tactics and operations (flexibility). Hosseini, Ivanov, et al. (Citation2019) present a review of the quantitative modeling of SCR, where qualitative and quantitative drivers of SCR are both reviewed. A qualitative analysis of SCR definitions from the previous works is presented. Accordingly, the authors present a new rational based on the concept of resilience capacity, which includes absorptive, adaptive, and restorative capacities. Lotfi (Citation2019) discusses lean, agile, and resilient practices using empirical research, the author uses the SCR definition of Ponomarov and Holcomb (Christopher & Peck, Citation2004), because of its alignment with the extant literature. On the other hand, the review paper of Golan et al. (Citation2020) discusses resilience modeling and quantification and connects the supply chain to networks including transportation, command and control. The authors stress that the main feature of SCR is its recovery after a disruptive event. Han et al. (Citation2020) synthesize from the aforementioned literature review that the most common elements found in the SCR definitions are the abilities to prepare for, respond, and recover from supply chain disruptions, where particular reference is made to readiness, response, and recovery as the three SCR dimensions in the development of SCR Capabilities-Performance Metrics Framework (SCPM). On the contrary, Sawyerr et al. (Citation2020) identify supply chain collaboration, flexibility, redundancy, and agility as the most common while sustainability and logistics capability as the least common formative elements of SCR definitions in their review of 107 articles. Negri et al. (Citation2021) take a deeper look at the concepts of sustainability and resilience in the supply chain and analyzed 108 papers and eventually offered several definitions of SCR from the previous works, explain tradeoffs and synergies between resilience and sustainability, and accordingly define SCR. Hägele et al. (Citation2022) contribute by focusing on balancing vulnerabilities and capabilities of the supply chain to achieve resilience, the authors elucidate SCR by summarizing what is perceived as the core of SCR definitions from previous works.
Ekanayake et al. (Citation2021) introduce a quantitative research mapping of the SCR domain, which provides a publication base, research hotspots, key milestones, and a dynamic platform to include future SCR research. They perform a co-citation analysis, which is a measure of a paper’s ‘prestige’ and is calculated by the number of times an article is cited by other highly cited publications. The review paper finds that Pettit et al. (Citation2010) and Ponomarov et al. (2009) are the first and second most highly cited papers, respectively. The latter receives high popularity due to its contribution by determining a comprehensive SCR definition, while the former receives its recognition from introducing the concept of resilience fitness space, which is considered a foundational research area that advocates for striking an optimum balance between SCR and supply chain vulnerabilities (SCV). Rahman et al. (Citation2022) review 151 articles from 2010 to 2021 for initiatives and strategies in SCR. Furthermore, the authors report that there is a lack of theoretically grounded and simulation-based studies of large-scale supply chain disruption events. Tortorella et al. (Citation2022) discuss the contribution of I4.0 in SCR and adopt the SCR definition of Ponomarov et al. (2009) because of its wide acceptance in the academic community. Among the selected 75 articles, the SCR definitions found in each one are summarized in .
Table 1. SCR definitions by authors from 2000 to 2022.
3.2. Supply chain resilience definition development
In the quest of analyzing previous SCR definitions, we find that a lack of consistency exists due to vast disagreement on the elements of SCR; therefore, researchers have yet to agree on a comprehensive SCR definition. In this review, a unique approach is adopted to analyze the SCR definitions, in which R programming is used to perform data analysis of the SCR definitions published in the articles published from 2000 to 2022. This aims to identify the common words or terms used to define SCR by calculating their frequencies, the obtained data analysis is then used to construct an SCR definition in an objective manner by using words that have a frequency greater than four.
We use the text mining library of R called ‘tidytext’ (Silge & Robinson, Citation2016) to process the text and to convert it into ‘tidy’ format (Silge & Robinson, Citation2017). Using this library, all the words from the text of definitions are picked and put in individual rows. Afterwards, the data manipulation library called ‘dplyr’ is used with the objective to resolve operation problems through providing simple functions. To simplify the process, simple common English words such as ‘an’, ‘to’, ‘as’, and others, are removed. These words are already included in the database of dplyr function ‘stop_words’, hence, removed using the function ‘anti_join (stop_words)’. In addition to the ‘stop words’ a custom list of words such as ‘chain’, ‘resilience’, ‘supply’, ‘system’, ‘ability’, and others is included with the objective to make the analysis more judicious because such words are common in most of the SCR definitions and including them in the analysis may not help in yielding the optimum results. At the end, the R library ‘ggplot2’ (Wickham, Citation2016) is used in order to create a bar plot of words with a frequency greater than 4, as seen in .
Figure 3. Bar plot of frequent words in the supply chain resilience (SCR) definitions of articles published from 2000 to 2022.
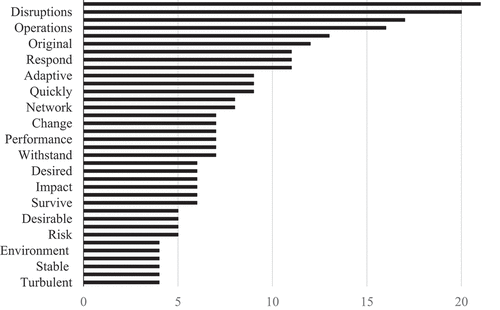
After completing the analysis of identifying high-frequency words and terms in the published SCR definitions, our SCR definition emerges as follows:
The capability of a supply chain to prepare for disruptions, respond to unexpected events, withstand disturbances, restore operation, and quickly recover the costs by maintaining desirable growth levels.
3.3. Industry 4.0 role in supply chain resilience
The fourth industrial revolution augmented supply chains with ample technology enablers that yield opportunities and threats, for example blockchain is a safe and secure peer-to-peer network that uses distributed ledgers (DL) and is independent of the control of the government, banks, and any intermediaries. Sophisticated encryption, and hashing processes, added to its decentralization, mitigate risks such as hacking, privacy breach, and effects of political instability. These advantages of blockchain technology make it suitable to be employed for building resilient supply chains (Min, Citation2019) and complex multi-echelon modern supply chain systems (Mukherjee et al., Citation2022). The DL technologies help solve supply chain bottlenecks such as obstructed data flows, bullwhip effect, new lines of product, and high inventory of products (Asante Boakye et al., Citation2022). The usage of blockchain and other I4.0 technologies such as IoT, AI, augmented reality (AR), and virtual reality (VR) has been discussed by Akram et al. (Citation2022) specifically for the supply chain of the fashion industry.
