ABSTRACT
Industry 4.0 technologies create a connected ecosystem that allows for real-time monitoring, control, and optimization. This enables the manufacturing industry to move the ultimate goal of their businesses from lean to leagile. Then, to meet the needs of leagile manufacturing, an integrated production planning and scheduling system is needed. The academic world responds to the need by the growth of papers. Many methodologies, models, frameworks, and methods have been proposed. Literature abounds on classifying them, yet it remains inconclusive mainly on their applicability to Industry 4.0. In this regard, this paper conducts a literature review aiming to show the state of the art and discover the most promising production planning and scheduling method for Industry 4.0 among the many. Lastly, on the basis of this study, recommendations on possible future research directions are provided for researchers, and the design of the next-generation production planning and scheduling system for Industry 4.0 is also suggested.
1. Introduction
Production planning and scheduling are among the most important aspects to consider in a factory. Scientific analysis of the manufacturing process first began with Frederick Taylor (Citation1911). Taylor created the specialty of industrial efficiency. He is widely known as the father of scientific management. Karol Adamiecki (Citation1931) and Henry Gantt (Citation1903) were Taylor’s contemporaries. They invented similar forms of graphical analysis based on a calendar, respectively. However, since Gantt published his effort much earlier than Adamiecki, Gantt charts became the primary tool for planning and scheduling. But Gantt charts worked well only in a single factory. Ellis A. Johnson (Citation1960) made a significant breakthrough. He provided Johnson’s rule for scheduling jobs in multiple production facilities. At the same time, operations research (OR) emerged in the military field during World War II. OR is concerned with finding an optimum solution using mathematical and statistical techniques and methods. Most production planning and scheduling systems today are built on OR (Johnson & Montgomery, Citation1974).
Production planning and scheduling herein refer to managerial decisions at tactical (medium-range) and operational (short-term) levels. Sometimes, the tactical planning level is decomposed into two parts: aggregate production planning (APP) and master production scheduling (MPS). Both planning and scheduling are related to arranging resources to meet production goals. They are often used interchangeably, but they actually refer to two separate activities. Production planning concerns using existing resources to meet demand as effectively and profitably as possible. Production capacity can be adjusted within limits by varying one or more of the following: the workforce size, the allowed overtime, the shifts, the production rate, the inventory level, and so on. Scheduling concerns the day-to-day operations within the guidelines established at the planning level. Scheduling involves assigning products to machines, sequencing and routing orders through the plant, determining replenishment quantities for each stock-keeping unit, and so on (Silver et al., Citation1998). Real-time scheduling is equivalent to control (Dong et al., Citation2016; Gyulai et al., Citation2017; Kan & Shen, Citation2020; Monostori et al., Citation2010; Santander et al., Citation2020).
Computerized production planning and scheduling systems became popular in the third industrial revolution (Industry 3.0) when robotics highly performed human tasks. Material requirements planning (MRP) was the earliest production planning and scheduling system. The primary function of an MRP is to ensure that materials are available when they are needed. In the 1980s, MRP evolved into MRP II by adding additional financial and employee needs data. Enterprise resource planning (ERP) originated with MRP II. ERP introduced full integration of manufacturing processes and the company as a whole. The first ERP system was released in the 1990s. However, the existing ERP systems are still weak regarding planning and scheduling as they are optimized for transaction processing, not large-scale, high-volume data analytics. Thus, complementing them with advanced planning and scheduling (APS) systems has become a trend. APS is the core of smart manufacturing.
Entering the Industry 4.0 era, the need for a bespoke APS is more pressing. While Industry 3.0 primarily focuses on physical systems, Industry 4.0 focuses heavily on cyber-physical systems (CPS), i.e. real and virtual exist simultaneously (Audaces, Citation2021). The critical factors of a CPS are the integration of virtual and physical systems and (or more precisely) the application of connectivity and fast computation among them.
Industry 4.0 technologies provide an incredible opportunity for the manufacturing industry to enter the stage of mass personalization at scale. Mass customization, a perfect combination of mass production and customization, is an attractive business model for those who want to maintain customer satisfaction and build a competitive advantage. Mass customization requires a leagile supply chain (Zhang & Qi, Citation2013). Leagile herein is a hybrid term for leanness and agility. Because the degree of leagility depends on upstream and downstream integration, production planning and scheduling must be integrated to support fast and smooth data exchange.
However, due to their different objectives and time scales, effective planning and scheduling integration has proven challenging (Kan & Shen, Citation2020). There are mainly two kinds of integration strategies: hierarchical and monolithic (Vogel et al., Citation2017). The hierarchical method involves a multi-level decision process. Though the implementation is more manageable, the hierarchical method cannot guarantee optimal solutions because decisions at the planning stage are made based on a rough approximation of the actual data. In an uncertain environment, the randomness of production makes it hard to determine production capacity, which hinders production planning. On the contrary, the monolithic method could, in principle, provide optimal solutions. The higher-level planning problem considers lower-level implicit scheduling reactions in advance. Still, the high computational cost arising from the degree of complexity prohibits their practical implementation. For many years, the academic world has continuously tried to address the coherence issue between planning and scheduling levels. A compromise between modeling accuracy and computational tractability is widely accepted, which overlaps the planning and detailed scheduling phases to some extent by hybridizing the two methods within a rolling horizon framework.
The current literature abounds with classification schemes for production planning, scheduling, and their combinations. For example, Mula et al. (Citation2006) classified general types of models in production systems into conceptual models, analytical models, artificial intelligence (AI) based models, and simulation models. Maravelias and Sung (Citation2009) grouped the modeling approaches into detailed scheduling models, relaxed and aggregated scheduling formulations, off-line surrogate models, and hybrid modeling for rolling horizon approaches. Meanwhile, they grouped the solution strategies into hierarchical, iterative, and full-space methods. Jamalnia et al. (Citation2019) classified the APP models into stochastic mathematical programming, fuzzy mathematical programming, simulation, metaheuristics, and evidential reasoning. Recently, Guzman et al. (Citation2022) proposed a holistic framework that summarized the most critical aspects characterizing production planning, scheduling, and sequencing problems from various perspectives, such as decision level, plan aggregation, planning horizon, modeling approach, mathematical model objectives, solution approach, development tool, proposed solution, application area, actual case application, data set size, and solution quality.
Yet, studies discussing the models and methods’ applicability to Industry 4.0 are relatively few and remain largely inconclusive. The gap has recently attracted increasing research attention. Herrmann et al. (Citation2022) reviewed and classified the publications according to the Aachen production planning and control (PPC) model’s tasks and functions. They proposed a CPS PPC architecture for realizing a PPC system in a smart factory. The Aachen PPC model is a widespread concept in German-speaking countries. Details of the Aachen PPC model can be found in Schuh and Stich (Citation2012). Additionally, Krishnan et al. (Citation2022) analyzed the current state, challenges, and future prospects of aggregate production planning and scheduling in light of emerging technologies including AI, machine learning (ML), and CPS. Luo et al. (Citation2022) proposed a digital twin (DT) framework that integrates production planning systems and frontier technologies such as the internet of things (IoT), cloud manufacturing, blockchain, and big data analytics.
This paper differs from the above reviews. The authors focus on the integration of production planning and scheduling toward the leagile business goal in the era of Industry 4.0. The focus helps to narrow down the scope of this study to a reasonable extent in light of the huge amount of literature. The following research questions are raised in this paper:
RQ1.
