ABSTRACT
Lyme borreliosis, recognized as one of the most important tick-borne diseases worldwide, has been increasing in incidence and spatial extent. Currently, there are few geographic studies about the distribution of Lyme borreliosis risk across China. Here we established a nationwide database that involved Borrelia burgdorferi sensu lato (B. burgdorferi) detected in humans, vectors, and animals in China. The eco-environmental factors that shaped the spatial pattern of B. burgdorferi were identified by using a two-stage boosted regression tree model and the model-predicted risks were mapped. During 1986−2020, a total of 2,584 human confirmed cases were reported in 25 provinces. Borrelia burgdorferi was detected from 35 tick species with the highest positive rates in Ixodes granulatus, Hyalomma asiaticum, Ixodes persulcatus, and Haemaphysalis concinna ranging 20.1%−24.0%. Thirteen factors including woodland, NDVI, rainfed cropland, and livestock density were determined as important drivers for the probability of B. burgdorferi occurrence based on the stage 1 model. The stage 2 model identified ten factors including temperature seasonality, NDVI, and grasslands that were the main determinants used to distinguish areas at high or low-medium risk of B. burgdorferi, interpreted as potential occurrence areas within the area projected by the stage 1 model. The projected high-risk areas were not only concentrated in high latitude areas, but also were distributed in middle and low latitude areas. These high-resolution evidence-based risk maps of B. burgdorferi was first created in China and can help as a guide to future surveillance and control and help inform disease burden and infection risk estimates.
Introduction
Lyme borreliosis, caused by the complex Borrelia (B.) burgdorferi sensu lato (hereinafter referred to as B. burgdorferi), is the most common tick-borne disease in the Northern Hemisphere[Citation1,Citation2], especially in the United States, with approximately 476,000 cases per year[Citation3]. In European countries such as Netherlands, Austria, and Estonia, the annual incidence of Lyme borreliosis has reached >80 cases per 100,000 individuals [Citation4]. Borrelia burgdorferi sensu lato infection in humans can cause skin, joints, heart, nervous system and other tissues and organs to be injured, leading to erythema migrans (EM), Lyme arthritis, meningo-radiculo-neuritis, acrodermatitis chronica atrophicans (ACA) and other clinical symptoms[Citation1,Citation2]. The main clinical features of Lyme borreliosis in North America and Europe are similar, however, there are more frequent systemic symptoms and seroreactivity in North America and more diverse clinical manifestations in Europe, such as ACA and borrelial lymphocytoma, which are not observed in North America[Citation1,Citation5]. Although more than 20 genospecies of B. burgdorferi complex have been found[Citation6], there are three genospecies that most commonly cause Lyme borreliosis in humans: B. afzelii, B. garinii and B. burgdorferi sensu stricto, which are characterized by different vector competences, geographical distributions, and pathogenicity to humans[Citation7]. In Europe, these three genospecies have been reported, and they are mainly transmitted by Ixodes (I.) ricinus[Citation5,Citation8]. Borrelia burgdorferi sensu stricto is more frequently reported in the United States, which is mainly spread by I. scapularis and I. pacificus[Citation1]. In Asia, B. afzelii and B. garinii are predominant, while B. burgdorferi sensu stricto is rare, and the main vector is often I. persulcatus [Citation8,Citation9].
In China, since Lyme borreliosis was first reported in Hailin county in Heilongjiang Province[Citation10], it has been found to be endemic in a large range of geographic regions, vectored mainly by Ixodes ticks, which maintain B. burgdorferi in a horizontal transmission cycle between ticks and multiple vertebrate hosts[Citation11]. According to the most recent literature review in China, Lyme borreliosis is carried by at least 13 tick species[Citation12]. Four genospecies of B. burgdorferi including B. afzelii, B. garinii, B. burgdorferi sensu stricto and B. valaisiana-related have been reported to cause Lyme borreliosis in China. Borrelia garinii is the most dominant genospecies, followed by B. afzelii, and the other two are much less frequent[Citation1,Citation13–16]. Ixodes persulcatus as well as various other Ixodes and Haemaphysalis species are the vectors of Lyme borreliosis in China[Citation14,Citation17]. Many studies have either predicted the distribution of vectors of B. burgdorferi, such as I. persulcatus and I. granulatus, through species distribution models, or focused on the investigations of B. burgdorferi infections in local areas[Citation18–22]. But there are no studies about distributions and risk assessment of B. burgdorferi infections in humans, animals and vectors throughout the mainland of China which has hindered informed control strategies.
In this study, we established a comprehensive database on distributions of B. burgdorferi across the mainland of China during 1986−2020, with the goal of acquiring a better understanding of the epidemiology and distribution of Lyme borreliosis since its first identification in 1986. We also associated a variety of eco-environmental factors with the risk of B. burgdorferi infection, to build a comprehensive predictive model of the occurrence of B. burgdorferi infection in China.
Method
Data collection and management
We assembled five datasets, including four involving the occurrence of B. burgdorferi (i) among human confirmed cases, (ii) in human individuals with serological evidence, (iii) in animals (livestock and wild animals) with molecular, serological, or pathogen isolation evidence, (iv) in ticks with molecular, serological or pathogen isolation evidence, as well as one dataset related to the eco-environmental factors, which were chosen due to their potential effect on the ecological suitability of B. burgdorferi occurrence based upon the previous studies[Citation23–25]. These included climate data from Worldclim, livestock density information from the Food and Agriculture Organization, mammalian richness from NASA, land cover, elevation, Normalized Difference Vegetation Index (NDVI, a proxy for the density of actively photosynthesising vegetation[Citation26]), population density, and night-time light from China Resource and Environment Science and Data Center (Supplementary Tables 1 and 2).
