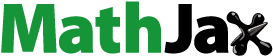
ABSTRACT
Several mechanisms of predictive language processing have been proposed. The possible influence of mediating factors such as working memory and processing speed, however, has largely been ignored. We sought to find evidence for such an influence using an individual differences approach. 105 participants from 32–77 years of age received spoken instructions (e.g. “Kijk naar deCOM afgebeelde pianoCOM”– look at the displayed piano) while viewing 4 objects. Articles (Dutch “het” or “de”) were gender-marked such that the article agreed in gender only with the target. Participants could thus use article gender information to predict the target. Multiple regression analyses showed that enhanced working memory abilities and faster processing speed predicted anticipatory eye movements. Models of predictive language processing therefore must take mediating factors into account. More generally, our results are consistent with the notion that working memory grounds language in space and time, linking linguistic and visual–spatial representations.
Language processing tends to be remarkably fast, efficient, and accurate. This is at least partly due to that developing (e.g. Borovsky, Elman, & Fernald, Citation2012; Mani & Huettig, Citation2012, Citation2014; Nation, Marshall, & Altmann, Citation2003) and mature (e.g. Altmann & Kamide, Citation1999; DeLong, Urbach, & Kutas, Citation2005; Kamide, Altmann, & Haywoo, Citation2003; Van Berkum, Brown, Kooijman, Zwitserlood, & Hagoort, Citation2005) language users anticipate upcoming language input. Many studies investigating anticipatory language processing have focused on the cues used for prediction (e.g. what types of information are used to anticipate upcoming words) and the contents of prediction (e.g. what types of representations are pre-activated).
Reading studies, for example, have shown that readers can make use of transitional probabilities (i.e. word co-occurrence statistics, McDonald & Shillcock, Citation2003) to predict upcoming words. ERP studies have shown that language users are able to use sentence (Federmeier & Kutas, Citation1999) and discourse context (Van Berkum et al., Citation2005) for prediction. In addition, visual world eye-tracking studies (see Huettig, Rommers, & Meyer, Citation2011 for review) have shown that listeners are also able to use case-marking (Kamide, Scheepers, & Altmann, Citation2003), gender-marking (Huettig & Brouwer, Citation2015), prosody (Weber, Grice, & Crocker, Citation2006), and visually presented events (Knoeferle, Crocker, Scheepers, & Pickering, Citation2005). With regard to the contents of predictions, reading studies have found evidence for the prediction of syntactic structures (Chen, Gibson, & Wolf, Citation2005; Staub & Clifton, Citation2006). ERP studies have also demonstrated that language users can pre-activate the semantic/conceptual features (Federmeier & Kutas, Citation1999; Federmeier, McLennan, Ochoa, & Kutas, Citation2002), morphosyntactic features (Van Berkum et al., Citation2005; Wicha, Moreno, & Kutas, Citation2003, Citation2004), the phonological form (DeLong et al., Citation2005), and the orthographic form (Laszlo & Federmeier, Citation2009) of up-coming words. In addition to the aforementioned representations, even the visual form of a predicted concept has been shown to be anticipated (Rommers, Meyer, & Huettig, Citation2015; Rommers, Meyer, Praamstra, & Huettig, Citation2013).
Over recent years, researchers have begun to focus on the mechanisms of anticipatory language processing. Some linguistic approaches assume that differences in the probability distribution of possible syntactic structures drive prediction (e.g. Hale, Citation2001; for experimental evidence see Arai & Keller, Citation2013). Recent influential accounts assume that we use the language production system covertly to anticipate what the other person or oneself might be likely to say (e.g. Chang, Dell, & Bock, Citation2006; Dell & Chang, Citation2014; Federmeier, Citation2007; Pickering & Garrod, Citation2007, Citation2013; and Mani & Huettig, Citation2012 for experimental evidence but see also Drake & Corley, Citation2014). It is also possible that predictive language processing is at least partly driven by low-level associations e.g. Kuperberg, Citation2007; Pickering & Garrod, Citation2013; for experimental evidence see Kukona, Fang, Aicher, Chen, & Magnuson, Citation2011) and combinatorial mechanisms (e.g. Kuperberg, Citation2007). Other accounts assume an important role for event knowledge (e.g. Altmann & Mirkovich, Citation2009; Metusalem et al., Citation2012), Indeed, it is likely that multiple-mechanism accounts are required to provide a full picture of anticipatory language processing (Huettig, in press; Mani & Huettig, Citation2013).
It is noteworthy that so far very little research has investigated the influence of mediating factors on prediction in language processing. The role of working memory and general processing speed on anticipatory language processing, for instance, has hardly been looked at. There are several reasons why ignoring mediating factors is problematic. First, it is very likely that mediating factors modulate anticipation in some situations but less in others. Anticipatory eye movements in the visual world (which occur in many real-world situations such as when we give and receive directions or comment on the state of our visual surroundings), for example, require the building of a representational network (i.e. online models) allowing for visual objects to be linked to unfolding linguistic information, places, times, and each other. It is therefore likely that working memory is particularly important for anticipatory processing in a situation when spoken language is used in relation to a co-present visual environment. Second, it is conceivable that mediating factors interact in particular ways with different mechanism of prediction. Perhaps working memory capacity is more important for combinatorial mechanisms of prediction (cf. Kuperberg, Citation2007) than for simple associative mechanisms (cf. Kuperberg, Citation2007; Pickering & Garrod, Citation2013). Third, theoretical models of predictive language processing will only be complete if they can fully account for the interplay of individual differences in the mediating factors and the mechanisms of prediction.
Indeed, the idea that working memory may be important for language–vision interactions is not new (Huettig, Olivers, & Hartsuiker, Citation2011; Knoeferle & Crocker, Citation2007; cf. Spivey, Richardson, & Fitneva, Citation2004). Huettig, Olivers, et al. (Citation2011) propose that working memory grounds language in space and time, allowing for short-term connections between objects and linking linguistic and visuospatial representations. Similar proposals have been made in vision research. Vision researchers have suggested that visual objects are “instantiated” and that type representations are bound to a specific location and moment in time (e.g. an object file, token, or index, Kahneman, Treisman, & Gibbs, Citation1992; Kanwisher, Citation1987; Pylyshyn, Citation2001). Huettig, Olivers, et al. (Citation2011) suggest that working memory enables us to link language to the here and now, or, when anticipating things, the there and then. If this notion is correct, then individual differences in working memory should influence language-mediated anticipatory eye movements. In the present study, we sought to find evidence for such an influence of working memory using an individual differences approach.
The notion that general processing speed and cognitive efficiency may impact on anticipatory language processing is also not entirely new. General processing speed has been linked to individual differences in many cognitive tasks (e.g. Kail & Salthouse, Citation1994; Salthouse, Citation1996). Furthermore, age-related cognitive decline has been accounted for as an effect of slowing of the speed with which processing operations can be executed (Salthouse, Citation1996). Processing speed may play a role in sentence processing if it indexes the speed with which information can be retrieved from long-term memory, and the speed with which unfolding information can be integrated into a representation of the sentence meaning. Good connectivity between different neural processing regions may contribute to a high speed of information integration. Peelle, Troiani, Wingfield, and Grossman (Citation2010), for example, investigated neural connectivity in younger and older adults and found that, compared to younger adults, neural connectivity in the older adults was reduced. Peelle et al. (Citation2010) argued that the pattern of reduced coordination of activity between processing regions may relate to older adults’ difficulty with sentence comprehension in certain situations (e.g. difficult listening conditions).
