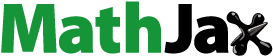
Abstract
Random regret minimization models have mostly relied on the assumption of identically and independently distributed error terms. Professionally designed stated choice experiments use various design and implementation principles to ensure that any errors meet this assumption. Based on the behavioral theory that individuals arrive at a decision by first processing the attribute values of a choice alternative according to an integration rule to derive the stochastic utility associated with the choice alternatives and then apply the deterministic utility maximizing rule, this assumption can be reasonable met for utility maximizing models. The question is whether this assumption is equally defendable for random regret minimization models in light of the difference in model specification, rooted in fundamental behavioral differences between the underlying decision theories. This study focuses on the effect of omitted variables which is one of main sources of error. Based on a formal analysis and empirical comparison, we argue and show that omitted variables cause correlation between unobserved regrets. Consequently, the independence assumption is difficult to defend because the omitted variables simultaneously affect the comparison of choice alternatives. To capture this effect, we propose an error components structure for regret-based choice models. Empirical results obtained for classic random regret-minimization models for two different data sets support our theoretical arguments. If these results can be generalized to other data sets, it is advisable to adopt an error components structure when estimating these classic random regret-minimization models unless one has reason to believe the effect of the omitted variables is small and therefore can be ignored.
1. Introduction
Discrete choice models have found many applications in travel demand forecasting, replacing traditional aggregate transport demand models. The imposed dominant decision rule has been the principle of utility maximization, which postulates that individuals derive a stochastic utility from their choice of an alternative, and choose the alternative that maximizes their utility. Choice models can be differentiated according to assumptions made about the variance-covariance matrix of the error terms of the utility function. The most common assumption is that error terms are identically and independently Gumbel distributed (IID-Gumbel). This assumption leads to the multinomial logit (MNL) model (McFadden Citation1974).
Recently, regret-based choice models (Chorus, Arentze, and Timmermans Citation2008) have attracted the attention of several scholars in transportation research as an alternative for the utility-based choice models. Based on seminal regret theory (Loomes and Sugden Citation1982, Citation1983, Citation1987; Bell Citation1982; Quiggin Citation1994), these models assume that individuals arrive at a choice by minimize regret instead of maximizing utility, where regret has been defined as a negative emotion that stems from the comparison of alternatives when an individual realizes he/she did not choose the best alternative after all. While the specification of the regret function has been subject of theoretical and empirical discussion, leading to several alternative specifications, random regret minimization models have almost invariably assumed that the error terms are IID Gumbel distributed under the principle of regret minimization. Originally, this assumption has primarily been made for convenience; most recently the assumption has been subject of critical reflection.
After its introduction, research on regret models has focused on the empirical performance of RRM models relative to RUM models (e.g. Chorus Citation2012a; Boeri and Masiero Citation2014; Sharma, Hickman, and Nassir Citation2019; Masiero, Yang, and Qiu Citation2019) using the MNL specification. These studies have assumed that unobserved regrets are identically and independently distributed. However, considering that regret is defined based on pairwise comparisons of alternatives, this assumption may be difficult to defend. Therefore, in this paper, the common specification of the error terms in classic random regret minimization models is put to scrutiny. In a previous paper (Jang, Rasouli, and Timmermans Citation2017), we focused on the effect of measurement error. In this paper, we explore the effect of omitted variables.
Manski (Citation1973) identified four main sources of error: (1) measurement errors, (2) omitted (relevant) attributes, (3) unobserved individual characteristics (‘unobserved taste variations'), (4) proxy or instrumental variables. These sources of error may cause significant bias in model results and may thus prevent researchers formulating the right transport policy recommendations. Among these possible sources of error, omission of relevant variables introduces bias in model parameters, and may lead to incorrect inferences (Washington, Karlaftis, and Mannering Citation2010). For a variety of reasons, the number of variables included in the deterministic part of the utility/regret function is limited. First, the principle of parsimony is still widely adopted in travel behavior research. A simple equation with few variables is still seen as the hallmark of academic research. Secondly, many models of travel demand are estimated from secondary data sets, which tend to contain general-purpose variables of limited scope and depth. Consequently, researchers have no choice but to accept and use the available data. Thirdly, even when researchers know that a larger set of variables is potentially influential to the phenomenon under investigation, budget constraints and/or (un)justified concerns about survey length and complexity reduce the number and kind of explanatory variables.