Literature related to B. burgdorferi were searched from the major databases (PubMed, China National Knowledge Infrastructure, China WanFang database, and Chinese Scientific Journal database), with the keywords “Lyme” or Borrelia burgdorferi and “China.” All the publications between January 1986 and December 2020 were searched without language limitations. Studies were eligible if they described the laboratory detection of B. burgdorferi in humans, ticks, or animals by any of the molecular assay, serological assay, or isolation. We excluded the following studies: (i) evaluation of the specificity and sensitivity of a detection method; (ii) B. burgdorferi infection efficiency trials; and (iii) studies using previously preserved strains. In addition, unpublished B. burgdorferi data from GenBank (https://www.ncbi.nlm.nih.gov/genbank/) were supplemented ().
Figure 1. Flow chart of literature review. *Other test assays included RFLP (restriction fragment length polymorphism) and MLSA (multilocus sequence analysis).
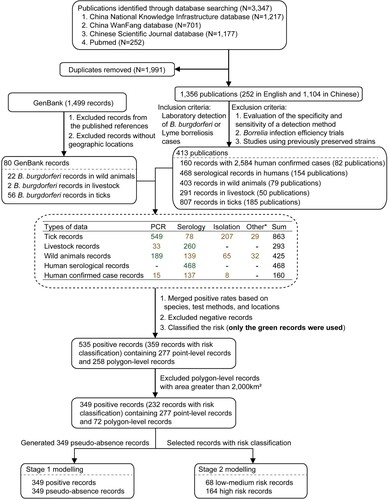
Title and abstracts of the retrieved studies using the search strategy were screened independently by two reviewers (QX, BGJ) to identify studies potentially eligible for inclusion. The full texts of the potentially eligible studies were retrieved and independently assessed for eligibility by two reviewers (TLC, JJC). All conflicts of opinion and uncertainties were discussed and resolved by consensus with a third reviewer. Attempts were also made to clarify any uncertainties or missing data in selected reports with the corresponding authors. The main content of the database was to summarize the positive rates of B. burgdorferi for different hosts or vector ticks in different locations. The positive rates of multiple surveys were combined when the surveyed species of hosts or vector ticks and detection method of B. burgdorferi were same in the same locations, e.g. the overall positive rate of B. burgdorferi in I. persulcatus was calculated by overall number of positive detections divided by the number of I. persulcatus tested if multiple investigations of I. persulcatus were performed in the same place. Then locations with negative tests for B. burgdorferi were excluded. The detailed data extraction, variables definition, numbers of publications and records, and data used for the two-stage BRT model are shown in and Supplementary method.
The risk classification of B. burgdorferi
A positive record was defined as one or more laboratory or clinically confirmed infections of B. burgdorferi in human beings, or B. burgdorferi detected from ticks or animals occurring at a unique location (the same administrative area or 10 km×10 km pixel for points). For each positive record, we used positive rates of B. burgdorferi in humans, livestock, wild animals, or ticks as proxies for the grading of the B. burgdorferi risk, and the positive rate was only valid when the samples tested were greater than 10. To ensure the homogeneity of these positive rates, we only included serological tests of livestock and humans (excluding investigations focusing on people with specific clinical conditions, such as psychosis and arthritis), PCR tests of ticks and wild animals for the risk classification since other methods had fewer records (). For a specific sampling site where multiple tick species were tested, the highest positive rate of tick species was considered as the final positive rate. The same method was applied to wild animals and livestock. Positive records not used for risk classification were considered unknown risk, including human confirmed cases, ticks and wild animals not tested by PCR, livestock not tested by serology, and data with less than 10 tests ().
Based on the final positive rates that were determined for each record, the 3rd quartile of each type of data (11% for humans, 17% for wild animals, 25% for livestock, and 24% in ticks) was used as a threshold to classify the risk for each of surveyed locations. Briefly, the surveyed locations were considered as with high-risk if (1) positive detection rate of B. burgdorferi by PCR higher than 24% in ticks or higher than 17% in wild animals; or (2) positive detection rate by serological assay higher than 11% in humans or higher than 25% in livestock. Otherwise, the low-medium risk classification was defined. Our goal was to obtain a comprehensive risk classification from different data sources, e.g. investigations of B. burgdorferi in ticks, wild animals, livestock and humans, because a single type of data was not enough to cover all regions. For those survey sites where they were more than one type of positive rate, the risk was determined based on the data type that produced the highest risk classification.
In addition, we selected pseudo-absence records considered as “controls” in the modelling analysis to indicate locations with no risk. The method was as follows: (1) Randomly sampled the pseudo-absence records from 10 km×10 km pixel map; (2) The sampling range was grids within 200 km of any occurrence record, but excluded grids within 20 km of any occurrence record. (3) The number of samples was the same as the occurrence records [Citation27,Citation28]. Finally, locations with 4 categories of B. burgdorferi risk were generated, including no risk (pseudo-absence records), medium-low risk (positive rate less than 3rd quartile), high risk (positive rate greater than 3rd quartile) and unknown risk.
