ABSTRACT
Prediction facilitates word processing in the moment, but the longer-term consequences of prediction remain unclear. We investigated whether prediction error during language encoding enhances memory for words later on. German-speaking participants read sentences in which the gender marking of the pre-nominal article was consistent or inconsistent with the predictable noun. During subsequent word recognition, we probed participants’ recognition memory for predictable and unpredictable nouns. Our results indicate that individuals who demonstrated early prediction error during sentence reading, showed enhanced recognition memory for nouns overall. Results from an exploratory step-wise regression showed that prenominal prediction error and general reading speed were the best proxies for recognition memory. Hence, prediction error may facilitate recognition by furnishing memory traces built during initial reading of the sentences. Results are discussed in the light of hypotheses positing that predictable words show a memory disadvantage because they are processed less thoroughly.
Introduction
One of the challenges of research on human language comprehension is that language can be processed at an extremely fast pace. During reading, for example, people process about 250 words per minute (Rayner, Citation1998). The ease of language comprehension may be afforded by the fact that, in many of our daily encounters, language is predictable. By actively anticipating upcoming information that is predictable based on context or world knowledge, the reader or listener can alleviate the burden of processing the continuous stream of words incrementally, word by word. In fact, it is now widely accepted that proactive processes of prediction and expectation come to bear during language processing (Bar, Citation2009; Kuperberg & Jaeger, Citation2016; Pickering & Gambi, Citation2018; Van Berkum et al., Citation2005). In situations when early predictions do not pan out, prediction error (i.e. having strong predictions disconfirmed) may have long-lasting consequences on memory representations, so that some researchers now believe that prediction error acts as the driving force behind developmental language learning (Chang et al., Citation2006; Ramscar et al., Citation2013). The goal of the present study is to investigate whether prediction error during sentence encoding has longer-term effects on subsequent recognition of words in adult readers. In this study, the encoding task is operationalised as a self-paced reading task, but we use the broad term encoding to refer to all kinds of experimental tasks that require initial processing or studying of stimuli which are later probed for recognition or recall (retrieval).
Prediction in language processing
In psycholinguistic research, the predictability of a word is normally measured by means of cloze tasks in which native speakers of a language are presented with sentence frames and asked to continue the sentence with the first word that comes to mind (i.e. cloze procedure, Taylor, Citation1953). The cloze probability of a word then corresponds to the proportion of people who responded with that particular word in the cloze task. During language processing, high-predictability words normally show a processing advantage over less predictable words, for example, reduced reading rates (Ehrlich & Rayner, Citation1981; Smith & Levy, Citation2013; for review, see Staub, Citation2015), lower N400 ERP components (for review, see Van Petten & Luka, Citation2012) or reductions in pupil size, signalling reduced processing effort (e.g. Häuser et al., Citation2018). However, in experimental conditions that measure predictability effects at the level of the anticipated word, it can be challenging to dissociate early predictability effects from late-stage integration effects (DeLong et al., Citation2005; Pickering & Gambi, Citation2018; Urbach et al., Citation2020). Since integration is assumed to be a major component of language comprehension anyways, parsimony frequently demands that early predictability effects are instead attributed to late-stage integration.
To date, the strongest evidence for early prediction comes from studies that measure predictability effects not at the level of the predicted noun, but pre-nominally, for example, at the level of a gender-marked article or modifier that precedes a predictable noun. Most languages in the world use gender marking to sort nouns into grammatical classes, and sentence constituents that precede a noun must be marked according to the gender of the head noun. Hence, the gender marking of pre-nominal constituents can be used as an early cue that indicates whether or not a highly anticipated noun will come up later or not. In a seminal study from Dutch, for example, Van Berkum and colleagues (Citation2005) showed that pre-nominal adjectives whose gender was inconsistent with the gender of the noun anticipated through discourse, are read more slowly in self-paced sentence reading (Experiment 2), and evoke different ERP components in EEG recording (Experiment 1), than prediction-consistent adjectives. Hence, readers actively anticipate information about upcoming nouns, including morpho-syntactic aspects such as grammatical gender. When new, incoming information is not consistent with the (gender) predictions generated through context, there is a processing cost.
Prediction and memory
However, very little is currently known about the longer-term consequences of prediction error and about how prediction relates to subsequent episodic memory retrieval for previously encoded words. Intuitively one might think that the use of prediction during language processing could boost subsequent memory, particularly for unpredictable information, because here, the parser might experience some kind of a double-take effect by means of early prediction error and late-stage integration difficulty. This might mean that unpredictable words boost memory by means of deeper semantic elaboration. On the other hand, using top-down prediction during language processing might be detrimental to subsequent memory especially for information that is highly predictable, because readers relying on prediction too much might encode sentences in some kind of a “top-down verification mode” (Van Berkum, Citation2010) that may go at the expense of thorough processing (Rommers & Federmeier, Citation2018a), which then may lead to the formation of less distinct memory traces (Shing & Brod, Citation2016).