An important concern when assessing performance on any task is of course that participants in the experiments are a representative sample of the whole population. It has been argued that the student participants used in most experiments in experimental psychology are the WEIRDest (Western Educated Industrialized Rich Democratic) people in the world from which to draw general conclusions about human behaviour (Henrich, Heine, & Norenzayan, Citation2010; see also Arnett, Citation2008). In the present study, we made an explicit attempt to get a more heterogeneous sample of participants. Our adult participants were of varying ages and educational backgrounds, such that the sample is potentially more variable in working memory and processing speed than in typical university student samples. Working memory and speed decline with advancing adult age (Hultsch, Hertzog, Small, McDonald-Miszczak, & Dixon, Citation1992; Park et al., Citation2002). Differences in working memory have been linked to individual differences in speech perception in noisy conditions (Akeroyd, Citation2008; Rönnberg et al., Citation2013). Additionally, verbal working memory ability has been shown to predict older adults’ ability to use context information for the recognition of upcoming words in listening to sentences (Janse & Jesse, 2014). These results suggest that those with better working memory are better able to keep and update a coherent representation of the sentence content in working memory.
Predictive language processing in older adults has mainly been investigated in reading studies. Rayner, Reichle, Stroud, Williams, and Pollatsek (Citation2006) suggested that older readers adopt a “riskier” reading strategy than younger adult readers, with older readers more often skipping words, possibly on the basis of their guess of what the next word will be. This finding could be interpreted as indicating that older adults predict more than younger adults to compensate for age-related cognitive decline. It should be noted, however, that the older adults also regressed more to earlier words, and that predictability effects on older readers’ fixation data were found to be equally large as for younger readers (Rayner et al., Citation2006). Another line of research has measured ERPs and used word-by-word reading to investigate younger and older adults’ semantic integration of final words in sentences varying in semantic constraint (e.g. Federmeier & Kutas, Citation2005). Federmeier and colleagues repeatedly found that older adults showed smaller and delayed effects of contextual constraint compared to young adults, which was attributed to decreased reliance on predictive processing in older age (Federmeier, Kutas, & Schul, Citation2010; Huang, Meyer, & Federmeier, Citation2012; Wlotko & Federmeier, Citation2012). Wlotko and Federmeier (Citation2012) speculate, in line with the argument put forward by Peelle et al. (Citation2010), that older adults’ decreased predictive processing may be due to less-efficient functional connectivity, or that predictive processing has become too costly or inefficient for older adults due to decreased availability of neural resources.
Present study
We conducted an eye-tracking experiment and administered several control tasks to assess whether working memory and processing speed independently contribute to language-mediated anticipatory eye movements in adult native speakers of Dutch. In order to reduce the likelihood that anticipation would be driven by semantically fitting thematic referents (Kamide, Altmann, & Haywoo, Citation2003; cf. Huettig & Altmann, Citation2005) and/or simple word associations (e.g. between verb and noun, e.g. censure and newspaper as in “to censure the newspaper”, or weave and cloth as in “to weave the cloth”), we presented participants with simple Dutch spoken instructions such as “kijk naar de afgebeelde piano” (look at the displayed piano) as they were looking at the target object (e.g. piano) and three unrelated distractor objects. This way the Dutch article “de” or “het” was the only cue in the sentence that could be used for anticipation. Note that Dutch has a two-way grammatical gender system and makes a distinction between common and neuter gender. Gender is marked on a number of agreeing elements accompanying the noun or referring to it such as determiners, adjectives, demonstratives, and pronouns (Blom, Polišenská, & Weerman, Citation2008, for further discussion). For the present study we used definite nouns, which are preceded by the definite determiner de (common nouns), as in de piano “the piano” or by the definite determiner het (neuter nouns), as in het paard “the horse”. Participants therefore could use gender information from the article for prediction of the upcoming noun, as targets but not unrelated distractors agreed in gender with the article presented in the spoken sentence.
We assessed individual differences in working memory using an auditory nonword repetition (NWR) task as an index of verbal/phonological short-term memory (Gathercole & Baddeley, Citation1996; Thorn & Gathercole, Citation1999) and a backwards digit span task which is more appropriate to measure the manipulation of items in memory rather than their storage and reproduction. In order to assess spatial working memory, we used the Corsi block tapping task (Corsi, Citation1972). To measure general processing speed, we used a digit–symbol substitution (DSS) test and a letter comparison task. Finally, to make sure that any potential individual differences would not just reflect non-verbal intelligence (often referred to as the “g-factor”), we also administered Raven's progressive matrices to participants.
Experiment
Participants
One hundred and five participants (21 of whom were male) were drawn from the Max Planck Institute for Psycholinguistics participant pool and were paid for their participation. They were all native speakers of Dutch, and none of them wore hearing aids. Their age varied from 32 to 77 years, with a mean age of 55.75 (SD = 9.81). Twenty-nine of these participants were aged over 60. Participants were tested without glasses or with glasses or contact lenses if they reported impaired vision. Participants were also given plenty of preview of the visual objects (4000 ms) before the onset of the spoken instruction so that they could recognise the objects for what they were.
Hearing sensitivity was assessed with a Maico ST20 portable audiometer (air conduction thresholds only) for both ears at octave frequencies from 250 Hz to 8 kHz. As the majority of our participants were aged below 60, we did not use the high-frequency hearing loss index (PTAhigh averaged over 1, 2, and 4 kHz, which is often used to index age-related hearing loss) but used the pure-tone average (PTA) threshold in the participant's better ear averaged over 0.5, 1, and 2 kHz (the standard PTA). However, due to logistic reasons (availability of the audiometer), hearing data were not available for all participants. Hence, hearing data are missing for 25 participants, all aged below 60, and all reported to have normal hearing. For the remaining 80 participants, mean PTA was 12.73 (SD = 6.41) (range = 0–33, and note that higher values indicate poorer hearing). PTA values in between 26 and 40 dB represent mild hearing loss, such that all participants in the sample had relatively normal hearing.
Materials and design
Participants received 40 spoken instructions (e.g. “kijk naar de afgebeelde piano” – look at the displayed piano; or “kijk naar het afgebeelde paard” – look at the displayed horse). Twenty of the instructions contained common gender words and 20 others neuter gender words. For example, on one trial ((a)) the target object piano was a “de word” but the three unrelated distractors (pig, paper, and plate) were neuter gender (“het”) words. Conversely, on some other trials ((b)) the target was a neuter gender (“het”) word (e.g. “paard” – horse) but the three unrelated distractors (scissors, shark, and screwdriver) were “de words”. Instructions containing common and neuter gender nouns were randomised. The word “afgebeelde”, displayed, was inserted between article and noun in the spoken instructions to ensure participants had ample time to anticipate the target object. The instructions were read aloud with a neutral intonation contour by a female native speaker of Dutch in a sound-damped booth. Digital recordings (sample rate 44.1 kHz, 16 bit sampling resolution) were stored on a computer. The average noun onset occurred 2009 ms after article onset.
Figure 1. Example displays for one “de” trial (a), with the target: de piano, and three “het” unrelated distractors) and a “het” trial (b), with the target: het paard (horse), and three “de” unrelated distractors).