Model development
The BRT model is usually used for ecological niche modelling[Citation29,Citation30], similar to logistic regression, with a binary outcome as the dependent variable and environmental variables as the independent variable. A two-stage boosted regression tree (BRT) model was applied to identify the potential ecological drivers for distribution of B. burgdorferi and create a high-resolution risk map using the R package “dismo”[Citation31] and “gbm”[Citation32] based on the locations with 4 categories of B. burgdorferi risk. The Pearson correlation coefficient was used to screen variables to reduce multicollinearity, only one variable was kept when two variables were correlated (r > 0.75) (Supplementary Figure 1). Subsequently, we filtered out variables with a relative contribution of less than 4% by conducting a pre-model for each of the two-stage BRT models by including all the variables filtered by the multicollinearity test. Based on 13 screened variables including 5 eco-climatic variables, 4 land cover types, livestock, population density, NDVI, and elevation, the stage 1 model was used to distinguish whether there was a risk of B. burgdorferi occurrence. In this case, all locations with medium-low risk, high risk and unknown risk were considered as “cases,” and those with no risk (pseudo-absence records) were considered as “controls.” The stage 2 model further distinguished high or low-medium risk for areas at risk of B. burgdorferi occurrence projected by the stage 1 model, and 10 screened variables, including 2 eco-climatic variables, 5 land cover types, population density, NDVI, and mammalian richness were included in the stage 2 modelling analysis.
The positive rates of different sources may vary somewhat, e.g. a higher positive rate of ticks in a particular area did not necessarily mean a higher positive rate of humans. Therefore, we separately ran the models by including only human or tick data as sensitivity analyses in the stage 2 model, while separate stage 2 model was not performed by including only wild animals and livestock due to few available records with specific locations. In addition, the stage 1 model was conducted by including all types of data, rather than by including only one type of data, because any positive detection in humans, ticks or animals can indicate the presence of this pathogen. In addition, we also performed sensitivity analyses using the 72nd and 78th percentiles, respectively, as thresholds for risk classification in the stage 2 BRT model. The Kappa coefficient was calculated to assess the consistency of sensitivity analyses with the main result. Details about model development, screening of multicollinearity, cross-validation for tuning parameters, assessment of goodness-of-fit, and sensitivity analysis were given in the Supplementary method and Supplementary Figure 1.
All analysis was performed in R 3.6.3 (R Foundation for Statistical Computing, Vienna, Austria.) and ArcGIS 10.7 (ESRI Inc., Redlands, CA, USA).
Results
Database assembly
The literature review yielded a total of 1,356 publications. In addition, 1,499 records from GenBank were determined. With the consensus of two independent reviewers, 413 publications and 80 records from GenBank met the inclusion criteria and were used for data extraction. After pooling data from all sources, we assembled a comprehensive database of B. burgdorferi occurrence including 160 records reporting on the human confirmed cases, 468 records on serological tests in humans, and 293, 425, and 863 records in livestock, wild animals, and ticks, respectively ().
Human confirmed cases and serological investigation of B. burgdorferi
A total of 2,584 confirmed cases were reported from 1986 to 2020 and were mainly located in the Northeast China (942 cases, 36.5%), Inner Mongolia-Xinjiang (1,034 cases, 40.0%) and North China regions (324 cases, 12.5%) (A and ). Based on available demographic data of cases, males accounted for 61.3% (1,013/1,653), and over half were aged 21–40 years (55.8%, 444/796), followed by 23.2% for 41–60 years olds and 17.5% for children and teenagers aged under 20 years. The age group over 60 had the lowest proportion (3.5%). Forest workers were the most frequent occupation of patients (39.7%, 305/768), followed by farmers and herdsmen (18.6%). Among 1,497 patients with known clinical symptoms, the most frequently seen were erythema migrans (44.7%), arthritis (24.4%), and neurologic manifestations (22.4%), while cardiac manifestations (5.3%), lymphadenopathy (2.2%), ocular manifestations (1.3%), and acrodermatitis chronica atrophicans (0.5%) were reported as the occasional complications. The incidence of erythema migrans in Northeast China attained as high as 60.6%, which differed from the South and the Qinghai-Tibet region of China, where arthritis was reported as the most common symptom (68.3% and 56.8%, respectively). A total of 468 records on serological surveys in human beings were reported from 154 publications, which resulted in an overall seroprevalence of 7.8% in healthy population, with particularly high seropositive rate in Xinjiang Uygur Autonomous Region (20.2%, 982/4,861) and Chongqing City (18.0%, 54/300), followed by Inner Mongolia Autonomous Region (14.2%, 729/5,133), Qinghai (12.6%, 373/2,969), Heilongjiang (11.4%, 911/7,965) and Hubei provinces (11.0%, 159/1,439) (B).
