ABSTRACT
The feasibility of unmanned-aerial-vehicle-based photogrammetry was assessed for the reconstruction of high-resolution topography and geomorphic features of quarries by nadir and off-nadir imagery. The test site was a quarry located in the rural area of Turi (Bari, southern Italy). Two processing scenarios were created. Nadir images were initially used, and images acquired with off-nadir angles were added. An accurate set of ground control points (GCPs) were surveyed for both georeferencing purposes and validation processes. In the reconstruction of the surfaces, an accuracy of a few centimeters was achieved in the final positioning of point clouds representing the main geometries of quarry environment. However, greatest differences were found along the edges or the lines characterized by sudden slope changes. To better understand such results, some characteristic quarry shapes depicted by both the scenarios were compared to those surveyed by a total station used as an independent benchmark technique. It allowed to define the benefits introduced by the joint use of nadir and oblique images in the delineation of quarry shapes, surface discontinuities and better descriptions of sub-vertical walls. Beside the evaluation of benefits introduced by use of oblique cameras, the effectiveness of the proposed methodology was also discussed with alternative technologies. Unmanned aerial platforms represent an effective solution, with the need for few accurate GCPs.
Introduction
Open-pit mines and quarries represent some of the most evident topographic signatures of landscapes for human activities over a wide range of geomorphic settings (Chen, Li, Chang, Sofia, & Tarolli, Citation2015). Visual impact and modifications to the geomorphological settings constitute the main issues related to such landscape alterations, both during mining and for many years post-mining. Furthermore, better knowledge of the new geomorphic features introduced after intensive quarrying activities can improve the design of the most appropriate strategies for reclamation and rehabilitation of mined sites (Damigos & Kaliampakos, Citation2003) or implementation of geomechanical models to assess the slope stability.
Prior to these activities, detailed topographic surveys are required. Also, depending on current regulations, mine managers have to report the amount of extracted materials and mine tailings on a regular basis (Shahbazi, Sohn, Théau, & Ménard, Citation2015) with problems related to a reliable estimation of volumetric changes over time. The search for accurate and repeatable methodologies and procedures to reconstruct three-dimensional (3D) geomorphic features of quarries with centimetric spatial resolution is important for mine managers.
For 3D surveying of quarries and open-pit mines, aerial light detection and ranging, airborne photogrammetry, terrestrial laser scanning (TLS), global navigation satellite system (GNSS) and traditional survey techniques can provide useful information. However, high-resolution topographic surveying of quarries is associated with high capital and logistical costs (Chen et al., Citation2015). In particular, surveys over small areas do not justify the use of traditional aerial technology. Over large areas, point-based observations such as GNSS and electronic total stations are time-consuming and cost-intensive. In general, the cost of ground-based methodologies is relatively high, and mining activities need to be closed down in the operational zones. Moreover, the design of ground surveys for remote and difficult-to-access areas might represent a very hard task. The 3D representation of break lines, dumps and sides with sub-vertical walls is another relevant point in the selection of the best technology for quarry monitoring, assessment of extraction costs/efficiency and volumes computation. TLS can be used to acquire 3D point clouds of slopes whenever a good visibility from the setting points is guaranteed. Conversely, aerial methodologies based on nadir views are not very suited to the mapping of slope zones.
In recent years, aerial photogrammetry based on images acquired by unmanned aerial vehicles (UAVs) has been used for the reconstruction of geomorphic features at an affordable and unprecedented spatial and temporal resolution. In particular, structure from motion (SfM) photogrammetry with nadir and, more rarely, oblique cameras has provided high-resolution digital surface models (DSMs) with vertical accuracies and spatial resolution at few centimeter level (Agüera-Vega, Carvajal-Ramírez, & Martínez-Carricondo, Citation2016; Bryson, Johnson-Roberson, Murphy, & Bongiorno, Citation2013; Casella et al., Citation2014; Eltner et al., Citation2016; Fonstad, Dietrich, Courville, Jensen, & Carbonneau, Citation2013; Harwin, Lucieer, & Osborn, Citation2015; Mancini et al., Citation2013; Rupnik, Nex, & Remondino, Citation2014).
Little has been published relating to the applicability of UAV-based methodologies in quarrying, to overcome the use of simplified representations and layouts of slope geometry. In this field, McLeod et al. (Citation2013) explored the feasibility of using UAVs to obtain point clouds to measure fracture orientation, more recently Shahbazi et al. (Citation2015) and Chen et al. (Citation2015) adopted UAV technology for 3D mapping and geomorphic feature characterization of open-pit mine areas. Tong et al. (Citation2015) highlighted the need to integrate point clouds from nadir UAV images of slope zones with TLS-derived point clouds for land cover classification.
The feasibility of UAV-based photogrammetry was evaluated for the reconstruction of topography and geomorphic features of quarries and further assessments on acquisition geometries and obtainable accuracies introduced. The case study provides inspiration for discussions and definitions of methodologies valid in environments where defined geometries and sharp break lines are recurring features.
