ABSTRACT
Rapid social, economic, and environmental changes in the northern territories of Canada have raised concerns about potentially increasing levels of chronic disease. This concern prompted us to compare multimorbidity prevalence in Canada between the territories and provinces. We analyzed Canadian Community Health Survey data for 2013/14. We defined multimorbidity, the outcome, as having 3 or more chronic conditions and used survey-weighted multivariable logistic regression for comparisons between territories and provinces. We found a prevalence of multimorbidity in Canada of 14.0% (95% CI: 13.6, 14.3). We could not find significant difference in multimorbidity prevalence between the territories and provinces of Canada overall; however, the territories tended to have lower prevalence estimates than provinces for multimorbidity (adj-OR = 0.88; 95% CI: 0.74–1.04). Sensitivity analyses from propensity score analyses had similar conclusions. Effect modification analyses identified lower multimorbidity in territories versus provinces among households without a post-secondary graduate (adj-OR = 0.46; 95% CI: 0.34–0.61 for northern residence), males (adj-OR = 0.71; 95% CI: 0.54–0.93), and ages 12–29 years (adj-OR = 0.63; 95% CI: 0.39–0.99). Caution is needed in interpreting the results in light of representativeness of CCHS in northern populations of Canada.
Introduction
Multimorbidity is defined as two or more co-occurring chronic conditions without an index condition[Citation1]. Multimorbidity is common among adults, with a general population prevalence estimated to be between 17.3 5 to 71.8%[Citation2]. Multimorbidity reduces physical and social functioning, increases utilization of healthcare services, and leads to higher personal and social costs [Citation3–Citation6]. In Ontario, multimorbidity was estimated to account for 86% of healthcare spending totalling over $26B annually[Citation7]. Healthcare policy and practice are rapidly shifting to patient-centred and coordinated care approaches, in part prompted by the challenges of multimorbidity [Citation2,Citation8–Citation10]. Many unanswered questions about incidence, prevalence, prevention, and intervention remain [Citation2,Citation8,Citation10,Citation11]. However, core risk factors for multimorbidity have been identified, including advanced age, lower socioeconomic status, and female sex[Citation10]. Other risk factors, such as tobacco use, physical inactivity, alcohol consumption, and urban living have also been described in various populations with mixed results[Citation2]. Canadian studies have produced evidence regarding these risk factors for multimorbidity, and have highlighted the role of socioeconomic disparities in multimorbidity [Citation3,Citation11–Citation13]. Other circumpolar countries have investigated multimorbidity prevalence, patterns, predictors, and prescribing practices, with varying results, and prevalence estimates ranging from 21.6% to 55%, depending on participants, data source, and included conditions [Citation14–Citation17].
Northern Canada, which includes the Northwest Territories (NWT), Yukon Territory (YT), and Nunavut (NU), has experienced a slower epidemiological transition from infectious to chronic disease than the rest of Canada [Citation18,Citation19]. However, a higher prevalence of risk factors for chronic disease in northern Canada suggests that multimorbidity may be an emerging phenomenon [Citation18,Citation20–Citation22]. Estimates of the burden of multimorbidity and the factors associated with this burden can help inform needed primary care reform in northern Canada [Citation23]. Two studies to date have included comparisons of multimorbidity between Canadian provinces and territories [Citation18,Citation24]. Deering et al. found a higher prevalence of risk factors for chronic disease in northern compared to southern Canada, although at the time of their study (years 2000/01 and 2005) the higher prevalence of risk factors may not have translated to higher prevalence of chronic disease in northern Canada[Citation18]. Their analysis was adjusted for age and sex yet did not consider risk factors for multimorbidity such as smoking, diet, physical activity, or socioeconomic status [Citation18]. Deering et al. predicted a rise in chronic disease in northern Canada, with implications for care, and called for continued monitoring [Citation18]. Feely et al. used the Canadian Chronic Disease Surveillance System (populated by administrative hospital discharge and physician claims data) to analyze multimorbidity across Canadian provinces and territories. They found slightly higher age-standardized rates of three or more chronic conditions in the Yukon and Nunavut compared to Canada overall with higher than average increases in all three territories between 2001/02 and 2011/12[Citation24]. However, their estimates are were also not adjusted for risk factors such as smoking, diet, physical activity, or socioeconomic status[Citation24].