Figure 2. Distribution of Lyme borreliosis cases and the positive rate of Borrelia burgdorferi sensu lato. The quartiles of each type of data were used to truncate the corresponding data. (A) Distribution of confirmed cases of Lyme borreliosis. (B) Distribution of infection rate of specific antibody for Borrelia burgdorferi sensu lato in humans. (C) Distribution of detection rate for Borrelia burgdorferi sensu lato in wild animals. (D) Distribution of infection rate of specific antibody for Borrelia burgdorferi sensu lato in livestock. (E) Distribution of detection rate for Borrelia burgdorferi sensu lato in ticks. (F) Genospecies distribution of Borrelia burgdorferi sensu lato in China. Records reported at the province level were represented as a triangle, while records reported at the point, county, city-level were represented as a circle. *Positive rate was not calculated for the data with the number of samples tested less than 10. #Other test assays included serological method, isolation, RFLP, and MLSA. †Other test assays included PCR. ‡It has been isolated from patients.

Table 1. Demographic characteristics and clinical manifestations of Lyme borreliosis cases, 1986–2020, China
B. burgdorferi in animals
A total of 403 records of B. burgdorferi in wild animals were reported, with an overall positive rate of 11.5% (945/8,226, 95% CI: 10.8%–12.2%) using PCR tests. The prevalence of B. burgdorferi varied by animal species when the study sites were combined, with the highest positive rate obtained from Apodemus (A.) sylvaticus (54.4%, 92/169) and followed by A. peninsulae (36.4%, 68/187) (Supplementary Table 3). A geographic difference existed, with the highest positive rates of B. burgdorferi infection among tested wild animals observed in Jiangxi Province (40.7%, 83/204) and in Tianjin City (32.0%, 32/100) (C).
Altogether 293 records of B. burgdorferi in livestock were reported, which yielded an overall seroprevalence of 17.4% (2,727/15,667, 95% CI: 16.8%–18.0%). When disaggregated by livestock types, we observed a highly similar positive rate between goats (18.1%, 1,583/9,318), cattle (21.2%, 653/3,079), and horses (18.6%, 45/242), all higher than that observed in dogs (12.0%, 429/3,566) (Supplementary Table 3). Spatially, the highest positive rate of B. burgdorferi in livestock was recorded in Sichuan Province (62.1%, 64/103), followed by Xinjiang Uygur Autonomous Region (28.2%, 640/2 267) and Anhui Province (27.8%, 40/144) (D).
B. burgdorferi in ticks
A total of 863 records of B. burgdorferi in ticks were reported, yielding positive detection in 35 tick species, and negatives in 12 tick species ( and Supplementary Table 4). The PCR prevalence of B. burgdorferi varied among the 35 tick species, with the high and comparable level attained among I. granulatus (24.0%, 92/384), Hyalomma (Hy.) asiaticum (22.0%, 304/1,379), I. persulcatus (20.7%, 1,821/8,777), and Haemaphysalis (Ha.) concinna (20.1%, 110/548), while low prevalence (less than 20%) obtained from other 31 tick species. The highest richness of tick species that were positive for B. burgdorferi (12 tick species out of 16 tick species) was observed in Xinjiang Uygur Autonomous Region. The highest number of tests and the highest positive rate (22.0%, 1,574/7,139) of B. burgdorferi in ticks were observed in Northeast China (E, ).
Figure 3. Distribution of the positive rate of Borrelia burgdorferi sensu lato detected in different tick species in China during 1986–2020. The quartiles of PCR positive rate in ticks were used to truncate the data. (A) Ixodes (I.). (B) Haemaphysalis (Ha.). (C) Dermacentor (D.). (D) Hyalomma (Hy.) and Rhipicephalus (R.). *The blue, red, and green asterisks represent I. crenulatus, I. kuntzi and Ha. nepalensis, respectively, and their positive rates were not calculated because the number of samples tested was less than 10.
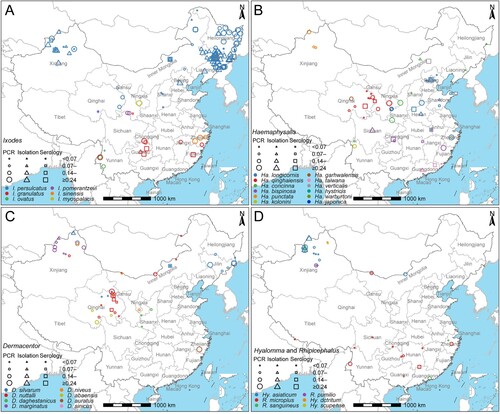
Genospecies of B. burgdorferi
A total of nine genospecies of B. burgdorferi were reported in the mainland of China (F, Supplementary Table 5). Borrelia garinii was the most predominant genospecies, and had been reported in 21 provinces of China. Borrelia afzelii or B. valaisiana was the most prevalent genospecies in Southwest China such as Yunnan, Guizhou, Guangxi provinces, and Chongqing municipality. Borrelia burgdorferi sensu stricto was predominantly detected in Qinghai, Xinjiang, Yunnan, and Jilin provinces, and B. valaisiana-related was detected in Heilongjiang Province and seven provinces in South and Central China. Other genospecies such as B. bavariensis, B. bissettii, B. japonica and, B. sinica were detected only sporadically. Among them, B. garinii, B. afzelii, B. burgdorferi sensu stricto, and B. valaisiana-related have been detected in Lyme borreliosis patients, but only in Xinjiang, Heilongjiang, Inner Mongolia, Shandong, Chongqing, and Hainan provinces.