The most common way to dampen the effect of omitted variables in RUM models is to use alternative specific constants (ASCs) (e.g. Tardiff Citation1978; Ben-Akiva and Lerman Citation1985). The ASCs have been used in the RUM models to reflect the average effect of the omitted variables (e.g. Hensher, Rose, and Greene Citation2015), assuming the omitted variable is not correlated with other variables, and the effect of the omitted variable is alternative-specific. The detailed effect of omitted variables in random regret minimization models has not received much attention yet. To the best of our knowledge, only Van Cranenburgh and Prato (Citation2016) examined the problem and argued that (choice set specific) ASCs are necessary for the RRM to achieve consistent parameter estimates. Considering the definition of ASCs, even if these constants are choice set specific, it implies that the error (unobserved regret) by omitted variables is specific for each alternative. However, realizing that the very notion of regret is based on a systematic comparison of alternatives, the question is whether their suggested approach can sufficiently offset the effect of omitted variables in random regret minimization models as ASCs do in random utility maximization models.
This study, therefore, investigates whether/how omitted variables differently affect (linear-additive) RUM and RRM models. Since the behavioral underpinnings of these models differ, the bias caused by omitted variables in RRM models is also expected to be different from RUM models. It will be shown that, whereas the errors caused by omitted variables in RUM models are alternative-specific and independent, errors in RRM models are specific for pairs of chosen and non-chosen alternatives, and correlated with each other. Furthermore, it will be explored how these effects can be more effectively cancelled in RRM models.
2. Formal analysis
In this section, we formally compare how the effect of omitted variables differs between RUM and RRM. Each variable is assumed to be exogenous, therefore, independent.
2.1. Random utility maximization models
According to random utility theory (McFadden Citation1974), researchers have commonly assumed that utility consists of two components: a deterministic and a random part. Consequently, the total utility of alternative i of individual n can be formulated as:
(1)
(1)
The deterministic utility
is generally expressed as a linear-additive function of observed attributes. Assume a binary choice set includes only a single variable (k = 1). The (deterministic) attribute-level utility of individual n for alternative i can then be formulated as
(2)
(2)
Then, extending to multi-attribute choice alternatives (k = 1, 2, 3, … , K), the deterministic utility is defined as the sum of attribute-level utilities.
(3)
(3)
Based on the logit structure with identical and independent Gumbel distributed error terms, since (linear-additive) random utility models assume that individuals assess each alternative independently and separately, any additional alternative in the choice set does not affect the utility and choice odds. This property is called the Independence of Irrelevant Alternatives (IIA) property (Arrow Citation1951).
If the Kth variable is omitted from the utility specification,
(4)
(4)
where
is the error generated by the omitted variable(s). If the omitted relevant variable(s) is not correlated with the explanatory variables
, the effect of omitted relevant variable(s) can be represented as a certain amount.
(5)
(5)
As shown in Equation (5), the error generated by omitted variable(s) is alternative-specific. Since the utility of an alternative only depends on its own attribute values, the effect of omitted variables is also alternative-specific. Therefore, alternative specific constants (ASCs) have been proposed to represent the average effect of the omitted variable(s) (Tardiff Citation1978; Ben-Akiva and Lerman Citation1985; Train Citation2009; Ortuzar and Willumsen Citation2011).
(6)
(6)
Based on the assumption that error terms are identically, independently Gumbel distributed (IID-Gumbel), the probability of choosing an alternative can be derived using maximum likelihood estimation:
(7)
(7)
(8)
(8)
where
is the likelihood function and
is log likelihood function.
is 1 if individual n chooses alternative i and 0 otherwise.
To normalize the effect of ASCs, one of the ASCs is set to zero. Then, the ASCs can be interpreted as a difference between utilities, relative preference between alternatives, when everything else is equal (Ben-Akiva and Lerman Citation1985).
(9)
(9)
2.2. Random regret minimization models
Similar to RUM, RRM assume that total regret is composed of deterministic regret and an error term:
(10)
(10)
Several (deterministic) regret functions have been suggested in the literature. The classic versions of regret can be summarized as (Hensher, Rose, and Greene Citation2015):
(11)
(11)
(12)
(12)
(13)
(13)
Chorus, Arentze, and Timmermans (Citation2008) first introduced RRM suggesting equation 11 (called Rmax). Regret is defined as the negative emotion that individuals experience in situations where a non-chosen alternative turns out to be more attractive than the chosen one. Therefore, regret at the attribute-level is assumed a function of the difference between chosen alternative i and non-chosen alternative j. Then, regret for the chosen alternative i based on a comparison with non-chosen alternative j is defined as the sum of attribute-level regret. Finally, total regret for the chosen alternative i is defined to depend only on the best non-chosen alternative in the choice set, following the principle of Irrelevance of Statewise Dominated Alternatives (ISDA) (Quiggin Citation1994).
