ABSTRACT
We investigate the effects of two types of relationship between the words of a sentence or text – predictability and semantic similarity – by reanalysing electroencephalography (EEG) and functional magnetic resonance imaging (fMRI) data from studies in which participants comprehend naturalistic stimuli. Each content word's predictability given previous words is quantified by a probabilistic language model, and semantic similarity to previous words is quantified by a distributional semantics model. Brain activity time-locked to each word is regressed on the two model-derived measures. Results show that predictability and semantic similarity have near identical N400 effects but are dissociated in the fMRI data, with word predictability related to activity in, among others, the visual word-form area, and semantic similarity related to activity in areas associated with the semantic network. This indicates that both predictability and similarity play a role during natural language comprehension and modulate distinct cortical regions.
1. Introduction
Expectations about upcoming material are believed to play an important role during language comprehension (for recent reviews, see Huettig, Citation2015; Kuperberg & Jaeger, Citation2016). These expectations are usually considered to result from (probabilistic) predictions that are based on contextual information and knowledge of the language and the world. To rehash a famous example, Altmann and Kamide (Citation1999) had participants listen to sentences like “The boy will eat the – ” while viewing an image containing several objects, among which were included a boy and a cake but no other edible object. Immediate looks at the cake revealed prediction of the upcoming word “cake”. Such prediction requires knowledge of the language (e.g. the SVO structure of English) and the world (cakes are edible) as well as the use of information from the linguistic and non-linguistic context (the spoken sentence and visually presented objects, respectively). More specifically, prediction relies on knowledge of the language's syntagmatic structure. For example, the words “boy”, “eat”, and “cake” are syntagmatically related: They need to occur in this order because English has SVO structure and “eat” is a verb for which “boy” is an appropriate subject and “cake” an appropriate object. Hence, knowledge of syntagmatic structure allows prediction of “cake” after “the boy will eat”.
The current study investigates the additional role of a different type of language knowledge, that of paradigmatic structure. The words “boy”, “eat”, and “cake” are paradigmatically unrelated because they cannot take each other's place without radically changing the meaning of the sentence (“the cake will eat the boy”) or making it ungrammatical (“The eat will boy the cake”). However, the word “cake” is paradigmatically related to, for example, “pie” because “pie” can take the place of “cake” in most contexts. Whereas syntagmatic relations give rise to probabilistic prediction (“cake” is likely to occur after “the boy will eat”), paradigmatic structure captures semantic similarity (“cake” can be replaced by “pie” because of their shared semantic features). Hence, predictability and similarity are conceptually distinct relations involving different dimensions of linguistic structure that may have separate effects during comprehension. The objective of the current study is to reveal whether effects of predictability and similarity are also neurally distinct, which would show that the cognitive system indeed makes use of syntagmatic and paradigmatic structure during language comprehension.
1.1. Relation to earlier studies
Less predictable (i.e. lower cloze probability) words elicit a larger N400 amplitude in ERP studies (for review, see Kutas & Federmeier, Citation2011). In functional magnetic resonance imaging (fMRI) research, the general finding is that occurrence of a less predictable word leads to increased activations in (left) (middle) temporal regions and (left) inferior frontal cortex (Hagoort, Baggio, & Willems, Citation2009). We will not review this literature here but rather focus in some depth on research that, like the present study, compares effects of predictability and semantic relatedness.
Earlier ERP studies that attempt to dissociate predictive and semantic effects differ in several important respects from the current work. Lau, Holcomb, and Kuperberg (Citation2013) use a semantic decision task with prime-target word pairs, where predictability of a target's semantic class is varied by manipulating the proportion of related-prime fillers in an experimental block. They take prediction of a word or semantic feature to come down to commitment in working memory whereas semantic relatedness increases activation in long-term memory. We refrain from assumptions regarding the relevant memory structures but take predictability and semantic similarity to differ in the type of language knowledge involved (syntagmatic and paradigmatic, respectively). In contrast, Lau et al. (Citation2013) make clear that they assume predictive and semantic effects to rely on the same knowledge.
Brothers, Swaab, and Traxler (Citation2015) follow Lau et al. (Citation2013) in assuming that word prediction comes down to commitment. They aim to “dissociate the effects of specific lexical pre-activation, from other sources of contextual support (e.g. semantic association or discourse plausibility)” (p. 136). Hence, they conflate semantic factors and contextual plausibility, and contrast these to an all-or-nothing “lexical pre-activation”, which is operationalised by instructing participants to explicitly predict the final word of a short discourse and indicate whether their prediction matched the actual final word.
Unlike Brothers et al. (Citation2015) and Lau et al. (Citation2013), we do not assume that prediction involves any sort of commitment to a lexical item or semantic feature. Rather, if one word (or a set of words sharing a semantic feature) is much more strongly predicted than all others, this may be viewed as a near commitment to that word or feature but it is not qualitatively different from just strong prediction (see Kuperberg & Jaeger, Citation2016, for a discussion of this issue).
In two similar ERP studies, Metusalem et al. (Citation2012) and Paczynski and Kuperberg (Citation2012) had participants read short narratives where the critical word in the final sentence was either highly expected or semantically anomalous (i.e. had zero cloze probability), and semantically anomalous words were either related or unrelated to the event described in the text. The N400 effect of cloze was attenuated in the event-related condition,Footnote1 suggesting independent effects of predictability and semantic relatedness to the words of the earlier discourse. Likewise, Camblin, Gordon, and Swaab (Citation2007) looked at the ERP response to critical words while manipulating discourse congruency (where incongruency implies unpredictability) and semantic association with a previous word. Both manipulations affected the N400; a finding we will return to in the Discussion.