The risk prediction of B. burgdorferi
According to the predictive model, four main high-risk areas for B. burgdorferi were defined that were centred around the mountains, i.e. Northeast China along the Changbai Mountains and the Greater Khingan Mountains; Inner Mongolia-Xinjiang region centreing around the Tianshan Mountains and the Altai Mountains; South, North China and Qinghai-Tibet region surrounding Qinling Mountains; Central China region, covering Guizhou Province, Chongqing and southwestern Hubei with the surrounding Wuling Mountains (). In addition to the four hotspot areas, there were also scattered high-risk areas in northern Hebei Province, easternmost Shandong Province, southernmost Yunnan Province, southern Anhui Province, northern Fujian Province, and northern Jiangxi Province. The predicted B. burgdorferi risk area was wider than actual observed from the literature review based on the grid map, with the area at high-risk increased from 11,000 to 971,400 km2, the population size at high-risk increased from 1.4 to 116.6 million people, the area of low-medium risk increased from 25,100 to 820,100 km2, and the population size of low-medium risk increased from 5.1 to 251.0 million people (). Sensitivity analysis results showed that the mixed data model by including all of the human, tick and animal data had a substantial spatial similarity with the model of human data (kappa coefficient = 0.60, P < 0.001), and had a moderate spatial similarity with the model of tick data (kappa coefficient = 0.42, P < 0.001). However, in Jilin Province and Fujian Province, the human data model did not predict high risk and the mixed data model predicted high risk. At the same time, we also observed that the tick data model predicted many high-risk areas in these two provinces (Supplementary Figure 2). In addition, the prediction results using the 72nd percentile and the 78th percentile as risk classification thresholds were also highly similar to the final prediction results, with kappa coefficients of 0.73 and 0.75 (P < 0.001), respectively (Supplementary Figure 3).
Figure 4. Recorded and predicted risk distribution of Borrelia burgdorferi sensu lato presence in China. (A) Borrelia burgdorferi sensu lato risk classification based on literature review. Background points sampled from the grid map based on distribution of positive records. The coordinates of polygon centroids were displayed for city-level or county-level evidence. (B) Predicted risk distribution of Borrelia burgdorferi sensu lato after averaged 100 bootstrapping BRT models. The thresholds of stage 1 and stage 2 model was determined by the cut-off values at which the Youden index of the test set was maximum. Black Doted Circles represent different high-risk hotspot areas. I = Northeast region, II = North China region, III = Inner Mongolia-Xinjiang region, IV = Qinghai-Tibet region, V = Southwest region, VI = Central China region, and VII = South China region.
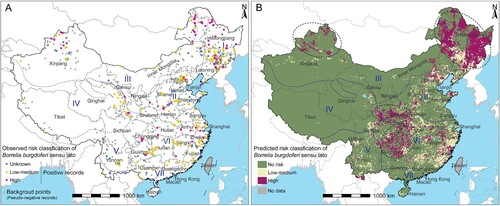
Table 2. The area and population size of the Borrelia burgdorferi sensu lato occurrence risk predicted by the BRT model.
Ecological niches suitable for B. burgdorferi distribution
In stage 1 of the BRT model, thirteen variables were determined as important drivers for distinguishing whether there was a risk of B. burgdorferi occurrence ( and Supplementary Figure 4). Six of them showed a positive correlation with the occurrence of B. burgdorferi, among which closed-canopy woodland has the largest relative contribution to the occurrence of B. burgdorferi (12.5%), followed by livestock density, population density, NDVI, other woodland, and rainfed cropland (Supplementary Figures 5−7). Three of them, the annual mean temperature, total precipitation, and isothermality, were negatively associated with B. burgdorferi occurrence. Nonlinear effects were determined from the remaining variables, including temperature seasonality, elevation, precipitation seasonality, and shrub.
Table 3. The relative contribution of environmental variables to predict the occurrence risk of Borrelia burgdorferi sensu lato based on BRT model.
In stage 2 of the BRT model, ten predictors were determined to attain good performance in discriminating regions at high risk and low-medium risk of B. burgdorferi ( and Supplementary Figure 4). Temperature seasonality, high coverage grasslands, NDVI, mammalian richness, moderate coverage grasslands, and sparse-canopy woodland were positively related to increased risk of B. burgdorferi, while isothermality was negatively associated with the risk of B. burgdorferi (Supplementary Figures 8−10). Nonlinear effects were observed from population density, shrub, and other woodland. In the stage 2 model including only human data, climatic factors such as annual mean temperature and temperature seasonality were dominant factors, while vegetation-related variables such as NDVI and moderate coverage grasslands were the most important factors in the stage 2 model using only tick data (Supplementary Table 6).
The validation statistics showed that both stages of the BRT model have decent predictive performance, with AUC of 0.82 and 0.80 respectively. In addition, the sensitivity, specificity, accuracy, and F1 score also showed good performance of the model (Supplementary Figure 11 and Supplementary Table 7).
Discussion
In China, evidence of Lyme borreliosis have been reported in many regions in recent years, while the disease burden and distribution of risk areas remained obscure, due to not being included in notifiable infectious diseases for surveillance. To our knowledge, this is the first systematic analysis of the appropriate niche of B. burgdorferi and risk mapping on distribution of B. burgdorferi in China. The demographic characteristics and clinical profile of Lyme borreliosis patients, the main tick vectors, animal hosts of B. burgdorferi, and distribution of B. burgdorferi genospecies in China, were comprehensively described.