Methods
Case study
The case study is a 9-ha quarry in the rural area of Turi (Bari, southern Italy) that started operations more than 50 years ago (see ). This site shows prevalent Mesozoic carbonate rock locally buried under alluvional-eluvial red soil deposits, with no vegetation present across the area. The main geomorphic features are a multilevel shaped pit with a bottom altitude of about 35 m from the surface () and sub-vertical walls located on the east side that have sharp break lines (). The surface roughness is related to deposits of quarried material and to the signs left by the machinery that was used to break up the rock walls. Outside the perimeter of the quarried area, there is some sparse vegetation that covers the most superficial areas; any 3D reconstruction of ground surfaces based on a photogrammetric approach is not effective and can generate errors. ) shows a high-resolution ortophoto that was reconstructed in this study. For the successive analysis of the impact and benefits introduced by the joint use here of nadir and oblique cameras, the investigated area was reduced to the large, 4.6-ha sector located on the east side (, dashed polygon) mostly characterized by sub-vertical walls.
Figure 1. (a) Ortophoto of the quarry, showing also location of the study site within Italy; (b–e) sub-vertical walls and main geomorphological features of the studied area.
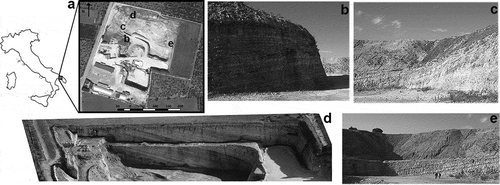
Figure 2. (a) Ortophoto of the entire site, with indication of the area where oblique images were collected (black dashed box; investigated area). Dots represent the location of the ground control points (GCPs) acquired through a global navigation satellite system (GNSS) survey, and squares represent the GNSS locations used as validation points (VPs). Some targets were used alternatively as GCPs and VPs; (b) three-dimensional (3D) model with a view of the camera positions; (c) GNSS antenna collecting data over a black and white target used as reference.
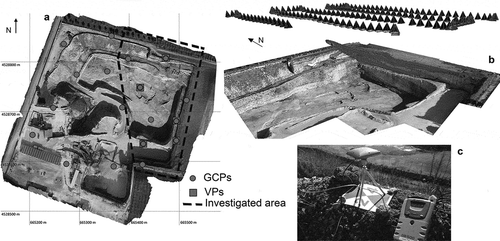
UAV flights
The UAV airframe used was a hexacopter ESAFLY A2500 that was designed and manufactured by Sea Air Land (SAL) Engineering, and equipped with a Canon EOS 550D digital camera with a fixed geometry during flights (focal length: 25.0 mm; sensor res. 5184 × 3456; average GSD: 0.01 m) and an on-board navigation-grade GNSS receiver. The maximum weight was 5.8 kg, with the upper limit of flight endurance of 20 min.
The flight plan with a conventional parallel flight lines development was planned at an altitude of 50 m a.g.l. using an aerial orthophoto as the reference map (the altitude provided here increases for inner portions due to the stepped topography). An autonomous mode of images acquisition was set for one shot per second, for a total of 786 acquired images. To cover the whole area, three flights were performed with nadir orientation of the camera, the acquisition timing gave up to a minimum of seven overlapping images for any single ground feature. However, a forward and side overlap of 90% was guaranteed. An additional flight with a 60° off-nadir camera (i.e. oblique images) and manual piloting was carried out to collect aerial images of the sub-vertical walls in the eastern part of the quarry (see , area within dashed line) at an average distance of 20 m from the walls. Images at finer scale were therefore produced during the flight with oblique camera. The redundant set of images acquired at the selected timing facilitates the SfM approach. Images acquired during the takeoff and landing operations and blurred images were filtered out during the later processing.
Ground control and validation points
Validation of reconstructed surfaces is a fundamental issue for multitemporal elevation data sets. A range of recent studies has advanced our understanding of sources of error, accuracy and precision of SfM techniques under different scenarios (Agüera-Vega et al., Citation2016; Fonstad et al., Citation2013; Harwin et al., Citation2015; James & Robson, Citation2012; James, Robson, d’Oleire-Oltmanns, & Niethammer, Citation2017). The number, density, spatial distribution and positioning accuracy of ground control points (GCPs) are also key factors for reliable positioning of UAV-SfM products. James and Robson (Citation2012) and Clapuyt, Vanacker, and Van Oost (Citation2015) demonstrated that the position error of DSMs decreases with the use of an increasing number of GCPs. James and Robson (Citation2012) assessed the effects of the distribution of GCPs on the results. These studies showed that widely dispersed GCPs and their orientation along the perimeter of an area promote fewer reconstruction errors. Similar data were obtained by Harwin et al. (Citation2015) in an accuracy validation procedure that determined the benefits of accurate GCPs and oblique imagery under different scenarios within a limited portion of an eroding coastal scarp. Depending also on the GCPs positional accuracy, such studies demonstrated that a number of 5–10 GCPs are able to produce an accuracy of about 5 cm of point clouds derived for limited surface extent. Considering a flight altitude of 50 m planned in this work, such value of accuracy is also comparable with the 1:1000 precision rates introduced by James and Robson (Citation2012).