Our study's methodology differs from those of the five studies discussed above in two ways. First, we make use of electroencephalography (EEG)/fMRI recordings of participants' brain activity during normal reading/listening comprehension of naturally occurring sentences or texts, rather than items constructed for the sake of the experiment. Consequently, the materials do not contain any semantic anomalies. Second, the EEG or fMRI signal at each content word is compared to measures of the word's predictability and its semantic similarity to previous content words. The measures are derived from computational models that quantify the syntagmatic and paradigmatic relatedness between the words of the stimuli. In this manner, we are able to tease apart predictive and semantic effects (as operationalised by the computational models) without explicitly manipulating (cloze) probability or semantic similarity.
1.2. Models of syntagmatic and paradigmatic relations
In the field of Computational Linguistics, a language model is by definition any probability model that assigns probabilities to sentences or, equivalently, assigns a conditional probability distribution over the potentially upcoming words
given the sequence of words so far
.Footnote2 Word probability can easily be transformed to word surprisal, defined as
, which has been argued to form a cognitively relevant measure of processing load when encountering word
in sentence context (Hale, Citation2001; Levy, Citation2008). Indeed, it has repeatedly been shown that surprisal predicts word-reading time (Frank & Thompson, Citation2012; Monsalve, Frank, & Vigliocco, Citation2012; Smith & Levy, Citation2013). Surprisal effects have also been found in brain imaging data: Higher surprisal value results in a stronger N400 ERP component (Frank, Otten, Galli, & Vigliocco, Citation2015) as well as its magnetoencephalography (MEG) equivalent (Parviz, Johnson, Johnson, & Brock, Citation2011; Wehbe, Vaswani, Knight, & Mitchell, Citation2014), and stronger Blood-Oxygenation Level Dependent (BOLD) response in anterior temporal cortex, inferior frontal gyrus, and the visual word-form area (VWFA; Hale, Lutz, Luh, & Brennan, Citation2015; Willems, Frank, Nijhof, Hagoort & Van den Bosch, Citation2016).
In the current study, we will use word surprisal as a formalisation of the extent to which the word is (or, rather, can be) probabilistically predicted. In contrast, we make use of a distributional lexical semantics model to quantify semantic similarity between words. Many such models have been proposed, the best known being Latent Semantic Analysis (LSA; Landauer & Dumais, Citation1997). More recent approaches include Bayesian models (Griffiths, Steyvers, & Tenenbaum, Citation2007) and neural networks (Mikolov, Chen, Corrado, & Dean, Citation2013). In each case, co-occurrences patterns in a large text corpus give rise to high-dimensional vector representations of words, and the distance between two vectors quantifies the semantic distance between the two words. To the extent that a distributional semantics model assigns similar vectors to words that tend to occur in similar local contexts, the word relations it captures are of a paradigmatic nature (Rapp, Citation2002; Sahlgren, Citation2008).Footnote3
Distances between word vectors have been shown to be predictive of semantic priming effects in word naming (Jones, Kintsch, & Mewhort, Citation2006) and lexical decision (Günther Dudschig, & Kaup, Citation2016; Lund, Burgess, & Atchley, Citation1995) experiments. However, in the context of sentence or text comprehension, computationally quantified semantic distance has been studied much less than surprisal. Pynte, New, and Kennedy (Citation2008) found that larger LSA distance between the current and previous content word(s) results in longer word-reading time but this effect could have been caused by a confound with predictability (Frank, Citation2017). Indeed, Van den Hoven, Burke, and Willems (Citation2016) did not find a reading time effect of semantic distance over and above surprisal.
To the best of our knowledge, there have not been any neuroimaging studies that look at effects of semantic similarity, as quantified by computational models, during the comprehension of naturalistic stimuli. In contrast, several reading experiments that used subjective semantic relatedness measures found effects on the N400 (Camblin et al., Citation2007; Metusalem et al., Citation2012; Stafura & Perfetti, Citation2014; Van Petten, Citation1993). Other studies applied distributional semantics to quantify semantic relatedness in experimental stimuli. In an analysis of MEG data on sentence-final words occurring in high- and low-constraining context pairs, Parviz et al. (Citation2011) showed that N400 strength correlates positively with word surprisal as well as LSA-based semantic distance to the sentence's previous words. More recently, Ettinger, Feldman, Resnik, and Philips (Citation2016) applied a distributional semantics model (the same we use in the current study) to compute semantic distance between the critical word and its sentence context in the stimuli of a well-known ERP sentence-reading experiment (Federmeier & Kutas, Citation1999) and showed that these semantic distances accounted for the N400 effects from that study. Others have found neural correlates of the semantic vectors themselves (rather than distances between them), both in single-word comprehension (Mitchell et al., Citation2008) and in narrative reading (Wehbe et al., Citation2014).
As an alternative to capturing either probabilistic predictability or semantic similarity, a few models combine both in a single system. For example, Jones and Mewhort's (Citation2007) BEAGLE model constructs word vectors that capture not only semantic relations but also word-order information. The reversed approach (including paradigmatic structure in a probabilistic next-word prediction model) is more common. Indeed, semantic vector representations can be incorporated into a language model to improve its surprisal estimates. Mitchell and Lapata (Citation2009) developed a model that explicitly uses semantic distance values to adjust word-probability estimates and Mitchell, Lapata, Demberg, and Keller (Citation2010) show that this not only improves the language model but also provides surprisal values that more accurately predict reading times. Recurrent Neural Network (RNN) language models, trained to perform next-word prediction, will automatically develop vector representations of words in their input connection weights, thereby capturing the words' semantic similarity (Brakel & Frank, Citation2009; Mesnil, He, Deng, & Bengio, Citation2013; Mikolov et al., Citation2013). Surprisal values by RNNs are therefore based on both syntagmatic and paradigmatic relations.
The Mitchell et al. (Citation2010) and RNN language models assign higher surprisal to words with larger semantic distance to the preceding context words. That is, semantic similarity affects prediction but has no independent processing effects according to these models. If the cognitive system adapts its predictions in a similar manner, we would expect word surprisal (under a language model that does not incorporate paradigmatic structure) and semantic similarity to have identical effects on brain activity. Conversely, if these two measures have dissociable effects, this would support the view that syntagmatic and paradigmatic structure independently affect the comprehension process. A third possibility, of course, is that the model-derived semantic measures have no measurable effect on brain activity during language comprehension.