Although our results showed a downward trend in the number of Lyme borreliosis cases in recent years, this did not reflect a reduction in the disease burden of Lyme borreliosis, but rather was an important piece of evidence that Lyme borreliosis has been neglected in China. The recent occurrence of emerging or reemerging tick-borne diseases with high disease severity have the possibility to led to the neglect of research on Lyme borreliosis in recent years, e.g. the main endemic areas of severe fever with thrombocytopenia syndrome (SFTS) in Central China region and of tick-borne encephalitis (TBE) in Northeast China region[Citation33,Citation34], have been shown a significant decrease of the reported number of Lyme borreliosis cases, while South China region with few endemic areas of SFTS and TBE was not shown a downward trend. In addition, the lag in publication of related papers may partly contribute to the perceived decline in the number of Lyme borreliosis cases.
Our results indicated that about 24% of Lyme borreliosis patients in China had arthritis, a late disease manifestation, which was much higher than the 2%–7% in Europe, although the main genospecies in China and Europe are the same[Citation1]. Lack of early intervention and treatment for Lyme borreliosis might be the reason for the high number of arthritis symptoms in Lyme borreliosis cases in China[Citation35], despite the therapeutic being widely available[Citation36]. Almost 60% of the patients were forest workers, farmers and herdsmen, who are more exposed to tick bites during their professional activities. According to the studies in Europe, agricultural and forestry workers have a higher B. burgdorferi seroprevalence than general population, which indicates high proportion of forest workers, farmers and herdsmen could overestimate the seroprevalence in the population[Citation23]. Therefore, we performed a comprehensive risk classification from different data sources as a proxy for risk at different locations, rather than directly using positive rates as an indicator of risk. Selecting tick species with the highest positive rate but with low population density as a proxy for B. burgdorferi risk assessment may overestimate the risk. Our modelling data showed that majority of tick species with the highest positive rate of B. burgdorferi infection were also the most predominant tick species in the regions [89.3% (42/47) locations], and the remaining 10.7% (5/47) locations, the tick species with the highest positive rate were also the subdominant tick species, which indicates a very low degree of overestimation for the risk estimation of B. burgdorferi infection in this study. In addition, livestock and wild animals are not the direct source of B. burgdorferi infection of humans or other animals, but only serve as a reference for the prevalence of B. burgdorferi in a region. Therefore, the species with the highest positive rate (usually the ones prone to tick bites) are more likely to reflect the actual prevalence of B. burgdorferi in this region.
Many tick species have been associated with Lyme borreliosis, but members of the genus Ixodes are considered the primary vectors and are the most common ticks known to transmit the pathogen to humans[Citation1,Citation2]. Among them, I. persulcatus, mainly distributed in northeastern and northwestern China, is considered to be the main vector of Lyme borreliosis in Asia and parts of Eastern Europe[Citation7]. In southern China, another Ixodes tick with a high positive rate of B. burgdorferi and widespread distribution is I. granulatus[Citation8], which is also considered to be a major vector of Lyme borreliosis in China[Citation11]. These two Ixodes are thought to be predominantly found in forests, which often have environments suitable for high tick density[Citation20,Citation34]. When humans enter forest areas for agricultural or forestry activities such as mushroom picking and tree felling, they are easily exposed to these Ixodes ticks and infected with B. burgdorferi[Citation34]. Hyalomma asiaticum and Ha. concinna also have high positive rates of B. burgdorferi. Haemaphysalis concinna is distributed in the northern and central regions, living in forests and shrubs and often parasitic on domestic animals, and the possibility of contact with rural residents is extremely high[Citation37,Citation38]. In a survey of Cangxi County, Sichuan Province, 53.8% of residents had been bitten by Ha. concinna[Citation38]. Hyalomma asiaticum also frequently bite humans, given that it is the most important vector of Crimean-Congo hemorrhagic fever in China[Citation39]. This tick is mainly found in the semi-desert areas of northwestern China[Citation37,Citation39]. A large number of forestry workers are in direct contact with this tick due to extensive afforestation activities[Citation40]. Therefore, we speculated that these two ticks are also potential vectors of Lyme borreliosis. In addition, the difference in the positive rates of ticks in different regions was more likely to be caused by the tick species because the positive rates of I. persulcatus in Northeast China and the Xinjiang Uygur Autonomous Region were both high, while the positive rates of most other tick species in these two regions were low. The geographic difference in the positive rate of B. burgdorferi infection in wild animals is likely related to the composition of wild animals surveyed in different environments, given that wild animals usually affect tick populations and have different competencies to B. burgdorferi infection[Citation41].