What is the state-of-the-art in integrated production planning and scheduling?
RQ2.
What are the trends in production planning and scheduling in light of the emerging frontier technologies?
RQ3.
How would the production planning and scheduling system be like in the Industry 4.0 context?
In order to answer the above research questions, this paper conducts a comprehensive literature review of contemporary literature. Section 2 describes the review methodology. After that, Section 3 talks about the state-of-the-art in integrated production planning and scheduling. And Section 4 identifies the impacts of Industry 4.0 technologies on production environments and discovers the trends in production planning and scheduling in light of the emerging frontier technologies. Next, Section 5 gives the design of the next generation production planning and scheduling system for Industry 4.0. Finally, Section 6 concludes the paper and gives the future research direction.
2. Literature review methodology
The search and review were synthesized by dividing them into two categories: methods (integrated production planning and scheduling under uncertainty) and implementation (production planning and scheduling in Industry 4.0). The synthesis was conducted to provide a systematic way to learn about the state of the art and future trends. The search for the literature was conducted in the Scopus database in September 2022 using the search strings depicted in .
Table 1. The keywords used in this research.
shows the flowchart for the literature review processes during the two searches. The initial search returned 1063 articles after removing duplicates. Since we only considered English journal articles between 2002 and 2022, 543 articles remained. By screening the titles of these articles, 11 off-subject articles were excluded. Additional 8 articles were discarded due to lack of citations or access to full texts. Then, the full texts of the remaining 527 articles were evaluated for eligibility according to their relevance to integrated production planning and scheduling. The majority focus on combining adjacent decisions in operations, such as integrated production and distribution planning, integrated production and maintenance planning, integrated production planning and quality control, and so on. They are not within the scope of this work. Finally, we selected 58 articles for detailed analysis. International Journal of Production Research, Computers and Chemical Engineering, and Computers and Industrial Engineering are the top three journals.
In addition, considering production planning and scheduling is a classical problem, the traditional books on this topic are also included in the review to assist in answering RQ1 - What is the state-of-the-art in integrated production planning and scheduling.
3. The state-of-the-art on integrated production planning and scheduling
3.1. Overview of the selected articles about production planning and scheduling integration
A total of 33 selected papers were reviewed in detail. According to their modeling approaches and solution strategies, these papers are classified in .
Table 2. Summary of papers about production planning and scheduling integration.
The majority of the papers consider uncertainties in their models. They either proactively enhance the performance of the plan by anticipating a certain degree of the occurrence of uncertain events at the planning level or reactively respond to the occurrence of random events at the scheduling level. The first approach results in excessive capacity, while the second approach incurs frequent adjustments. Meanwhile, the modeling methods for uncertainty are many. They can be broadly categorized into discrete methods and continuous methods. Discrete methods assume discrete events for which a scenario tree is created. The first stage in the tree models the present, without considering any uncertainty. It gives a baseline plan. The following stages are associated with a series of stage-related decision variables used to correct the baseline plan after the occurrence of uncertain events. Such a multi-stage optimization model is usually solved using dynamic programming (DP) in papers. On the contrary, continuous methods use probability distributions with mean and standard deviation parameters for uncertain factors. Expected values then replace the parameters and variables in the models. Consequently, the models become the same as the deterministic models. We see in these papers that normal distributions are widely assumed even though most data are not normally distributed.
Almost half of the papers use simulation methods. The effect of uncertainties can be quantified in simulations. The simulation methods used in these papers are heterogeneous. The discrete event simulator (DES) performs the planning and scheduling process. The agent-based simulator (ABS) emulates the behaviors of human workers. The system dynamics (SD) model simulates interactions between order costs and finished order prices. The process simulators estimate the machine processing times for a process plan given a set of machining parameters. High-fidelity simulation is made possible by feeding DT data to the simulators.
3.2. Review of the production decision-making framework
Silver et al. (Citation1998) present a single general framework for production planning and scheduling, as illustrated in . The framework embraces decision hierarchy as well as system integration. The bidirectional arrows indicate the mutual impact as the information flow goes both ways.
Figure 2. A production decision-making framework (Silver et al., Citation1998).
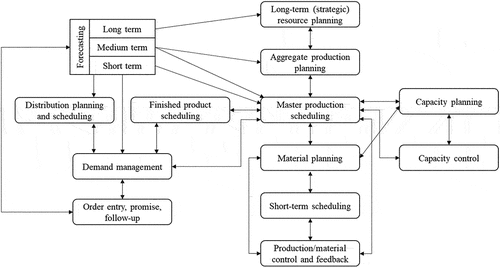
The backbone of the entire framework is MPS, which acts as the primary link between the marketing and production departments. It breaks down the APP into a production schedule that specifies which products should be manufactured at particular times in each production plant. MRP then takes the MPS and breaks it down into detailed production or procurement schedules for all components and raw materials. Based on the MPS and MRP plan, the next step is short-term scheduling, which determines the order in which tasks are performed on each machine on the shop floor. Finished product scheduling is relevant when MPS is not done at the finished product stage. This type of scheduling is initiated by customer requests (pull), while MPS relies on forecasts (push).
Both the MPS module and the MRP module are supported by the capacity planning module. At the MPS decision level, a rough capacity check is performed to ensure the master schedule is feasible. At the MRP decision level, a more detailed check is conducted to ensure the accuracy of the plan. If the capacity planning module detects any significant capacity issues, the information will be communicated to the higher-level APP.
3.3. Review of the approaches for coping with the hierarchy
There are two main approaches for managing the hierarchy (strategic, tactical, and operational) of managerial decision-making: hierarchical production planning (HPP) and monolithic production planning (MPP). Silver et al. (Citation1998) identified three methods: explicit HPP, implicit HPP, and MPP. Implicit HPP addresses capacity constraints on a trial-and-error basis without offering much prescriptive advice.
3.3.1. Hierarchical production planning
The first HPP model was presented by Hax and Meal (Citation1973) who considered product type, family, and item. Typically, HPP is modeled as a two-level (aggregation and disaggregation) top-down hierarchy. Each level is solved separately. Optimal decisions at the upper level provide constraints for the lower level. In turn, the lower level provides feedback to evaluate the quality of the upper level solutions. At the aggregation level, three types of aggregations are performed: upgrading the perspective from individual level to family level, machine level to shop floor level, and short time period to long time horizon. A knapsack method could be applied to disaggregation. The knapsack problem is a combinatorial optimization that determines the number of items, each with a weight and a value, to include in a collection so that the total value is the largest within the weight capacity. The disaggregation process is done period by period (Ghazanfari & Murtagh, Citation2002), moving the perspective from a long-term planning horizon to a short-term scheduling period.
In HPP systems, the parameters at the upper level are mostly uncertain because they are predicted. On the other hand, parameters at the lower level are mostly deterministic as there is enough data available to determine them, except for market demands and production capacities which still remain ambiguous. However, it has been observed in the literature, the upper level models are often deterministic, which assume perfect forecast information, leaving the uncertainties to be tackled at the lower level. Even though this approach reduces computational complexity, the decisions made at the higher level are imposed on the lower level as hard constraints, which may cause coherence issues.