1.3. The current study
In what follows, we will briefly describe the previously published EEG and fMRI data sets in which surprisal and semantic distance effects will be identified (Section 2.1) after which we explain the two models that estimated these formal measures (Section 2.2). A large-scale regression analysis is then applied to identify unique effects of surprisal and semantic distance in the neuroimaging data, over and above a number of covariates. If syntagmatic and paradigmatic structure give rise to neurally distinct processes, we should find that the two model-based measures differ in the timing or distribution of their ERP effects or in the associated brain areas as measured with fMRI. The results (Section 3) show near identical effects on EEG, at least as far as the N400 is concerned, but a clear dissociation in the fMRI data. The latter result strongly suggests that probabilistic prediction and semantic similarity independently contribute to comprehension.
2. Method
2.1. Neuroimaging data
We reanalysed data from two publications on neuroimaging during language comprehension, one an EEG study (Frank et al., Citation2015) and the other applying fMRI (Willems et al., Citation2016). We only briefly discuss the stimuli materials and data collection here. Full details can be found in the original papers.
In the EEG study, 24 native speakers of English read 205 individual sentences that were sampled from English narratives, presented centrally one word at a time (RSVP method) with a word-length-dependent SOA of at least 627 ms. All sentences contained at least two content words (see Frank, Monsalve, Thompson, & Vigliocco, Citation2013, for the sentence selection constraints). Comprehension was tested by means of yes/no questions that appeared after approximately 50% of the sentences. EEG was recorded on 32 channels at 500 Hz (downsampled to 250 Hz and band-pass filtered between 0.5 and 25 Hz) and epoched into trials from to
relative to word onset. Trials with artefacts were identified visually and removed.
In the fMRI study, 24 native speakers of Dutch listened to three short excerpts from Dutch narrative audiobooks for a total of 19:27 minutes. The reversed audio files were presented as a baseline condition. There was no explicit task but participants were tested post-hoc for their memory and comprehension of the narratives. Images of BOLD changes were acquired on a 3T Siemens scanner with a T2*-weighted 3D EPI sequence (Poser, Koopmans, Witzel, Wald & Barth, Citation2010; TR: 880 ms, TE: 28 ms, flip angle: 14 degrees, voxel size: , 36 slices). Preprocessing involved motion correction (spatial realignment), spatial normalisation to MNI space, and spatial smoothing (8 mm FWHM), using SPM8 (http://www.fil.ion.ucl.ac.uk/spm).
2.2. Quantifying similarity and predictability
As explained in detail below, each content word from the English and Dutch stimuli was characterised by two measures: semantic distance and surprisal. Semantic distance quantifies the extent to which the current and previous content words tend to occur in different contexts, which requires knowledge of paradigmatic relations in the language. Surprisal quantifies the extent to which the word's occurrence is unexpected given the previous words and knowledge of syntagmatic relations in the language.
Both the semantic distance and surprisal model were trained on the first slice of Corpora from the Web (Schäfer, Citation2015); a large collection of individual sentences from web sources. The English ENCOW14 corpus comprises 28.9 million sentences with 644.5 million word tokens of 2.81 million types. The Dutch NLCOW14 corpus comprises 37.0 million sentences with 683.6 million word tokens of 4.95 million types. Words include punctuation, numbers, and other non-verbal symbols; and word-type count is case-insensitive. The much larger number of word types in Dutch compared to English is mostly due the fact that noun–noun compounds are written as single words in Dutch.
2.2.1. Semantic distance
Semantic vector representations of words were generated by Mikolov et al.'s (Citation2013) skipgram model.Footnote4 As illustrated in Figure , this is a two-layer feedforward neural network that receives as input individual word tokens from the training corpus and learns to produce as output the previous and upcoming five words (or less, respecting sentence boundaries) in the training sentence. Error is backpropagated through the network to update the connection weights, so that the weight vectors from two input nodes become more similar if the corresponding two words often occur in similar contexts, that is, if they are paradigmatically related. In this way, input weight vectors become semantic representations of words. Note that words that tend to co-occur (e.g. “eat the cake”) do not usually occur in similar contexts (i.e. “the” follows “eat” but precedes “cake”) so do not receive similar vector representations. Rather, their syntagmatic relatedness is captured by the surprisal model presented in Section 2.2.2.
Figure 1. Architecture of the Mikolov et al. (Citation2013) neural network model for obtaining word vector representations. The input layer and each of the 10 output blocks contain one unit for each word type in the training corpus. Each column of the input weight matrix is the 300-dimensional vector that represents the corresponding word.
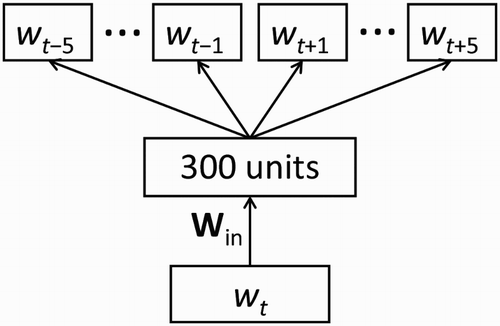
In distributional semantics models, the semantic relatedness between two words and
is commonly quantified using the cosine of the angle between the words' vector representations:
. The cosine value lies between
and
and is a measure of the two vectors' similarity. To get a measures of semantic distance we therefore take the negative cosine between the vectors.
A simple and commonly applied method for obtaining a semantic vector representation for a collection of words (e.g. a sentence or paragraph) is to sum the vectors of the individual words. Formally, we define as the collection of content words that precede the current word
up to a certain distance. The vector representation of A is the sum of its word vectors:
. The semantic distance between word
and the previous content words in A then equals
.