Providing a different perspective, Saengchai and Jermsittiparsert (Citation2019) note that supply chain agility and resilience provide organizational stability for I4.0 implementation. However, Berawi et al. (Citation2020) discuss that the modern digital technologies of I4.0 such as AI, deep learning, machine learning, IoT, and big data are helping businesses to achieve a competitive advantage by transforming their business models and creating more resilient supply chains. Miceli et al. (Citation2021) also report that a proactive, opportunity-focused attitude along with digitalized and agile processes help to create strategic resilience.
Business excellence can be achieved by applying the concepts of Lean, Agile, Green, and Resilient (collectively called LARG) in modern manufacturing with the help of I4.0 technologies, which can result in environmental, financial, and operational benefits (Amjad et al., Citation2020). LARG concepts in a supply chain are proposed by Sahu et al. (Citation2022). They present LARG matrices to achieve sustainable supply chain operations.
Ripple-effect disruptions pose a significant threat to normal supply chain operations. Kinra et al. (Citation2020) study the consequences of ripple-effect supply chain disruptions by developing a model based on the maximum possible loss, which is validated by simulating actual data. It helps in finding dormant high-risk supplier relations, and prioritizing risk mitigation measures under tough probability estimations. In this context, Mithani (Citation2020) gives a fresh perspective on organizational challenges to adaptation in the wake of increasing life-threatening events. The author identifies models that are more suited to cope with these challenges. A framework based on integrated quality function deployment (QFD) and multi-criteria decision-making (MCDM), coupled with the most urgent I4.0 enablers such as data security and privacy, infrastructure development for big data management, transition to digitalization, new I4.0 equipment, and investment, can improve supply chain agility, enhance economic efficiency and mitigate ripple effects (Hsu et al., Citation2022).
Digital supply chains (DSCs) can help manufacturing companies increase their quality management functions. Joshi and Sharma (Citation2022) identify several crucial factors of DSCs for suppliers. The cognitive ability of digital twins is their characteristic to predict and be flexible in dynamic situations. Kalaboukas et al. (Citation2021) explain the concept of Cognitive Digital Twins (CDTs) and their deployment in agile supply chain models. By integrating simulation and machine learning-based models, digital twins can be used for managing supply chain disruptions. Badakhshan and Ball (Citation2022) minimize the impact of disruptions on supply chain performance by using the digital twin model for the identification of inventory and cash refilling policies. The model benefits upstream supply chain members by reducing their cash conversion cycle.
DSCs can be developed by strategically investing in digital technologies and its associated distinctive resources (Irfan et al., Citation2022). Cloud-based supply chains fall under the category of DSCs. Ivanov et al. (Citation2022) conceptualize the cloud supply chain as a distinctive research area, present it as a business model, and outlined its chief characteristics. Furthermore, continuous conversion and consolidation of DSC management are needed to increase business resilience, sustainability, and agility (Minculete et al., Citation2022). In the bid to develop DSCs, there is always a looming risk of cyberattacks that could cause supply chain disruptions. Melnyk et al. (Citation2022) discuss the economic, political, and social effects of breaches in cybersecurity and discuss the critical need for cyber-secure supply chain operations in the context of SCR.
Kaur and Singh (Citation2021) develop a multi-stage hybrid model for integrated supplier selection, segmentation, and order allocation in the scenario of risk or occurrence of a disruptive event. The authors consider suppliers to be critical to supply chain functioning as they need to adapt to positive (transition to I4.0) or negative events. The model reduces the economic impact of abnormal events on the supply or demand side and ensures supply chain operations by allocating emergency orders. Chari et al. (Citation2022) explore how dynamic capabilities theory enables SCR and circular economy. Furthermore, Tamym et al. (Citation2021) propose a collaborative mixed supply chain network architecture based on big data. The connected supply chains in the mixed network collaborate by sharing information, resources, and competencies to mitigate, analyze, predict, and respond to risks. This creates a robust network with resilience capabilities.
3.4. Investment in the industry 4.0
Investment is generally associated with expenditures of financial funds in projects with the objective of yielding future returns, however investment can be argued to include closing down non-revenue generating businesses which eventually saves funds that can be invested in businesses with better financial future prospects (Dixit & Pindyck, Citation2009). In the same connection, Ioannou et al. (Citation2018) evaluate the investment in installing wind energy capacity, and addressed the capital expenditures (CAPEX) and the operating expenses (OPEX), where CAPEX are an organization’s major and long term expenses, while OPEX are an organization’s operational expenses. Furthermore, Bosman et al. (Citation2020) build on the abovementioned concept and addressed the importance of investment in I4.0, as an important consideration for manufacturing companies who aims to be competitive in the current volatile and ever-changing global economy. The authors have also stressed on the fact that decisions pertaining to (a) how much to invest? and (b) which technologies to invest in? continues to be engulfed with uncertainties and complexities, which are problems most of today’s organizations are suffering from. This dilemma is further addressed by Pettit et al. (Citation2010), however with an attempt to demistify the process, as their research introduce the concept of resilience fitness space (RFS), where it is the space where an organization strikes a balance between optimum investment that creates ideal resilience. As over investment in the building resilience capacity leads to eroding an organization profits, while under investment exposes the organization to increasing risks. Hence, careful reviews and assessment of the effectiveness of all potential I4.0 technology enablers are to be conducted against the particular and targeted risks and vulnerabilities. Where only the most suitable solutions are to be considered in a manner that ensure yielding best investment decisions.
As outcome of this review paper, a roadmap towards DSCR under investment constraints may be addressed. It can offer, as example, key recommendations to enhance the trustworthiness of an AI+IoT eco-system via knowledge-based considerations of its interconnected layers that encompass the device, the internet, the support, and the application layer. The IoT ecosystem is categorized in Sharma et al. (Citation2020) into four layers, as shown in . In the maritime shipping environment, for example, sending huge amounts of data from the middle of the ocean to the headquarters of the ship owners for review and analysis, can be an expensive affair, as well as it could be subject to an array of risks that may affect the quantity and quality of the transmitted data.
Figure 4. Four-layer architecture of IoT critical eco-system (adapted from Sharma et al., Citation2020).