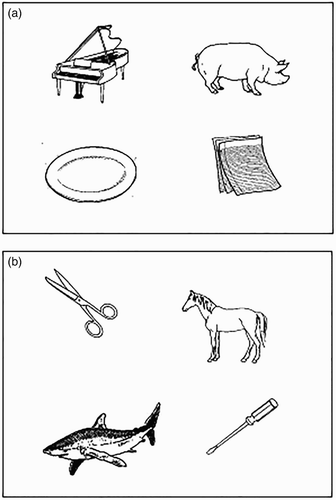
Pictures were line drawings taken from the Severens, Van Lommel, Ratinckx, and Hartsuiker, (Citation2005) set and were matched for CELEX word frequency, number of picture names, h-statistic (which compensates for overestimating name agreement when participants assigned many different names infrequently and one single name very frequently), and picture naming time.
Individual differences measures
Working memory: nonword repetition
An auditory NWR task was used as an index of verbal/phonological short-term memory (Gathercole & Baddeley, Citation1996; Thorn & Gathercole, Citation1999). Others have referred to this task as indexing phonological storage (e.g. Gathercole, Citation2006), phonological buffer capacity (Bates, Luciano, Montgomery, Wright, & Martin, Citation2011), or phonological working memory (Gathercole, Willis, Baddeley, & Emslie, Citation1994; McGettigan et al., Citation2011). The task has been widely used in research on developmental dyslexia (e.g. Ramus & Szenkovits, Citation2008) and specific language impairment (Botting & Conti-Ramsden, Citation2001).
The task consisted of the presentation of 50 nonwords, all of which were phonotactically legal in Dutch (de Jong & van der Leij, Citation1999). The nonword items were presented over headphones at a fixed mean presentation level of 70 dB SPL for all participants. Participants were seated in a sound-attenuating booth. Each nonword was presented only once, after which participants were asked to repeat the nonword. Inter-trial time was three seconds. Nonwords of different syllable lengths (two to five syllables long) were presented intermixed, but the order in which they were presented was kept constant for all participants. Due to technical failure, NWR data for two participants were missing. Mean score for this task was 38.55 (out of a total of 50, SD = 4.14), and ranged from 23.32 to 46.77. Higher scores reflected better auditory verbal short-term memory.
Working memory: backward digit span
A backwards-recall digit span task (a subpart of the Wechsler Adult Intelligence Test, Citation2004) was used to measure individual working memory capacity. Since the backwards-recall variant of this task requires manipulation of presented materials, rather than storage and reproduction, the task is considered to be an index of working, rather than short-term, memory (Baddeley, Citation2006). We added a general working memory task such as digit span because we were interested in the influence of general working memory capacity in addition to the influence of verbal/phonological working memory (as assessed by NWR, which is more directly related to the spoken language input) and visuospatial working memory (cf. Corsi blocks measure below, which is more directly related to the visual input). In the computerized variant of this task used here, a series of digits was shown sequentially in the centre of the computer screen. Each digit was presented for one second and with one second in between consecutive digits. Digits were presented in a large white font (Arial, font size 100) against a black background. After presentation of the digit sequence (e.g. 3 6 2), the participant was prompted to recall the digits in the reverse order (e.g. 2 6 3). Participants typed in their responses with a computer keyboard. Participants were first presented with two three-digit trials to become familiarized with the task. They were then tested on digit sequences of two up to eight digits (two trials for each sequence length, making up 14 trials in total). Note that participants were asked to proceed to up to eight-digit sequences, regardless of their performance on shorter digit sequences. Data for one participant were missing due to technical failure. Individual working memory performance was operationalised as the number of correctly recalled digit sequences (out of 14 test trials). Mean number of correct trials in this task was 6.92 (SD = 2.28, range 2–12): the higher the score, the better working memory this participant has.
Spatial working memory: Corsi block task
The visual Corsi block tapping task (Corsi, Citation1972) was used as a measure of visuospatial short-term memory performance. A computerized variant of this task was used here, in which participants saw a pattern of nine identical blocks, irregularly positioned on the computer screen. A number of these blocks are then highlighted at a rate of one block per second. The participant's task is to click the same blocks in their order of highlighting. The task gradually becomes more challenging as the length of the block sequences increases from 2 to 9, with two trials for each block sequence length (resulting in 16 trials in total). Individual performance on this task was coded as the total number of correctly imitated trials (out of the total of 16 trials): the higher the score, the better spatial short-term memory. Mean number of correct trials was 7.05 (SD = 2.24) and ranged from 0 to 12.
Processing speed: DSS
Participants performed the DSS test, which is a pencil-and-paper subtest of the Wechsler Adult Intelligence Test (Citation2004). Participants are provided with a key of 9 different symbols, each symbol paired with one of the numbers from 1 to 9. Participants then perform an assignment in which they have to substitute rows of numbers for their corresponding symbols, by writing the symbol below each number. Participants get 90 seconds to substitute as many digits for symbols as possible (the maximum number of digits to be substituted is 133). DSS test scores have been related to perceptual speed or processing speed (Hoyer, Stawski, Wasylyshyn, & Verhaeghen, Citation2004; Salthouse, Citation2000). Substitution time per symbol was calculated by dividing 90 seconds by the number of symbols the participant had coded, such that higher scores indicate poorer (i.e. slower) performance. Mean substitution time per symbol was 1.67 sec (SD = 0.41), and ranged from 0.97 to 3.21.
Processing speed: letter comparison
This task was based on a paper-and-pencil task thought to index processing speed as described in Earles and Salthouse (Citation1995) and Salthouse (Citation1996). Participants were presented with two-letter strings (all consonants) on a computer screen: one centred in the top half of the screen, and one string centred in the lower half. Participants were asked to decide as quickly and as accurately as possible whether the two-letter strings were same or different by pressing buttons on a response button box labelled “same” or “different”. The first experimental block consisted of letter strings made up of three letters (e.g. TZF). The second block consisted of six-letter strings (e.g. RNHKTG) to be compared. Letters were presented in a large black font (Arial 60) against a white background. Within each block, 12 trials contained identical strings and 12 trials contained different strings, making up 48 trials in total. If the strings were different, they would only differ in one letter in any of the three (for the three-letter strings) or six (for the six-letter strings) positions. Participants were first presented with six practice trials representing three “same” and three “different” trials. Each trial started with the presentation of a fixation cross, which stayed on the screen for 500 ms. After another 100 ms, the two-letter strings would be presented and stayed on the screen until the participant had responded. The next trial was presented after an inter-trial time of 1000 ms. Mean accuracy proportion over the 48 test trials was 0.95 (SD = 0.04, range 0.83–1). For all correct responses, an reaction time (RT) cut-off criterion of 3 SDs above the grand mean was calculated. This led to a further exclusion of 1.3% of the data points (for each participant at least 37 out of 48 trials were left; overall 93.3% of the original trials). On the basis of this dataset (incorrect and extremely slow responses excluded), each participant's mean RT was calculated and was entered as individual speed. Mean letter comparison RT was 1526 ms (SD = 285), and RTs ranged from 1002 to 2302 ms. A higher RT value corresponds to a lower processing speed.
Non-verbal intelligence: Raven's matrices
Raven's advanced progressive matrices were administered as a measure of non-verbal intelligence. In the computerized version we used here, participants had to indicate which out of eight possible shapes completed a matrix of geometric patterns by clicking on it with a computer mouse. Target matrices were presented as large pictures in the centre of the screen, and below the target matrix were always two rows of four pictures each to represent the eight options. Items could be skipped to be presented again at the end. Participants were given 20 minutes to complete the 36 items. The time was indicated in the top right corner of the screen. Individual performance was the total number of correct responses. Mean score was 14.87 (SD = 5.95), and scores ranged from 3 to 28. All individual differences measures were normally distributed (according to Kolmogorov–Smirnov testing for normality).