The question remains which words are selected to take part in the vector sum when computing . For the individual sentences from the EEG study, A comprises all content words preceding
in the sentence. For the narrative texts of the fMRI study, A comprises the preceding four content words (or fewer, for words at the very beginning of a text). If
is the first content word of the sentence (EEG study) or text (fMRI study) then A is empty so semantic distance is undefined and the word is discarded from analysis.
2.2.2. Surprisal
Markov models, more commonly known as n-gram models, are among the simplest yet most successful language models for generating word-surprisal values. These models are ‘myopic’ in that a word's probability estimate depends only on the previous n−1 words: The full conditional probability is simplified to
, which is estimated directly from the training corpus frequencies of n-word (and shorter) sequences. The value of n needs to be small (n=3 being the most common) because corpus frequencies quickly drop to zero for larger n. Consequently, n-gram based surprisal values are not ideal for comparison to our semantic distance measures. This is because semantic distance can depend on paradigmatic relations with words beyond the n-word window, resulting in a confound between the measure (semantic distance versus surprisal) and the previous context words taken into account to compute it.
We therefore opted to combine the standard n-gram model with what we call a “skip-bigram” language model that estimates the probability of from occurrence frequencies of word pairs
, where
occurs before
but they may be separated by other words. Specifically, let
contain exactly the content words preceding
that were relevant for computing semantic distance (see Section 2.2.1). Under the simplifying assumption that each
provides an independent cue to the occurrence probability of
, we can define the skip-bigram language model:
The unigram and skip-bigram probabilities,
and
, are estimated by their relative frequencies in the corpus. The surprisal of
is then computed by linear interpolation of the n-gram and skip-bigram language models:
(2) where
is the probability under the n-gram (Markov) model. The Markov model order n and the weighting parameter
are set empirically to minimise the average surprisal over each of the two sets of experimental stimuli (see Supplementary Materials for details). Average surprisal was lowest for n=5, and
for the English sentences and
for the Dutch audiobook texts. The fact that the ideal λs are smaller than 1 shows that interpolating the n-gram with the skip-bigram probabilities indeed results in a more accurate language model.
2.2.3. Comparing semantic distance and surprisal
As an illustration of how the two model-derived measures quantify syntagmatic and paradigmatic relations between words, Table displays two sentence fragments from the English materials, where the final word has high semantic distance but low surprisal, or vice versa. In the first example, “wall” is predictable from the context “Alec stood against the – ” but none of the context words are semantically similar. In the second example, the fragment-final word “Ellen” is highly unexpected given the context but it has a strong paradigmatic relation to the earlier word “Frank”.
Table 1. Sentence fragment examples with semantic distance and surprisal values (expressed as z-scores) of the final word.
Over all, surprisal and semantic distance measures are only weakly correlated: r = .27 and r = .05 for the English and Dutch stimuli, respectively. Crucially, as demonstrated in the Supplementary Materials, further interpolation of surprisal with word probabilities derived from the semantic vector cosines is detrimental to the language model, which means that the vector cosines do not encode any information that is useful for next-word prediction.
3. Results
Both the EEG and fMRI data sets were analysed by regressing brain activity measures (electrode potential or BOLD response) on surprisal and semantic distance, but analysis details differed because of differences between the stimuli presentation and neuroimaging methods. Note that the surprisal and distance measures are included in the regression analysis together, so any effect of one of the measures will be over and above what is already explained by the other.
3.1. EEG
As discussed in the Introduction, earlier EEG sentence comprehension studies looking at effects of semantic relatedness between words found effects on the N400. For this reason, we look only at the seven most central electrodes, where Frank et al. (Citation2015) already found an N400 effect of surprisal in this EEG data set. The objectives here are to ascertain if semantic distance, too, affects the N400 and, if so, to compare its timing, distribution, and effect size to that of the surprisal-elicited N400 wave.
The analysis roughly followed the rERP method recently proposed by Smith and Kutas (Citation2015) where the set of electrode potentials at each sample point, collected over word tokens and subjects, is regressed on the relevant predictor. The statistics of interest are then the regression coefficients of surprisal and semantic distance. Compared to the traditional ERP averaging method, rERP analysis makes better use of the available data and can more easily include any number of continuous covariates. Following Frank et al. (Citation2015), the covariates included here were: position of sentence in the experiment session, position of word in the sentence, word length, word frequency (in the training corpus, log transformed), and EEG baseline (average electrode potential in the 100 ms leading up to word onset). All independent variables were standardised. The linear mixed-effects regression model included by-subject and by-word random intercepts and by-subject random slopes of surprisal and semantic distance.
Figure displays the time course of the coefficients b from the regression analysis (expressed in μV per standard deviation increase in the predictor) for the effects of surprisal and semantic distance on electrode potential, time-locked to word onset. The two effects have nearly identical onset and offset, and even the effect sizes are very similar, that is, a unit increase in surprisal leads to the same change in electrode potential as a unit increase in semantic distance, when both measures are expressed in standard deviations of the distribution over the stimuli materials.
3.2. fMRI
The analysis of fMRI was identical to that in Willems et al. (Citation2016) except that semantic distance was included as a predictor in addition to word surprisal and frequency (in the training corpus, log transformed) and that function words, for which no semantic distance values are computed, were modelled as events of no interest. In contrast to the EEG study above, we had no clear prior expectations about where any effect of semantic distance would appear. Hence, a whole-brain analysis was performed to identify areas where (after multiple-comparison correction) higher surprisal or semantic distance resulted in more activity compared to the reversed-speech baseline. Single-subject statistical maps were estimated using the General Linear Model as implemented in SPM8, and subsequent group analysis involved testing every voxel's significance over participants against zero (“Random-effects analysis”). Single-subject maps for the semantic distance and surprisal regressors tested for positive relationships with neural activity, and compared whether these positive relationships were stronger during listening to the stories than the reversed-speech baseline. We combined a voxel-level statistical threshold with a cluster size threshold to realise family-wise error correction at the p < .05 level. For this, we combined a voxel-level threshold of p < .005 with a 54-voxel cluster size threshold. The correction was based on a large number (10,000) of simulations estimating the critical number of voxels per region to arrive by chance (Slotnick, Moo, Segal, & Hart Jr., Citation2003). The smoothing kernel used in the simulations was based on the functional imaging data, as suggested in Bennett, Wolford, and Miller (Citation2009).