To avoid the non-smooth likelihood function due to the max operator, Chorus (Citation2010) proposed an alternative logarithmic specification (called Rlog in Equation (12)). This specification has been called the classic RRM (Van Cranenburgh, Guevara, and Chorus Citation2015). With respect to attribute-level regret, these two formulations become asymptotically identical for larger the attribute differences. However, Rlog generates a regret equal to when two alternatives are identical and thus have exactly the same attribute values. Chorus (Citation2014) proposed a correction term,
for all alternatives and individuals. The difference between the models is then one of interpretation, rejoice (counterpart of regret) is generated when the chosen alternative outperforms the non-chosen one, and converges to
for larger attribute-difference due to the shape of the logarithmic function.
Another distinctive feature of this specification is the substitution of the comparison against the best only by the comparison with all non-chosen alternatives. Rasouli and Timmermans (Citation2014, Citation2017), examined a mixture of the two specification to isolate the effect of the specification of the regret function, and called it the Rsum formulation (Equation (13)). There were no claims that this specification is necessarily the better model. They argued that the best specification of regret (best only vs. all pairwise comparisons) in multi-alternative choice sets may depend on the decision context, and is primarily an empirical matter (unless one chooses a model for theoretical reasons). Van Cranenburgh, Guevara, and Chorus (Citation2015) called this formulation the pure RRM model.
Rasouli and Timmermans (Citation2017) theoretically and empirically compared these three types of regret, and argued that Rmax is theoretically more appealing and a more valid representation of the concept of regret. The model was shown to outperform the other two specifications in a dedicated example with small attribute differences, the attribute domain where one expects the largest differences between the model specifications. Additional data are however required to judge the generalizability of this finding. With larger attribute differences, the different specifications of the regret function will lead to asymptotically identical models.
All these regret specifications assume that regret is a linear function of objective attribute-level differences. Therefore, the regret function can be generalized as:
(14)
(14)
where
is an attribute-level regret function. As shown in Equations (11)–(13), it can be
or
.
is a function to represent the amount of regret in multi-alternative choice sets. If it only depends on best non-chosen alternative
if it is defined against all non-chosen alternatives
.
The negatives of the error terms in Equation (10) are generally assumed IID-Gumbel. In this case, the probability of choosing an alternative can be derived using maximum likelihood estimation:
(15)
(15)
Later, Van Cranenburgh and Prato (Citation2016) asserted that ASCs are necessary for labeled data but not useful for unlabeled data. More specifically, they argued that the ASCs need to be choice-set specific depending on the number of alternatives in the choice set. Note that if all respondents have the same number of alternatives, the use of choice-set specific ASCs is the same as general ASCs. Then, the choice probability equals:
(16)
(16)
where
means the choice-set specific ASCs.
However, whereas regret is generated by comparing attribute values between alternatives, the ASCs imply that the bias by omitted variables is alternative-specific. This seems conceptually inconsistent.
Let us explore how omitted variables formally affect the generation of regret. Assume a binary choice set including only a single variable (k = 1). The (deterministic) attribute-level regret of individual n for alternative i can then be formulated as
(17)
(17)
Then, extending to multi-attributes choice alternatives (k = 1, 2, 3, … , K), the deterministic regret is defined as the summation of attribute-level regrets.
(18)
(18)
If the Kth variable is omitted from the choice set, it directly affects the comparison between two multi-attribute alternatives.
(19)
(19)
where
is the error generated by omitted variable(s) in comparison of chosen alternative
and non-chosen alternative
.
In multi-alternatives choice sets, the regret of chosen alternative i with omitted variable(s) is:
(20)
(20)
The error caused by omitted variable(s) in RRM is formally different. Whereas the error in RUM is alternative-specific, it is ‘pair of alternatives’-specific in RRM. While the error in RUM is independent of omitted variables for non-chosen alternatives, it is correlated with omitted variables for (at least one) other alternative(s) in RRM. This is because, behaviorally, regret is generated based on a comparison of alternatives. Therefore, the error by omitted variables can be formulated as:
(21)
(21)
Consequently, the error caused by omitted variables in the regret of chosen alternative i depends not only on the attribute value of chosen alternative i
but also the attribute value of non-chosen alternative j
. This indicates that
and
are correlated (Both errors share
and
). To consider such correlation caused by
and
, we introduce an error component
that alternatives i and j have in commonFootnote1. Likewise, while the ACSs reflect the average of the omitted variables in the linear-additive utility (Hensher, Rose, and Greene Citation2015), the error component
is the average effect of omitted variable(s) on the comparison of alternatives
and
. Furthermore, researchers do not know which variables are omitted, nor their value, and their regret weight. Therefore, we assume that the amount of regret caused by the omitted variable follows a certain distribution across individuals with mean and variance. Since the effect of omitted variables in regret should be positive to maintain its ratio properties, we assume a lognormal distribution for
. Then, total regret can be generalized as:
(22)
(22)
where,
is the remaining error caused by other sources rather than measurement error and is assumed to independently and identically distributed.