Procedure
Participants were tested individually and seated at a comfortable distance from the computer screen. Eye movements were recorded with an SR Research Eyelink 1000 Tower mount system sampling at 1000 Hz. The system was calibrated using the standard Eyelink set-up. The positions of the pictures were randomised. The spoken instructions were presented via headphones at a fixed mean presentation level of 70 dB SPL. At the beginning of each trial, a central fixation dot appeared allowing for drift correction. Then the visual displays appeared. Auditory presentation of the spoken sentences was initiated 4000 ms after the pictures appeared on the screen. After the onset of the spoken instruction, the display remained on the screen for another 5000 ms followed by a 500 ms blank screen. Participants were told to listen carefully to the instructions of the speaker (e.g. “look at the displayed piano”). They were reminded not to take their eyes off the screen if possible. The whole experiment lasted around 20 minutes. After participants finished the eye-tracking experiment, the individual differences measures were administered.
Data coding procedure
Data for the eye-tracking experiment were coded as fixations, saccades, or blinks using the Eyelink algorithm. The timing of the fixations was established relative to the onset of the critical article (“de” or “het”) in the spoken instructions. Gaze position was categorised by object quadrant. Fixations were coded as directed to the target objects, or the unrelated distractors.
Results
The data were analyzed using a magnitude estimation approach. Cumming (Citation2014) points out that the field needs to change towards a cumulative quantitative science. Cumming argues (convincingly in our view) that to make progress researchers should strive to avoid invoking null-hypothesis testing and interpret results by using measures of effect sizes and confidence intervals. Indeed, Fidler and Loftus (Citation2009) provide evidence that reporting confidence intervals leads to much better interpretation of the results than a research report based on null-hypothesis testing (see Cumming, Citation2012, Citation2014, for extensive discussion).
shows a time-course graph of the fixation proportions to the target objects specified in the instruction and the averaged unrelated distractors plotted from the acoustic onset of the spoken article (“de” or “het”) until 3000 ms post-article onset. By-participant 95% confidence intervals were computed at every sampling step for target and averaged distractor proportions. The area shaded in grey in the graph represents the upper and lower bounds of the 95% confidence intervals for target and distractor looks across time. The graph shows that participants anticipated the target object (i.e. looks to the target objects diverged from the looks to the unrelated distractors) well before noun onset.
Figure 2. Time-course graph of fixation proportions to targets and averaged distractors. Zero on the time line refers to the acoustic onset of the article. The red solid line represents looks to the target objects; the dashed red line represents looks to the averaged distractors. The areas shaded in grey in the graph represent the upper and lower bounds of the 95% confidence intervals. The dashed vertical represents the average acoustic onset of the target words.
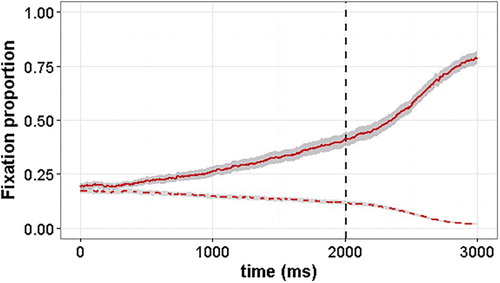
shows a time-course graph of log-transformed difference scores between target and distractor fixations. Fixation proportions were transformed logistically, and zeroes and ones were replaced by 0.01 and 0.99 (cf. Macmillan & Creelman, Citation1991). The dependent variable is the ratio of the transformed target and distractor looks as a function of time using the following log-ratio (cf. Arai, Van Gompel, & Scheepers, Citation2007):
Figure 3. Time-course graph of fixation proportions of log-transformed difference scores between target and distractor fixations. Zero on the time line refers to the acoustic onset of the article. Equal proportions of looks yield a score of zero, higher proportions on the targets result in a positive score, and higher proportions on the distractor result in a negative score. The area shaded in grey represents the upper and lower bounds of the 95% confidence intervals of the difference scores. The dashed vertical represents the average acoustic onset of the target words.

where P(T) refers to the probability of gazes on the target objects and P(D) refers to the probability of gazes on the averaged distractor objects. The measure is symmetrical around zero such that equal proportions of looks yield a score of zero, higher proportions on the targets result in a positive score, and higher proportions on the distractor result in a negative score. The area shaded in grey in the graph represents the upper and lower bounds of the 95% confidence intervals for the ratio scores. A Kolmogorov–Smirnov test showed that these ratio scores were normally distributed (Z = 0.86).
shows the log-transformed difference ratios of anticipatory fixations over the course of the experiment. The figure suggests that there may have been some task familiarisation effect over the first few trials and perhaps some small learning effect across the experiment. However, note that there is no reason that prediction should not be sensitive to contingencies over time in the environment. Correlations among the individual differences measures, and between the individual differences measures and the measure of predictive looks to the target, are given in . Pearson correlation coefficients (with their confidence intervals, based on Fisher's z transform) are reported, rather than Spearman correlation coefficients, because all measures were normally distributed.
Figure 4. Log-transformed difference ratios of anticipatory fixations over the course of the experiment.
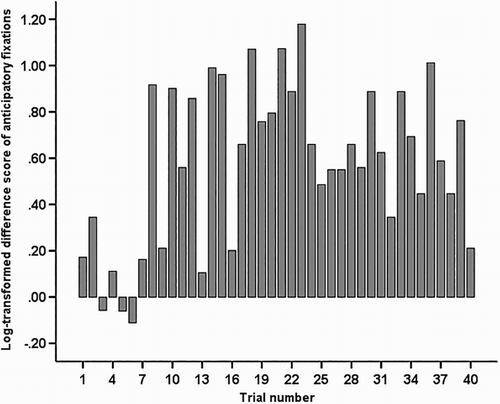
Table 1. Correlations between different measures (Pearson correlation coefficients are provided, plus their 95% confidence intervals in brackets).
We used principal component analysis to derive one Working Memory construct underlying the three memory measures (spatial short-term memory, auditory short-term memory, and working memory), and one Processing Speed construct underlying the two speed measures (DSS and letter matching). Factor loadings on the Working Memory construct (unrotated factor solution) were 0.71 for the visual working memory measure (i.e. Corsi block task), 0.81 for the digit span working memory measure, and 0.75 for the NWR working memory measure. Factor loadings on the Processing Speed construct (unrotated factor solution) were 0.90 for both speed measures. Individual scores on the extracted variables Working Memory and Processing Speed were saved as new predictors of prediction behaviour. presents the correlations between the new construct variables Working Memory and Processing Speed and predictive looks. shows that the Working Memory construct correlated positively and the Processing Speed construct negatively with anticipatory looks (note again that higher values on the Speed construct indicate slower processing).
Table 2. Correlations between construct scores (Working Memory and Processing Speed) and anticipatory looking behaviour (Pearson correlation coefficients are provided, plus their 95% confidence intervals in brackets).