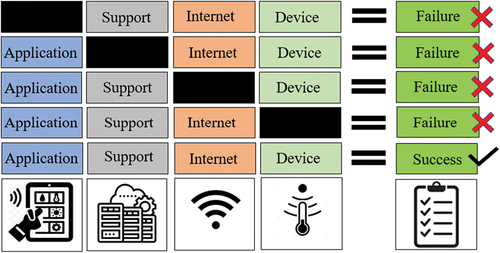
3.5. Cost of cybersecurity vs. cyberattacks
In the context of DSCR investment, cybersecurity is considered one of the highest priorities because of the increasing reliance on digital technologies and interconnectivity across the various supply chain ecosystem elements, which exposes organizations to new and evolving cyber threats. As highlighted by Radanliev et al. (Citation2021, Citation2022), Haner and Knake (Citation2021), Melnyk et al. (Citation2022), the integration of emerging technologies such as AI, IoT, digital twins, among others presents new cyber risks that must be effectively managed to maintain an efficient and reliable digital supply chain resilience (Zeb & Kortelainen, Citation2021). Furthermore, Radanliev and De Roure’s (Citation2022) research about advancing the cybersecurity of the healthcare system proposes a self-optimizing and self-adaptive AI system that aims to enhance the cybersecurity of the healthcare system. The research highlights the increasing frequency and complexity of cyber attacks on healthcare systems, which can have serious consequences for patient care and privacy. Hence, it is crucial to consider a robust cybersecurity framework that utilizes self-optimizing and self-adaptive AI to improve the detection and response to cyber threats, which yields towards an elevated healthcare systems resilience against cyber threats and protect the confidentiality, integrity, and availability of patient data. In the manufacturing industry, Pérez et al. (Citation2020) proposed a methodology for multi-robot manufacturing cell commissioning using digital twin and virtual reality, which can improve the efficiency of the commissioning process and identify potential vulnerabilities and threats before they occur. Zeb and Kortelainen (Citation2021) also emphasized the importance of integrating cybersecurity measures in the design phase of manufacturing systems to prevent cyberattacks (Zeb & Kortelainen, Citation2021). And Pérez et al. (Citation2020) proposed a framework for secure design and operation of I4.0 systems, which includes threat modeling and risk assessment as key components. These research efforts highlight the need for proactive and comprehensive cybersecurity measures in the commissioning and design phases of manufacturing facilities to prevent cyberattacks and minimize their impact.
From a holistic perspective, the increasing complexity of global supply chains and the dependence on digital technologies have made organizations more vulnerable to cyberattacks, which can result in significant financial losses, reputational damage, and disruption of business operations. This emphasize the need for effective cybersecurity measures such as encryption, intrusion detection systems, and firewalls to manage potential cyber risks and maintain supply chain resilience.
Cyberattacks frequency and intensity have rapidly increased during the recent years, with some of the largest and most costly incidents occurring in 2020 and 2021. For example, the SolarWinds cyberattack was one of the most significant breaches in history, affecting numerous government agencies and major corporations. The attackers were able to compromise SolarWinds’ software and use it to gain access to sensitive data, resulting in huge financial and reputational damages Burmeister and Meng (Citation2021). This incident highlights the growing threat of supply chain attacks, where attackers target a third-party vendor to gain access to an organization’s network. Another major cyberattack in 2021 was the Colonial Pipeline ransomware attack, which caused widespread disruptions to fuel supplies on the east coast of the United States. As highlighted by Dudley and Golden (Citation2021) the attack was attributed to the DarkSide hacking group and resulted in a ransom payment of more than US$ 4 million. The incident highlights the growing trend of ransomware attacks, where attackers encrypt an organization’s data and demand payment to restore access The cost of cyberattacks can be significant, with estimates ranging from millions to billions of dollars in damages. In addition to direct financial costs, cyberattacks can also result in reputational damage, legal liability, and lost productivity (Hobbs, Citation2021).
To address the growing threat of cyberattacks, organizations are investing in cybersecurity measures such as network monitoring, employee training, and incident response planning. However, as the attacks become increasingly sophisticated, there is a need for continued research and innovation in the field of cybersecurity to stay ahead of evolving threats. Therefore, investment in cybersecurity measures and regular cybersecurity training for all stakeholders in the supply chain is crucial to mitigate potential cyber risks and ensure digital supply chain resilience. Melnyk et al. (Citation2022) also advocate for the adoption of standardized security protocols across the supply chain to promote cybersecurity best practices and enhance information sharing and collaboration.
4. Conclusion
The paper highlights the importance of SCR in the current fast-paced business environment and the need for organizations to adopt a proactive approach towards DSCR instead of traditional SCR. The paper emphasizes the significance of investing in the right I4.0 technology enablers and addressing potential vulnerabilities to achieve DSCR. The paper concludes that organizations need to define their own Resilience Fitness Space (RFS) based on their organizational needs, industry’s current and desired SCR status, their regulatory and operating environment, among others. To adopt DSCR, organizations must identify the optimum I4.0 technology enablers to invest in, along with their supporting ecosystems. The selection of the technology enablers must interlink SCR and SCV drivers with the I4.0 dimension. The ideal identification of the I4.0 technology enabler and the optimum level of investment could be considered by interlinking Zhang et al. (Citation2021) two key denominators of supply chain resilience (SCR) and supply chain vulnerabilities (SCV) drivers with the I4.0 dimension. Such a matrix helps in selecting the ideal I4.0 technology enabler that supports a targeted SCR driver aimed at combating a specific SCV driver. This structured approach towards digital transformation is essential for organizations, and they must follow a roadmap that is cautious in terms of investment to mitigate financial constraints, especially after the COVID-19 pandemic’s financial damages. However, implementing DSCR can be complex, multi-faceted, and time-consuming, leading to multiple challenges. These challenges include -but are not limited to- resistance to change, lack of expertise in digital supply chain technologies, and difficulty in justifying the investment to shareholders and stakeholders due to the fact that the benefits of investing in DSCR are not usually immediately visible. Therefore, organizations must consider various strategies to facilitate efficient uptake of DSCR investment and sufficient adoption rates of the deployed I4.0 technologies. The first strategy to consider is to educate employees about the benefits of DSCR and its implementation in daily operations. Training sessions can help employees understand the concept of DSCR and how it can improve organizational SCR, performance, and eventually the financial performance of the organization, resulting in enhanced compensation and benefits for the employees. The second strategy is to conduct a cost-benefit analysis to determine the potential return on investment (ROI) of investing in DSCR. The ROI can be estimated by considering the potential reduction in supply chain disruptions, increased efficiency, and improved customer satisfaction. A positive ROI can help justify the investment to shareholders and stakeholders. The third strategy is collaborating with supply chain partners to identify vulnerabilities in their supply chains and determining the appropriate digital supply chain technologies to mitigate those vulnerabilities. This can also help reduce implementation costs by sharing investment costs and benefits. Finally, the fourth strategy is to pilot new technologies on a small scale to determine their effectiveness before integrating them into the organization’s existing supply chain ecosystems. This can help to reduce the risks associated with implementing new technologies and provide proof of concept to stakeholders.