We found several areas to become activated with increasing semantic distance. These were the left anterior temporal pole stretching into the anterior middle temporal sulcus, the precuneus, and the angular gyri bilaterally (see Figure and Table ). For surprisal, the relevant areas corresponded largely to what was found before by Willems et al. (Citation2016). In particular, the left inferior temporal sulcus/posterior fusiform gyrus (VWFA), bilateral posterior superior temporal gyrus, and the bilateral amygdala were found to be sensitive to word surprisal (see Figure and Table ).
Figure 3. Brain areas that become significantly more active (p<.05 corrected for multiple comparisons) in response to larger surprisal (blue) or semantic distance (red).
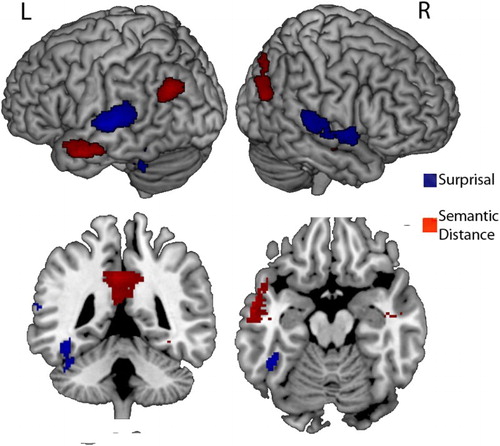
Table 2. Brain areas that become significantly more active in response to larger semantic distance.
Table 3. Brain areas that become significantly more active in response to word surprisal.
From the image in Figure , distinct areas appear to be activated by surprisal and semantic distance. However, the plotted activation map is thresholded: Activation that does not reach significance is not displayed. When an area is significantly activated by one measure but not by the other, this does not imply that the difference in activation due to the measures is significant because the two measures can be very close on either side of the threshold. However, when the threshold is decreased to p<.01 without multiple-comparison correction, the areas activated by the two measures remain distinct (Figure ).
4. Discussion
We investigated how two types of relations between words affect neural activation during language comprehension: Syntagmatic relations that can drive probabilistic prediction of the upcoming word, and paradigmatic relations that reflect lexical-semantic feature overlap. These two relationship types potentially give rise to cognitive processes that are conceptually very different, as becomes clear when we think of these processes in terms of their usefulness. A predictive language-processing system is accurate to the extent that it assigns high next-word probability to the actual upcoming words, thereby increasing processing efficiency (Smith and Levy, Citation2008, Citation2013). Inaccurate predictions are useful too, when the mismatch between what is predicted and what actually occurs forms an error signal to improve future predictions, that is, to increase the quality of the cognitive language model. In contrast, there is no such strategic value in the effect of semantic feature overlap: Observing the word “cake” may affect future processing of “pie” but unless the occurrence probability of “pie” has actually increased (i.e. there is a syntagmatic relation that makes “pie” predictable from “cake”) there is no sense in which the effect of “cake” on “pie” can be (in)accurate so no processing efficiency or language knowledge is to be gained.
The fact that we can conceive of distinct cognitive processes corresponding to predictability and semantic similarity does not imply that both play a role during language comprehension. Although effects of word predictability, as formalised by surprisal, are robust and well established, semantic relatedness effects on sentence comprehension appear to be more fickle Camblin et al. (Citation2007).
We collected surprisal and semantic distance measures on all content words from natural sentences and texts, computed by models trained on a large text corpus. The language model does not include any notion of lexical semantics so the surprisal values it estimates do not capture semantic properties, except insofar as these have shaped the language's syntagmatic structure and are therefore informative for prediction. Conversely, the cosine distances between word vectors from the distributional semantics model do not encode word order or any other syntagmatic relation between words. If they nevertheless contain any information that can help predict the upcoming word, this is already captured in the surprisal values: As demonstrated in the Supplementary Materials, integrating the cosine distances into the surprisal measure yields worse next-word predictions.
A comparison between the surprisal and distance measures to brain activation during language comprehension revealed first of all that each measure has significant effects over and above the other. Under the common assumption that a weaker BOLD signal or N400 is indicative of reduced processing effort, we found that words that are more predictable or semantically more similar to earlier words are easier to access or process. This was the case in both data sets, despite their differences in language (English versus Dutch), brain imaging method (EEG versus fMRI), stimuli type (individual sentences versus narratives), and presentation modality (written versus spoken stimuli).
Higher surprisal resulted in increased activity in bilateral posterior superior temporal areas, as well as the putative VWFA, overlapping with what was found by Willems et al. (Citation2016) on the same data set but using a different language model. They took this surprisal effect to suggest that probabilistic next-word prediction goes all the way down to pre-activating word form. It has been reported that lexical-semantic variables, too, affect activation levels in the VWFA (Levy et al., Citation2009; Vinckier et al., Citation2007) which offers an alternative, lexical-semantic interpretation of the surprisal effect. However, if the VWFA result is indeed lexical-semantic rather than form-based, we would (also) expect an effect of semantic relatedness on VWFA activation, but no such effect was found.