Planning in a manner that can address uncertainty during the planning stage is a crucial requirement for leagile. A plan that is robust can be efficient, while the opposite is not true. Torabi et al. (Citation2010) developed a formulation of the HPP using fuzzy linear programming (LP), which facilitates making decisions at the lower level while allowing for minor deviations from the outputs of the upper level.
The two levels of the HPP can refer to different time periods, such as long-term and short-term; or different levels of product development, such as product types and families (Ghazanfari & Murtagh, Citation2002), product families and end products (Torabi et al., Citation2010), semi-finished products and finished products (Aghezzaf et al., Citation2011). They can also represent different facility levels, such as enterprise level and shop floor level (Venkateswaran & Son, Citation2005); or different business operation steps, such as order acceptance and order production (Rafiei et al., Citation2013).
The HPP model has the potential to be used in different manufacturing scenarios. For example, it can be applied to solve a multi-objective production planning with stochastic demand (Ghazanfari & Murtagh, Citation2002), a multi-product and multi-facility manufacturing enterprise (Venkateswaran & Son, Citation2005), a capacity constrained, multi-product, multi-period manufacturing system (Torabi et al., Citation2010), a capacitated two-level production system (Aghezzaf et al., Citation2011), or a hybrid make-to-stock (MTS) and make-to-order (MTO) production (Rafiei et al., Citation2013). As HPP provides a useful framework for the establishment of strategic and tactical level objectives and constraints, it can act as a guide at the operational level for an organization, even for small- and medium-sized enterprises (SMEs) (O’Reilly et al., Citation2015).
3.3.2. Monolithic production planning
The use of a single simultaneous planning and scheduling model can enhance the quality of decision solutions. While building a full-space optimization model is the easiest way to construct an MPP, it can be challenging to solve due to the large size of the detailed scheduling model. Therefore, breaking down a large-scale production planning problem into smaller, more manageable parts is a common method to address the issue of model simplification.
One way is to decompose the entire time horizon into equivalent time periods (Aguirre & Papageorgiou, Citation2018; Han et al., Citation2020; Monostori et al., Citation2010; Shah & Ierapetritou, Citation2012; Susara et al., Citation2003; Wen et al., Citation2017; Yan et al., Citation2015; Zhang et al., Citation2019; Zhi-Min et al., Citation2021; Zukui & Ierapetritou, Citation2009, Citation2010). Each planning period consists of one or more scheduling periods. Then, during each planning time period, the problem becomes a bilevel optimization problem. The resulting model is relatively small-scale, making it more tractable. It may be possible to establish a fixed pattern and repeat it.
Another way is to decompose the entire time horizon into multiple stages with varying durations, with more details in the immediate future than in the distant future (Alfieri et al., Citation2012; Dan & Ierapetritou, Citation2007; Gyulai et al., Citation2017; Jiang & Yan, Citation2022; Luo & Rong, Citation2009; Zanjani et al., Citation2010). The first stage represents the current period, where parameters are considered deterministic and has the smallest duration. The latter stages have increasing durations and reflect the rising uncertainties for the far future. As a result, only a few early periods use the detailed scheduling model. After that, the relaxation/aggregation or surrogate models are used for the remaining periods. This approach reduces the problem size and complexity. A surrogate model is trained using a data-driven approach, which makes it more accurate and computationally efficient (Dias & Ierapetritou, Citation2020). In the literature, some common surrogate models include linear regression, artificial neural networks (ANN), polynomial regression surfaces, kriging, radial basis functions, and support vector machines. For a comprehensive review of the advances in surrogate-based modeling, feasibility analysis, and optimization, one can refer to Bhosekar and Ierapetritou (Citation2018).
An iterative framework has been developed for the entire time horizon. It is important to note that each subproblem along the time horizon should not be solved independently. Instead, a looking forward strategy is highly effective in ensuring feasible solutions in subsequent time periods. If the setup costs are significant, the looking forward strategy can also benefit the low-cost business goal by producing extra products in certain time periods to cover the needs in the subsequent periods. As such, no production is needed in some time periods. One can join the subproblems by either alternatively running the planning model and the scheduling model, like Yan et al. (Citation2015) did, or using a rolling-horizon approach that incorporates additional constraints from proximately following periods in the periodical problem solving. The latter is more popular. Glomb et al. (Citation2022) investigated the several drawbacks of the classical rolling-horizon approach and developed an algorithm that compensates for these drawbacks both theoretically and practically.
3.4. Review of the solution strategies
Developing efficient solutions is important. Particularly, the production scheduling problem is an NP-hard problem when the number of machines is greater than three (Lenstra & Rinnooym Kan, Citation1979). Even for the two-machine case, where each job has a maximum of three operations, it is already an NP-hard problem. NP-hard problems cannot be solved in polynomial time. Therefore, the original large-scale problem has to be relaxed and decomposed to reduce computational complexity.
In practice, most mixed integer linear programming (MILP) models are solved by linear relaxation techniques (Dan & Ierapetritou, Citation2007). The branch-and-bound algorithm, combined with real-valued algorithms and other strategies, such as cutting planes, pricing, and custom heuristics, is an effective one (Monostori et al., Citation2010; Susara et al., Citation2003).
Lagrangian relaxation has proven to be a powerful means of solving large MIP, including mixed integer nonlinear programming (MINLP) (Shah & Ierapetritou, Citation2012; Zukui & Ierapetritou, Citation2009, Citation2010). To overcome the duality gap in classical Lagrangian relaxation, Zukui and Ierapetritou (Citation2010) developed an augmented one where they introduced a positive penalty parameter. They have the optimum of the dual problem equal to the optimum of the primal problem, even if the primal problem is non-convex.
Another popular way to solve the full-space model is to use meta-heuristics, such as genetic algorithms (GA), simulated annealing (SA), and particle swarm optimization (PSO) (Han et al., Citation2020; Jiang & Yan, Citation2022; Rafiei et al., Citation2013; Wen et al., Citation2017; Yan et al., Citation2015, Citation2015; Zhang et al., Citation2019; Zhi-Min et al., Citation2021). These methods employ randomized search techniques to select candidates using some kind of randomness or probability. They iteratively move toward better solutions in the search space. Random search techniques are relatively easy to implement on large-scale and complex problems with ‘black-box’ function evaluations. However, their drawback is that there is no one-size-fits-all algorithm, and they must be customized to each specific problem through trial-and-error. There is also no guarantee of reaching the optimal solution.
Real-world problems are very complex. Most optimization algorithms tend to focus on tackling NP-hard yet idealized problems. These algorithms are not practicable for real-world problems, which are difficult to represent in a rigorous mathematical model. In this regard, other approaches have been developed, such as simulation-based optimization methods (Ghazanfari & Murtagh, Citation2002; Gyulai et al., Citation2017; Kang & Choi, Citation2010; Luo & Rong, Citation2009; Monostori et al., Citation2010; Venkateswaran & Son, Citation2005). Simulation is a primary tool for modeling complex systems that are affected by uncertainty. It helps decision-makers by providing various scenarios that can be tested to examine the impact of alternative plans. However, simulation alone cannot identify and suggest optimal solutions. Therefore, it is often combined with optimization techniques such as exact, heuristic, and metaheuristic methods. For a comprehensive review of contemporary simulation optimization methods, we recommend referring to the paper by Jay et al. (Citation2003).