The paper’s findings also suggest several potential research gaps, including the need for more empirical studies on the impact of digital transformation on supply chain resilience, the role of organizational culture in supporting digital transformation initiatives, and the potential trade-offs between efficiency and resilience in the context of digital transformation. The paper also highlights the importance of considering the cost of cybersecurity versus the cost of cyber attacks.
In conclusion, the paper’s research provides valuable insights for organizations seeking to enhance their digital resilience in the face of disruptive shocks. By embracing digital transformation and integrating SCR, I4.0, and INV, organizations can build more resilient supply chains that can adapt and thrive in the rapidly changing global economy. Furthermore, by considering the cost of cybersecurity versus the cost of cyber attacks, organizations can make informed decisions on investing in digital supply chain resilience to mitigate financial damages caused by potential cyber attacks, leading to an efficient, secure and robust digital supply chains resilience.
Acknowledgments
Open Access funding provided by the Qatar National Library.
Disclosure statement
No potential conflict of interest was reported by the author(s).
Data availability statement
The data of this study are available from the corresponding author upon request.
References
- Akram, S. V., Malik, P. K., Singh, R., Gehlot, A., Juyal, A., Ghafoor, K. Z., & Shrestha, S. (2022). Implementation of digitalized technologies for fashion industry 4.0: Opportunities and challenges. Scientific Programming.
- AlBanna, A., Franzoi, R. E., Menezes, B. C., Al-Enazi, A., Rogers, S., & Kelly, J. D. (2022). Roadmap to digital supply chain resilience. Computer aided chemical engineering (Vol. 49, pp. 571–23). Elsevier. https://doi.org/10.1016/B978-0-323-85159-6.50095-6.
- Al-Banna A, Yaqot M and Menezes B. (2023). Roadmap to digital supply chain resilience under investment constraints. Production & Manufacturing Research, 11(1). https://doi.org/10.1080/21693277.2023.2194943
- Ali, A., Mahfouz, A., & Arisha, A. (2017). Analysing supply chain resilience: Integrating the constructs in a concept mapping framework via a systematic literature review. Supply Chain Management: An International Journal, 22(1), 16–39. https://doi.org/10.1108/SCM-06-2016-0197
- Ambulkar, S., Blackhurst, J., & Grawe, S. (2015). Firm’s resilience to supply chain disruptions: Scale development and empirical examination. Journal of Operations Management, 33-34, 111–122. https://doi.org/10.1016/j.jom.2014.11.002
- Amjad, M. S., Rafique, M. Z., Hussain, S., & Khan, M. A. (2020). A new vision of LARG Manufacturing - a trail towards industry 4.0. CIRP Journal of Manufacturing Science and Technology, 31, 377–393. https://doi.org/10.1016/j.cirpj.2020.06.012
- Asante Boakye, E., Zhao, H., & Ahia, B. N. K. (2022). Blockchain technology prospects in transforming Ghana’s economy: A phenomenon-based approach. Information Technology for Development.
- Badakhshan, E., & Ball, P. (2022). Applying digital twins for inventory and cash management in supply chains under physical and financial disruptions. International Journal of Production Research, 23, 1–23. https://doi.org/10.1080/00207543.2022.2093682
- Barroso, A. P., Machado, V. H., & Machado, V. C. (2011). Supply chain resilience using the mapping approach. Supply Chain Management, 161–184.
- Berawi, M. A., Suwartha, N., Asvial, M., Harwahyu, R., Suryanegara, M., Setiawan, E. A., Surjandari, I., Zagloel, T. Y. M., & Maknun, I. J. (2020). Digital innovation: Creating competitive advantages. International Journal of Technology, 11(6), 1076–1080. https://doi.org/10.14716/ijtech.v11i6.4581
- Berle, Ø., Norstad, I., & Asbjørnslett, B. E. (2013). Optimization, risk assessment and resilience in LNG transportation systems. Supply Chain Management, 18(3), 253–264. https://doi.org/10.1108/SCM-03-2012-0109
- Blackhurst, J., Kaitlin, S. D., & C, C. W. (2011). An empirically derived framework of global supply resiliency. Journal of Business Logistics, 32(4), 374–391. https://doi.org/10.1111/j.0000-0000.2011.01032.x
- Bosman, L., Hartman, N., & Sutherland, J. (2020). How manufacturing firm characteristics can influence decision making for investing in Industry 4.0 technologies. Journal of Manufacturing Technology Management, 31(5), 1117–1141.
- Brandon‐Jones, E., Squire, B., Autry, C. W., & Petersen, K. J. (2014). A contingent resource‐based perspective of supply chain resilience and robustness. The Journal of Supply Chain Management, 50(3), 55–73. https://doi.org/10.1111/jscm.12050
- Burmeister, J. K., & Meng, Z. (2021). Kant, cybernetics, and cybersecurity: Integration and secure computation. https://doi.org/10.1186/s13328-021-00476-6
- Cabral, I., Grilo, A., & Cruz Machado, V. (2012). A decision-making model for lean, agile, resilient and green supply chain management. International Journal of Production Research, 50(17), 4830–4845. https://doi.org/10.1080/00207543.2012.657970
- Caniato, F. F. A., & Rice, J. (2003). Building a secure and resilient supply chain, 7(5), 22–30.
- Carvalho, H., Azevedo, S. G., & Cruz Machado, V. (2012). Agile and resilient approaches to supply chain management: Influence on performance and competitiveness. Logistics Research, 4(1–2), 49–62. https://doi.org/10.1007/s12159-012-0064-2
- Carvalho, H., Duarte, S., & Machado, V. C. (2011). Lean, agile, resilient and green: Divergencies and synergies. International Journal of Lean Six Sigma, 2(2), 151–179. https://doi.org/10.1108/20401461111135037
- Chari, A., Niedenzu, D., Despeisse, M., Machado, C. G., Azevedo, J. D., Boavida Dias, R., & Johansson, B. (2022). Dynamic capabilities for circular manufacturing supply chains—Exploring the role of industry 4.0 and resilience. Business Strategy and the Environment, 31(5), 2500–2517. https://doi.org/10.1002/bse.3040
- Chen, Y. (2017). Integrated and intelligent manufacturing: Perspectives and enablers. Engineering, 3(5), 588–595. https://doi.org/10.1016/J.ENG.2017.04.009
- Chen, Y. G., Wang, S., Yao, J. R., Li, Y. X., & Yang, S. Q. (2018). Socially responsible supplier selection and sustainable supply chain development: A combined approach of total interpretive structural modeling and fuzzy analytic network process. Business Strategy and the Environment, 27(8), 1708–1719. https://doi.org/10.1002/bse.2236
- Chowdhury, M. M. H., & Quaddus, M. (2017). Supply chain resilience: Conceptualization and scale development using dynamic capability theory. International Journal of Production Economics, 188, 185–204. https://doi.org/10.1016/j.ijpe.2017.03.020
- Christopher, M., & Peck, H. (2004). Building the resilient supply chain. The International Journal of Logistics Management, 15(2), 1–14. https://doi.org/10.1108/09574090410700275
- Closs, D. J., & McGarrell, E. F. (2004). Enhancing security throughout the supply chain. IBM Center for the Business of Government.