Finally, we carried out multiple regression analyses to estimate the independent contribution of the variables and constructs to prediction performance in the eye-tracking task. The dependent variable was the log-transformed ratio of predictive looks in the critical time region (i.e. the difference of the log-transformed target and average distractor looks between article onset and noun onset). In the first multiple regression analysis, the Working Memory and Processing Speed constructs were entered into regression models in addition to age, hearing loss, and Raven's performance. All variables were entered at once. Given the missing data for several of these predictors for 27 participants in total (mainly for the hearing loss measure), this first analysis was run on a subsample of 78 participants. This model, with a R2 of 0.32, showed the following independent contributions to predictive eye gaze: Working Memory (unstandardised β = 0.23, SEβ = 0.08; standardised beta = 0.36), Processing Speed (unstandardised β = −0.28, SEβ = 0.09; standardised beta = −0.42), age (unstandardised β = 0.02, SEβ = 0.01; standardised beta = 0.35), Raven's (unstandardised β = 0.01, SEβ = 0.02; standardised beta = 0.12), and hearing loss (unstandardised β = 0.002, SEβ = 0.01; standardised beta = 0.02).
In a second regression analysis, we excluded hearing from the set of predictor variables to allow for inclusion of more participants from our sample. This second regression analysis, in which the Working Memory and Speed constructs were entered together with age and Raven's performance (all entered at once), was run on data from 102 participants. This model, with a R2 of 0.21, showed the following contributions to prediction: Working Memory (unstandardised β = 0.23, SEβ = 0.07, standardised beta = 0.36), Processing Speed (unstandardised β = −0.20, SEβ = 0.08, standardised beta = −0.30), age (unstandardised β = 0.01, SEβ = 0.01, standardised beta = 0.20), and Raven's (unstandardised β = −0.001, SEβ = 0.01, standardised beta = −0.01). Note the minimal amount of unique variance of the Raven's measure beyond what was already accounted for by working memory and speed (the correlation between Raven's and the Working Memory construct being r = .60, with 95% confidence interval from .46 to 71; and between Raven's and the Speed construct: r = −.58, with 95% confidence interval from −.70 to −.43).Footnote1
We also compared explained variance in the criterion variable between a model containing both Working Memory and Speed (with an R2 of 0.18, N = 102) to models containing either Working Memory or Speed only (with R2 values of 0.15 for a Working Memory only model and 0.11 for a Speed-only model, based on the same N). Thus, the portion of explained variance (change in R2) uniquely attributable to Working Memory was 0.07 (0.18 – 0.11, i.e. the difference between the WM+Speed model and the Speed-only model), and that of Speed was 0.03 (0.18 – 0.15, i.e. the difference between the WM+Speed model and the Working Memory only model). Scatter plots depicting the relation between the criterion variable (ratio of predictive looks) and the Working Memory and Speed constructs are provided in .
General discussion
Participants heard instructions such as “Kijk naar deCOM afgebeelde pianoCOM” (look at the displayed piano) while viewing four objects. The Dutch articles (“het” or “de”) were gender-marked such that the article agreed in gender only with the target, allowing for gender information from the article to be used to predict the upcoming target object. Participants fixated the target well before noun onset, which strongly suggests that they anticipated the target objects. Multiple regression analyses revealed that working memory and processing speed independently accounted for the largest amount of variance in participants’ language-mediated anticipatory eye movements in the present study. We will discuss these mediating factors in turn.
Our results are consistent with the notion that working memory serves as the nexus in which long-term visual as well as linguistic representations (i.e. types) are bound to specific locations (i.e. tokens or indices). As such, working memory capacity plays an important role in language-mediated anticipatory eye movements (Huettig, Olivers et al., Citation2011). Our data are compatible with the view of working memory as the capacity to hold and bind arbitrary pieces of information. How may such a working memory mediate anticipatory eye movements? In line with Huettig, Olivers et al. (Citation2011), we suggest that the objects in the visual display in the present experiment are first encoded in a visuospatial type of working memory (cf. Cavanagh & Alvarez, Citation2005; Pylyshyn, Citation1989). The perception of the familiar objects triggers perceptual hypotheses in long-term memory. Activation of these visual representations leads to cascaded activation at “higher” levels of representation (e.g. activation of semantic and phonological representations) within a few hundreds of milliseconds (see Huettig & McQueen, Citation2007; McQueen & Huettig, Citation2014 for experimental evidence). This results in a representational conglomerate of object knowledge and associated linguistic knowledge (including representations of the gender of the object names), which is bound to the objects’ locations in working memory. Hearing the article in the spoken instruction will then trigger a similar chain of events from the linguistic input, in which relevant representations (e.g. phonological, syntactic) will match up with those activated by the visual input. This activation is fed back to the object's location. This then increases the likelihood that a saccadic eye movement towards this location is triggered. We assume that the strength of activation of a particular representation translates into the probability of attending towards whatever shares those representations. In short, we suggest that language-mediated anticipatory eye movements require substantial working memory capacities to ground language in space and time, allowing for short-term connections among objects and linking linguistic and visual-spatial representations. According to this account, better working memory abilities result in more anticipatory eye gaze.
There is no doubt that many aspects of the exact nature of working memory require further exploration. According to some working memory models, for example, working memory contains or consists of activated long-term memory representations (e.g. Cowan, Citation2005; Ericsson & Kintsch, Citation1995; MacDonald & Christiansen, Citation2002). Working memory in these models therefore is the ability to activate long-term memory representations and keep them active for online processing. Working memory then indexes an individual's ability to activate and keep active multiple long-term memory representations in order to efficiently resolve competition processes. In other words, working memory either relates to the activation of a limited set of candidates, or to keep this set active in such a way that the competition among them can be resolved successfully. Another important characteristic of these long-term memory-based models (Cowan, Citation2005; Ericsson & Kintsch, Citation1995; MacDonald & Christiansen, Citation2002) is that working memory capacity is thought to be domain-specific and mediated by an individual's expertise or experience. According to these accounts, working memory capacity does not just reflect processing resources available for any task at hand but is specifically operationalised as memory for (for example) verbal material (as reflected in verbal working memory measures such as digit span and NWR) and spatial information (as measured by spatial working memory tests). Our data are consistent with the view that both verbal working memory and spatial working memory influence language-mediated anticipatory eye movements ().
We stress that the influence of working memory abilities on anticipatory spoken language processing may be particularly strong in the situational context tested in the present study. As discussed in the introduction, the particular task situation tested here requires the building of a representational network linking visual objects to unfolding linguistic information, places, times, and each other. To what extent does the experimental set-up in the current study then reflect core, context-invariant predictive language processing? We believe there is no such thing as context-invariant predictive language processing. We conjecture that situational context determines at least partly which mechanisms of prediction are engaged and the influence of particular mediating factors of prediction. Working memory abilities may be particularly important for anticipatory processing in situations when spoken language is used in relation to a co-present visual environment. Note, however, that this holds for many everyday situations when people give or receive instructions for action or talk about real-world events. Indeed, predictive language processing in our daily interactions is often very similar to choosing among a set of pre-activated referents. It is also conceivable that there are situations in which the influence of working memory is actually greater than in the present study. Knoeferle, Urbach, and Kutas (Citation2011), for instance, found that working memory contributed an R2 variance of 0.13 to a verb–action congruence reaction time effect (i.e. considerably more unique variance explained by working memory than in the present study). In short, the influence of working memory on anticipatory spoken language processing in other situations remains to be explored. Future work could usefully (especially) explore the influence of relevant visual environments on working memory effects.