Thus, in the attempt to provide a reliable procedure for quarries monitoring and reduce the efforts in the field, the locations of 18 evenly distributed targets were surveyed for successive use as GCPs in the final point-cloud orientation and validation points (VPs) for an accuracy assessments of the UAV-derived elevation data set (see for GCPs and VPs spatial distribution). In particular, GCPs were acquired along perimeter areas and at different elevations. Then, 20 cm × 25 cm plastic boards with alternate black and white triangles intersecting in the center were placed on horizontal surfaces only ().
Rapid-static GNSS surveying was performed to connect the target locations with a reference GNSS station located in the vicinity of the investigated area. The absolute coordinates of the reference station were calculated using static positioning over longer ranges within the GNSS permanent infrastructure managed by the positioning facility of Puglia Region. The post-processing of the baselines that connected the target locations with the reference point produced a level of accuracy better than 1 cm in the 3D positioning of the targets. Errors due to incorrect positioning of plates over rough surfaces are included in this level of uncertainty. A compact GNSS L1/L2 antenna was placed on a small tripod with fixed quotes and an upper thread adapted to fit a geodetic antenna (see for representation of the GNSS antenna mounted over a target). The same configuration was used over all of the targets.
To delineate some significant shapes of the investigated areas by an independent benchmark technique, several vertical profiles were also surveyed by a total station for further comparisons with shapes depicted by both the scenarios in correspondence of sudden changes in the quarry topography. A Leica TCR 1200+ series total station was used for this purpose (accuracies; angles: 1”, distances: 1 mm + 1.5 ppm).
Point-cloud generation
Initially, the reconstruction of the quarry area was performed using the whole data set, composed of 786 acquired images (nadir plus oblique views) and the 18 targets. No methodology for spatial sampling was applied; image quality was assessed just visually (with only three images of poor quality removed from the data set). To investigate the effects of using additional oblique imagery to better reconstruct the structure of the vertical walls, the analysis focused on the area of the quarry where both nadir and oblique images were available. The spatial distribution of GCPs and VPs reported in ) was selected (see the area of interest within the dashed polygon). A total of nine targets fell in the study area, four of which were used as GCPs and five as VPs.
Thus, two scenarios obtained from subsets of collected images were created with the same settings in the processing steps maintained. The first scenario (scenario N) contained 182 nadir images, while the second scenario (scenario N + O) included 224 oblique images in addition to scenario N. The SfM technique was applied to obtain a 3D georeferenced point cloud (Chen et al., Citation2015; Snavely, Seitz, & Szeliski, Citation2006); this approach reconstructs the camera pose and scene geometry simultaneously, through automatic identification of matching features in multiple images. A scale-invariant feature-transform algorithm (Lowe, Citation2004) detects and tracks these features from image to image, which enables initial estimates of the internal parameters, camera positions and object coordinates that are then refined iteratively using nonlinear least squares minimization during the bundle adjustment step. The dense matching algorithms used the parameters calculated in the previous stage to create the dense point cloud (Furukawa & Ponce, Citation2010; Hirschmuller, Citation2008; Rothermel & Haala, Citation2011). The steps involved in the generation of a 3D point cloud are similar regardless of the SfM software used. In the present study, the data were processed using the Agisoft PhotoScan photogrammetric software package (v.1.1.6, build 2038) and Pix4D mapper (v. 2.0.89).
The workflow is outlined in , and it comprised the following main steps: (i) data import, (ii) image alignment, (iii) creation of the sparse cloud, (iv) optimization of image alignment and (v) dense image matching. reports the settings values used in the image processing with Photoscan and Pix4D mapper.
Table 1. Settings values used in the image processing with Photoscan and Pix4D mapper.
After importing the images and the navigational GNSS data, preliminary alignment was carried out to facilitate the positioning of the GCPs. The GCPs were manually detected in the imagery, and their ground survey coordinates were loaded into the project, with each marker checked and edited when required, to ensure it was located and centered in as many images as feasible; they were not located on the edge of the image and in oblique images. The GCPs were a constraint in the creation of the model (GCPs’ accuracy equal to 0.005 m); their position in the imagery was manually refined (mean reprojection error equal to 0.068 pix) and then used to optimize the camera parameters (f, b1, b2, cx, cy, k1, k2, k3, p1, p2) and the orientation of the data, which allowed for improved accuracy and reconstruction results of the sparse point cloud (Chen et al., Citation2015).