The equivocacy of findings coupled with predictions of epidemiological change and known disparities in healthcare access, warrant research into multimorbidity in northern Canada. Recent CCHS data for 2013/14 enables an updated analysis of chronic disease burden between the territories and provinces of Canada. The primary aim of this study was to answer the following question: What is the prevalence of multimorbidity in northern compared to southern Canada? A higher prevalence of multimorbidity in northern Canada, after adjustment for known risk factors, was hypothesized. This study describes multimorbidity prevalence and associated factors in the general Canadian population using nationally representative data and modern regression methods appropriate for complex surveys to compare territories and provinces in terms of multimorbidity prevalence.
Methods
Data source, design, and study population
Data from the Canadian Community Health Survey (CCHS) 2013/14 Public Use Microdata File (PUMF) was used for this cross-sectional study. The study population for CCHS includes Canadians age ≥12 years through a multistage stratified cluster random sampling strategy[Citation25]. Survey weights are provided with the PUMF to produce accurate point estimates based on the complex survey design. However, bootstrap weights and necessary design information (strata and primary sampling units) are not provided with the CCHS PUMF for privacy reasons, potentially reducing users’ ability to estimate variance precisely.[Citation25]
Analytic sample and study variables
All CCHS participants were included. The primary outcome, multimorbidity, was derived from 14 chronic condition questions (“do you have <condition>?”) asked of all participants. Chronic conditions included in the definition of multimorbidity: asthma, arthritis (excluding fibromyalgia), back problems (excluding fibromyalgia/arthritis), high blood pressure, migraine headaches, chronic obstructive pulmonary disorder (COPD), diabetes, heart disease, cancer, stomach or intestinal ulcers, urinary incontinence, bowel disorder, as well as two mental health conditions (mood disorder and anxiety disorder). These conditions fit with the literature and the recommendation by Fortin et al. to use at least 12 candidate conditions [Citation8,Citation9]. Indicator variables for each question were set to 1:(“yes”) and 0:(“no”, “don’t know”, “not applicable”, “not stated”, “refused”). The sum of these indicator variables was dichotomized into the outcome variable: “< 3 conditions” versus “3+ conditions”[Citation26].
The dichotomous exposure variable for this study was residence in northern Canada during data collection: residence in a territory of Canada (Yukon, Northwest Territories, or Nunavut; north = 1) or in a province of Canada (north = 0). Covariates were identified based on Canadian literature examining chronic disease using CCHS data [Citation3,Citation12,Citation18,Citation20,Citation21]. Covariates that potentially confound the relationship between residence in northern Canada and multimorbidity were grouped as follows: age group (12–17, 18–24, 25–34, 35–44, 45–54, 55–64, 65–74, 75+), sex (male, female), leisure physical activity index (active/moderately active, inactive), total household annual income (<$20,000, $20,000–39,999. $40,000–59,999, $60,000–79,999, $80,000+), type of smoker (current, former, never), highest household education (post-secondary graduate, non-post-secondary graduate), alcohol consumption (regular, occasional, not in the last 12 months), and daily consumption of fruits and vegetable (<5 servings, 5+ servings)[Citation27]. Participants with invalid covariate values (“don’t know”, “refused”, “not stated”, or “not applicable”) were excluded from the analytic sample. displays participant flow in/out of the analytic sample (n = 110,924).
Figure 1. Flow diagram of analytic sample inclusion and exclusion by residence North (Territories) and South (Provinces)
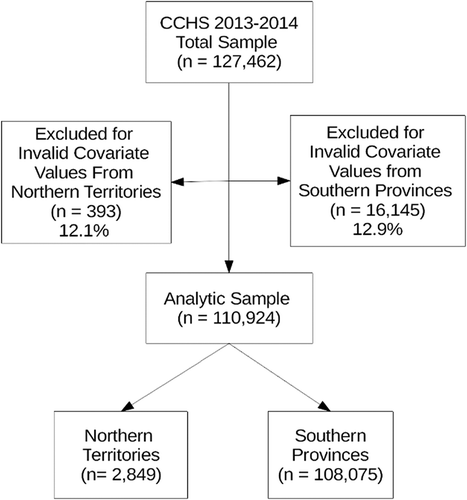
Statistical analysis
Descriptive analysis
Analytic sample characteristics are described through frequency and percent of participants in the covariate groups stratified by residence in territories or provinces and compared using the standardized mean difference (SMD) to assess covariate balance[Citation28]. Analytic sample characteristics were also stratified by the outcome variable, multimorbidity status, and their statistical association with the outcome assessed through the Rao-Scott Chi-square test[Citation29].