We do believe, however, that the visual world experimental set-up is a particularly ecologically valid paradigm for the investigation of prediction when we talk about objects and events in our surroundings. We also believe that prediction and learning are often (though not always) closely linked (cf. Chang et al., Citation2006; Chang, Kidd, & Rowland, Citation2013; see Huettig & Mani, Citation2015, for further discussion). We maintain that even if participants in the present study had learned to some extent that the determiner provided a reliable cue for prediction, such an explanation would still enhance our understanding of prediction and its mediating factors (for instance that working memory mediates such predictive learning). The influence of working memory on anticipatory spoken language processing in other situations of course remains to be explored. Our point is simply that predictive language processing occurs in many different situations and that, in order to obtain a comprehensive understanding of prediction, we should look at multiple situations.
Our findings suggest that processing speed is a second cognitive ability mediating predictive processing. Our results indicate that speed of processing does not just relate to predictive processing through its association with working memory, but explains variance in language-mediated anticipatory eye movements beyond what is already explained by working memory. This suggests that individual differences in how quickly information is processed play an important role for predictive processing. It has been suggested that processing speed is related to the speed with which neural signals are conducted along axons. Speed of neural transmission has also been linked to the degree of myelination (Gutiérrez, Boison, Heinemann, & Stoffel, Citation1995). This could potentially provide a neural mechanism underlying processing speed though this proposal requires further investigation. We do point out, however, that the regression analysis suggests that non-verbal intelligence (as measured by performance in Raven's progressive matrices) did account for very little unique variance in anticipatory eye gaze in the present study. In other words, there is no evidence that a simple “g-factor” (a psychometric construct that performance of individuals at any one type of cognitive task predicts performance at other cognitive tasks) explains much variance in language-mediated anticipatory eye movements beyond what is already accounted for by working memory and speed.
The role of age in predictive processing was found to be very small, relative to working memory and speed, and only surfaced in the regression analysis. It is still noteworthy, however, that, if anything, advanced age seemed to relate to more/better predictive processing, which may appear to be surprising given previous findings that older adults show smaller and delayed effects of contextual constraint in anticipating upcoming words (Federmeier & Kutas, Citation2005; Huang et al., Citation2012; Janse & Jesse Citation2014). Several differences between these studies and the present study may account for this. First, our sample covered quite a large age range (participants were between 32 and 77 years of age, mean age being 56), but “only” 29 of our 105 participants were over the age of 60, and only 10 were over 70. Hence, investigating age effects continuously in this age range may yield different results than when (very young) students are compared to older adults (all aged 60+). Second, the present study investigated anticipation of nouns on the basis of article gender, rather than on the basis of semantic context effects. Whereas the latter effects often build up over the course of the sentence, the cue for prediction in our study was a relatively local, or adjacent, one. It seems unlikely, however, that this difference in the cue for anticipation accounts fully for the differential results, as detrimental influences of age were also found on rapid integration of information in adjective-noun units (Huang et al., Citation2012). This suggests that influences of age do not just occur when information needs to be integrated over longer time windows. Rather, the reason why increasing age in our sample, if anything, seemed to play a neutral or positive role in predictive processing may have been that we have been able to disentangle age effects from the confounds of age-related effects on memory and speed. shows how older age is associated with poorer memory and, particularly, with slower processing speed. It is only in the regression analysis, after having accounted for age effects on memory and speed, that age turns out to be (marginally) positively related to predictive processing. Thus, older adults may be more advanced language users, or at least not disadvantaged, compared to younger ones because of their lifelong experience (cf. Ramscar, Hendrix, Shaoul, Milin, & Baayen, Citation2014), even though this advantage may often be overshadowed by age-related cognitive decline. If age-related differences in processing speed and working memory as well as in sensory processing are accounted for, which is by no means trivial, age may play a neutral to positive role in predictive processing because of older adults’ increased lifelong experience. Future studies could further explore this possibility.
To conclude, we investigated whether individual differences in working memory and processing speed are important predictors of language-mediated anticipatory eye movements. We sought to find evidence for such a role using an individual differences approach. We observed that working memory abilities and processing speed explain most of the variance (of the mediating factors tested here) in language-mediated anticipatory eye movements. Enhanced working memory abilities support anticipatory spoken language processing whereas a decreased general processing speed has the opposite effect. These findings demonstrate that models of predictive language processing have to be revised to take mediating factors such as working memory and processing speed into account. More generally, our results are consistent with the notion that working memory enables us to link language to the here and now, or, when anticipating things, the there and then (Huettig, Olivers et al., Citation2011).
Acknowledgements
We thank Sophie Kirkels for assistance in running the study and Florian Hintz and Joost Rommers for discussion on how best to implement the new statistics approach (Cumming, Citation2014) for eye-tracking time-course graphs.
Disclosure statement
No potential conflict of interest was reported by the authors.
Notes
1. We performed standard multiple regression in which all predictor variables are entered simultaneously into the regression equation in order to estimate the unique contribution of each predictor variable. At reviewer request we also performed step-wise regression in which predictor variables are entered into the regression equation one at a time based on statistical thresholds. This did not change the pattern of results.
References
- Akeroyd, M. A. (2008). Are individual differences in speech reception related to individual differences in cognitive ability? A survey of twenty experimental studies with normal and hearing-impaired adults. International Journal of Audiology, 47( Suppl. 2), S53–S71.
- Altmann, G. T., & Kamide, Y. (1999). Incremental interpretation at verbs: Restricting the domain of subsequent reference. Cognition, 73(3), 247–264.
- Altmann, G., & Mirković, J. (2009). Incrementality and prediction in human sentence processing. Cognitive Science, 33(4), 583–609.
- Arai, M., & Keller, F. (2013). The use of verb-specific information for prediction in sentence processing. Language and Cognitive Processes, 28(4), 525–560.
- Arai, M., Van Gompel, R. P., & Scheepers, C. (2007). Priming ditransitive structures in comprehension. Cognitive Psychology, 54(3), 218–250.
- Arnett, J. J. (2008). The neglected 95%: Why American psychology needs to become less American. American Psychologist, 63(7), 602–614.
- Baddeley, A. D. (2006). Working memory: An overview. In S. Pickering (Ed.), Working memory and education (pp. 1–31). New York: Academic Press.
- Bates, T. C., Luciano, M., Montgomery, G. W., Wright, M. J., & Martin, N. G. (2011). Genetic variance in a component of the language acquisition device: ROBO1 polymorphisms associated with phonological buffer deficits. Behavior Genetics, 41, 50–57.
- Blom, E., Polišenská, D., & Weerman, F. (2008). Articles, adjectives and age of onset: The acquisition of Dutch grammatical gender. Second Language Research, 24(3), 297–331.
- Borovsky, A., Elman, J. L., & Fernald, A. (2012). Knowing a lot for one's age: Vocabulary skill and not age is associated with anticipatory incremental sentence interpretation in children and adults. Journal of Experimental Child Psychology, 112(4), 417–436.
- Botting, N., & Conti-Ramsden, G. (2001). Non-word repetition and language development in children with specific language impairment (SLI). International Journal of Language and Communication Disorders, 36(4), 421–432.
- Cavanagh, P., & Alvarez, G. A. (2005). Tracking multiple targets with multifocal attention. Trends in Cognitive Sciences, 9(7), 349–354.
- Chang, F., Dell, G. S., & Bock, K. (2006). Becoming syntactic. Psychological Review, 113(2), 234–272.
- Chang, F., Kidd, E., & Rowland, C. F. (2013). Prediction in processing is a by-product of language learning. Behavioral and Brain Sciences, 36(04), 350–351.
- Chen, E., Gibson, E., & Wolf, F. (2005). Online syntactic storage costs in sentence comprehension. Journal of Memory and Language, 52(1), 144–169.