Thus, whereas previous studies assume that the effect of omitted variables is alternative-specific and homogeneous, in this study, we argue that the effect is correlated between alternatives and is individual-specific.
For a better understanding, assume three alternatives 1, 2, 3 with two attributes a, b in the choice set. Assume regret is generated based on the model, expressed in equation 13. Then, the deterministic regret
can be defined as:
(23)
(23)
(24)
(24)
(25)
(25)
Assume attribute b is omitted,
(26)
(26)
(27)
(27)
(28)
(28)
Then, if we assume error terms are IID-Gumbel distributed, the probability of choosing an alternative can be derived:
(29)
(29)
where
is assumed to be lognormal distributed with density
, and
refers collectively to the parameters of the distribution (generally mean and standard deviations of
).
Since the choice probability in Equation (29) is obtained by solving multiple integrals, it causes higher complexity in computation. The alternative way is to simulate the average probability, called the maximum simulated likelihood estimator (17).
(30)
(30)
(31)
(31)
where C is a certain number of draws estimating threshold parameters,
is unbiased estimator of
.
Therefore, we can conclude that the classic ASCs are formally not appropriate to represent the errors by omitted variables in RRM. Since regret is generated from pairwise comparisons, the omitted variable(s) causes specific bias in pairs of alternatives. More precisely, the omitted variable(s) for alternative 1 causes bias for alternatives 2 and 3. Likewise, the omitted variable(s) for alternative 2
and 3
also cause errors in alternative 1. Therefore, the errors due to omitted variables for the chosen alternative are correlated with the other non-chosen alternatives. This also applies to the other regret formulations, Rmax (in Equation (11)) and Rlog (in Equation (12)), since all regret specifications are based on pairwise comparisonsFootnote2.
By using an error components (ECs) structure, total regret with an omitted variable can be formulated as:
(32)
(32)
(33)
(33)
(34)
(34)
The covariance matrix is shown in the Appendix.
3. Empirical evidence
In this section, the effect of omitted variables on the model performance in RRM and (linear-additive) RUM is empirically explored. Since the purpose of this paper is to analyze how the effect of omitted variables differs between utility and regret, in particular, this paper focuses on the issue of the effect of omitted variables for labeled choice alternatives. Two labeled data sets about transportation mode choice based on orthogonal stated choice experiments are used. The following analyses are conducted for both the RRM and RUM models: (i) the effect of an omitted variable is compared for both models; (ii) the effect of ASCs on mitigating such error is examined; (iii) the effect of the proposed error components structure for improvement model performance of RRM is investigated, and (iv) the model performance in the estimation results is determined by randomly splitting the data into K subsets. K–1 subsets are used to estimate the model (each subset is excluded once), and the average model performance is compared across subsets. Finally (v), the change in market share due to the omitted variable is discussed.
3.1. Data
The use of ASCs has been disputed. For instance, Hensher, Rose, and Greene (Citation2015) mentioned that a data set with labeled alternatives is preferred over one where the alternatives are not defined in terms of a label (called unlabeled alternatives), because the labeled alternatives enable us to study the important role of ASCs. When alternatives are unlabeled, they have no utility over and above the characteristics attributed to them in the experiment. Therefore, to clearly analyze the effect of the omitted variables and offset such effect by modeling, in this study, we used data from a stated preference survey based on an orthogonal design in context of labelled mode choice.
The data used in this study were collected in Beijing, China to analyze travel demand for electronic bikes compared to the other travel modes. Data were collected under a total of six scenarios. Two of these scenarios are used in this section. Each respondent was asked to choose his/her preferred transportation mode from choice sets consisting of two or three alternatives. Since RRM generates the same output as RUM in binary choice sets, only respondents faced with three alternatives were extracted.