Statistical inference
Survey-weighted multivariable logistic regression estimated differences in multimorbidity prevalence after adjusting for confounding, with results presented as odds ratios (OR) and confidence intervals (CI). All covariates were entered into the main effects model simultaneously based on their presumed effect. All covariates were significant, hence none were removed. Potential effect modification of northern residence by age, sex, income, or education was explored. Interactions between covariates that might better explain the relationship between exposure and outcome were considered, with a focus on sex-based interactions. As recommended, male and female sub-groups were analyzed separately to identify differences in factors associated with multimorbidity[Citation8]. The main effects model was evaluated through the weighted area under the receiver operating characteristics curve (AUC) and the Archer-Lemeshow goodness-of-fit test[Citation30].
Sensitivity analyses
First, two age-restricted samples (age 18 years and over and age 35 years and over) were used to account for low cell counts among ages 12–17 years (see ) and because urinary incontinence and COPD were not measured for those under 25 years and 35 years, respectively. Next, a series of interaction models assessed covariate interactions that may alter the adjusted odds ratio for northern residence from the main effects model.
Third, propensity score (PS) methods were employed to balance the exposure and control groups through (a) PS matching and (b) PS weighting of the analytic sample [Citation28,Citation31]. All covariates were used to create propensity scores in both cases. The PS for this study was the predicted probability of being a resident of the northern territories based on the covariates in main analysis. Improvements in the balance of treatment and exposure groups on covariates was assessed through the reduction in SMD between the unmatched and PS-matched samples[Citation28]. For the matching approach, a 1:10 nearest neighbour (logit distance)-matched-with-replacement analytic sample was assembled for territorial and provincial residents[Citation32]. Two propensity score estimation methods were used for the weighting approach: logistic regression and ensembling through a 5-fold cross-validated super learner[Citation33]. In the logistic regression PS model, all variables used in the main effects analysis were included. In the super learning PS method, all covariates were entered into the ensembling algorithm with three candidate algorithms: generalized linear model (GLM) (main effects only), GLM stepwise interaction, and recursive partitioning with pruned regression trees using cross-validated mean square error (CV-MSE) to evaluate predictive performance[Citation34].
Finally, a multiple imputation analysis was conducted using a larger set of observations where participants with missing covariate values who were removed from the previous analysis were included. Missing covariate values for six covariates were multiply imputed in 20 datasets, and analyzed using appropriate methods[Citation35]. Data analyses were conducted in R v.3.4.4[Citation36].
Results
Descriptive analysis: study population characteristics and multimorbidity
Bivariable comparisons of the exposure and covariates with the outcome suggest that all study variables are significantly associated with multimorbidity (). The estimated prevalence of multimorbidity in Canada during 2013/14 was 14% (N = 3,732,160 people): 14%(N = 3,722,786) in the provinces, and 12% (N = 9,374) in the territories (). A higher proportion of persons with multimorbidity are female, older, consuming less than 5 servings of fruits and vegetables per day, consuming alcohol occasionally or not in the last 12 months, current and former smokers, and physically active (). A large proportion (80%) of the population without multimorbidity report “post-secondary graduate” as highest household education level and approximately half (47%) report household income over $80,000/year ().