4. Production planning and scheduling in the Industry 4.0 context
4.1. Overview of the selected articles about production planning and scheduling in the Industry 4.0 context
The term Industry 4.0 was first introduced at Germany’s Hannover Messe, the country’s most important industrial fair, in 2011. Smart factories and online production management systems began emerging in 2014. The 25 selected papers, published between 2013 and 2023, were reviewed based on their focused problems impacting frontier technologies. provides a summary of these papers.
Table 3. Summary of papers about production planning and scheduling in the industry 4.0 context.
The papers include real-life case studies and survey/literature reviews. In the former category, Zangiacomi et al. (Citation2017) gave a case study for implementing manufacturing apps. Tan et al. (Citation2019) gave a case study for CPS-based smart industrial robot production. Mohamad et al. (Citation2022) studied the impact of the Industry 4.0 revolution in the textile industry in the Association of Southeast Asian Nations (ASEAN) countries. In the latter category, Khakifirooz et al. (Citation2019) reviewed the success story of smart manufacturing in the semiconductor industry in the literature. Lee (Citation2021) conducted an empirical survey of 222 hands-on workers who operate smart factories in small and medium-sized Korean firms. Rajesh et al. (Citation2022) conducted a literature review to identify and rank advanced Industry 4.0 technologies, operational excellence strategies, and reconfigurable manufacturing system practices for improving the performance of manufacturing organizations. Sharma et al. (Citation2023) carried out a survey to identify critical barriers and suitable solution initiatives for the adoption of Industry 4.0 technologies in sustainable supply chain management.
The rest attempt to propose various conceptual frameworks. For example, Giordani et al. (Citation2013), Kumar et al. (Citation2020), and D’Aniello et al. (Citation2021) developed multi-agent systems (MAS) frameworks for integrated yet distributed operations planning. Zhong et al (Citation2013, Citation2015) and Yaqiong and Lin (Citation2017) developed radio frequency identification (RFID) system frameworks for real-time operation planning. Bao et al. (Citation2019) and Park et al. (Citation2020) developed DT frameworks in the context of manufacturing. Jun et al. (Citation2017) and Cheng et al. (Citation2020) developed cloud system frameworks for distributed and collaborative manufacturing operations. Chunyang et al. (Citation2020) developed a blockchain system framework for shared manufacturing. Farooqui et al. (Citation2020) developed an event driven architecture (EDA) framework for data collection from the factory floor. Holmstrom et al. (Citation2017) developed a roadmap for developing and implementing direct digital manufacturing (DDM)-based operational practices. Moreover, Mourtzis et al. (Citation2015), Legat and Vogel-Heuser (Citation2017), and Daqiang et al. (Citation2021) developed AI tools for planning, scheduling, execution, and control.
4.2. The impact of Industry 4.0 on production environments
The use of Industry 4.0 technologies has brought about significant changes in the management of production operations (Daqiang et al., Citation2021). These cutting-edge technologies have made it easier to manage operations efficiently, increase productivity, enhance communication and collaboration, and reduce wastage through lean practices (Mohamad et al., Citation2022; Pansare & Yadav, Citation2022, Citation2022; Sharma et al., Citation2023). The primary Industry 4.0 technologies comprise CPS, DT, RFID, cloud technology, collaborative robot technology, DDM, EDA, and mobile apps. Together, they create an advanced production environment.
4.2.1. Digital twin
DT technology is a key part of CPS. It has gained a lot of attention as a new generation technology for modeling, simulation, and optimization. It not only provides a simulation platform before production but also works as a real-time control platform during production. DT can support advanced control and decision making with less gap through horizontal and vertical integrations (Park et al., Citation2020).
4.2.2. Radio frequency identification
RFID, based on IoT technologies, assigns a unique digital identifier to individual items, connecting the physical and digital worlds. The DTs powered by RFID allows for real-time tracking and tracing down to the item level, making it possible to capture changes and disturbances at they occur (Yaqiong & Lin, Citation2017; Zhong et al., Citation2013, Citation2015).
4.2.3. Cloud technology
Cloud technology enables businesses of all sizes to quickly adapt to Industry 4.0 by providing scalable storage space and computing resources. Among the various cloud services, software as a service (SaaS) offers standardized applications on line, making it a good option for SMEs without IT infrastructure (Jun et al., Citation2017). Cloud technology plays a very important role in the resource sharing system (Chunyang et al., Citation2020). It breaks the traditional habits of producing only within an enterprise, and supports distributed collaborations among lots of networked enterprises in the ecosystem (Cheng et al., Citation2020). The trend of resource sharing promotes the upgrade from the traditional production-oriented manufacturing strategy to a service-oriented one. Rather than relying on inventories to satisfy demand, the new strategy suggests a reliance on the production capacity available to meet demand.
4.2.4. Robot technology
The use of industrial robots is rapidly increasing in almost all manufacturing industries. Collaborative robots contribute to a more dynamic facility layout, which results in a less constrained facility where design decisions are postponed to the operational level and become reversible options (Giordani et al., Citation2013). Traditional production planning that assumes a permanent facility layout becomes unviable in this case. It requires the definition and solution of a complex planning and scheduling problem for this particularly flexible layout.
4.2.5. Direct digital manufacturing
DDM is a process that uses technologies such as 3D printing and additive manufacturing to convert digital models into physical objects without the need for tooling. It is particularly advantageous for small batch production or mass customization. DDM offers advantages such as production efficiency and cost reduction. However, if not planned and scheduled properly in a factory setting, these benefits may quickly disappear (Holmstrom et al., Citation2017).
4.2.6. Event driven architecture
Handling the vast amounts of data generated by distributed and asynchronous systems is beyond the capabilities of traditional data architectures. To address this issue, an EDA software design pattern has been developed. This architecture promotes the development of systems as a series of loosely coupled events, which has become increasingly popular in recent years due to its scalability and flexibility. The EDA can be applied to both legacy and modern systems to collect data from the shop floor (Farooqui et al., Citation2020).
4.2.7. Mobile apps
Mobile devices serve as a bridge between workers and technology. With the help of mobile apps, connecting and communicating with AI or IoT becomes effortless, leading to an agile, productive, and collaborative environment between humans and machines. The design of mobile apps is highly customizable to meet company-specific needs. During different planning activities, mobile apps can aid in the dissemination of information and improve information management for the various decision-makers involved (Zangiacomi et al., Citation2017).
4.3. New trends in production planning and scheduling
Industry 4.0 paves the way for a strategy of mass customization. Companies, especially SMEs, should focus on establishing production planning and scheduling systems to raise innovative performance (Lee, Citation2021). In today’s business environment, which is characterized by globalization, mass customization, and high demand volatility, uncertainties and complexity, planning and scheduling have become increasingly difficult and challenging (Mourtzis et al., Citation2015).
A practical production planning and scheduling method should fulfil the following four requirements proposed by Kang and Choi (Citation2010): generality, solution quality, computation efficiency, and implementation ease. Unfortunately, most of the production planning and scheduling methods developed in the literature are rarely used by the industry to solve real-world problems. This is mainly due to two drawbacks: (a) the absence of real-time interaction and close-loop feedback mechanisms between physical and virtual objects, and (b) the lack of a platform in the dynamic discrete manufacturing environment for data transmission and sharing (Bao et al., Citation2019).
Simulation is a promising technique for handling complex real-world production planning and scheduling. With the advent of Industry 4.0, there are new opportunities to develop high-fidelity simulations using DTs. However, this also brings new challenges to the field of simulation, especially due to the growing complexity of the systems that need to be modeled. The main simulation approaches in Industry 4.0 are agent-based (Paula Ferreira et al., Citation2021). A multi-agent system (MAS) is a distributed computer system containing multiple intelligent agents that communicate and cooperate with each other in the problem-solving process. Each intelligent agent has its own data and knowledge and can make its own decisions. The bottom-up structure allocates computational resources and capabilities among the agents and does not suffer from the problems caused by the computational and communication bottlenecks and the vulnerability of system failure that are associated with centralized systems (Giordani et al., Citation2013). There is an increasing interest in addressing the distributed scheduling problem using MAS (D’Aniello et al., Citation2021; Giordani et al., Citation2013; Tan et al., Citation2019).
The previous MRP system, which was developed in the 1960s before the widespread use of computers, and its successor MRPII, which was introduced in the 1980s, have become somewhat outdated. This is because some of the assumptions they were based on no longer apply in the current era. Additionally, commercially available ERP or MES planning systems and various simulation tools provide centralized approaches for decision-making that are structurally inflexible, and hence not very suitable for leagile manufacturing (Kumar et al., Citation2020). Furthermore, the signal-oriented languages used in classical programming approaches for field automation software require disproportionate effort to ensure the necessary flexibility (Legat & Vogel-Heuser, Citation2017).
Advanced planning and scheduling (APS) emerges to explicitly support the on-going, dynamic nature of planning. Rather than dealing with small parts of the problem separately as in the traditional production planning process, APS addresses the whole planning problem directly, enabled by modern computing technology. The current commercial APS software is based on the HPP model, which consists of two separate software components: advanced planning software and advanced scheduling software. It needs to work with ERP systems. The APS software is basically a highly customized system in a different production context.
5. The proposed integrated production planning and scheduling system for Industry 4.0
The shift towards Industry 4.0 poses challenges to the centralized and hierarchical decision process. A smart factory featuring autonomous robots and real-time DTs needs a more flexible and robust planning and scheduling system, which should be based on data-driven intelligence. From the literature review, we see the advantages and disadvantages of different methodologies, models, frameworks, and methods. We thereby propose the framework of an integrated production planning and scheduling system for Industry 4.0, as shown in .
The framework focuses on production planning and scheduling at the tactical and operational levels. Many previous studies have given similar frameworks; one example is illustrated in . But few have suggested communication links between the APS and existing manufacturing software such as ERP, MES, and MRP. Therefore, the main novelty of our proposed framework may be the clear guidance for data and information flow as well as the detailed methods (simulation-based optimization, matheuristics optimization, and so on), which can offer a conceptual model for further software system development.
In , the left-hand side is a planning system, and the right-hand side is a scheduling system. The left-hand and the right-hand construct a closed-loop system. Planning decisions are made based on aggregate forecasts that are estimated from scheduling results, while scheduling decisions are made based on real shop floor data that is reflected in DT. The left-hand side is pushed by inventory level, hence lean-oriented, while the left-hand side is pulled by internal company orders, hence agile-oriented. The left-hand side and the right-hand side are decoupled by setting buffers in the orders.
Overall, the integrated production planning and scheduling system is a hybrid HPP and MPP system within a rolling horizon framework that is applicable to dynamic bottom-up decision-making environments. On the one hand, the left-hand side planning system links with the right-hand side scheduling system, constructing a two-level HPP. On the other hand, the left-hand side itself is an MPP, which consists of a planning engine and a surrogate scheduling engine. As explained earlier, the classical HPP system is easier to solve yet prone to inconsistency issues in uncertain dynamic systems, while the pure MPP system gives planning and scheduling solutions at once but suffers from high computational costs and poor scalability. The purpose of using hybridization is to make use of the benefits of both HPP and MPP while avoiding their drawbacks.
To save computational cost without sacrificing solution quality, we need to make solutions robust and keep calculations fast. To realize the first aim, we design a scheduling model in the planning system, which is a surrogate model, to assist the planning practice with detailed scheduling data. The surrogate model is a simplified approximation of the more complex model, thus making it more amenable for large-scale comparative analysis, achieving the second aim. Furthermore, we feed the surrogate model with the execution results from the scheduling system to keep it updated. We suggest using matheuristics (a hybrid of mathematical modeling and heuristics) to solve the left-hand side model, which meets fuzzy data in the forecast of the future, and simulation-based optimization to solve the right-hand side model, which encounters dynamics and uncertainty on the shop floor.
For real-time scheduling, a mix of domain expertise and ML is proposed. ML has several drawbacks, like requiring large amounts of training data and time. Considering an APS is bespoke and a factory may not run long enough to accumulate enough training data, the ML results may be biased. In the past, researchers have made great efforts to improve the prediction performance of ML models. For example, researchers increased the number of hidden layers in an ANN to improve its accuracy. However, as the model becomes ‘deeper’ and ‘darker’, it becomes even more difficult for practitioners to trust the model’s recommendations. Integrating domain knowledge into the ML models improves the model’s interpretability. And interpretability is a useful debugging tool for detecting bias in ML models.
6. Conclusions and future research directions
In view of the impact of frontier technologies on production environments, this paper makes an effort to review and analyze the state-of-the-art research on relevant domains with the aim of identifying the most promising production planning and scheduling methods for Industry 4.0. We conducted parallel searches in two domains: methods (integrated production planning and scheduling under uncertainty) and implementation (production planning and scheduling in Industry 4.0). Through a structural filtering process, we selected some published research literature for a full text review. The literature review identified trends in production planning and scheduling during the course of digital transformation. Based on the review results, we ultimately proposed the framework of an integrated production planning and scheduling system for Industry 4.0.
The proposed system is a hybrid HPP and MPP system. From theory to practice, the following two areas deserve a more detailed study:
6.1. Smooth system integration
Bao et al. (Citation2019) suggested future research on continuous and online interaction between physical and virtual spaces. Kang and Choi (Citation2010) suggested future research on an effective integration of the centralized approach with distributed MAS. And Fatorachian and Kazemi (Citation2018) suggested future research on interoperability among heterogeneous hardware and software entities.
6.2. Intelligent data analysis in big data
Fatorachian and Kazemi (Citation2018) suggested future research on intelligent algorithm development for big data analysis. Khakifirooz et al. (Citation2019) suggested future research on simulation-optimization methods that make use of real-time manufacturing data. Mourtzis et al. (Citation2015) suggested future research on the discovery of innate characteristics of a production system. And Zhong et al. (Citation2013) suggested future research on APS development for highly synchronized information flow in job shops.
In this review paper, we answered the following three research questions: ‘What is the state-of-the-art on integrated production planning and scheduling?’, ‘What are the trends in production planning and scheduling in light of the emerging frontier technologies?’, and ‘How would the production planning and scheduling system be like in the Industry 4.0 context?’ in Sections 3, 4, and 5, respectively. In the future, we will develop our proposed integrated production planning and scheduling system for industrial practice.
Acknowledgment
The authors would like to extend their heartfelt appreciation to the diligent reviewers, whose insightful comments and constructive feedback proved to be invaluable in shaping and improving the final version of the manuscript. The authors would also like to express their sincere thanks to the editor for their unwavering support, patience, and guidance throughout the entire publication process. Without the invaluable contributions of the reviewers and the unwavering support of the editor, this work would not have been possible.