The camera calibration was carried out simultaneously with the image processing by a self-calibration approach, which provides a solution for unknown camera parameters in an arbitrary coordinate system or, in the presence of control measurements included within the bundle adjustment, to a specific reference system. The validation of the 3D model accuracy involved the use of the remaining targets used as VPs. Five VPs were loaded and edited on the imagery in the same way as the GCPs; the method used to determine the accuracy of the derived model in comparison to the GNSS survey was to report the differences between the precisely surveyed VPs and their identified locations in the sparse point cloud.
Comparison of point clouds
In this study, the comparison of point clouds was adopted to discuss differences and improvements by the introduction of oblique imagery in the degree of distinctness in outlining the quarry shapes. Lague, Brodu, and Leroux (Citation2013) introduced an approach that allows direct comparisons of point clouds without meshing or gridding and to compute the local distances between point clouds along the surface normal direction. This method is called a multiscale model to model cloud comparison (M3C2), and it can be found as a plugin of the Cloud Compare software V2 (freely available at http://www.cloudcompare.org). Significant distances between point clouds were detected by the M3C2 algorithm after the estimate of a confidence interval based on the point-cloud roughness. Significant change indicated whether the distance measured by M3C2 was significantly greater (at a 95% confidence interval) than a reference measure of the roughness. The significant change was 0 if the surface was very rough compared to the mean distance measured between the two clouds or if there were not enough points in the “projection cylinder” (typically 4 by default). The dense cloud ensured a sufficient number of points for such an assessment. In this paper the M3C2 algorithm was selected because of its suitability in the comparisons of point clouds from monitoring operations of objects marked by well-defined surface orientation and prevailing shapes.
The operational diagram in ) shows the M3C2 analysis. For every point i, the neighboring points within a diameter D are used to calculate the punctual normal (N). Then the algorithm requires the definition of a search cylinder, with its axis aligned with the normal for i. The cylinder has diameter d (search scale), and a maximum length is imposed to speed up the calculation.
Figure 4. (a) Operational diagram of the M3C2 technique. The normal for i is calculated at scale D. A cylinder with diameter d and specified length is used to select points i1 and i2. LM3C2 is the distance between i1 and i2, and local roughness is calculated as σ1 and σ2 (from Lague et al. (Citation2013), with modifications); (b) influence of the complex topography on the determination of distances near the break lines. The normal directions influence the distance value; LM3C2 ≠ L’M3C2.
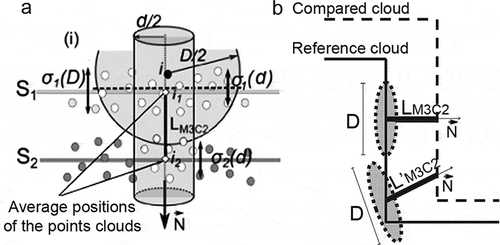
Results
Point-cloud generation and accuracy assessment
The proposed methodologies allowed the generation of point clouds from UAV images after the processing of the nadir (scenario N) and nadir plus oblique (scenario N + O) imagery. PhotoScan produced a sparse cloud compose of 15,924 points in the scenario N and 21,884 in the scenario N + O with better representation that the nadir view provided for sub-vertical walls and hidden surfaces. The processing of a dense cloud by setting the accuracy at the “medium” level resulted in 26,702,644 and 43,046,029 points for N and N + O scenario, respectively, with an average point density of 4 cm. In the dense cloud, the average point density related to walls changed from 330 points/m2 to 620 points/m2 after the introduction of oblique imageries, providing a more complete description of the area. The processing was repeated in a very similar configuration using Pix4D for further comparison. Pix4D could not match all the images of the scenario N + O together due to the absence of intermediate or transitional frames between the nadir and oblique acquisitions. So, the N and N + O data sets were processed separately and then aligned. Final joint optimization based on a bundle adjustment procedure made the refinement of the unknowns possible. Despite this, the results confirmed the achievement in the sparse and dense cloud generation.
The VP positions derived from accurate GNSS and their corresponding positions in the derived point cloud were used to calculate the error metrics (dx, dy, dz) for the dual scenarios (). The methodologies applied were effective for the production of accurate solutions, as the differences between the point-cloud positions and GNSS locations were limited to a few centimeters. Both scenarios N and N + O provided accurate solutions.
Table 2. Distances between the point clouds created by PhotoScan and Pix4D Mapper according to the dual scenarios (N, N + O) and the reference GNSS locations.
Moreover, to provide an assessment of the similarity between point clouds provided by PhotoScan and Pix4D within the investigated area in the N + O scenario, a comparison was performed using the M3C2 methodology. Parameters used in the M3C2 methodology assumed the following values: D varies between 0.3 m and 0.7 m, with step equal to 0.1 m; d = 0.1 m and maximum displacement distance = 10 m. This parameter setting allowed the calculation of the normal considering the roughness of the surface and with respect to the geometric complexity. The D value is suitable for the case study, and it is intermediate between the values proposed by Lague et al. (Citation2013) for gravel and cobbles (0.25 m, 0.7 m, respectively). The d value was chosen to limit the vertical search range. The M3C2 algorithm calculates the best-fit planes; to be effective, a minimum number of points is required to fall in the area of study (Lague et al., Citation2013). This comparison exhibits very small residuals, with averages values of −0.004 m (SD = 0.033 m). Due to this similarity between point clouds, the remaining analyses presented were carried out using Photoscan alone.