- Datta, P. P., Christopher, M., & Allen, P. (2007). Agent-based modelling of complex production/distribution systems to improve resilience. International Journal of Logistics: Research & Applications, 10(3), 187–203. https://doi.org/10.1080/13675560701467144
- Day, J. M. (2014). Fostering emergent resilience: The complex adaptive supply network of disaster relief. International Journal of Production Research, 52(7), 1970–1988. https://doi.org/10.1080/00207543.2013.787496
- Dixit, A. K., & Pindyck, R. S. (2009). The options approach to capital investment. In The economic impact of knowledge (pp. 325–340). Routledge. https://doi.org/10.1016/B978-0-7506-7009-8.50024-0
- Dudley, R., & Golden, D. (2021). The colonial pipeline ransomware hackers had a secret weapon: Self-promoting cybersecurity firms. MIT Technology Review and ProPublica.
- Ekanayake, E. M. A. C., Shen, G. Q., & Kumaraswamy, M. (2021). Supply chain resilience: Mapping the knowledge domains through a bibliometric approach. Built Environment Project and Asset Management, 11(4), 705–721. https://doi.org/10.1108/BEPAM-03-2020-0040
- Erol, O., Sauser, B. J., & Mansouri, M. (2010). A framework for investigation into extended enterprise resilience. Enterprise Information Systems, 4(2), 111–136. https://doi.org/10.1080/17517570903474304
- Fiksel, J. (2006). Sustainability and resilience: Toward a systems approach. Sustainability: Science, Practice & Policy, 2(2), 14–21. https://doi.org/10.1080/15487733.2006.11907980
- Gaonkar, R. S., & Viswanadham, N. (2007). Analytical framework for the management of risk in supply chains. IEEE Transactions on Automation Science and Engineering, 4(2), 265–273. https://doi.org/10.1109/TASE.2006.880540
- Geng, L., Xiao, R., & Xie, S. (2013). Research on self-organization in resilient recovery of cluster supply chains. Discrete Dynamics in Nature & Society, 2013, 758967. https://doi.org/10.1155/2013/758967
- Gligor, D., Gligor, N., Holcomb, M., & Bozkurt, S. (2019). Distinguishing between the concepts of supply chain agility and resilience. The International Journal of Logistics Management, 30(2), 467–487. https://doi.org/10.1108/IJLM-10-2017-0259
- Golan, M. S., Jernegan, L. H., & Linkov, I. (2020). Trends and applications of resilience analytics in supply chain modeling: Systematic literature review in the context of the COVID-19 pandemic. Environment Systems and Decisions, 40(2), 222–243. https://doi.org/10.1007/s10669-020-09777-w
- Golgeci, I., & Ponomarov, S. Y. (2013). Does firm innovativeness enable effective responses to supply chain disruptions? An empirical study. Supply Chain Management: An International Journal, 18(6), 604–617. https://doi.org/10.1108/SCM-10-2012-0331
- Hägele, S., Grosse, E., & Ivanov, D. (2022). Supply chain resilience: A tertiary study. International Journal of Integrated Supply Management, 16(1), 52. https://doi.org/10.1504/IJISM.2023.127660
- Han, Y., Chong, W. K., & Li, D. (2020). A systematic literature review of the capabilities and performance metrics of supply chain resilience. International Journal of Production Research, 58(15), 4541–4566. https://doi.org/10.1080/00207543.2020.1785034
- Haner, J. K., & Knake, R. K. (2021). Breaking botnets: A quantitative analysis of individual, technical, isolationist, and multilateral approaches to cybersecurity. Journal of Cybersecurity, 7(1), tyab003. https://doi.org/10.1093/cybsec/tyab003
- Hearnshaw, E. J. S., & Wilson, M. M. J. (2013). A complex network approach to supply chain network theory. International Journal of Operations & Production Management, 33(4), 442–469. https://doi.org/10.1108/01443571311307343
- Heckmann, I., Comes, T., & Nickel, S. (2015). A critical review on supply chain risk - definition, measure and modeling. Omega-International Journal of Management Science, 52, 119–132. https://doi.org/10.1016/j.omega.2014.10.004
- Higgins, A. J. M., Miller, C. J., Archer, A. A., Ton, T., Flecher, C. S., & McAllister, R. R. J. (2010). Challenges of operations research practice in agricultural value chains. Journal of Operational Research Society, 61(6), 964–973. https://doi.org/10.1057/jors.2009.57
- Hobbs, A. (2021). The colonial pipeline hack: Exposing vulnerabilities in us cybersecurity. In SAGE business cases. SAGE Publications: SAGE Business Cases Originals. https://doi.org/10.4135/9781529789768
- Hohenstein, N. O., Feisel, E., Hartmann, E., Giunipero, L., Maria Jesus Saenz, P., & Xenophon Koufteros, D. (2015). Research on the phenomenon of supply chain resilience a systematic review and paths for further investigation. International Journal of Physical Distribution & Logistics Management, 45(1/2), 90–117. https://doi.org/10.1108/IJPDLM-05-2013-0128
- Hosseini, S., Ivanov, D., & Dolgui, A. (2019). Review of quantitative methods for supply chain resilience analysis. Transportation Research Part E: Logistics & Transportation Review, 125, 285–307. https://doi.org/10.1016/j.tre.2019.03.001
- Hosseini, S., Morshedlou, N., Ivanov, D., Sarderd, M., Barker, K., & Al Khaled, A. (2019). Resilient supplier selection and optimal order allocation under disruption risks. International Journal of Production Economics, 213, 124–137. https://doi.org/10.1016/j.ijpe.2019.03.018
- Hsu, C. H., He, X., Zhang, T. Y., Chang, A. Y., Liu, W. L., & Lin, Z. Q. (2022). Enhancing supply chain agility with industry 4.0 enablers to mitigate ripple effects based on integrated QFD-MCDM: An empirical study of new energy materials manufacturers. Mathematics, 10(10), 35. https://doi.org/10.3390/math10101635
- Iakovou, E., Vlachos, D., & Xanthopoulos, A. (2010). A stochastic inventory management model for a dual sourcing supply chain with disruptions. International Journal of Systems Science, 41(3), 315–324. https://doi.org/10.1080/00207720903326894
- Ioannou, A., Angus, A., & Brennan, F. (2018). Parametric CAPEX, OPEX, and LCOE expressions for offshore wind farms based on global deployment parameters. Energy Sources, Part B: Economics, Planning, & Policy, 13(5), 281–290. https://doi.org/10.1080/15567249.2018.1461150
- Irfan, I., Sumbal, M., Khurshid, F., & Chan, F. T. S. (2022). Toward a resilient supply chain model: Critical role of knowledge management and dynamic capabilities. Industrial Management & Data Systems, 122(5), 1153–1182. https://doi.org/10.1108/IMDS-06-2021-0356
- Ishfaq, R. (2012). Resilience through flexibility in transportation operations. International Journal of Logistics: Research & Applications, 15, 215–229. https://doi.org/10.1080/13675567.2012.709835.