3.2. Case study I
The first case study is about mode choice among alternatives labeled as E-bike, Car, and Bus. An orthogonal fractional factorial choice set design was constructed to systematically vary the attributes within and between modes. A total of 297 participants indicated for 9 choice sets their preferred transportation mode. The attribute levels are shown in (a).
Table 1. Attribute level.a
Table 2 shows the estimation results of the reference model and the curtailed models with an omitted variable. Each variable was omitted in turn. We applied the same error components for utility. However, most of parameters in the error components are estimated as zero and t-values are also zero. This implies that the error caused by omitted variables is correlated between alternatives in RRM, not in RUM. Therefore, they are not reported.
The omission of a variable causes a significant downward bias for all taste parameters, and a decrease in the model fit of both RUM and RRM (RRsum) models in all cases. This is because the variance of the errors grows and the scale goes down (see Guevara Citation2015). The predictive power is higher when omitting travel time than travel cost, implying that the effect of travel cost is more important than travel time to make a decision. The ASCs are significant and improve model fit in both utility and regret models, furthermore, the ECs show the best model fit in regret model.
In the case of omitting travel cost ((a)), the absolute value of the parameter for travel time is increased in the utility model. The signs of the ASCs are negative for both car and bus. The ASC is larger in size for car than bus. This implies that the omitted attributes cause larger unobserved (dis)utilities for car and bus, compared to e-bike. The signs of the ASCs are positive for both car and bus in the regret model, implying that the omitted attributes generate larger unobserved regrets for car and bus than e-bike. The largest value of ASC for car indicates that the unobserved regret is highest for car, implying that unobserved regret due to omitted attributes is largest for car, and smallest for e-bike. However, we should note here that discrete choice models assume that evaluation for an alternative are derived from the observed attributes (systematic part) and omitted attributes (error), and since regret is generated based on the pairwise comparisons of attribute values among alternativesFootnote3, the interpretation of ASCs in regret models is still questionable as we pointed out in formal analysis. The mean value of the ECs which is shared errors (unobserved regrets) between alternatives caused by omitted variables is the largest between car and bus, and it is the smallest between e-bike and bus. The standard deviation of the EC is the largest between e-bike and bus, implying that the unobserved regrets are more heterogeneous when comparing these modes.
Table 2. Estimation results – first case.
Table 3. Observed and predicted market shares – first case.
Table 4. Validation results – first case.
The results for omitted travel time ((b)) show that the ASCs have a negative sign for both car and bus in the utility model. However, while the ASC was the largest for the car when omitting travel cost, it is largest for bus when omitting travel time. In the regret models, the ASCs are positive for car and bus, and the value is larger for bus, and the mean EC is the largest between car and bus and the smallest between e-bike and car. These results lead different interpretations about omitted attributes: the results of ASCs indicate that the omitted attributes cause the largest (unobserved) regrets for bus in the choice set, whereas the results of ECs show that the omitted attributes generate the largest (unobserved) regrets between car and bus. Considering regret is generated based on attribute difference between alternatives, we argue that the interpretation of ECs is more consistent with the concept of regret. The standard deviation of EC is the largest for the comparison of car and bus, and the smallest for the comparison of e-bike and bus, indicating that the unobserved regret is the most heterogeneous when comparing car and bus.
The comparison of observed and predicted market shares is shown in Table 3. In order to measure the degree of accuracy of the predicted market shares, the root mean square error (RMSE) is used. Since the results with ASCs are the same as the results of the basic case (without ASCs), we do not attach the result separately in the table. Results indicate that whereas the utility models over-predict the market share of e-bike and car and under-predict the market share of the bike, regret models over-predict the market share of e-bike and bike and under-predict the car’s market share. The omission of a variable leads to reduced predictive power with respect to market share: The RMSE increases by omitting each variable, and commonly the omission of travel cost causes the higher increase in RMSE. Most importantly, the use of an error components structure is most efficient in regret models. The predictive market share becomes closer to the observed values. Thus, we can argue that it is important to consider the correlation between alternatives in regret models to reflect the effect of omitted variables.
To validate the results, in total 9 subsets were constructed, randomly assigning the choice tasks to the subsets. Of the 9 subsets, 8 subsets were used to estimate the models, and then the model performance was compared. This process is repeated 9 times by excluding each subset once. Table 4 shows the average model performance from the 9 subsets. Akin to the estimation results for the full data set: (i) the omission of an attribute significantly reduces goodness-of-fit of both RUM and RRM models, (ii) ASCs effectively improve model performance and the improvement appeared larger in utility model and (iii) the proposed ECs structure more effectively improve model performance than the ASCs in the RRM. However, the model performance is still worse than the full model.