Table 1. Study sample characteristics stratified by multimorbidity status with survey-weighted proportions and associations: Canadian Community Health Survey, 2013/14
Table 2. Model estimates from survey-weighted logistic regression of multimorbidity prevalence (3+ conditions): Canadian Community Health Survey, 2013/14
Main analyses: survey-weighted logistic regression
The crude odds of multimorbidity was 18% lower among residents of territories than provinces (OR = 0.82, 95% CI: 0.70–0.96). After adjusting for numerous risk factors for multimorbidity there appeared to be a lower prevalence of multimorbidity in territories than provinces, although not statistically significant (aOR = 0.88, 95% CI: 0.74–1.04) (). Females, older persons, current or former smokers, the physically active, and those who consume alcohol occasionally or not in the last year had higher odds of multimorbidity after adjusting for covariates (). Eating five or more servings of fruits/vegetables, having higher levels of household income, or having highest household education of post-secondary graduate, were all associated with lower odds of multimorbidity after adjusting for covariates (). The final model discriminated between persons with and without multimorbidity 28% better than chance (AUC = 0.78). Archer-Lemeshow goodness-of-fit test did not show evidence of lack of model fit (p = 0.3).
Effect modification, interaction, and sub-group analyses
Effect modification models showed lower odds of multimorbidity in territories than provinces for ages 12–29 years (aOR = 0.63; 95% CI: 0.39–0.99), males (aOR = 0.71; 95% CI: 0.54–0.93), and people in households with a post-secondary graduate (aOR = 0.46; 95% CI: 0.34–0.61) (). Females had larger aOR in the territories (aOR = 2.17, 95% CI: 1.54–3.05) than the provinces (aOR = 1.51, 95% CI: 1.41–1.62) (). The increased odds of multimorbidity for females compared to males was larger in younger years and became smaller at older ages (). Sex-specific models showed differences in adjusted odds ratios between males and females for effects of northern residence and age group, with slight differences for household education, physical activity, alcohol consumption, smoker type and fruit & vegetable consumption ().
Table 3. Effect modification, covariate interaction, and sub-group model effect estimates for northern residence on multimorbidity prevalence (3+ Conditions): Canadian Community Health Survey, 2013/14
Table 4. Sex-specific and modified sex effect model estimates from survey-weighted logistic regression of multimorbidity prevalence (3+ chronic conditions): Canadian Community Health Survey, 2013/14
Sensitivity analyses
Models including interaction terms for sex by age, sex by smoking, age by alcohol consumption, age by household income, and alcohol by smoking, all returned northern residence estimates similar to the main analysis (). Restricting the analysis to ages ≥ 35 years yielded the same northern residence adjusted OR of 0.88 (95% CI: 0.59–1.35) as the main analysis (aOR = 0.88), as did restricting to ages ≥ 18 years with aOR = 0.80 (95% CI: 0.50–1.27) (.).
Propensity score analyses
A substantial improvement was observed in covariate balance in the PS-matched sample (all SMD<0.1) compared to the original analytic sample (several SMD>0.25). PS-adjusted effect estimates for residence in territories on odds of multimorbidity varied slightly from the main analysis, depending on PS and outcome analysis methods, although the conclusion from these models did not change ().
Table 5. Propensity score-adjusted analyses of multimorbidity prevalence (3+ Conditions) between Territories and Provinces: Canadian Community Health Survey, 2013/14
Missing data analysis
Six covariates were subject to multiple imputation leaving an analytic sample of n = 127,230 . After imputation, the covariate-adjusted odds of multimorbidity was significantly lower within territories compared to the provinces (aOR = 0.83; 95% CI: 0.71–0.98) (results not shown).
Discussion
This study hypothesized a higher prevalence of multimorbidity in northern Canada. Instead, lower prevalence of multimorbidity in the territories compared to provinces is apparent, although not statistically significant (). The matched analysis suggested no difference between territorial and provincial residents. The logistic regression PS analysis confirmed the results from the main analysis (). The super learner-based PS analysis found a significantly lower prevalence of multimorbidity in territories than provinces, which may be owing to better covariate balance between territories and provinces, although the upper confidence limit was close to null (). A series of covariate interaction terms, when individually added to the final model, did not alter the main conclusion of no significant difference between territories and provinces () The missing data analysis further confirmed the results from the main analysis (results not shown). The results are in relative harmony that there is no difference in the prevalence of multimorbidity between territories and provinces.