Words with larger semantic distance to previous words resulted in higher activation in, among others, the left temporal pole, angular gyrus, and precuneus. In a meta-analysis by Binder, Desai, Graves, and Conant (Citation2009) these areas were identified as parts of the lexical-semantic system, which is consistent with our claim that the word vector representations quantify relevant lexical-semantic properties (see Binder et al. for the extensive literature implicating these areas in semantic comprehension). Wehbe et al. (Citation2014) recently found angular gyrus activity to correlate with semantic features from a distributional semantics model, again demonstrating the importance of this region to lexical semantics. Crucially, brain areas related to surprisal and to semantic distance did not overlap, not even within the left temporal cortex, so even if we refrain from functional interpretation we can conclude that syntagmatic and paradigmatic knowledge have neurally (and, therefore, most likely also cognitively) distinct effects during language comprehension.
Turning now to the EEG results, we see that surprisal and semantic distance have identically timed ERP effects, at least to the extent they relate to the N400. It is quite remarkable that the two measures have the same effect size, in the sense that one standard deviation increase in either measure resulted in the same amount of increase in N400 size, considering that predictability effects are much more robust than semantic relatedness effects, at least in reading times (Camblin et al., Citation2007; Frank, Citation2017; Van den Hoven et al., Citation2016).
Camblin et al. (Citation2007) found that N400 effects of discourse congruency (which correlates with predictability) precede those of priming. We did not replicate this but their study used short pieces of cohesive discourse which may have resulted in stronger predictions than our individual sentences. A time-separation between congruency and priming effects is of course fully consistent with our claim that surprisal and semantic distance are neurally distinguishable.
In addition to the N400 effects, Figure provides some evidence for an early frontal positivity, possibly a P2 component, which is sensitive to surprisal first and to semantic distance later. Keeping in mind that we cannot make strong claims about the reliability of this effect as we did not plan to look at other components than N400, it does match Camblin et al.'s (Citation2007) finding of priming effects arising later than congruency effects.
5. Conclusion
We showed that surprisal and semantic distance can have neurally distinguishable effects during language comprehension. Although these effects were highly similar in the N400 response, the fMRI results showed separate neural correlates for the two measures. This calls for current models of sentence comprehension to explicitly take into account separate mechanisms giving rise to effects of predictability and semantic similarity.
In neuroimaging studies of language, there is a recent trend towards the use of naturalistic stimuli as opposed to hand-crafted experimental items (e.g. Wehbe et al., Citation2014; Willems, Citation2015). Making use of natural variation in language, rather than imposing extremes such as semantic anomalies or syntactic violations, increases generalisability of the results and reduces the risk of artefacts, for example caused by participants adjusting their processing strategies to the nature of the stimuli. In addition, it has the advantage that very rich data sets can be collected, which allow for many different analyses. As a case in point, we opted for re-analysing published fMRI and EEG data as a first test of the relation between brain activity and computational measures of semantic relatedness. Although the individual data sets suited our needs and converging results strengthen the evidence that both surprisal and semantic distance affect processing, it can be considered a drawback that different stimuli sets and presentation modalities were used for the fMRI and EEG studies. For example, we cannot be sure that identical timing of surprisal and semantic distance effects holds up in spoken narratives as opposed to written individual sentences. Future work using EEG or MEG with spoken narrative stimuli may provide the answer to this question.
The use of naturalistic stimuli combines well with parametric designs where computational characterisation of materials is compared to brain activity, because the models can quantify every word of the stimuli. This method has previously relied on probabilistic language models (Frank et al., Citation2015 Hale et al., Citation2015; Wehbe et al., Citation2014; Willems et al., Citation2016) and the current work is the first that also applies it to study the effect of semantic similarity using a computational measure that dissociates similarity from surprisal. Hence, our results further highlight the value of using computational models for predicting brain activity during comprehension of naturalistic stimuli.
Supplementary_Material_1323109.pdf
Download PDF (192.6 KB)Disclosure statement
No potential conflict of interest was reported by the authors.
ORCID
Stefan L. Frank http://orcid.org/0000-0002-7026-711X
Additional information
Funding
Notes
1. In Paczynski and Kuperberg (Citation2012), this attenuation did not occur if the semantically anomalous word violated its verb's animacy selection restrictions. The extreme nature of such a violation makes this condition less relevant to the current study.
2. The probability of could also depend on the non-linguistic context, such as the current visual scene, but few language models take non-linguistic information into account.
3. But see Lapesa, Evert, and Schulte im Walde (Citation2014) for results suggesting that, to a lesser extent, such models may also be sensitive to syntagmatic relations.
4. Model settings were: initial learning rate 0.025; five negative samples; down-sampling of words with frequency above ; five iterations through the training data.
References
- Altmann, G. T. M., & Kamide, Y. (1999). Incremental interpretation at verbs: Restricting the domain of subsequent reference. Cognition, 73, 247–264. doi: 10.1016/S0010-0277(99)00059-1
- Bennett, C. M., Wolford, G. L., & Miller, M. B. (2009). The principled control of false positives in neuroimaging. Social Cognitive and Affective Neuroscience, 4, 417–422. doi: 10.1093/scan/nsp053
- Binder, J. R., Desai, R. H., Graves, W. W., & Conant, L. L. (2009). Where is the semantic system? A critical review and meta-analysis of 120 functional neuroimaging studies. Cerebral Cortex, 19, 2767–2796. doi: 10.1093/cercor/bhp055
- Brakel, P., & Frank, S. L. (2009). Strong systematicity on sentence processing by simple recurrent networks. In N. A. Taatgen and H. van Rijn (Eds.), Proceedings of the 31st annual conference of the Cognitive Science Society (pp. 1599–1604). Austin, TX: Cognitive Science Society.
- Brothers, T., Swaab, T. Y., & Traxler, M. J. (2015). Effects of predictions and contextual support on lexical processing: prediction takes precedence. Cognition, 136, 135–149. doi: 10.1016/j.cognition.2014.10.017
- Camblin, C. C., Gordon, P. C., & Swaab, T. Y. (2007). The interplay of discourse congruence and lexical association during sentence processing: Evidence from ERPs and eye tracking. Journal of Memory and Language, 56, 103–128. doi: 10.1016/j.jml.2006.07.005
- Ettinger, A., Feldman, N. H., Resnik, P., & Philips, C. (2016). Modeling N400 amplitude using vector space models of word representation. In A. Papafragou, D. Grodner, D. Mirman, and J. Trueswell (Eds.), Proceedings of the 38th annual conference of the Cognitive Science Society (pp. 1445–1450). Austin, TX: Cognitive Science Society.