3.3. Case study II
The data set used in this second case is about mode choice among E-bike, Taxi, and Metro. An orthogonal fractional factorial design was constructed to independently vary the attributes within and between modes. A total of 332 participants provided their preferred choice for 9 choice sets. The attribute levels are shown in (b).
The detailed estimation results of the reference and curtailed models are shown in Table 5. Travel time and cost are omitted respectively. As in the first case, the omission of an attribute causes the downward bias in the remaining parameter. The reduction in model fit is higher when omitting travel cost than travel time.
The results of the omission of travel cost ((a)) show that, in the utility model, the ASCs improve model fit and the parameter for travel time shifts upward. The values of the ASCs are larger in size for taxi compared to metro. In the regret model, both the ASCs and ECs improve model fit and shift the parameter for travel time upward. The ECs show the best model fit. The ASCs show the higher value for taxi than metro, indicating that the regret due to the omitted attributes (excluding travel time) is the largest for taxi and smallest for e-bike. The ECs have the largest mean value between taxi and metro, and the smallest between e-bike and taxi. The standard deviation of the EC is the largest for the comparison of taxi and metro, implying that the generated regrets are more heterogeneously when comparing taxi and metro.
Table 5. Estimation results – second case.
Table 6. Observed and predicted market shares – second case.
Table 7. Validation results – second case.
In the case of the omission of travel cost ((b)), the results of the utility model show that the ASCs improve model fit and make the parameter shift upward. As in the former case, the ASCs are largest in size for taxi, meaning that the omitted attributes generate the largest (dis)utility for taxi and the smallest for e-bike. It can be interpreted as that the omitted attributes excluding travel cost cause larger (unobserved) regret for taxi and smaller regret for e-bike. The use of ECs results in the best model fit, compared to the basic and ASCs models. As in the case of omission of travel cost, the mean value of EC is the largest for the comparison of car and bike, and the smallest for the comparison between e-bike and taxi. This implies that the (unobserved) regret generated from the omitted attributes (except for travel cost) are the largest when comparing car and bike, and smallest between e-bike and taxi. The standard deviation of the EC is smallest for the comparison of e-bike and metro, implying that the regrets are less heterogeneously between the alternatives.
Table 6 shows the predicted market shares for each model compared to the observed values. Overall, the market share of taxi is over-predicted in all models. While utility models under-predict the share of metro, the e-bike’s market share is under-predicted in regret models. The overall tendency is similar to the findings of the first case study. Still, the use of EC is effective in the regret models and improves the accuracy of the predicted market shares in regret models.
The estimation results in this second case study were also validated. The data were randomly split into 8 different subsets, and the models were estimated pooling 7 of these subsets (each subset was excluded once respectively). The average model performance for the models is listed in Table 7. The results from 8 combinations of subsets are consistent with the estimation results for the full dataset: The proposed ECs structure effectively offsets bias by omitted variables in RRM, similar to ASCs in RUM.
4. Conclusions and discussion
Random regret minimization models have almost invariably assumed that the error terms of the regret function are identically and independently Gumbel distributed, leading to a logit-type probability distribution. The validity of this assumption has not received much attention and very few studies have empirically examined the effect of omitted variables.
Through formal analysis, this paper showed that whereas the effect of omitted variables in the (linear-additive) RUM model is alternative-specific, it is correlated between alternatives in the RRM model. This implies that whereas ASCs are appropriate to represent the average effect of omitted variables in (linear-additive) RUM models, they are not in RRM models. This seems reasonable considering that attribute-level regret is defined in the comparison of alternatives, implying that the regrets are correlated, and errors are also correlated. Therefore, an error component structure is proposed to consider the effect of omitted variables in RRM models. By using two empirical data sets, the effect of omitted variables is tested and the empirical results of ASCs and ECs are discussed. The results are consistent with the formal analysis. Although both ASCs and ECs significantly capture the effect of omitted attributes, the ECs show better performance by providing alternative interpretation of the effect.