- Ivanov, D., Dolgui, A., & Sokolov, B. (2022). Cloud supply chain: Integrating industry 4.0 and digital platforms in the “Supply Chain-as-a-Service”. Transportation Research Part E: Logistics & Transportation Review, 160, 102676. https://doi.org/10.1016/j.tre.2022.102676
- Ivanov, D., & Sokolov, B. (2013). Control and system-theoretic identification of the supply chain dynamics domain for planning, analysis and adaptation of performance under uncertainty. European Journal of Operational Research, 224(2), 313–323. https://doi.org/10.1016/j.ejor.2012.08.021
- Johnson, N., Elliott, D., & Drake, P. (2013). Exploring the role of social capital in facilitating supply chain resilience. Supply Chain Management: An International Journal, 18(3), 324–336. https://doi.org/10.1108/SCM-06-2012-0203
- Joshi, S., & Sharma, M. (2022). Digital technologies (DT) adoption in agri-food supply chains amidst COVID-19: An approach towards food security concerns in developing countries. Journal of Global Operations and Strategic Sourcing, 15(2), 262–282. https://doi.org/10.1108/JGOSS-02-2021-0014
- Jüttner, U., & Maklan, S. (2011). Supply chain resilience in the global financial crisis: An empirical study. Supply Chain Management: An International Journal, 16(4), 246–259. https://doi.org/10.1108/13598541111139062
- Kalaboukas, K., Rožanec, J., Košmerlj, A., Kiritsis, D., & Arampatzis, G. (2021). Implementation of cognitive digital twins in connected and agile supply networks-an operational model. Applied Sciences (Switzerland), 11. https://doi.org/10.3390/app11094103
- Kamalahmadi, M., & Parast, M. M. (2016). A review of the literature on the principles of enterprise and supply chain resilience: Major findings and directions for future research. International Journal of Production Economics, 171, 116–133. https://doi.org/10.1016/j.ijpe.2015.10.023
- Kaur, H., & Singh, S. P. (2021). Multi-stage hybrid model for supplier selection and order allocation considering disruption risks and disruptive technologies. International Journal of Production Economics, 231, 25. https://doi.org/10.1016/j.ijpe.2020.107830
- Kim, Y., Chen, Y. S., & Linderman, K. (2015). Supply network disruption and resilience: A network structural perspective. Journal of Operations Management, 33-34(1), 43–59. https://doi.org/10.1016/j.jom.2014.10.006
- Kinra, A., Ivanov, D., Das, A., & Dolgui, A. (2020). Ripple effect quantification by supplier risk exposure assessment. International Journal of Production Research, 58(18), 5559–5578. https://doi.org/10.1080/00207543.2019.1675919
- Klibi, W., Martel, A., & Guitouni, A. (2010). The design of robust value-creating supply chain networks: A critical review. European Journal of Operational Research, 203(2), 283–293. https://doi.org/10.1016/j.ejor.2009.06.011
- Kochan, C. G., & Nowicki, D. R. (2018). Supply chain resilience: A systematic literature review and typological framework. International Journal of Physical Distribution and Logistics Management, 48(8), 842–865. https://doi.org/10.1108/IJPDLM-02-2017-0099
- Kumar, S., & Sosnoski, M. (2011). Decision framework for the analysis and selection of appropriate transfer pricing for a resilient global SME manufacturing operation – a business case. International Journal of Production Research, 49(18), 5431–5448. https://doi.org/10.1080/00207543.2011.563835
- Kumar, S. K., Tiwari, M. K., & B, R. F. (2010). Minimisation of supply chain cost with embedded risk using computational intelligence approaches. International Journal of Production Research, 48(13), 3717–3739. https://doi.org/10.1080/00207540902893425
- Levesque, P. J. (2012). Building resilience and sustainability into the Chinese supply chain. Global Business and Organizational Excellence, 31(3), 69–83. https://doi.org/10.1002/joe.21427
- Lin, H., Chen, C., Wang, J., Qi, J., Jin, D., Kalbarczyk, Z. T., & Iyer, R. K. (2016). Self-healing attack-resilient PMU network for power system operation. IEEE Transactions on Smart Grid, 9(3), 1551–1565. https://doi.org/10.1109/TSG.2016.2593021
- Lotfi, M. (2019). Which practices are lean, agile and resilient? Literature review and practitioners’ perspective. International Journal of Advanced Operations Management, 11(1/2), 142–170. https://doi.org/10.1504/IJAOM.2019.098522
- Machowiak, W. (2012). Risk management-unappreciated instrument of supply chain management strategy. LogForum, 8(4). https://doi.org/10.17270/B.M.978-83-62285-25-9.2.1
- Mandal, S. (2012). An empirical investigation into supply chain resilience. IUP Journal of Supply Chain Management, 9, 46–61.
- Mandal, S. (2014). Supply chain resilience: A state-of-the-art review and research directions. International Journal of Disaster Resilience in the Built Environment, 5(4), 427–453. https://doi.org/10.1108/IJDRBE-03-2013-0003
- Melnyk, S. A., Closs, D. J., Griffis, S. E., Zobel, C. W., & Macdonald, J. R. (2014). Understanding supply chain resilience. Supply Chain Management Review, 18, 34–41.