The prediction map of the BRT model showed that the potential risk area was much larger than the area observed in the survey, especially in the Central China region, which might be caused by insufficient surveillance. The four high-risk areas identified by this study surround four mountain areas, which were the Changbai and the Greater Khingan Mountains, Tianshan and Altai Mountains, Wuling Mountains, and Qinling Mountains. Previous studies have found that I. persulcatus, a tick species with a high infection rate of B. burgdorferi, is widely distributed in the first two high-risk areas[Citation37], while two other tick species with high infection rates of B. burgdorferi, including I. granulatus and Ha. concinna, are widely located in the latter two high-risk areas[Citation19,Citation20]. Although featured by different ecological niches, all representing indicate higher environmental suitability for the propagation of tick vectors and wild animals. Vegetation-related variables such as NDVI and closed-canopy woodland were almost positively correlated at both stages of the model, which was in line with our expectations, as abundant vegetation is suitable for B. burgdorferi and its vectors and hosts[Citation25,Citation42]. In the stage 1 model, closed canopy woodland was the most important factor, indicating forests are important for B. burgdorferi to establish ecological cycles[Citation25]. However, this factor was less important in the stage 2 model, possibly because more specific habitats including composition and population size of different vectors and animal hosts have a greater impact on whether there was a high risk of B. burgdorferi. Climate factors played a more important role in the model via only human data than the model that only via tick data. This might be due to the fact that different climatic regions have different ecological environments, which are closely related to human activities such as different types of agricultural production. Our previous studies have also indicated that agricultural production plays an important role in the occurrence of tick-borne diseases [Citation33,Citation34].
There are of course limitations to any ecological study of this kind. A report of absence may be not published due to the lack of positive results, and the efforts and quality of detection and report of B. burgdorferi infection can be variable by region. For example, a positive serological test can be due to a previous infection, and even nucleic acid testing methods may have errors, which can affect risk prediction[Citation6]. Furthermore, the included data spanned a long duration of more than 30 years, with some of the historical documents inevitably suffering from flaws of changing criteria or standards that were applied in the curated data, all might influence the prediction of risk.
In conclusion, we applied an integrated approach to identify active B. burgdorferi foci in China. Compared with previous studies where B. burgdorferi hazard has been always evaluated using one single method and focusing on limited areas, this is the first attempt to adopt an integrated approach at the national level to include tick vectors, animals, and human beings. Areas predicted as suitable but with no records of disease might also be prudent to check for disease occurrence. Ultimately, to quantitatively assess the risk of Lyme borreliosis to humans, additional factors including density of predominant vector ticks, the bite rate and transmission capacity of vector ticks, the species and density of animal hosts, as well as the human behaviours and health care awareness, will be needed in future studies.
Supplemental Material
Download MS Word (5 MB)Acknowledgements
LQF, WL, SIH conceived and designed the study. TLC, BGJ, QX, and JJC contributed to data collection. TLC, QX, YQZ, CLL, and YJT contributed to data analysis under the supervised by LQF, WL and SIH. LQF, WL, TLC, BGJ, and YY contributed to the writing of the report. All the authors read and approved the final manuscript.
Disclosure statement
No potential conflict of interest was reported by the author(s).
Data availability statement
The datasets used and/or analyzed during the current study are available from the corresponding author on reasonable request.
Additional information
Funding
References
- Stanek G, Wormser GP, Gray J, et al. Lyme borreliosis. Lancet. 2012;379(9814):461–473.
- Kullberg BJ, Vrijmoeth HD, van de Schoor F, et al. Lyme borreliosis: diagnosis and management. Br Med J. 2020;369:m1041.
- Bobe JR, Jutras BL, Horn EJ, et al. Recent progress in Lyme disease and remaining challenges. Front Med. 2021;8:666554.
- Steere AC, Strle F, Wormser GP, et al. Lyme borreliosis. Nat Rev Dis Primers. 2016;2:16090.
- Stanek G, Fingerle V, Hunfeld KP, et al. Lyme borreliosis: clinical case definitions for diagnosis and management in Europe. Clin Microbiol Infect. 2011;17(1):69–79.
- Wolcott KA, Margos G, Fingerle V, et al. Host association of Borrelia burgdorferi sensu lato: A review. Ticks Tick Borne Dis. 2021;12(5):101766.
- Kurokawa C, Lynn GE, Pedra JHF, et al. Interactions between Borrelia burgdorferi and ticks. Nat Rev Microbiol. 2020;18(10):587–600.
- Eisen L. Vector competence studies with hard ticks and Borrelia burgdorferi sensu lato spirochetes: A review. Ticks Tick Borne Dis. 2020;11(3):101359.
- Hussain S, Hussain A, Aziz U, et al. The role of ticks in the emergence of Borrelia burgdorferi as a zoonotic pathogen and its vector control: a global systemic review. Microorganisms. 2021;9(12):2412.
- Ai CX, Wen YX, Zhang YG, et al. Clinical manifestations and epidemiological characteristics of Lyme disease in Hailin county, Heilongjiang Province, China. Ann N Y Acad Sci. 1988;539:302–313.
- Zhang ZF, Wan KL, Zhang JS, et al. Studies on epidemiology and etiology of Lyme disease in China. Chin J Epidemiol. 1997;1:4. (in Chinese).
- Yu Z, Wang H, Wang T, et al. Tick-borne pathogens and the vector potential of ticks in China. Parasit Vectors. 2015;8:24.
- Ni XB, Jia N, Jiang BG, et al. Lyme borreliosis caused by diverse genospecies of Borrelia burgdorferi sensu lato in northeastern China. Clin Microbiol Infect. 2014;20(8):808–814.
- Hao Q, Hou X, Geng Z, et al. Distribution of Borrelia burgdorferi sensu lato in China. J Clin Microbiol. 2011;49(2):647–650.
- Xuefei D, Qin H, Xiaodi G, et al. Epidemiological and clinical features of three clustered cases co-infected with Lyme disease and rickettsioses. Zoonoses Public Health. 2013;60(7):487–493.