A few valuable directions for further research can be identified. First, the current empirical analysis is only based on the RRsum model. However, as shown in the formal analysis, since regret is defined in the comparison of alternatives in all regret models, omitted variables cause correlation between error terms in all these models, only the degree of correlation is different. Therefore, it would be interesting in future research to examine how correlations introduced by omitted variables differ for the different specifications of regret. Second, Van Cranenburgh and Prato (Citation2016) showed that the effect of omitted variables depends on choice set composition. Since the proposed error components structure for regret can easily represent choice set composition effects, empirical analyses of data with varying choice set composition would be worthwhile to conduct. Finally, certain variables are not independent of other explanatory variables. For instance, crowding in public transportation may depend on travel cost. Therefore, if the variable ‘crowding' is omitted, it may induce endogeneity. Several methods have been developed to capture endogeneity (e.g. Rivers and Vuong Citation1988; Train Citation2009; and Guevara Citation2015). In this case, more complex assumptions about error terms are necessary. Empirically, it may occur when analyzing revealed preference data. Whereas current methodologies have been developed to consider endogeneity in (linear-additive) RUM models such as the BLP method (e.g. Berry, Levinsohn, and Pakes Citation1995) and the control function method (e.g. Hausman Citation1978), the issue how to consider endogeneity in RRM models considering the correlation between alternatives needs further investigation.
Acknowledgement
The authors would like to thank Qiong Tian (Beihang University) for providing the data used in this study.
Disclosure statement
No potential conflict of interest was reported by the authors.
Notes
1 Note that we can also use an asymmetrical relationship. However, in this case, the number of additional parameters is at least doubled, compared to the symmetrical relationship.
2 Note that since regret-based choice models represent semi-compensatory behavior (Chorus, Arentze, andTimmermans Citation2008; Chorus Citation2012b), if the chosen alternative is superior to the non-chosen alternatives with respect to a certain attribute b for individual n, then the omission of attribute b does not cause any error in the regret for chosen alternative. However, if we also consider rejoice, the counterpart of regret, the omission causes error in rejoice, and the bias would be larger.
3 Even though some attributes are alternative specific, the effect of attributes in regret model still concerns the comparison of alternatives. For instance, the effect of headway in bus can be analyzed by fixing the values of other alternatives as zero. Then, the regret for bus from the headway is
, where
. Therefore, the longer bus headway generates higher regret for the bus alternative, while it does not affect the other alternatives:
. Therefore, a higher bus headway decreases the choice probability of the bus. Note that in this case the regrets are correlated by
. Therefore, the application of ASCs is still questionable.
References
- Arrow, K. J. 1951. Social Choice and Individual Values. New York: John Wiley and Sons.
- Bell, D. E. 1982. “Regret in Decision Making Under Uncertainty.” Operations Research 30 (5): 961–981. doi: 10.1287/opre.30.5.961
- Ben-Akiva, M., and S. Lerman. 1985. Discrete Choice Analysis: Theory and Application to Travel Demand. Cambridge, MA: MIT Press.
- Berry, S., J. Levinsohn, and A. Pakes. 1995. “Automobile Prices in Market Equilibrium.” Econometrica 63: 841–889. doi: 10.2307/2171802
- Boeri, M., and L. Masiero. 2014. “Regret Minimisation and Utility Maximisation in a Freight Transport Context.” Transportmetrica A: Transport Science 10 (6): 548–560. doi: 10.1080/23249935.2013.809818
- Chorus, C. G. 2010. “A New Model of Random Regret Minimization.” European Journal of Transport and Infrastructure Research 10 (2): 181–196.
- Chorus, C. G. 2012a. “Logsums for Utility-Maximizers and Regret-Minimizers, and Their Relation with Desirability and Satisfaction.” Transportation Research Part A: Policy and Practice 46 (7): 1003–1012.
- Chorus, C. G. 2012b. Random Regret-Based Discrete Choice Modeling: A Tutorial. Heidelberg: Springer.
- Chorus, C. G. 2014. “Benefit of Adding An Alternative to One's Choice Set: A Regret Minimization Perspective.” Journal of Choice Modelling 13: 1–11. doi: 10.1016/j.jocm.2014.04.001
- Chorus, C. G., T. A. Arentze, and H. J. P. Timmermans. 2008. “A Random Regret Minimization Model of Travel Choice.” Transportation Research Part B: Methodological 42 (1): 1–18. doi: 10.1016/j.trb.2007.05.004
- Guevara, C. A. 2015. “Critical Assessment of Five Methods to Correct for Endogeneity in Discrete-Choice Models.” Transportation Research Part A: Policy and Practice 82: 240–254.
- Hausman, J. 1978. “Specification Tests in Econometrics.” Econometrica 46 (6): 1251–1272. doi: 10.2307/1913827
- Hensher, D. A., J. M. Rose, and W. H. Greene. 2015. Applied Choice Analysis. 2nd ed. Cambridge, UK: Cambridge University Press.