To compare our Canadian prevalence estimate of 14% to other circumpolar states we provide a brief selection of prevalence estimates below. In Iceland, multimorbidity (2+ conditions) prevalence was estimated at 35% using primary care data (n = 221,822)[Citation14]. Analysis of a prospective rural northern cohort in Norway identified multimorbidity (2+ conditions) prevalence of 30.8% (n = 20,365)[Citation15]. In Sweden, a study including people ages 77 to 100 years (n = 1,099) found a prevalence of multimorbidity (2+ conditions) of 55%. based on medical records[Citation16]. In Denmark, a cross-sectional study of capital region residents (n = 1,397,173) found a prevalence of multimorbidity (2+ conditions) of 20.6%[Citation17]. These studies used a less strict definition of multimorbidity (2+ conditions) than our study (3+ conditions), which is the most obvious reason our prevalence estimates are lower; other reasons being differences in data source, participants, and conditions considered.
Demographic factors: age and sex
As expected, a strong relationship between age and multimorbidity was observed (). Finer age groups were retained to produce a more complete picture of the increasing prevalence of multimorbidity with advancing age, as recommended[Citation8]. For females, 52% higher adjusted odds of multimorbidity is consistent with the literature [Citation3,Citation10,Citation11]. Higher age-specific adjusted OR of multimorbidity for females compared to males at younger ages () is a novel finding and may be related to higher prevalence of mood and anxiety disorders among females in at those ages in Canada[Citation37]. The finding of lower multimorbidity prevalence among males in territories compared to males in provinces is also a new finding. Similarly, higher adjusted odds ratios for females compared to males in territories than provinces is also a novel finding (). These findings require more in-depth exploration of specific conditions involved
Socioeconomic status (SES): household income and household education
This study found a linear relationship between increasing income and lower prevalence of multimorbidity after adjusting for other factors ( and ), which agrees with existing research [Citation3,Citation8,Citation11,Citation38]. How income affects multimorbidity is unclear, involving multiple potential pathways [Citation2,Citation9]. One Canadian study suggests that the effect of income inequality can be attributed primarily to income itself, as opposed to the risk factors mentioned previously[Citation3]. Those findings contradict the argument that behind socioeconomic gradients in health are a higher prevalence of risky health behaviours among the poor, such as smoking and alcohol consumption, which are posited as the actual cause of the socioeconomic gradient observed in social epidemiological studies[Citation39].
Behavioural risk factors: smoking, alcohol consumption, and physical activity
Smoking was strongly associated with multimorbidity, whether current or former (). Physical inactivity, which is generally associated with a higher prevalence of multimorbidity, was related to lower multimorbidity in our study (). However, only leisure physical activity was examined, which limits the generalizability of these results to leisure physical activity. For alcohol consumption, occasional and abstention (not in the last 12 months) both had higher adjusted odds of multimorbidity when compared to those with regular alcohol consumption (). This result aligns with an analysis of the Twenty-07 cohort, which also found an increased risk among non-drinkers compared to regular and excess drinkers [Citation40]. Han et al. (2012), noted a lower prevalence of multimorbidity among binge compared to non-binge drinkers age≥50, describing the “sick-quitter” hypothesis and survivor bias as selecting out unhealthy binge drinkers over time, leaving a group of healthy drinkers compared with unhealthy non-drinkers[Citation41], which may be the case in our study.
Strengths and limitations
Previous analyses showing lower but increasing chronic disease prevalence in northern Canada were not adjusted for known risk factors beyond age and sex [Citation18,Citation21,Citation24]. Our results contradict predictions of rising chronic disease among northern populations made in previous studies; however, we did not use a longitudinal design so could not test for trend. We found a higher proportion of the population with 3+ chronic conditions (14%) than Roberts et al. (3.9%) using CCHS data, although they included fewer chronic conditions [Citation12]. Different age inclusion, outcome definition, and analysis methods preclude direct comparison with previous studies. This study has specific strengths, such as the use of a prospective statistical analysis plan [Citation42], with a directed acyclic graph (DAG) to select analysis variables grounded in the literature and a priori assumptions [Citation2,Citation43]. As recommended for multimorbidity prevalence studies [Citation8]: our data source was the general population, more than 12 chronic conditions were considered, fine age bands were employed, and we reported sex-specific results. The application of survey-weighted multivariable logistic regression to a large sample with adjustment for individual risk factors provide a solid foundation for study results. The main analysis conclusion was robust to multiple sensitivity analyses including PS as an alternative method for adjustment for confounding [Citation28]. The super learning approach to PS estimation enabled construction of a better prediction model for the PS [Citation44], with a very low CV-MSE of 0.025 (SE = 0.0004). Multiple imputation demonstrated that the main analysis results were not sensitive to missing covariate data. The low cell count for ages 12–17 in the north with multimorbidity was a concern (). We conducted a sensitivity analysis removing this age group (; Sub-Group Analysis), and the conclusion remained unchanged compared to the main analysis. Another limitation is the small cell sizes in northern territories for some covariates.