- Hao Q, Du P, Zhang W, et al. Genomic characteristics of Chinese Borrelia burgdorferi isolates. PLoS One. 2016;11(4):e0153149.
- Fang LQ, Liu K, Li XL, et al. Emerging tick-borne infections in mainland China: an increasing public health threat. Lancet Infect Dis. 2015;15(12):1467–1479.
- Wu XB, Na RH, Wei SS, et al. Distribution of tick-borne diseases in China. Parasit Vectors. 2013;6:119.
- Yang X, Gao Z, Zhou T, et al. Mapping the potential distribution of major tick species in China. Int J Environ Res Public Health. 2020;17(14):5145.
- Yang X, Gao Z, Wang L, et al. Projecting the potential distribution of ticks in China under climate and land use change. Int J Parasitol. 2021;51(9):749–759.
- Dou X, Lyu Y, Jiang Y, et al. Seroprevalence of Lyme disease and associated risk factors in rural population of Beijing. Int J Clin Exp Med. 2015;8(5):7995–7999.
- Masuzawa T. Terrestrial distribution of the Lyme borreliosis agent Borrelia burgdorferi sensu lato in east Asia. Jpn J Infect Dis. 2004;57(6):229–235.
- De Keukeleire M, Vanwambeke SO, Cochez C, et al. Seroprevalence of Borrelia burgdorferi, Anaplasma phagocytophilum, and Francisella tularensis infections in Belgium: results of three population-based samples. Vector Borne Zoonotic Dis. 2017;17(2):108–115.
- Bisanzio D, Fernández MP, Martello E, et al. Current and future spatiotemporal patterns of Lyme disease reporting in the northeastern United States. JAMA Netw Open. 2020;3(3):e200319.
- Gardner AM, Pawlikowski NC, Hamer SA, et al. Landscape features predict the current and forecast the future geographic spread of Lyme disease. Proc Biol Sci. 2020;287(1941):20202278.
- Rogers DJ, Randolph SE, Snow RW, et al. Satellite imagery in the study and forecast of malaria. Nature. 2002;415(6872):710–715.
- Barbet-Massin M, Jiguet F, Albert CH, et al. Selecting pseudo-absences for species distribution models: how, where and how many? Methods Ecol Evol. 2012;3(2):327–338.
- VanDerWal J, Shoo LP, Graham C, et al. Selecting pseudo-absence data for presence-only distribution modeling: How far should you stray from what you know? Ecol Model. 2009;220(4):589–594.
- Crockett PF, Keough MJ. Ecological niches of three abundant Caulerpa species in port Phillip Bay, southeast Australia. Aquat Bot. 2014;119:120–131.
- Kraemer MU, Sinka ME, Duda KA, et al. The global distribution of the arbovirus vectors Aedes aegypti and Ae. albopictus. Elife. 2015;4:e08347.
- Hijmans RJ, Phillips S, Leathwick J, et al. dismo: species distribution modeling. R package version 1.3-3. 2020 [cited 2020/09/01]. Available from: https://cran.r-project.org/package = dismo.
- Brandon G, Bradley B, Jay C. gbm: generalized boosted regression models. R package version 2.1.8. 2020 [cited 2020/09/01]. Available from: https://CRAN.R-project.org/package = gbm.
- Miao D, Liu MJ, Wang YX, et al. Epidemiology and ecology of severe fever with thrombocytopenia syndrome in China, 2010‒2018. Clin Infect Dis. 2020;73(11):e3851–e3858.
- Sun RX, Lai SJ, Yang Y, et al. Mapping the distribution of tick-borne encephalitis in mainland China. Ticks Tick Borne Dis. 2017;8(4):631–639.
- Arvikar SL, Steere AC. Diagnosis and treatment of Lyme arthritis. Infect Dis Clin North Am. 2015;29(2):269–280.
- Torbahn G, Hofmann H, Rücker G, et al. Efficacy and safety of antibiotic therapy in early cutaneous Lyme borreliosis: a network meta-analysis. JAMA Dermatol. 2018;154(11):1292–1303.
- Zhao GP, Wang YX, Fan ZW, et al. Mapping ticks and tick-borne pathogens in China. Nat Commun. 2021;12(1):1075.
- Sun JG, Li KJ, Wei H, et al. Studies of Haemaphysalis concinna outbreaks in Cangxi County of Sichuan province. Veterinary Science in China. 2006;36(6):719–723. (in Chinese).
- Zhang Y, Shen S, Fang Y, et al. Isolation, characterization, and phylogenetic analysis of two new Crimean-Congo hemorrhagic fever virus strains from the northern region of Xinjiang province, China. Virol Sin. 2018;33(1):74–86.
- Xiao Y, Xiao Q. The ecological consequences of the large quantities of trees planted in northwest China by the Government of China. Environ Sci Pollut Res Int. 2019;26(32):33043–33053.
- Mysterud A, Stigum VM, Jaarsma RI, et al. Genospecies of Borrelia burgdorferi sensu lato detected in 16 mammal species and questing ticks from Northern Europe. Sci Rep. 2019;9(1):5088.
- Talbot B, Slatculescu A, Thickstun CR, et al. Landscape determinants of density of blacklegged ticks, vectors of Lyme disease, at the northern edge of their distribution in Canada. Sci Rep. 2019;9(1):16652.