- Federmeier, K. D., & Kutas, M. (1999). A rose by any other name: Long-term memory structure and sentence processing. Journal of Memory and Language, 41, 469–495. doi: 10.1006/jmla.1999.2660
- Frank, S. L. (2017). Word embedding distance does not predict word reading time. Proceedings of the 39th annual conference of the Cognitive Science Society. Austin, TX: Cognitive Science Society.
- Frank, S. L., Monsalve, I. F., Thompson, R. L., & Vigliocco, G. (2013). Reading time data for evaluating broad-coverage models of English sentence processing. Behavior Research Methods, 45, 1182–1190. doi: 10.3758/s13428-012-0313-y
- Frank, S. L., Otten, L. J., Galli, G., & Vigliocco, G. (2015). The ERP response to the amount of information conveyed by words in sentences. Brain and Language, 140, 1–11. doi: 10.1016/j.bandl.2014.10.006
- Frank, S. L., & Thompson, R. L. (2012). Early effects of word surprisal on pupil size during reading. In Proceedings of the 34th annual conference of the Cognitive Science Society (pp. 1554–1559). Austin, TX: Cognitive Science Society.
- Griffiths, T. L., Steyvers, M., & Tenenbaum, J. B. (2007). Topics in semantic representation. Psychological Review, 114, 211–244. doi: 10.1037/0033-295X.114.2.211
- Günther, F., Dudschig, C., & Kaup, B. (2016). Latent semantic analysis cosines as a cognitive similarity measure: Evidence from priming studies. The Quarterly Journal of Experimental Psychology, 69, 626–653. doi: 10.1080/17470218.2015.1038280
- Hagoort, P., Baggio, G., & Willems, R. M. (2009). Semantic unification. In M. S. Gazzaniga (Ed.), The Cognitive neurosciences IV. Cambridge, MA: MIT press.
- Hale, J. T. (2001). A probabilistic Early parser as a psycholinguistic model. In Proceedings of the second conference of the North American chapter of the Association for Computational Linguistics (Vol. 2, pp. 159–166). Pittsburgh, PA: Association for Computational Linguistics.
- Hale, J. T., Lutz, D., Luh, W., & Brennan, J. (2015). Modeling fMRI time courses with linguistic structure at various grain sizes. In Proceedings of the 6th workshop on cognitive modeling and computational linguistics (pp. 89–97). Denver, CO: Association for Computational Linguistics.
- Huettig, F. (2015). Four central questions about prediction in language processing. Brain Research, 1626, 118–135. doi: 10.1016/j.brainres.2015.02.014
- Jones, M. N., Kintsch, W., & Mewhort, D. J. K. (2006). High-dimensional semantic space accounts of priming. Journal of Memory and Language, 55, 534–552. doi: 10.1016/j.jml.2006.07.003
- Jones, M. N., & Mewhort, D. J. K. (2007). Representing word meaning and order information in a composite holographic lexicon. Psychological Review, 114, 1–37. doi: 10.1037/0033-295X.114.1.1
- Kuperberg, G. R., & Jaeger, T. F. (2016). What do we mean by prediction in language comprehension?. Language, Cognition and Neuroscience, 31, 32–59. doi: 10.1080/23273798.2015.1102299
- Kutas, M., & Federmeier, K. D. (2011). Thirty years and counting: Finding meaning in the N400 component of the event-related brain potential (ERP). Annual Review of Psychology, 62, 621–647. doi: 10.1146/annurev.psych.093008.131123
- Landauer, T. K., & Dumais, S. T. (1997). A solution to Plato's problem: The latent semantic analysis theory of acquisition, induction, and representation of knowledge. Psychological Review, 104, 211–240. doi: 10.1037/0033-295X.104.2.211
- Lapesa, G., Evert, S., & Schulte im Walde, S. (2014). Contrasting syntagmatic and paradigmatic relations: Insights from distributional semantic models. In Proceedings of the third joint conference on lexical and computational semantics (pp. 160–170). Dublin, Ireland.
- Lau, E. F., Holcomb, P. J., & Kuperberg, G. R. (2013). Dissociating N400 effects of prediction from association in single-word contexts. Journal of Cognitive Neuroscience, 25, 484–502. doi: 10.1162/jocn_a_00328
- Levy, R. (2008). Expectation-based syntactic comprehension. Cognition, 106, 1126–1177. doi: 10.1016/j.cognition.2007.05.006
- Levy, J., Pernet, C., Treserras, S., Boulanouar, K., Aubry, F., Démonet, J.-F., Celsis, P., & García, A. V. (2009). Testing for the dual-route cascade reading model in the brain: an fMRI effective connectivity account of an efficient reading style. PloS one, 4, e6675. doi: 10.1371/journal.pone.0006675
- Lund, K., Burgess, C., & Atchley, R. A. (1995). Semantic and associative priming in high-dimensional semantic space. In J. D. Moore and J. F. Lehman (Eds.), Proceedings of the 17th annual conference of the Cognitive Science Society (pp. 660–665). Mahwah, NJ: Erlbaum.
- Mesnil, G., He, X., Deng, L., & Bengio, Y. (2013). Investigation of recurrent-neural-network architectures and learning methods for spoken language understanding. In Interspeech-2013, pp. 3771-3775. Retrieved from http://www.isca-speech.org/archive/interspeech_2013/i13_3771.html.
- Metusalem, R., Kutas, M., Urbach, T. P., Hare, M., McRae, K., & Elman, J. L. (2012). Generalized event knowledge activation during online sentence comprehension. Journal of Memory and Language, 66, 545–567. doi: 10.1016/j.jml.2012.01.001
- Mikolov, T., Chen, K., Corrado, G., & Dean, J. (2013). Efficient estimation of word representations in vector space. Proceedings of the ICLR workshop.
- Mitchell, J., & Lapata, M. (2009). Language models based on semantic composition. In Proceedings of the 2009 conference on empirical methods in natural language processing (pp. 430–439). Singapore: Association for Computational Linguistics.
- Mitchell, J., Lapata, M., Demberg, V., & Keller, F. (2010). Syntactic and semantic factors in processing difficulty: An integrated measure. In Proceedings of the 48th annual meeting of the Association for Computational Linguistics (pp. 196–206). Uppsala: Association for Computational Linguistics.
- Mitchell, T., Shinkareva, S. V., Carlson, A., Chang, K., Malave, V. L., Mason, R. A., & Just, M. A. (2008). Predicting human brain activity associated with the meanings of nouns. Science, 320, 1191–1195. doi: 10.1126/science.1152876
- Monsalve, I. F., Frank, S. L., & Vigliocco, G. (2012). Lexical surprisal as a general predictor of reading time. In Proceedings of the 13th conference of the European Chapter of the Association for Computational Linguistics (pp. 398–408). Avignon: Association for Computational Linguistics.
- Paczynski, M., & Kuperberg, G. R. (2012). Multiple influences of semantic memory on sentence processing: Distinct effects of semantic relatedness on violations of real-world event/state knowledge and animacy selection restrictions. Journal of Memory and Language, 67, 426–448. doi: 10.1016/j.jml.2012.07.003
- Parviz, M., Johnson, M., Johnson, B., & Brock, J. (2011). Using language models and Latent Semantic Analysis to characterise the N400m neural response. In Proceedings of the Australasian Language Technology Association Workshop 2011 (pp. 38–46). Canberra, Australia.
- Poser, B. A., Koopmans, P. J., Witzel, T., Wald, L. L., & Barth, M. (2010). Three dimensional echo-planar imaging at 7 Tesla. NeuroImage, 51, 261–266. doi: 10.1016/j.neuroimage.2010.01.108
- Pynte, J., New, B., & Kennedy, A. (2008). On-line contextual influences during reading normal text: A multiple-regression analysis. Vision Research, 48, 2172–2183. doi: 10.1016/j.visres.2008.02.004
- Rapp, R. (2002). The computation of word associations: Comparing syntagmatic and paradigmatic approaches. In Proceedings of the 19th international conference on computational linguistics (vol. 1, pp. 1–7). Stroudsburg, PA: Association for Computational Linguistics.
- Sahlgren, M. (2008). The distributional hypothesis. Rivista di Linguistica, 20, 33–53.
- Schäfer, R. (2015). Processing and querying large web corpora with the COW14 architecture. In P. Bański, H. Biber, E. Breiteneder, M. Kupietz, H. Lüngen, and A. Witt (Eds.), Proceedings of the 3rd workshop on the challenges in the management of large corpora (pp. 28–34).
- Slotnick, S. D., Moo, L. R., Segal, J. B., & Hart Jr., J. (2003). Distinct prefrontal cortex activity associated with item memory and source memory for visual shapes. Cognitive Brain Research, 17, 75–82. doi: 10.1016/S0926-6410(03)00082-X
- Smith, N. J., & Kutas, M. (2015). Regression-based estimation of ERP waveforms: I. The rERP framework. Psychophysiology, 52, 157–168. doi: 10.1111/psyp.12317
- Smith, N. J., & Levy, R. (2008). Optimal processing times in reading: A formal model and empirical investigation. In B. C. Love, K. McRae, and V. M. Sloutsky (Eds.), Proceedings of the 30th annual meeting of the Cognitive Science Society (pp. 595–600). Austin, TX: Cognitive Science Society.
- Smith, N. J., & Levy, R. (2013). The effect of word predictability on reading time is logarithmic. Cognition, 128, 302–319. doi: 10.1016/j.cognition.2013.02.013
- Stafura, J. Z., & Perfetti, C. A. (2014). Word-to-text integration: message level and lexical level influences in ERPs. Neuropsychologia, 64, 41–53. doi: 10.1016/j.neuropsychologia.2014.09.012
- Van den Hoven, E., Hartung, F., Burke, M., & Willems, R. M. (2016). Individual differences in sensitivity to style during literary reading: Insights from eye-tracking. Collabra, 2, 25, 1–16. doi: 10.1525/collabra.39
- Van Petten, C. (1993). A comparison of lexical and sentence-level context effects in event-related potentials. Language and Cognitive Processes, 8, 485–531. doi: 10.1080/01690969308407586
- Vinckier, F., Dehaene, S., Jobert, A., Dubus, J. P., Sigman, M., & Cohen, L. (2007). Hierarchical coding of letter strings in the ventral stream: Dissecting the inner organization of the visual wordform system. Neuron, 55, 143–156. doi: 10.1016/j.neuron.2007.05.031
- Wehbe, L., Murphy, B., Talukdar, P., Fyshe, A., Ramdas, A., Mitchell, T., & Paterson, K. (2014). Simultaneously uncovering the patterns of brain regions involved in different story reading subprocesses. PloS one, 9, e112575. doi: 10.1371/journal.pone.0112575
- Wehbe, L., Vaswani, A., Knight, K., & Mitchell, T. (2014). Aligning context-based statistical models of language with brain activity during reading. In Proceedings of the 2014 conference on empirical methods in natural language processing (pp. 233–243). Doha: Association for Computational Linguistics.
- Willems, R. M. (Ed.) (2015). Cognitive neuroscience of natural language use. Cambridge: Cambridge University Press.
- Willems, R. M., Frank, S. L., Nijhof, A. D., Hagoort, P., & Van den Bosch, A. (2016). Prediction during natural language comprehension. Cerebral Cortex, 26, 2506–2516. doi: 10.1093/cercor/bhv075