Contribution of nadir imagery to point clouds
A simple observation of the resulting dense clouds (see ) shows that complete representation of the vertical wall is obtained from the combination of the nadir and oblique images. shows the holes in the point cloud that indicate areas that were not detected in the nadir survey (i.e. scenario N). The texture of the points has a different quality in ) and 5(b), whereby in ) the different orientations of the camera and the variations in the lighting of the surfaces allowed a clear and more detailed representation of the vertical wall.
Figure 5. Three-dimensional dense point cloud for a vertical wall. (a) Scenario N; (b) scenario N+O.
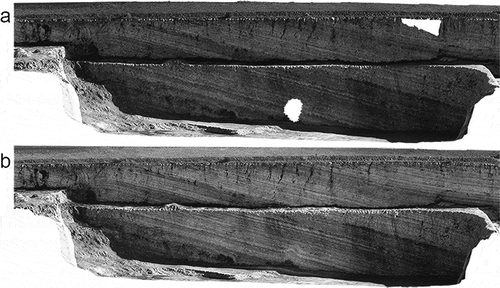
To determine the possible benefits introduced by the use of the oblique camera with respect to the prevailing orientations of geometric features, the differences between dense point clouds from the dual scenarios were computed within the study area using the M3C2 methodology and the parameter discussed earlier. The point cloud selected as reference layer was the one obtained using the nadir and oblique cameras (i.e. scenario N + O). The M3C2 algorithm calculated the distance between the reference and the compared cloud for every single point belonging to the reference surface. The comparison shows diffuse similarities and a few zones with positive and negative differences. As shown in ), the differences were evaluated in the area of the vertical walls. The M3C2-calculated distances resulted in absolute values distributed as a Gaussian function with mean = −0.001 m and SD = 0.365 m, with 99.2% of the values falling in the range of −0.365 to +0.365 m. As expected, the greatest differences were mainly oriented along the edges or the lines characterized by sudden slope changes and in zones outside the excavated area. The outside areas were characterized by lower overlapping of the frames and the presence of vegetation, whereby the automated processing based on SfM would be difficult. The scale for the calculation of the normal was linked to the creation of the optimal best plan fitting the data set. The variability of D between 0.7 and 0.3 m generates, in this case, 36.5% of the core points with a D value of 0.7 m, 25.7% of 0.6 m, 18.5% of 0.5 m, 12.3% of 0.4 m and 7.0% of 0.3 m. Here, 95.1% of the core points had significant changes of 0; it means that calculated distance are significative.
Figure 6. Distances calculated by the M3C2 algorithm and comparison with the independent data set provided by surveying with total station. The lowest values (white in the legend) are transparent in the 3D model, for easy viewing. The upper figure shows the position of six profiles (named with letters from b to g) where data are compared. The lower figures show the vertical sections of the two point clouds, with an indication of the significant distances, and a comparison with the reference data used as benchmark. Red lines, vertical profile from scenario N+O used as reference by the M3C2 algorithm; blue lines, compared model of scenario N; black solid lines, vertical profiles detected by total station measurements. The horizontal distance (X-axis) is from the starting point of the section; Y-axis represents the height values along the profiles.
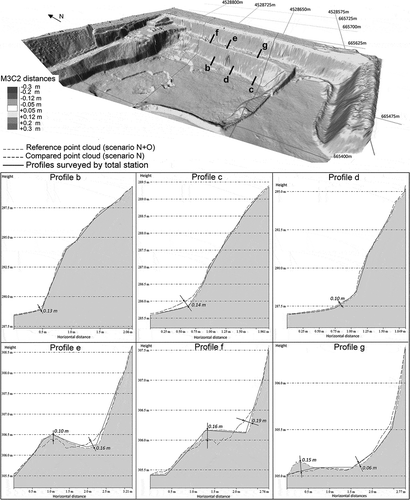
) focuses on the comparison of the point clouds along the vertical wall, where potential benefits in the representation of edges and sudden changes in surface orientations could be expected after the use of oblique imageries. The distances vary indeed between −0.3 and +0.3 m, with the highest located in discontinuities that are characterized by sudden slope changes. The values between −0.05 and +0.05 m are transparent in the representation, because these were deemed nonsignificant considering the metric accuracies obtainable with this methodology (according to the precision ratios of about 1:1000 reported by James & Robson, Citation2012). The point clouds generated by the two scenarios (i.e. N, N + O) were cut with the vertical planes (producing vertical profiles) to determine whether the calculated distances were real or were an incorrect estimation of the M3C2 algorithm. Also, to provide an independent technique as benchmark in the assessment of benefits introduced by the processing of oblique view, the aforementioned sections have been compared with data collected by the total station. , profiles b–g, shows some of the profiles created, where the black solid line indicates the position of the reference point cloud. We note that there is a general agreement (gray areas) with the distances calculated by the algorithm and those represented in ). The yellow and red zones in ) indicate a positive distance, and the profiles confirm these results, where the compared point cloud (profiles b–g, blue line) is above the reference (profiles b–g, red line). In the blue areas in ), the two point clouds are reversed and the distances are negative. The comparison with profiles surveyed by total stations suggested that areas characterized by sudden changes in topography and surface orientations are better represented by the scenario (N + O). In particular, this is evident for profiles e and f, where the reference profile is more closely represented by the vertical sections obtained from the N + O scenario.
Discussion
Oblique images, GCPs and accuracy
The accuracy at centimeter level achieved in the final positioning of the point clouds from UAV photogrammetry is comparable with performances provided by long range TLS, differential GNSS or total station, although the costs are not always comparable. Certainly, patches of vegetation affect the elevation models produced by any of the available photogrammetry-based methodologies. However, the aerial nadir view is not very suited for surveying sub-vertical walls, although the contributions of the oblique cameras demonstrated the possibility to strengthen the consistency of the reconstructed surfaces.
However, to fully exploit the potentialities of the imagery collected by the oblique camera, an algorithm that can process nadir and oblique images simultaneously is needed (e.g. PhotoScan). Even with the inclusion of oblique imagery, the use of a few accurate GCPs is strongly recommended. As shown by James and Robson (Citation2014), in the absence of GCPs, systematic errors in the DSM creation can be significantly reduced through the collection of oblique imagery.
Quantitative studies on the benefits introduced by oblique imagery in the reconstruction of natural landscapes are not very common. Harwin et al. (Citation2015) investigated the joint use of nadir and oblique imagery and several scenarios related to GCP accuracy (centimeter and millimeter levels) and distribution. They indicated that the benefits introduced by oblique imagery can be particularly noted with inaccurate GCPs (a few centimeters in the level of accuracy). The dual scenario examined in the present study used accurate GCPs but differed in the geometry of the acquisition of the imagery. reports the very small discrepancies for both scenarios N and N + O, although the arrangement of the VPs should be commented upon. The VPs were placed at variable depths on the horizontal surfaces only, with no VPs placed on the vertical walls. This means that only horizontal VPs contributed to the validation stage. However, in the case of the use of VPs on the vertical walls only, the nadir imagery would barely identify the targets. For this reason, a comparison of the accuracy achieved by the scenarios N and N + O cannot be performed with the same VP distribution if the VPs were distributed on the walls. Thus, for logistic reasons and with the need to establish a methodology for an effective and timely UAV aerial survey, the targets were placed only on horizontal surfaces.
Ability to reconstruct shapes for sub-vertical surfaces
As shown in , the scenario with nadir and oblique images (N + O) allowed detailed representation of the vertical wall without voids. The distances calculated between the two point clouds indicated that the greatest differences were along discontinuities. These values might have been partly generated by the M3C2 algorithm, according to the delineation of the normal direction. In sharp break lines, the matching area for the normal calculation (D) falls on differently oriented surfaces. As shown in ) for point clouds that fall close to break lines, the normal directions might influence the distance. However, inspection of the vertical sections makes the reasons for such heterogeneities more comprehensible. indicated that the differences between the vertical profiles from scenarios N and N + O arose from well-recognizable differences in geometric features. In , the profiles reconstructed from scenario N + O (red line) that are shown are more suitable to represent the complex geometries and are closer to the reference profile than profiles generated by the use of nadir mages only. In the N + O scenario, the higher point density allowed better delineation of the break lines. Moreover, scenario N (blue line) did not allow precise modeling of the debris material at the base of the vertical walls (see also profiles e–g). In all of the profiles, scenario N produced smoothed geometries and a more gradual transition between the horizontal and vertical surfaces than scenario N + O.
Pros and cons of UAV to other surveys technologies
An investigation on the feasibility and affordability of UAV-based surveys as a tool to reconstruct high-resolution topography of quarries deserves a short discussion on their performance with different technologies. GNSS and electronic total station might sometimes have difficulties for the monitoring of large areas and thus can generate high costs (Tong et al., Citation2015). TLS and UAV photogrammetry generate models with higher point densities. However, within areas with complex morphology, TLS might require many station points to avoid occlusions, and the whole survey could become time and cost demanding. UAV photogrammetry requires mission planning and flights design based on nadir and oblique camera poses and a GNSS survey for the accurate positioning of the GCPs and VPs. The whole procedure ensures rapid execution of the survey; although, based on the number of acquired images, the processing of the data could be time-consuming. The execution costs of survey based on the mentioned technologies could vary largely and relies on logistic conditions, the size of the area and the required degree of detail. Also, they are connected to the productivity, the cost of the skilled personnel/equipment and processing steps (Bangen, Wheaton, Bouwes N., Bouwes B., & Jordan, Citation2014). The extent of surveyed surface and the required detail in the reconstruction of quarry topography play a fundamental role. Technologies based on aerial surveys are less influenced by the increase in the surveyed surface.
For small sites, the acquisition is simple and rapid; the traditional methods have competitive costs and are well suited. By increasing the surveyed area, the technologies that are more productive (i.e. TLS, UAV) become more convenient. For large area, the UAV survey could become the most effective solution, being able to provide a reasonable accuracy for quarry monitoring purposes under a wide range of operative conditions. TLS surveys could provide an even better accuracy in the surfaces reconstruction, but the number of stations needed to represent the whole structure could increase dramatically for large extents. Under these conditions GNSS and total station cannot be considered the best practices. Usually, GNSS surveys for morphological reconstructions refer to a real-time or post-processed kinematic survey. Detected paths can allow the identification of the main geometry of a quarry or provide a more detailed description (e.g. two points per square meter). Similarly, total station can detect only the main geometric features of the area, while higher numbers of points require significant increases in the costs and effort. An increasing in time required for surveying the quarry environment could constitute an unfavorable circumstance.
During surveys, the mining activities have to be totally or partially stopped, with additional costs related to the potential loss of productivity and any trouble in optimization of the machine activity. UAV allows the survey of very large extent within one working day and could become the most suitable method when complex quarry environments have to be reconstructed, with high point densities and in a rapid and economically competitive way.
Conclusions
The analysis of UAV images by SfM approach constitutes a reliable and validated tool for surveyors who are interested in high-resolution reconstruction and monitoring of quarries. The methodology is well suited for the definition of regular geometries and for sudden changes of surfaces’ slope. The accuracy and performance of UAV-SfM methodology make it competitive with technologies used for this kind of application. The rapid execution of the survey and the few necessary observations on the ground make it a suitable technique for manufacturing areas and dangerous zones, where speed and safety of the personnel are essential requirements. The quality of the SfM 3D models is influenced by the quality of the images and the factors related to the mission planning. Careful planning of the UAV trajectories and the nadir and oblique image acquisitions with some overflights and the survey of limited and accurate GCPs have been all crucial for the success of the whole survey.
This UAV-based photogrammetry with nadir and oblique imagery provides a valuable tool for quarry management by operators and for the monitoring of mining activities on a regular basis. UAV surveys also provide a useful tool for management of the environmental risk of dangerous and inaccessible areas, and they enhance the geotechnical interpretation of spatially variable soil conditions with a reasonable measure of reliability. A permanent array of targets deployed within the mined areas could strengthen the repeatability of the resulting 3D model and reinforce the analysis of mined volumes from comparisons of successive 3D models.
Disclosure statement
No potential conflict of interest was reported by the authors.
Additional information
Funding
References
- Agüera-Vega, F., Carvajal-Ramírez, F., & Martínez-Carricondo, P. (2016). Accuracy of digital surface models and orthophotos derived from unmanned aerial vehicle photogrammetry. Journal of Surveying Engineering, 04016025. doi:10.1061/(ASCE)SU.1943-5428.0000206
- Bangen, S.G., Wheaton, J.M., Bouwes, N., Bouwes, B., & Jordan, C. (2014). A methodological intercomparison of topographic survey techniques for characterizing wadeable streams and rivers. Geomorphology, 206, 343–221. doi:10.1016/j.geomorph.2013.10.010
- Bryson, M., Johnson-Roberson, M., Murphy, R.J., & Bongiorno, D. (2013). Kite aerial photography for low-cost, ultra-high spatial resolution multi-spectral mapping of intertidal landscapes. PLoS One, 8(9), e73550. doi:10.1371/journal.pone.0073550
- Casella, E., Rovere, A., Pedroncini, A., Mucerino, L., Casella, M., Cusati, L.A., … Firpo, M. (2014). Study of wave run up using numerical models and low-altitude aerial photogrammetry: A tool for coastal management. Estuarine, Coastal and Shelf Science, 149, 160–167. doi:10.1016/j.ecss.2014.08.012
- Chen, J., Li, K., Chang, K.J., Sofia, G., & Tarolli, P. (2015). Open-pit mining geomorphic feature characterization. International Journal of Applied Earth Observation and Geoinformation, 42, 76–86. doi:10.1016/j.jag.2015.05.001
- Clapuyt, F., Vanacker, V., & Van Oost, K. (2015). Reproducibility of UAV-based earth topography reconstructions based on structure-from-motion algorithms. Geomorphology, 260, 4–15. doi:10.1016/j.geomorph.2015.05.011
- Damigos, D., & Kaliampakos, D. (2003). Assessing the benefits of reclaiming urban quarries: A CVM analysis. Landscape and Urban Planning, 64(4), 249–258. doi:10.1016/S0169-2046(02)00243-8
- Eltner, A., Kaiser, A., Castillo, C., Rock, G., Neugirg, F., & Abellán, A. (2016). Image-based surface reconstruction in geomorphometry – Merits, limits and developments. Earth Surface Dynamics, 4(2), 359–389. doi:10.5194/esurf-4-359-2016
- Fonstad, M.A., Dietrich, J.T., Courville, B.C., Jensen, J.L., & Carbonneau, P.E. (2013). Topographic structure from motion: A new development in photogrammetric measurement. Earth Surface Processes and Landforms, 38(4), 421–430. doi:10.1002/esp.v38.4
- Furukawa, Y., & Ponce, J. (2010). Accurate, dense, and robust multiview stereopsis. IEEE Transactions on Pattern Analysis and Machine Intelligence, 32(8), 1362–1376. doi:10.1109/TPAMI.2009.161
- Harwin, S., Lucieer, A., & Osborn, J. (2015). The impact of the calibration method on the accuracy of point clouds derived using unmanned aerial vehicle multi-view stereopsis. Remote Sensing, 7(9), 11933–11953. doi:10.3390/rs70911933
- Hirschmuller, H. (2008). Stereo processing by semiglobal matching and mutual information. IEEE Transactions on Pattern Analysis and Machine Intelligence, 30(2), 328–341. doi:10.1109/TPAMI.2007.1166
- James, M.R., & Robson, S. (2012). Straightforward reconstruction of 3D surfaces and topography with a camera: Accuracy and geoscience application. Journal of Geophysical Research: Earth Surface, 117(F03). doi:10.1029/2011JF002289
- James, M.R., & Robson, S. (2014). Mitigating systematic error in topographic models derived from UAV and ground‐based image networks. Earth Surface Processes and Landforms, 39(10), 1413–1420. doi:10.1002/esp.v39.10
- James, M.R., Robson, S., d’Oleire-Oltmanns, S., & Niethammer, U. (2017). Optimising UAV topographic surveys processed with structure-from-motion: Ground control quality, quantity and bundle adjustment. Geomorphology, 280, 51–66. doi:10.1016/j.geomorph.2016.11.021
- Lague, D., Brodu, N., & Leroux, J. (2013). Accurate 3D comparison of complex topography with terrestrial laser scanner: Application to the Rangitikei canyon (NZ). ISPRS Journal of Photogrammetry and Remote Sensing, 82, 10–26. doi:10.1016/j.isprsjprs.2013.04.009
- Lowe, D.G. (2004). Distinctive image features from scale-invariant keypoints. International Journal of Computer Vision, 60(2), 91–110. doi:10.1023/B:VISI.0000029664.99615.94
- Mancini, F., Dubbini, M., Gattelli, M., Stecchi, F., Fabbri, S., & Gabbianelli, G. (2013). Using unmanned aerial vehicles (UAV) for high-resolution reconstruction of topography: The structure from motion approach on coastal environments. Remote Sensing, 5(12), 6880–6898. doi:10.3390/rs5126880
- McLeod, T., Samson, C., Labrie, M., Shehata, K., Mah, J., Lai, P., & Elder, J.H. (2013). Using video acquired from an unmanned aerial vehicle (UAV) to measure fracture orientation in an open-pit mine. Geomatica, 67(3), 173–180. doi:10.5623/cig2013-036
- Rothermel, M., & Haala, N. (2011, June 14-17). Potential of dense matching for the generation of high quality digital elevation models. In ISPRS Hannover 2011 Workshop High-Resoultion Earth Imaging for Geospatial Information, Hannover (pp. 331–343).
- Rupnik, E., Nex, F., & Remondino, F. (2014). Oblique multi-camera systems–orientation and dense matching issues. The International Archives of Photogrammetry, Remote Sensing and Spatial Information Sciences, 40(3), 107. doi:10.5194/isprsarchives-XL-3-W1-107-2014
- Shahbazi, M., Sohn, G., Théau, J., & Ménard, P. (2015). UAV-based point cloud generation for open-pit mine modelling. The International Archives of Photogrammetry, Remote Sensing and Spatial Information Sciences, 40(1), 313. doi:10.5194/isprsarchives-XL-1-W4-313-2015
- Snavely, N., Seitz, S.M., & Szeliski, R. (2006). Photo tourism: Exploring photo collections in 3D. ACM Transactions on Graphics, 25(3), 835–846. doi:10.1145/1141911
- Tong, X., Liu, X., Chen, P., Liu, S., Luan, K., Li, L., … Hong, Z. (2015). Integration of UAV-based photogrammetry and terrestrial laser scanning for the three-dimensional mapping and monitoring of open-pit mine areas. Remote Sensing, 7(6), 6635–6662. doi:10.3390/rs70606635