- Corsi, P. M. (1972). Human memory and the medial temporal region of the brain. (Ph.D.). McGill University.
- Cowan, N. (2005). Working memory capacity. Hove: Psychology Press.
- Cumming, G. (2012). Understanding the new statistics. Effect sizes, confidence intervals, and meta-analysis. Routledge.
- Cumming, G. (2014). The new statistics: Why and how. Psychological Science, 25(1), 7–29.
- Dell, G. S., & Chang, F. (2014). The P-chain: Relating sentence production and its disorders to comprehension and acquisition. Philosophical Transactions of the Royal Society B: Biological Sciences, 369(1634), 20120394. Retrieved from http://dx.doi.org/10.1098/rstb.2012.0394
- DeLong, K. A., Urbach, T. P., & Kutas, M. (2005). Probabilistic word pre-activation during language comprehension inferred from electrical brain activity. Nature Neuroscience, 8(8), 1117–1121.
- Drake, E., & Corley, M. (2014). Effects in production of word pre-activation during listening: Are listener-generated predictions specified at a speech-sound level? Memory & Cognition. 43(1), 111–120.
- Earles, J. L., & Salthouse, T. A. (1995). Interrelations of age, health, and speed. The Journals of Gerontology Series B: Psychological Sciences and Social Sciences, 50B, P33–P41.
- Ericsson, K. A., & Kintsch, W. (1995). Long-term working memory. Psychological Review, 102, 211–245.
- Federmeier, K. D. (2007). Thinking ahead: The role and roots of prediction in language comprehension. Psychophysiology, 44(4), 491–505.
- Federmeier, K. D., & Kutas, M. (1999). A rose by any other name: Long-term memory structure and sentence processing. Journal of Memory and Language, 41(4), 469–495.
- Federmeier, K. D., & Kutas, M. (2005). Aging in context: Age-related changes in context use during language comprehension. Psychophysiology, 42(2), 133–141.
- Federmeier, K. D., Kutas, M., & Schul, R. (2010). Age-related and individual differences in the use of prediction during language comprehension. Brain and Language, 115(3), 149–161.
- Federmeier, K. D., McLennan, D. B., Ochoa, E., & Kutas, M. (2002). The impact of semantic memory organization and sentence context information on spoken language processing by younger and older adults: An ERP study. Psychophysiology, 39(2), 133–146.
- Fidler, F., & Loftus, G. R. (2009). Why figures with error bars should replace p values. Zeitschrift für Psychologie/Journal of Psychology, 217(1), 27–37.
- Gathercole, S. E. (2006). Nonword repetition and word learning: The nature of the relationship. Applied Psycholinguistics, 27, 513–543.
- Gathercole, S. E., & Baddeley, A. D. (1996). The children's test of nonword repetition. London: Psychological Corporation.
- Gathercole, S. E., Willis, C. S., Baddeley, A. D., & Emslie, H. (1994). The children's test of nonword repetition: A test of phonological working memory. Memory, 2, 103–127.
- Gutiérrez, R., Boison, D., Heinemann, U., & Stoffel, W. (1995). Decompaction of CNS myelin leads to a reduction of the conduction velocity of action potentials in optic nerve. Neuroscience Letters, 195(2), 93–96.
- Hale, J. (2001, June). A probabilistic early parser as a psycholinguistic model. In Proceedings of the Second Meeting of the North American Chapter of the Association for Computational Linguistics on Language Technologies (pp. 1–8). Association for Computational Linguistics.
- Henrich, J., Heine, S. J., & Norenzayan, A. (2010). The weirdest people in the world? Behavioral and Brain Sciences, 33(2–3), 61–83.
- Hoyer, W. J., Stawski, R. S., Wasylyshyn, C., & Verhaeghen, P. (2004). Adult age and digit symbol substitution performance: A meta-analysis. Psychology and Aging, 19, 211–214.
- Huang, H.-W., Meyer, A. M., & Federmeier, K. D. (2012). A “concrete view” of aging: Event related potentials reveal age-related changes in basic integrative processes in language. Neuropsychologia, 50, 26–35.
- Huettig, F. (in press). Four central questions about prediction in language processing. Brain Research. doi:10.1016/j.brainres.2015.02.014
- Huettig, F., & Altmann, G. (2005). Word meaning and the control of eye fixation: Semantic competitor effects and the visual world paradigm. Cognition, 96(1), B23–B32.
- Huettig, F., & Brouwer, S. (2015). Delayed anticipatory spoken language processing in adults with dyslexia – evidence from eye-tracking. Dyslexia, 21(2), 97–122.
- Huettig, F., & Mani, N. (2015). Is prediction necessary to understand language? Probably not. Language, Cognition and Neuroscience. Advance online publication. http://dx.doi.org/10.1080/23273798.2015.1072223
- Huettig, F., & McQueen, J. M. (2007). The tug of war between phonological, semantic and shape information in language-mediated visual search. Journal of Memory and Language, 57(4), 460–482.
- Huettig, F., Olivers, C. N., & Hartsuiker, R. J. (2011). Looking, language, and memory: Bridging research from the visual world and visual search paradigms. Acta Psychologica, 137(2), 138–150.
- Huettig, F., Rommers, J., & Meyer, A. S. (2011). Using the visual world paradigm to study language processing: A review and critical evaluation. Acta Psychologica, 137(2), 151–171.
- Hultsch, D. F., Hertzog, C., Small, B. J., McDonald-Miszczak, L., & Dixon, R. A. (1992). Short-term longitudinal change in cognitive performance in later life. Psychology and Aging, 7, 571–584.
- Janse, E., & Jesse, A. (2014). Working memory affects older adults’ use of context in spoken-word recognition. The Quarterly Journal of Experimental Psychology, 67(9), 1842–1862.
- de Jong, P. F., & van der Leij, A. (1999). Specific contributions of phonological abilities to early reading acquisition: Results from a Dutch latent variable longitudinal study. Journal of Educational Psychology and Aging, 91(3), 450–476.
- Kahneman, D., Treisman, A., & Gibbs, B. J. (1992). The reviewing of object files: Object-specific integration of information. Cognitive Psychology, 24(2), 175–219.
- Kail, R., & Salthouse, T. A. (1994). Processing speed as a mental capacity. Acta Psychologica, 86(2), 199–225.
- Kamide, Y., Altmann, G., & Haywood, S. L. (2003). The time-course of prediction in incremental sentence processing: Evidence from anticipatory eye movements. Journal of Memory and Language, 49(1), 133–156.
- Kamide, Y., Scheepers, C., & Altmann, G. T. (2003). Integration of syntactic and semantic information in predictive processing: Cross-linguistic evidence from German and English. Journal of Psycholinguistic Research, 32(1), 37–55.
- Kanwisher, N. G. (1987). Repetition blindness: Type recognition without token individuation. Cognition, 27(2), 117–143.
- Knoeferle, P., & Crocker, M. W. (2007). The influence of recent scene events on spoken comprehension: Evidence from eye movements. Journal of Memory and Language, 57(4), 519–543.
- Knoeferle, P., Crocker, M. W., Scheepers, C., & Pickering, M. J. (2005). The influence of the immediate visual context on incremental thematic role-assignment: Evidence from eye-movements in depicted events. Cognition, 95(1), 95–127.
- Knoeferle, P., Urbach, T. P., & Kutas, M. (2011). Comprehending how visual context influences incremental sentence processing: Insights from ERPs and picture-sentence verification. Psychophysiology, 48(4), 495–506.