In addition to standard limitations for cross-sectional health surveys, this study has particular limitations related to the representativeness of CCHS for territories, related to phone coverage and language[Citation45]. Explicitly, 2% of the Canadian population is not represented in the CCHS, including persons living in First Nations communities; however this number grows in the north[Citation45]. Language barriers may create selection bias in a study of multimorbidity, which generally occurs among older persons, particularly among northern residents, whose elders may be more likely to speak Indigenous languages not used by CCHS interviewers[Citation25]. Moreover, access to physicians to diagnose conditions may also create a case-ascertainment bias where those in provinces are more likely to be diagnosed with a condition. We could not address these problems analytically so results must be interpreted with caution. Finally, because prevalence measures both the incidence and duration of disease, there is a possibility that differences in survival between the territories and the provinces influence our estimates, with known higher age-standardized mortality in the territories[Citation46]. However, for Nunavut the leading cause of death is suicide, which would not be captured by our estimates, and injury also contributes a greater proportion to the causes of mortality in northern territories [Citation46,Citation47]. How different mortality patterns may affect multimorbidity estimates was beyond the scope of our project, but should be considered in future research.
Conclusion
This study found a prevalence of multimorbidity (3+ conditions) of 14% in Canada (over 3.7 M people). Northern Canada did not have a higher prevalence of multimorbidity than the provinces in our main analysis, which was confirmed through multiple sensitivity analyses. However, lower prevalence of multimorbidity in the territories compared to provinces among those in households with less than post-secondary education, males, and youth are novel findings.
Other circumpolar states expecting an epidemiological transition to chronic disease in their northern-most populations may find these estimates and our methodology of interest. While multimorbidity prevalence estimates are not directly comparable with other circumpolar countries, given variations in data sources, conditions considered, and definitions of multimorbidity, our methods may be of particular interest to researchers investigating multimorbidity prevalence using survey data. Use of a larger sample by combining several CCHS cycles, and using the confidential CCHS microdata file, including population origin and specific territory, rather than PUMF, would enrich future analyses based on our preliminary findings.
Author Contributions
CAB conceptualized and designed study. CAB acquired and analyzed data, and interpreted the results with advice from MEK. CAB wrote the manuscript with critical review and commenting from MEK. Both authors were involved in the approval of the final manuscript.
Acknowledgments
The authors gratefully acknowledge comments on previous drafts from course participants at the University of British Columbia, School of Population and Public Health (504/007; Winter 2018).
Disclosure statement
No potential conflict of interest was reported by the authors.
Additional information
Funding
References
- Van Den Akker M, Buntinx F, Knottnerus JA. Comorbidity or multimorbidity: what’s in a name? A review of literature. Eur J Gen Pract. 1996;2(2):65–11.
- Xu X, Mishra GD, Jones M. Evidence on multimorbidity from definition to intervention: an overview of systematic reviews. Ageing Res Rev. 2017;37:53–68.
- Mondor L, Cohen D, Khan AI, et al. Income inequalities in multimorbidity prevalence in Ontario, Canada: a decomposition analysis of linked survey and health administrative data. Int J Equity Health. 2018;17:90–103.
- Chang VC, Do MT. Risk factors for falls among seniors: implications of gender. Am J Epidemiol. 2015;181(7):521–531.
- Picco L, Achilla E, Abdin E, et al. Economic burden of multimorbidity among older adults: impact on healthcare and societal costs. BMC Health Serv Res. 2016;16(1):1–12.
- Glynn LG, Valderas JM, Healy P, et al. The prevalence of multimorbidity in primary care and its effect on health care utilization and cost. Fam Pract. 2011;28(5):516–523.
- Thavorn K, Wodchis W, Maxwell C, et al. Economic burden of multimorbidity in Ontario’s health care system. Poster session presented at Health System Performance Research Network Symposium, October 22, Toronto. 2013.http://hsprn.ca/uploads/files/Thavorn_Economic Burden_(2013_05_17)_FINAL.pdf
- Fortin M, Stewart M, Poitras ME, et al. A systematic review of prevalence studies on multimorbidity: toward a more uniform methodology. Ann Fam Med. 2012;10(2):142–151.
- Prados-Torres A, Calderón-Larrañaga A, Hancco-Saavedra J, et al. Multimorbidity patterns: A systematic review. J Clin Epidemiol. 2014;67(3):254–266.
- Violan C, Foguet-Boreu Q, Flores-Mateo G, et al. Prevalence, determinants and patterns of multimorbidity in primary care: a systematic review of observational studies. PLoS One. 2014;9(7):3–11.
- Barnett K, Mercer SW, Norbury M, et al. Epidemiology of multimorbidity and implications for health care, research, and medical education: a cross-sectional study. Lancet. 2012;380(9836):37–43.
- Roberts KC, Rao DP, Bennett TL, et al., Prevalence and patterns of chronic disease multimorbidity and associated determinants in Canada. Heal Promot Chronic Dis Prev Canada. 2015;35(6):87–94. cited 2018 Sept 15 http://www.ncbi.nlm.nih.gov/pubmed/26302227.
- Kuwornu J, Lix L, Shooshtari S, Multimorbidity disease clusters in Aboriginal and non-Aboriginal Caucasian populations in Canada. Chronic Dis Inj Can. 2014;34(4):218–225. [Accessed 2018 Sept 15] https://www.canada.ca/content/dam/phac-aspc/migration/phac-aspc/publicat/hpcdp-pspmc/34-4/assets/pdf/CDIC_MCC_Vol34_4_5_Kuwornu_eng.pdf.
- Linnet K, Gudmundsson LS, Birgisdottir FG, et al. Multimorbidity and use of hypnotic and anxiolytic drugs: cross-sectional and follow-up study in primary healthcare in Iceland. BMC Fam Pract. 2016;17(69):1–10.
- Tomasdottir MO, Sigurdsson JA, Petursson H, et al. Does ‘existential unease’ predict adult multimorbidity? analytical cohort study on embodiment based on the Norwegian HUNT population. BMJ Open. 2016;6(e012602):1–9.
- Marengoni A. Prevalence of chronic diseases and multimorbidity among the elderly population in Sweden. Am J Public Health. 2008;98(7):1198–1200.
- Schiøtz ML, Stockmarr A, Høst D, et al. Social disparities in the prevalence of multimorbidity - a register-based population study. BMC Public Health. 2017;17(1):1–11.
- Deering KN, Lix LM, Bruce S, et al. Chronic diseases and risk factors in Canada’s northern populations: longitudinal and geographic comparisons. Can J Public Heal. 2009;100(1):14–17.
- Canadian Polar Commission. Health and well-being in the Canadian north: recent advances and remaining knowledge gaps and research opportunities. 2014 [cited 2018 Oct 1]. http://www.polarcom.gc.ca/sites/default/files/health_and_wellbeing_summary.pdf
- Bruce SG, Riediger ND, Lix LM, Chronic disease and chronic disease risk factors among First Nations, Inuit and Métis populations of Northern Canada. Chronic Dis Inj Can. 2014;34(4):210–217. [cited 2018 Sept 15] https://www.canada.ca/content/dam/phac-aspc/migration/phac-aspc/publicat/hpcdp-pspmc/34-4/assets/pdf/CDIC_MCC_Vol34_4_4_Bruce_eng.pdf.
- Sarkar J, Lix LM, Bruce S, et al. Ethnic and regional differences in prevalence and correlates of chronic diseases and risk factors in northern Canada. Prev Chronic Dis. 2010;7(1):A13.
- Cui Y, Shooshtari S, Forget EL, et al. Smoking during pregnancy: findings from the 2009-2010 Canadian Community Health Survey. PLoS One. 2014;9(1):84640.
- Young TK, Chatwood S. Delivering more equitable primary health care in Northern Canada. CMAJ. 2017;189(45):E1377–E1378.