Disclosure statement
No potential conflict of interest was reported by the author(s).
Data availability statement
The authors confirm the data supporting the findings of this study are available within the article [and/or] its supplementary materials.
References
- Adamiecki, K. (1931). Der Harmonograph, ein neuartiges Mittel zur schaubildlichen Darstellung von Betriebsvorgängen. Wirtschaftlichkeit, 108. https://bibliotekanauki.pl/articles/589427
- Aghezzaf, E.-H., Sitompul, C., & Van den Broecke, F. (2011). A robust hierarchical production planning for a capacitated two-stage production system. Computers & Industrial Engineering, 60 (2), 361–24. https://doi.org/10.1016/j.cie.2010.12.005
- Aguirre, A. M., & Papageorgiou, L. G. (2018). Medium-term optimization-based approach for the integration of production planning, scheduling and maintenance. Computers and Chemical Engineering, 116, 191–211. https://doi.org/10.1016/j.compchemeng.2018.04.030
- Alfieri, A., Tolio, T., & Urgo, M. (2012). A two-stage stochastic programming project scheduling approach to production planning. The International Journal of Advanced Manufacturing Technology, 62(1–4), 279–290. https://doi.org/10.1007/s00170-011-3794-4
- Altendorfer, K., Felberbauer, T., & Jodlbauer, H. (2016). Effects of forecast errors on optimal utilisation in aggregate production planning with stochastic customer demand. International Journal of Production Research, 54(12), 3718–3735. https://doi.org/10.1080/00207543.2016.1162918
- Arias-Bolzmann, L., Fernando Anton Agurto, W., Maria Coronel Chavez, A., Retamozo Pantoja, R., & Ticona Pinto, A. (2018). Decisions in hierarchical production planning: Goals, heuristics and bias. ESPACIOS, 39(14). www.revistaespacios.com/a18v39n14/a18v39n14p03.pdf
- Asmussen, J. N., Kristensen, J., Steger-Jensen, K., & Vejrum Wahrens, B. (2018). When to integrate strategic and tactical decisions? Introduction of an asset/inventory ratio guiding fit for purpose production planning. International Journal of Physical Distribution & Logistics Management, 48(5), 545–558. https://doi.org/10.1108/IJPDLM-02-2018-0058
- Audaces. 2021. Retrieved May09, 2023, from https://audaces.com/en/industry-3-0-industry-4-0-2/.
- Bao, J., Guo, D., Jie, L., & Zhang, J. (2019). The modelling and operations for the digital twin in the context of manufacturing. Enterprise Information Systems, 13(4), 534–556. https://doi.org/10.1080/17517575.2018.1526324
- Bhosekar, A., & Ierapetritou, M. (2018). Advances in surrogate based modeling, feasibility analysis, and optimization: A review. Computers and Chemical Engineering, 108, 250–267. https://doi.org/10.1016/j.compchemeng.2017.09.017
- Cheng, Y., Luning, B., Tao, F., & Ping, J. (2020). Hypernetwork-based manufacturing service scheduling for distributed and collaborative manufacturing operations towards smart manufacturing. Journal of Intelligent Manufacturing, 31(7), 1707–1720. https://doi.org/10.1007/s10845-018-1417-8
- Chunyang, Y., Jiang, X., Shiqiang, Y., & Yang, C. (2020). Blockchain-based shared manufacturing in support of cyber physical systems: Concept, framework, and operation. Robotics and Computer Integrated Manufacturing, 64, 101931. https://doi.org/10.1016/j.rcim.2019.101931
- D’Aniello, G., De Falco, M., & Mastrandrea, N. (2021). Designing a multi-agent system architecture for managing distributed operations within cloud manufacturing. Evolutionary Intelligence, 14(4), 2051–2058. https://doi.org/10.1007/s12065-020-00390-z
- Dan, W., & Ierapetritou, M. (2007). Hierarchical approach for production planning and scheduling under uncertainty. Chemical Engineering and Processing, 46(11), 1129–1140. https://doi.org/10.1016/j.cep.2007.02.021
- Daqiang, G., Mingxing, L., Zhong, R., & Huang, G. Q. (2021). Graduation intelligent manufacturing system (GiMS): An Industry 4.0 paradigm for production and operations management. Industrial Management & Data Systems, 121(1), 86–98. https://doi.org/10.1108/IMDS-08-2020-0489
- Dias, L. S., & Ierapetritou, M. G. (2020). Integration of planning, scheduling and control problems using data-driven feasibility analysis and surrogate models. Computers and Chemical Engineering, 134, 106714. https://doi.org/10.1016/j.compchemeng.2019.106714
- Dong, X., Srinivas Nageshwaraniyer, S., & Son, Y.J. (2016). A service-oriented simulation integration platform for hierarchical manufacturing planning and control. International Journal of Production Research, 54(23), 7212–7230. https://doi.org/10.1080/00207543.2016.1221535
- Farooqui, A., Bengtsson, K., Falkman, P., & Fabian, M. (2020). Towards data-driven approaches in manufacturing: An architecture to collect sequences of operations. International Journal of Production Research, 58(16), 4947–4963. https://doi.org/10.1080/00207543.2020.1735660
- Fatorachian, H., & Kazemi, H. (2018). A critical investigation of industry 4.0 in manufacturing: Theoretical operationalisation framework. Production Planning & Control, 29(8), 633–644. https://doi.org/10.1080/09537287.2018.1424960
- Gantt, H. L. (1903). A graphical daily balance in manufacture. Transactions of the American Society of Mechanical Engineers, 24, 1322–1336. https://babel.hathitrust.org/cgi/pt?id=mdp.39015023119541&view=1up&seq=1634
- Ghazanfari, M., & Murtagh, B. A. (2002). A multi-objective hierarchical production planning model under stochastic demand. Scientia Iranica, 9(3), 203–214.
- Giordani, S., Lujak, M., & Martinelli, F. (2013). A distributed multi-agent production planning and scheduling framework for mobile robots. Computers & Industrial Engineering, 64(1), 19–30. https://doi.org/10.1016/j.cie.2012.09.004
- Glomb, L., Liers, F., & Rosel, F. (2022). A rolling-horizon approach for multi-period optimization. European Journal of Operational Research, 300(1), 189–206. https://doi.org/10.1016/j.ejor.2021.07.043
- Guzman, E., Andres, B., & Poler, R. (2022). Models and algorithms for production planning, scheduling and sequencing problems: A holistic framework and a systematic review. Journal of Industrial Information Integration, 27, 100287. https://doi.org/10.1016/j.jii.2021.100287
- Gyulai, D., Pfeiffer, A., & Monostori, L. (2017). Robust production planning and control for multi-stage systems with flexible final assembly lines. International Journal of Production Research, 55(13), 3657–3673. https://doi.org/10.1080/00207543.2016.1198506
- Han, J., Liu, Y., Luo, L., & Mao, M. (2020). Integrated production planning and scheduling under uncertainty: A fuzzy bi-level decision-making approach. Knowledge-Based Systems, 201-202, 106056–106202. https://doi.org/10.1016/j.knosys.2020.106056
- Hax, A. C., & Meal, H. C. 1973. “Hierarchical integration of production planning and scheduling.” https://prolog.univie.ac.at/teaching/LVAs/KFK-Seminar/SS14/HaxMeal1973.pdf.
- Herrmann, J.-P., Tackenberg, S., Padoano, E., & Gamber, T. (2022). Approaches of production planning and control under Industry 4.0: A literature review. Journal of Industrial Engineering & Management, 15(1), 4–30.
- Holmstrom, J., Liotta, G., & Chaudhuri, A. (2017). Sustainability outcomes through direct digital manufacturing-based operational practices: A design theory approach. Journal of Cleaner Production, 167, 951–961. https://doi.org/10.1016/j.jclepro.2017.03.092
- Jamalnia, A., Yang, J.-B., Feili, A., Dong-Ling, X., & Jamali, G. (2019). Aggregate production planning under uncertainty: A comprehensive literature survey and future research directions. The International Journal of Advanced Manufacturing Technology, 102(1–4), 159–181. https://doi.org/10.1007/s00170-018-3151-y
- Jay, A., Glover, F., Kelly, J. P., & Laguna, M. 2003. Simulation-based optimization: practical introduction to simulation optimization. Proceedings of the 35th Winter Simulation Conference: Driving Innovation. New Orleans, Lousiana, USA.
- Jiang, N.-Y., & Yan, H.-S. (2022). Integrated optimization of production planning and scheduling in uncertain re-entrance environment for fixed-position assembly workshops. Journal of Intelligent & Fuzzy Systems, 42(3), 1705–1722. https://doi.org/10.3233/JIFS-211159
- Johnson, E. A. (1960). The long-range future of operational research. Operations Research, 8(1), 1–23. https://doi.org/10.1287/opre.8.1.1
- Johnson, L. A., & Montgomery, D. C. (1974). Operations research in production planning, scheduling, and inventory control (1st ed.). John Wiley & Sons.
- Jun, C., Yeon Lee, J., Yoon, J.-S., & Hyun Kim, B. 2017. Applications’ integration and operation platform to support smart manufacturing by small and medium-sized enterprises. Procedia Manufacturing. 1950–1957.
- Kang, S. G., & Choi, S. H. (2010). Multi-agent based beam search for intelligent production planning and scheduling. International Journal of Production Research, 48(11), 3319–3353. https://doi.org/10.1080/00207540902810502
- Kan, W., & Shen, Y. (2020). A unified view on planning, scheduling and dispatching for a factory. Advanced Engineering Informatics, 46, 101188. https://doi.org/10.1016/j.aei.2020.101188
- Khakifirooz, M., Fathi, M., & Kan, W. (2019). Development of smart semiconductor manufacturing: Operations research and data science perspectives. Institute of Electrical and Electronics Engineers Access, 7, 108419–108430. https://doi.org/10.1109/ACCESS.2019.2933167
- Krishnan, T., Khan, A., & Alqurni, J. (2022). Aggregate production planning and scheduling in the Industry 4.0 environment. Procedia Computer Science, 204, 784–793. https://doi.org/10.1016/j.procs.2022.08.095
- Kumar, S., Manjrekar, V., Singh, V., & Kumar Lad, B. (2020). Integrated yet distributed operations planning approach: A next generation manufacturing planning system. Journal of Manufacturing Systems, 54, 103–122. https://doi.org/10.1016/j.jmsy.2019.12.001
- Lee, R. (2021). The effects of smart factory operational strategies and system management on the innovative performance of small- and medium- sized manufacturing firms. Sustainability, 13(6), 3087. https://doi.org/10.3390/su13063087
- Legat, C., & Vogel-Heuser, B. (2017). A configurable partial-order planning approach for field level operation strategies of PLC-based industry 4.0 automated manufacturing systems. Engineering Applications of Artificial Intelligence, 66, 128–144. https://doi.org/10.1016/j.engappai.2017.06.014
- Lenstra, J., & Rinnooym Kan, A. (1979). Computational complexity of discrete optimization problems. Annals of Discrete Mathematics, 4, 121–140. https://doi.org/10.1016/S0167-5060(08)70821-5
- Luo, C., & Rong, G. (2009). A strategy for the integration of production planning and scheduling in refineries under uncertainty. Chinese Journal of Chemical Engineering, 17(1), 113–127. https://doi.org/10.1016/S1004-9541(09)60042-2
- Luo, D., Thevenin, S., & Dolgui, A. (2022). A state-of-the-art on production planning in Industry 4.0. International Journal of Production Research. https://doi.org/10.1080/00207543.2022.2122622
- Maravelias, C. T., & Sung, C. (2009). Integration of production planning and scheduling: Overview, challenges and opportunities. Computers and Chemical Engineering, 33(12), 1919–1930. https://doi.org/10.1016/j.compchemeng.2009.06.007
- Matthias, T., Fernandes, N. O., & Stevenson, M. 2020. Production planning and control in multi-stage assembly systems: An assessment of kanban, MRP, OPT (DBR) and DDMRP by simulation. International Journal of Production Research 1036–1050.
- Menezes, G. C., Robson Meteus, G., & Gomez Ravetti, M. (2016). A hierarchical approach to solve a production planning and scheduling problem in bulk cargo terminal. Computers & Industrial Engineering, 97, 1–14. https://doi.org/10.1016/j.cie.2016.04.007
- Mohamad, S., Pantamee, A. A., Keong, O. C., Hieu, V. M., Mutira, P., & Chong, K. W. (2022). Impact of industry 4.0 revolution and leadership support on the operational efficiency management of the manufacturing industry in ASEAN countries. Polish Journal of Management Studies, 25(2), 191–204. https://doi.org/10.17512/pjms.2022.25.2.12
- Monostori, L., Erdos, G., Kadar, B., Kis, T., Kovacs, A., Pfeiffer, A., & Vancza, J. (2010). Digital enterprise solution for integrated production planning and control. Computers in Industry, 61(2), 112–126. https://doi.org/10.1016/j.compind.2009.10.008
- Mourtzis, D., Doukas, M., & Psarommatis, F. (2015). A toolbox for the design, planning and operation of manufacturing networks in a mass customisation environment. Journal of Manufacturing Systems, 36, 274–286. https://doi.org/10.1016/j.jmsy.2014.06.004
- Mula, J., Poler, R., Garcia-Sabater, J. P., & Lario, F. C. (2006). Models for production planning under uncertainty: A review. International Journal of Production Economics, 103(1), 271–285. https://doi.org/10.1016/j.ijpe.2005.09.001
- O’Reilly, S., Kumar, A., & Adam, F. (2015). The role of hierarchical production planning in food manufacturing SMEs. International Journal of Operations & Production Management, 35(10), 1362–1385. https://doi.org/10.1108/IJOPM-04-2014-0157
- Pansare, R., & Yadav, G. (2022). Repurposing production operations during COVID-19 pandemic by integrating Industry 4.0 and reconfigurable manufacturing practices: An emerging economy perspective. Operations Management Research, 15(3–4), 1270–1289. https://doi.org/10.1007/s12063-022-00310-7
- Park, K. T., Lee, D., & Do Noh, S. (2020). Operation procedures of a work-center-level digital twin for sustainable and smart manufacturing. International Journal of Precision Engineering and Manufacturing-Green Technology, 7(3), 791–814. https://doi.org/10.1007/s40684-020-00227-1
- Paula Ferreira, W., Armellini, F., Santa-Eulalia, L., & Rebolledo, C. (2021). Modeling and simulation for Industry 4.0. In Dingli, A., Haddod, F., & Klüver, C. (Eds.), Artificial intelligence in industry 4.0 - a collection of innovative research case-studies that are reworking the way we look at industry 4.0 thanks to artificial intelligence (pp. 57). Springer International Publishing. https://doi.org/10.1007/978-3-030-61045-6_5
- Rafiei, H., & Rabbani, M. (2009). An MADM framework toward hierarchical production planning in hybrid MTS/MTO environments. International Journal of Industrial and Manufacturing Engineering, 3(10), 1214–1218. https://publications.waset.org/5435/an-madm-framework-toward-hierarchical-production-planning-in-hybrid-mtsmto-environments
- Rafiei, H., Rabbani, M., & Alimardani, M. (2013). Novel bi-level hierarchical production planning in hybrid MTS/MTO production contexts. International Journal of Production Research, 51(5), 1331–1346. https://doi.org/10.1080/00207543.2012.661089
- Rajesh, P., Yadav, G., & Nagare, M. R. (2022). Integrating operational excellence strategies with Industry 4.0 technologies through reconfigurable manufacturing system practices. The TQM Journal. https://doi.org/10.1108/TQM-06-2022-0184
- Santander, O., Betts, C. L., Archer, E. E., & Baldea, M. (2020). On the interaction and integration of production planning and (advanced) process control. Computers and Chemical Engineering, 133, 106627. https://doi.org/10.1016/j.compchemeng.2019.106627
- Schuh, G., & Stich, V. (2012). Produktionsplanung und -steuerung 1. Springer.