- Melnyk, S. A., Davis, E. W., Spekman, R. E., & Sandor, J. (2010). Outcome-driven supply chains. MIT Sloan Management Review, 51, 32–38.
- Melnyk, S. A., Schoenherr, T., Speier-Pero, C., Peters, C., Chang, J. F., & Friday, D. (2022). New challenges in supply chain management: Cybersecurity across the supply chain. International Journal of Production Research, 60(1), 162–183. https://doi.org/10.1080/00207543.2021.1984606
- Menezes, B. C., Kelly, J. D., & Leal, A. G. (2019). Identification and design of industry 4.0 opportunities in manufacturing: Examples from mature industries to laboratory level systems. IFAC-Papersonline, 52(13), 2494–2500. https://doi.org/10.1016/j.ifacol.2019.11.581
- Miceli, A., Hagen, B., Riccardi, M. P., Sotti, F., & Settembre-Blundo, D. (2021). Thriving, not just surviving in changing times: How sustainability, agility and digitalization intertwine with organizational resilience. Sustainability, 13(4), 2052. https://doi.org/10.3390/su13042052
- Min, H. (2019). Blockchain technology for enhancing supply chain resilience. Business Horizons, 62(1), 35–45. https://doi.org/10.1016/j.bushor.2018.08.012
- Minculete, G., Stan, S. E., Ispas, L., Virca, I., Stanciu, L., Milandru, M., Mănescu, G., & Bădilă, M. I. (2022). Relational approaches related to digital supply chain management consolidation. Sustainability, 14(17), 10727. https://doi.org/10.3390/su141710727
- Mithani, M. A. (2020). Adaptation in the face of the new normal. Academy of Management Perspectives, 34(4), 508–530. https://doi.org/10.5465/amp.2019.0054
- Mukherjee, A. A., Singh, R. K., Mishra, R., & Bag, S. (2022). Application of blockchain technology for sustainability development in agricultural supply chain: Justification framework. Operations Management Research, 15(1–2), 46–61. https://doi.org/10.1007/s12063-021-00180-5
- Negri, M., Cagno, E., Colicchia, C., & Sarkis, J. (2021). Integrating sustainability and resilience in the supply chain: A systematic literature review and a research agenda. Business Strategy and the Environment, 30(7), 2858–2886. https://doi.org/10.1002/bse.2776
- Peck, H. (2006). Reconciling supply chain vulnerability, risk and supply chain management. International Journal of Logistics: Research & Applications, 9(2), 127–142. https://doi.org/10.1080/13675560600673578
- Pereira, J. V. (2009). SD‐DES model: A new approach for implementing an e‐supply chain. Journal of Modelling in Management, 4(2), 134–148. https://doi.org/10.1108/17465660910973952
- Pereira, C. R., Christopher, M., & Da Silva, A. L. (2014). Achieving supply chain resilience: The role of procurement. Supply Chain Management: An International Journal.
- Pérez, L., Rodríguez-Jiménez, S., Rodríguez, N., Usamentiaga, R., & García, D. F. (2020). Digital twin and virtual reality based methodology for multi-robot manufacturing cell commissioning. Applied Sciences, 10(10), 3633. https://doi.org/10.3390/app10103633
- Pettit, T. J., Croxton, K. L., & Fiksel, J. (2013). Ensuring supply chain resilience: Development and implementation of an assessment tool. Journal of Business Logistics, 34(1), 46–76. https://doi.org/10.1111/jbl.12009
- Pettit, T. J., Fiksel, J., & Croxton, K. L. (2010). Ensuring supply chain resilience: Development of a conceptual framework. Journal of Business Logistics, 31(1), 1–21. https://doi.org/10.1002/j.2158-1592.2010.tb00125.x
- Ponis, S. T., & Koronis, E. (2012). Supply chain resilience? Definition of concept and its formative elements. The Journal of Applied Business Research, 28(5), 921–935. https://doi.org/10.19030/jabr.v28i5.7234
- Ponomarov, S. Y., & Holcomb, M. C. (2009). Understanding the concept of supply chain resilience. The International Journal of Logistics Management, 20(1), 124–143. https://doi.org/10.1108/09574090910954873
- Radanliev, P., & De Roure, D. (2022). Advancing the cybersecurity of the healthcare system with self-optimising and self-adaptive artificial intelligence (part 2). Health Technology, 12(4), 923–929. https://doi.org/10.1007/s12553-022-00691-6
- Radanliev, P., & De Roure, D. (2023). New and emerging forms of data and technologies: Literature and bibliometric review. Multimedia Tools and Applications, 82(3), 2887–2911. https://doi.org/10.1007/s11042-022-13451-5
- Radanliev, P., De Roure, D., Nicolescu, R., Burnap, P., & Chai, K. H. (2021). Artificial intelligence and the internet of things in industry 4.0. CCF Transactions on Pervasive Computing and Interaction, 3(4), 329–338. https://doi.org/10.1007/s42486-021-00057-3
- Radanliev, P., De Roure, D., Nicolescu, R., Burnap, P., & Chai, K. H. (2022). Digital twins: Artificial intelligence and the IoT cyber-physical systems in industry 4.0. International Journal of Intelligent Robotics and Applications, 6(2), 171–185. https://doi.org/10.1007/s41315-021-00180-5
- Rahman, T., Paul, S. K., Shukla, N., Agarwal, R., & Taghikhah, F. (2022). Supply chain resilience initiatives and strategies: A systematic review. Computers & Industrial Engineering, 170, 108317. https://doi.org/10.1016/j.cie.2022.108317
- Ribeiro, J. P., & Barbosa-Povoa, A. (2018). Supply chain resilience: Definitions and quantitative modelling approaches–A literature review. Computers & Industrial Engineering, 115, 109–122. https://doi.org/10.1016/j.cie.2017.11.006
- Saengchai, S., & Jermsittiparsert, K. (2019). Coping strategy to counter the challenges towards implementation of industry 4.0 in Thailand: Role of supply chain agility and resilience. International Journal of Supply Chain Management, 8(5), 733–744.