- Feely A, Lix LM, Reimer K. Estimating multimorbidity prevalence with the Canadian chronic Disease Surveillance system. Heal Promot Chronic Dis Prev Canada. 2017;37(7):215–222.
- Statistics Canada. Canadian Community Health Survey - annual component user guide: 2014 and 2013-2014 Microdata Files. Ottawa: Government of Canada; 2015. Available from: http://www23.statcan.gc.ca/imdb/p2SV.pl?Function=getSurvey&Id=164081
- Harrison C, Britt H, Miller G, et al. Examining different measures of multimorbidity, using a large prospective cross-sectional study in Australian general practice. BMJ Open. 2014;4(7):1–9.
- Statistics Canada. Canadian Community Health Survey (CCHS) 2013-14 Public Use Microdata File (PUMF) derived variable specifications. Ottawa: Government of Canada; 2016.
- Austin PC. A tutorial and case study in propensity score analysis: an application to estimating the effect of in-hospital smoking cessation counseling on mortality. Multivariate Behav Res. 2011;46(1):119–151.
- Rao JNK, Scott A. On Chi-Squared tests for multiway contingency tables with cell proportions estimated from survey data. Math Stat. 1984;12(1):46–60.
- Archer KJ, Lemeshow S. Goodness-of-fit test for a logistic regression model fitted using survey sample data. Stata J. 2006;6(1):97–105.
- Randolph JJ, Falbe K, Manuel AK, et al. A step-by-step guide to propensity score matching in R. Pract Assessment Res Eval. 2014;19(18):1–6.
- Olmos A, Govindasamy P. Propensity scores: a practical introduction using R. J Multidiscip Eval. 2015;11(25):1556–8180.
- Pirracchio R, Petersen ML, Van Der Laan M. Improving propensity score estimators’ robustness to model misspecification using super learner. Am J Epidemiol. 2015;181(2):108–119.
- Rose S, van der Laan MJ. Why match? investigating matched case-control study designs with causal effect estimation. Int J Biostat. 2009;5(1):Article1.
- Sterne JAC, White IR, Carlin JB, et al. Multiple imputation for missing data in epidemiological and clinical research: potential and pitfalls. BMJ. 2009;338:b2393.
- R Core Team. R: A language and environment for statistical computing. 2018. https://www.r-project.org/
- McRae L, O’Donnell S, Loukine L, et al. Mood and anxiety disorders in Canada, 2016. Heal Promot Chronic Dis Prev Canada. 2016;36(12):314–315.
- Moin JS, Moineddin R, Upshur REG. Measuring the association between marginalization and multimorbidity in Ontario, Canada: a cross-sectional study. J Comorbidity. 2018;8(1):2235042X1881493.
- Pampel FC, Krueger PM, Denney JT. Socioeconomic disparities in health behaviors. Annu Rev Sociol. 2010;36(August):349–370.
- Katikireddi SV, Skivington K, Leyland AH, et al. The contribution of risk factors to socioeconomic inequalities in multimorbidity across the lifecourse: a longitudinal analysis of the twenty-07 cohort. BMC Med. 2017;15(1):1–10.
- Han BH, Moore AA, Sherman SE, et al. Prevalence and correlates of binge drinking among older adults with multimorbidity. Drug Alcohol Depend. 2018;187:48–54.
- Thomas L, Peterson ED. The value of statistical analysis plans in observational research defining high-quality research from the start. JAMA. 2012;308(8):773–774.
- Greenland S, Pearl J, Robins JM. Causal diagrams for epidemiologic research. Epidemiology. 1999;10(1):37–48.
- Rose S. Mortality risk score prediction in an elderly population using machine learning. Am J Epidemiol. 2013;177(5):443–452.
- Young TK, Ng C, Chatwood S. Assessing health care in Canada’s North: what can we learn from national and regional surveys? Int J Circumpolar Health. 2015;74:1–14.
- Statistics Canada. Trends in Mortality rates, 2000 to 2013. Ottawa. 2017. https://www150.statcan.gc.ca/n1/pub/82-625-x/2017001/article/14775-eng.htm
- Hannah H, Santos M, Wong M, et al. Injuries in the Northwest Territories, Canada: 2000-09. Int J Epidemiol. 2015;44(S1):i57.