- Shah, N. K., & Ierapetritou, M. G. (2012). Integrated production planning and scheduling optimization of multisite, multiproduct process industry. Computers and Chemical Engineering, 37, 214–226. https://doi.org/10.1016/j.compchemeng.2011.08.007
- Sharma, M., Raut, R. D., Sehrawat, R., & Ishizaka, A. (2023). Digitalisation of manufacturing operations: The influential role of organisational, social, environmental, and technological impediments. Experts Systems with Applications, 211, 211. https://doi.org/10.1016/j.eswa.2022.118501
- Silver, E. A., Pyke, D. F., & Peterson, R. (1998). Inventory management and production planning and scheduling (3rd ed.). John Wiley & Sons.
- Susara, A., Grossmann, van den Heever, I. E., & Ignacio, E. G. (2003). A strategy for the integration of production planning and reactive scheduling in the optimization of a hydrogen supply network. Computers & Chemical Engineering, 27(12), 1813–1839. https://doi.org/10.1016/S0098-1354(03)00158-3
- Tan, Q., Tong, Y., Shaofeng, W., & Dongbo, L. (2019). Modeling, planning, and scheduling of shop-floor assembly process with dynamic cyber-physical interactions: A case study for CPS-based smart industrial robot production. The International Journal of Advanced Manufacturing Technology, 105(9), 3979–3989. https://doi.org/10.1007/s00170-019-03940-7
- Taylor, F. W. (1911). Shop management. Harper & Brothers. https://ia800808.us.archive.org/25/items/TaylorShopManagementGood/Taylor%20shop%20management%20good.pdf
- Torabi, S. A., Ebadian, M., & Tanha, R. (2010). Fuzzy hierarchical production planning (with a case study). Fuzzy Sets and Systems, 161(11), 1511–1529. https://doi.org/10.1016/j.fss.2009.11.006
- Venkateswaran, J., & Son, Y. J. (2005). Hybrid system dynamic - discrete event simulation-based architecture for hierarchical production planning. International Journal of Production Research, 43(20), 4397–4429. https://doi.org/10.1080/00207540500142472
- Vogel, T., Almada-Lobo, B., & Almeder, C. (2017). Integrated versus hierarchical approach to aggregate production planning and master production scheduling. OR Spectrum, 39(1), 193–229. https://doi.org/10.1007/s00291-016-0450-2
- Wen, H., Hou, S., Liu, Z., & Liu, Y. (2017). An optimization algorithm for integrated remanufacturing production planning and scheduling system. Chaos, Solitons and Fractals, 105, 69–76. https://doi.org/10.1016/j.chaos.2017.10.012
- Yan, H.-S., Wan, X.-Q., & Xiong, F.-L. (2015). Integrated production planning and scheduling for a mixed batch job-shop based on alternant iterative genetic algorithm. Journal of the Operational Research Society, 66(8), 1250–1258. https://doi.org/10.1057/jors.2014.88
- Yaqiong, L., & Lin, D. (2017). Design an intelligent real-time operation planning system in distributed manufacturing network. Industrial Management & Data Systems, 117(4), 742–753. https://doi.org/10.1108/IMDS-06-2016-0220
- Zangiacomi, A., Oesterle, J., Fornasiero, R., Sacco, M., & Azevedo, A. (2017). The implementation of digital technologies for operations management: A case study for manufacturing apps. Production Planning & Control, 28(16), 1318–1331. https://doi.org/10.1080/09537287.2017.1375142
- Zanjani, M. K., Nourelfath, M., & Ait-Kadi, D. (2010). A multi-stage stochastic programming approach for production planning with uncertainty in the quality of raw materials and demand. International Journal of Production Research, 48(16), 4701–4723. https://doi.org/10.1080/00207540903055727
- Zhang, M., & Qi, Y. (2013). Impact of supply chain strategy on mass customization implementation and effectiveness: Evidence from China. International Journal of Information and Decision Sciences, 5(4), 393–413. https://doi.org/10.1504/IJIDS.2013.058287
- Zhang, T., Wang, Y., Jin, X., & Shan, L. (2019). Integration of production planning and scheduling based on RTN representation under uncertainties. Algorithms, 12(6), 120. https://doi.org/10.3390/a12060120
- Zhi-Min, L., Jiang, T.-R., & Zai-Wei, L. (2021). Multiproduct and multistage integrated production planning model and algorithm based on an available production capacity network. International Journal of Minerals, Metallurgy and Materials, 28(8), 1343–1352. https://doi.org/10.1007/s12613-021-2310-6
- Zhong, R. Y., Huang, G. Q., Shulin Lan, Q. Y. D., Zhang, T., Chen, X., & Xu, C. (2015). A two-level advanced production planning and scheduling model for RFID-enabled ubiquitous manufacturing. Advanced Engineering Informatics, 29(4), 799–812. https://doi.org/10.1016/j.aei.2015.01.002
- Zhong, R. Y., Li, Z., Pang, L. Y., Pan, Y., Qu, T., & Huang, G. Q. (2013). RFID-enabled real-time advanced planning and scheduling shell for production decision making. International Journal of Computer Integrated Manufacturing, 26(7), 649–662. https://doi.org/10.1080/0951192X.2012.749532
- Zukui, L., & Ierapetritou, M. G. (2009). Integrated production planning and scheduling using a decomposition framework. Chemical Engineering Science, 64(16), 3585–3597. https://doi.org/10.1016/j.ces.2009.04.047
- Zukui, L., & Ierapetritou, M. G. (2010). Production planning and scheduling integration through augmented Lagrangian optimization. Computers and Chemical Engineering, 34(6), 996–1006. https://doi.org/10.1016/j.compchemeng.2009.11.016