- Kukona, A., Fang, S. Y., Aicher, K. A., Chen, H., & Magnuson, J. S. (2011). The time course of anticipatory constraint integration. Cognition, 119(1), 23–42.
- Kuperberg, G. R. (2007). Neural mechanisms of language comprehension: Challenges to syntax. Brain research, 1146, 23–49.
- Laszlo, S., & Federmeier, K. D. (2009). A beautiful day in the neighborhood: An event-related potential study of lexical relationships and prediction in context. Journal of Memory and Language, 61(3), 326–338.
- MacDonald, M. C., & Christiansen, M. H. (2002). Reassessing working memory: Comment on Just and Carpenter (1992) and Waters and Caplan (1996).. Psychological Review, 109, 35–54.
- Macmillan, N. A., & Creelman, C. D. (1991). Detection theory: A user's guide. Cambridge: Cambridge University Press.
- Mani, N., & Huettig, F. (2012). Prediction during language processing is a piece of cake – But only for skilled producers. Journal of Experimental Psychology: Human Perception and Performance, 38(4), 843–847.
- Mani, N., & Huettig, F. (2013). Towards a complete multiple-mechanism account of predictive language processing. Behavioral and Brain Sciences, 36(04), 365–366.
- Mani, N., & Huettig, F. (2014). Word reading skill predicts anticipation of upcoming spoken language input: A study of children developing proficiency in reading. Journal of Experimental Child Psychology, 126, 264–279.
- McDonald, S. A., & Shillcock, R. C. (2003). Eye movements reveal the on-line computation of lexical probabilities during reading. Psychological Science, 14(6), 648–652.
- McGettigan, C., Warren, J. E., Eisner, F., Marshall, C. R., Shanmugalingam, P., & Scott, S. K. (2011). Neural correlates of sublexical processing in phonological working memory. Journal of Cognitive Neuroscience, 23(4), 961–977.
- McQueen, J. M., & Huettig, F. (2014). Interference of spoken word recognition through phonological priming from visual objects and printed words. Attention, Perception, & Psychophysics, 76(1), 190–200.
- Metusalem, R., Kutas, M., Urbach, T. P., Hare, M., McRae, K., & Elman, J. L. (2012). Generalized event knowledge activation during online sentence comprehension. Journal of Memory and Language, 66(4), 545–567.
- Nation, K., Marshall, C. M., & Altmann, G. (2003). Investigating individual differences in children's real-time sentence comprehension using language-mediated eye movements. Journal of Experimental Child Psychology, 86(4), 314–329.
- Park, D. C., Lautenschlager, G., Hedden, T., Davidson, N. S., Smith, A. D., & Smith, P. K. (2002). Models of visuospatial and verbal memory across the adult life span. Psychology and Aging, 17(2), 299–320.
- Peelle, J. E., Troiani, V., Wingfield, A., & Grossman, M. (2010). Neural processing during older adults’ comprehension of spoken sentences: Age differences in resource allocation and connectivity. Cerebral Cortex, 20, 773–782.
- Pickering, M. J., & Garrod, S. (2007). Do people use language production to make predictions during comprehension? Trends in Cognitive Sciences, 11(3), 105–110.
- Pickering, M. J., & Garrod, S. (2013). An integrated theory of language production and comprehension. Behavioral and Brain Sciences, 36(4), 329–347.
- Pylyshyn, Z. (1989). The role of location indexes in spatial perception: A sketch of the FINST spatial-index model. Cognition, 32(1), 65–97.
- Pylyshyn, Z. W. (2001). Visual indexes, preconceptual objects, and situated vision. Cognition, 80(1), 127–158.
- Ramscar, M., Hendrix, P., Shaoul, C., Milin, P., & Baayen, H. (2014). The myth of cognitive decline: Non-linear dynamics of lifelong learning. Topics in Cognitive Science, 6(1), 5–42.
- Ramus, F., & Szenkovits, G. (2008). What phonological deficit? The Quarterly Journal of Experimental Psychology, 61(1), 129–141.
- Rayner, K., Reichle, E. D., Stroud, M. J., Williams, C. C., & Pollatsek, A. (2006). The effect of word frequency, word predictability, and font difficulty on the eye movements of young and older readers. Psychology and Aging, 21(3), 448–465.
- Rommers, J., Meyer, A. S., & Huettig, F. (2015). Verbal and nonverbal predictors of language-mediated anticipatory eye movements. Attention, Perception, & Psychophysics, 77(3), 720–730.
- Rommers, J., Meyer, A. S., Praamstra, P., & Huettig, F. (2013). The contents of predictions in sentence comprehension: Activation of the shape of objects before they are referred to. Neuropsychologia, 51(3), 437–447.
- Rönnberg, J., Lunner, T., Zekveld, A., Sörqvist, P., Danielsson, H., Lyxell, B., Dahlström, O., … Rudner, M. (2013). The ease of language understanding (ELU) model: Theoretical, empirical and clinical advances. Frontiers in Systems Neuroscience, 7, 31.
- Salthouse, T. A. (1996). The processing-speed theory of adult age differences in cognition. Psychological Review, 103, 403–428.
- Salthouse, T. A. (2000). Aging and measures of processing speed. Biological Psychology, 54, 35–54.
- Severens, E., Van Lommel, S., Ratinckx, E., & Hartsuiker, R. J. (2005). Timed picture naming norms for 590 pictures in Dutch. Acta Psychologica, 119, 159–187.
- Spivey, M. J., Richardson, D. C., & Fitneva, S. A. (2004). Thinking outside the brain: Spatial indices to visual and linguistic information. In J. Henderson & F. Ferreira (Eds.), The interface of language, vision, and action: Eye movements and the visual world, 161–189. Hove: Psychology Press
- Staub, A., & Clifton, C. Jr. (2006). Syntactic prediction in language comprehension: Evidence from either … or. Journal of Experimental Psychology: Learning, Memory, and Cognition, 32(2), 425–436.
- Thorn, A. S. C., & Gathercole, S. E. (1999). Language-specific knowledge and short-term memory in bilingual and non-bilingual children. The Quarterly Journal of Experimental Psychology Section A, 52(2), 303–324.
- Van Berkum, J. J., Brown, C. M., Zwitserlood, P., Kooijman, V., & Hagoort, P. (2005). Anticipating upcoming words in discourse: Evidence from ERPs and reading times. Journal of Experimental Psychology: Learning, Memory, and Cognition, 31(3), 443–467.
- Weber, A., Grice, M., & Crocker, M. W. (2006). The role of prosody in the interpretation of structural ambiguities: A study of anticipatory eye movements. Cognition, 99(2), B63–B72.
- Wechsler Adult Intelligence Test. (2004; 3rd ed., Dutch version). Amsterdam, The Netherlands: Harcourt Test.
- Wicha, N. Y., Moreno, E. M., & Kutas, M. (2003). Expecting gender: An event related brain potential study on the role of grammatical gender in comprehending a line drawing within a written sentence in Spanish. Cortex, 39(3), 483–508.
- Wicha, N. Y., Moreno, E. M., & Kutas, M. (2004). Anticipating words and their gender: An event-related brain potential study of semantic integration, gender expectancy, and gender agreement in Spanish sentence reading. Journal of Cognitive Neuroscience, 16(7), 1272–1288.
- Wlotko, E. W., & Federmeier, K. D. (2012). Age-related changes in the impact of contextual strength on multiple aspects of sentence comprehension. Psychophysiology, 49, 770–785.