- Jang, S., S. Rasouli, and H. J. P. Timmermans. 2017. “Bias in Random Regret Models Due to Measurement Error: Formal and Empirical Comparison with Random Utility Model.” Transportmetrica A 13 (5): 405–434. doi: 10.1080/23249935.2017.1285366
- Johnson, N. L., S. Kotz, and N. Balakrishnan. 1994. Continuous Multivariate Distributions, Volume 1: Models and Applications. New York: John Wiley and Sons.
- Loomes, G., and R. Sugden. 1982. “Regret Theory: An Alternative Theory of Rational Choice Under Uncertainty.” The Economic Journal 92: 805–824. doi: 10.2307/2232669
- Loomes, G., and R. Sugden. 1983. “A Rationale for Preference Reversal.” The American Economic Review 73 (3): 428–432.
- Loomes, G., and R. Sugden. 1987. “Some Implications of a More General Form of Regret Theory.” Journal of Economic Theory 41 (2): 270–287. doi: 10.1016/0022-0531(87)90020-2
- Manski, C. 1973. “The Analysis of Qualitative Choice.” PhD diss., Department of Economics, Massachusetts Institute of Technology.
- Masiero, L., Y. Yang, and R. Qiu. 2019. “Understanding Hotel Location Preference of Customers: Comparing Random Utility and Random Regret Decision Rules.” Tourism Management 73: 83–93. doi: 10.1016/j.tourman.2018.12.002
- McFadden, D. 1974. “Conditional Logit Analysis of Qualitative Choice Behavior.” In Frontiers in Econometrics, edited by P. Zarembka, 105–142. New York: Academic Press.
- Ortuzar, J. de D., and L. G. Willumsen. 2011. Modelling Transport. 4th ed. New York: John Wiley and Sons.
- Quiggin, J. 1994. “Regret Theory with General Choice Sets.” Journal of Risk and Uncertainty 8 (2): 153–165. doi: 10.1007/BF01065370
- Rasouli, S., and H. J. P. Timmermans. 2014. “Regret-Based Models of Consumer Choice of Shopping Center.” Proceedings of the 21th RARCS Conference, Bucharest, Romania.
- Rasouli, S., and H. J. P. Timmermans. 2017. “Specification of Regret-Based Models of Choice Behavior: Formal Analyses and Experimental Design Based Evidence.” Transportation 44 (6): 1555–1576. doi: 10.1007/s11116-016-9714-6
- Rivers, D., and Q. H. Vuong. 1988. “Limited Information Estimators and Exogeneity Tests for Simultaneous Probit Models.” Journal of Econometrics 39 (3): 347–366. doi: 10.1016/0304-4076(88)90063-2
- Sharma, B., M. Hickman, and N. Nassir. 2019. “Park-and-Ride Lot Choice Model Using Random Utility Maximization and Random Regret Minimization.” Transportation 46 (1): 217–232. doi: 10.1007/s11116-017-9804-0
- Tardiff, T. T. 1978. The Use of Alternative Specific Constants in Choice Modelling. Working paper, Institute of Transportation Studies ITS-SP-78-6, University of California at Irvine, California.
- Train, K. E. 2009. Discrete Choice Analysis with Simulation. 2nd ed. Cambridge, UK: Cambridge University Press.
- Van Cranenburgh, S., C. A. Guevara, and C. G. Chorus. 2015. “New Insights on Random Regret Minimization Models.” Transportation Research Part A: Policy and Practice 74: 91–109.
- Van Cranenburgh, S., and C. G. Prato. 2016. “On the Robustness of Random Regret Minimization Modelling Outcomes towards Omitted Attributes.” Journal of Choice Modeling 18: 51–70. doi: 10.1016/j.jocm.2016.04.004
- Washington, S., M. C. Karlaftis, and F. L. Mannering. 2010. Statistical and Econometric Methods for Transportation Data Analysis. 2nd ed. Boca Raton, FL: Chapman and Hall/CRC.
Appendix. Variance-covariance matrix
As discussed, we assume that each error component follows a lognormal distribution .
where
is a random variable following a standard normal distribution.
According to the Johnson, Kotz, and Balakrishnan (Citation1994) the variance of the lognormal distributed random variable is:
Therefore, the variance of total regret for alternative 1 in Equation (32) can be obtained (see Hensher, Rose, and Greene Citation2015):
As in the same manner, the variance-covariance matrix from Equations (32–34) is: