ABSTRACT
In this paper, an adaptive RBF neural network-based sliding mode controller is developed for superconducting magnetic energy storage (SMES) installed in a wind–diesel power system. Due to sudden load and wind power variations in a wind–diesel power system, power imbalances may force the system frequency to deviate from its nominal value and drive the system to operate in an unstable mode. Therefore, in a wind–diesel power system using a converter interface, a fast-acting and high power density SMES device is interconnected to carry the required power exchange whenever power imbalances occur. Based on switching manifold design, a sliding mode controller is developed to control the charging and discharging operations of SMES coil as per the power requirements, and the same is achieved by controlling the converter. A neural network using a radial basis function (RBF) is developed to estimate the unknown function of the system. Lyapunov stability analysis is conducted to guarantee the asymptotic stability of the system. MATLAB simulations are carried out and are presented to show the improved performance with the system exposed to disturbances in load and wind power.
Graphical abstract
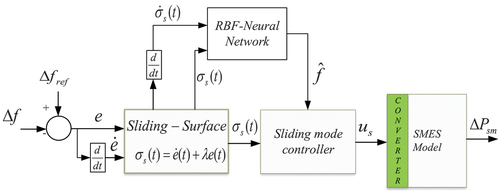
Disclosure Statement
No potential conflict of interest was reported by the author(s).