- Sahu, A. K., Raut, R. D., Gedam, V. V., Cheikhrouhou, N., & Sahu, A. K. (2022). Lean–agile–resilience–green practices adoption challenges in sustainable agri‐food supply chains. Business Strategy and the Environment. https://doi.org/10.1002/bse.3299
- Sarathy, R. (2006). Security and the global supply chain. Transportation Journal, 45(4), 28–51. https://doi.org/10.2307/20713653
- Sawik, T. (2013). Selection of resilient supply portfolio under disruption risks. Omega, 41(2), 259–269. https://doi.org/10.1016/j.omega.2012.05.003
- Sawyerr, E., & Harrison, C. (2020). Developing resilient supply chains: Lessons from high-reliability organisations. Supply Chain Management: An International Journal, 25(1), 77–100. https://doi.org/10.1108/SCM-09-2018-0329
- Schmitt, A. J., & Singh, M. (2012). A quantitative analysis of disruption risk in a multi-echelon supply chain. International Journal of Production Economics, 139(1), 22–32. https://doi.org/10.1016/j.ijpe.2012.01.004
- Scholten, K., & Schilder, S. (2015). The role of collaboration in supply chain resilience. Supply Chain Management: An International Journal, 20(4), 471–484. https://doi.org/10.1108/SCM-11-2014-0386
- Scholten, K., Scott, P. S., & Fynes, B. (2014). Mitigation processes–antecedents for building supply chain resilience. Supply Chain Management: An International Journal, 19(2), 211–228. https://doi.org/10.1108/SCM-06-2013-0191
- Sharma, A., Pilli, E. S., Mazumdar, A. P., & Gera, P. (2020). Towards trustworthy internet of things: A survey on trust management applications and schemes. Computer Communication, 160, 475–493. https://doi.org/10.1016/j.comcom.2020.06.030
- Sheffi, Y., & Rice, J. B. (2005). A supply chain view of the resilient enterprise. MIT Sloan Management Review, 47(1), 41.
- Shuai, Y., Wang, X., & Zhao, L. (2011, December). Research on measuring method of supply chain resilience based on biological cell elasticity theory. In 2011 IEEE International Conference on Industrial Engineering and Engineering Management (pp. 264–268). IEEE.
- Silge, J., & Robinson, D. (2016). Tidytext: Text mining and analysis using tidy data principles in R. The Journal of Open Source Software, 1(3), 37. https://doi.org/10.21105/joss.00037
- Silge, J., & Robinson, D. (2017). Text mining with R: A tidy approach. “O’Reilly Media, Inc.”.
- Spiegler, V., Naim, M., & Wikner, J. (2012). A control engineering approach to the assessment of supply chain resilience. International Journal of Production Research, 50(21), 6162–6187. https://doi.org/10.1080/00207543.2012.710764
- Sprecher, B., Daigo, I., Murakami, S., Kleijn, R., Vos, M., & Kramer, G. J. (2015). Framework for resilience in material supply chains, with a case study from the 2010 rare earth crisis. Environmental Science & Technology, 49(11), 6740–6750. https://doi.org/10.1021/acs.est.5b00206
- Stewart, G. T., Kolluru, R., & Smith, M. (2009). Leveraging public‐private partnerships to improve community resilience in times of disaster. International Journal of Physical Distribution & Logistics Management, 39(5), 343–364. https://doi.org/10.1108/09600030910973724
- Tamym, L., Benyoucef, L., Moh, A. N. S., & El Ouadghiri, M. D. (2021). A big data based architecture for collaborative networks: Supply chains mixed-network. Computer Communications, 175, 102–111. https://doi.org/10.1016/j.comcom.2021.05.008
- Thomsett, M. C. (2011). Global supply chain risk management: Viewing the past to manage today’s risks from an historical perspective. Proceedings of Intellectbase International Consortium, 13, 49–55.
- Tortorella, G. L., Fogliatto, F. S., Saurin, T. A., Tonetto, L. M., & McFarlane, D. (2022). Contributions of healthcare 4.0 digital applications to the resilience of healthcare organizations during the COVID-19 outbreak. Technovation, 111, 102379. https://doi.org/10.1016/j.technovation.2021.102379
- Tukamuhabwa, B. R., Stevenson, M., Busby, J., & Zorzini, M. (2015). Supply chain resilience: Definition, review and theoretical foundations for further study. International Journal of Production Research, 53(18), 5592–5623. https://doi.org/10.1080/00207543.2015.1037934
- Urciuoli, L. (2015). Cyber-resilience: A strategic approach for supply chain management. Technology Innovation Management Review, 5(4), 13–18. https://doi.org/10.22215/timreview/886
- Verbano, C., & Venturini, K. (2011). Development paths of risk management: Approaches, methods and fields of application. Journal of Risk Research, 14(5), 519–550. https://doi.org/10.1080/13669877.2010.541562
- Voss, M. D., Whipple, J. M., & Closs, D. J. (2009). The role of strategic security: Internal and external security measures with security performance implications. Transportation Journal, 48(2), 5–23. https://doi.org/10.2307/25702520
- Wickham, H. (2016). Programming with ggplot2. In ggplot2 (pp. 241–253). Springer International Publishing. https://doi.org/10.1007/978-3-319-24277-4_12
- Wieland, A., Wallenburg, C. M., & Töyli Harri Lorentz, and Lauri Ojala, J. (2013). The influence of relational competencies on supply chain resilience: A relational view. International Journal of Physical Distribution & Logistics Management, 43(4), 300–320. https://doi.org/10.1108/IJPDLM-08-2012-0243
- Williams, Z., Lueg, J. E., LeMay, S. A., & Waller, M. (2008). Supply chain security: An overview and research agenda. The International Journal of Logistics Management, 19(2), 254–281. https://doi.org/10.1108/09574090810895988
- Xiao, R., Yu, T., & Gong, X. (2012). Modeling and simulation of ant colony’s labor division with constraints for task allocation of resilient supply chains. International Journal on Artificial Intelligence Tools, 21(3), 1240014. https://doi.org/10.1142/S0218213012400143
- Yang, B., & Yang, Y. (2010). Postponement in supply chain risk management: A complexity perspective. International Journal of Production Research, 48(7), 1901–1912. https://doi.org/10.1080/00207540902791850
- Zeb, A., & Kortelainen, J. (2021). Industrial IoT solutions for digital twins: An overview. Journal of Ambient Intelligence and Humanized Computing, Advance online publication. https://doi.org/10.1007/s12652-021-03486-6
- Zhang, C., Chen, Y., Chen, H., & Chong, D. (2021). Industry 4.0 and its implementation: A review. Information Systems Frontiers, 1–11. https://doi.org/10.1007/s10796-021-10153-5
- Zsidisin, G. A., & Wagner, S. M. (2010). Do perceptions become reality? The moderating role of supply chain resiliency on disruption occurrence. Journal of Business Logistics, 31(2), 1–20. https://doi.org/10.1002/j.2158-1592.2010.tb00140.x