Few studies have systematically investigated how prediction error relates to word memory in adult speakers, and the ones that have obtained somewhat mixed findings regarding whether word predictability enhances or impairs subsequent memory. Some studies have demonstrated that unpredictable words show an advantage over predictable words in tests of recognition memory (Corley et al., Citation2007; Federmeier, Wlotko, DeOchoa-Dewald, & Kutas, Citation2007; Rommers & Federmeier, Citation2018b). For example, Corley and colleagues (Citation2007) found that recognition memory for sentence-final nouns (e.g. nails) was enhanced when words were pre previously encoded in an unpredictable sentence context (e.g. That drink’s too hot; I have just burnt my nails) rather than a predictable sentence context (e.g. Everyone’s got bad habits and mine is biting my nails). Findings such as these could indicate that prediction error during encoding triggers processes of re-integration and revision, and consequently, furnishes memory traces. This interpretation also fits with a larger body of evidence from the memory domain suggesting that event novelty (i.e. unexpectedness based on prior world or event knowledge) drives memory (e.g. Schomaker & Meeter, Citation2015).
Other studies, in contrast, have suggested that more predictable, expected, words or events are remembered more successfully in subsequent tests of memory (e.g. Brod et al., Citation2013; Höltje, Lubhan, & Mecklinger, Citation2019; Perry & Wingfield, Citation1994; Riggs et al., Citation1993; Staresina et al., Citation2009). For example, Riggs and colleagues (Citation1993) found that recall and recognition of previously encoded text constituents were systematically enhanced the more predictable these propositions were during an earlier encoding phase. Findings such as these contradict the notion of a prediction-error related memory boost, because they indicate that prediction-consistent information that is encoded against the backdrop of world or event knowledge is remembered more distinctly later on (Craik & Tulving, Citation1975). Indeed, this might be the case because schema representations (i.e. regularities extracted from multiple encounters; Van Kesteren et al., Citation2012) become activated during encoding and lead to the enhanced semantic elaboration and relational binding operations that facilitate later memory retrieval (Staresina et al., Citation2009). Hence, there is also evidence that schema congruency, and not so much novelty or surprisal, may render the memory trace more accessible for subsequent memory tests.
The conditions under which schema congruency and novelty improve or impair memory are not fully understood, but studies have shown that task- and item-related characteristics can push effects in on or the other direction. For example, not all kinds of prediction error might readily trigger superior memory effects, but only those that involve the strongest effects of re-integration and revision during encoding. As an example, when pepper and fox are encoded as exemplars of the category a four-legged animal, the only fox might show a memory advantage later on, because pepper cannot be plausibly integrated given the context (Höltje et al., Citation2019). In line with this, unpredictable-plausible stimuli (e.g. fox in the previous example) have been related to different neural and behavioural signatures during encoding than unpredictable-implausible stimuli (e.g. pepper; see DeLong et al., Citation2014; DeLong & Kutas, Citation2020; Rayner et al., Citation2004). An additional factor that can push memory effects is task demands. In the psycholinguistic literature, for example, high-frequency words normally show a memory advantage in tests of direct recall, but not in tests of word recognition, where low-frequency words show superior memory (dubbed the word frequency paradox; for review see Popov & Reder, Citation2020). Yet other studies have argued that distinctiveness of incongruent information triggers memory, so that for example, the ratio between congruent and incongruent stimuli during encoding might play a role. In encoding situations when incongruent items are sparse, they might be remembered more successfully because they stand out more, whereas in conditions when incongruent stimuli abound, there might be no memory advantage for these items (Reggev et al., Citation2018).
The present study
Here, we aim to establish a more direct link between prediction error and subsequent memory retrieval by relating individual differences in the use of predictive processing during encoding to subsequent recognition memory of nouns. During encoding (a self-paced reading task), native German participants read sentence contexts that were completed either with the most predictable noun, or a noun from a different grammatical class. In these unpredictable sentences, the gender-marking of the pre-nominal definite article could be used as an early cue to indicate whether or not the most expected noun would appear later on. After sentence encoding, participants completed a (surprise) word recognition task, which probed participants’ recognition memory for predictable and unpredictable target nouns.
We had two major research questions. Our first question was whether early prediction error during encoding would enhance or impair subsequent memory retrieval overall. For example, individuals who used early prediction at the level of the gender-marked article might encode sentences in a more thorough and deep semantic fashion, which in turn may boost subsequent memory. On the other hand, the use of predictive processing during encoding could also be detrimental for subsequent memory, due to a “top-down verification mode” and shallower processing overall (cf. Rommers & Federmeier, Citation2018a).
In light of conflicting results from prior literature about memory advantages for events that are congruent or incongruent with a pre-existing knowledge structures (i.e. schema congruency vs novelty), our second question concerned memory effects for predictable and unpredictable nouns. Do predictable or unpredictable nouns show a memory advantage during retrieval, and how does sentence plausibility push around general effects of word predictability?
Materials and methods
Participants
Participants were 70 native speakers of German (47 female and 23 male) between the ages of 18 and 35 (M = 21, SD = 3), a subset of the young adult’s sample in Haeuser et al. (Citation2020). All participants reported normal or corrected-to-normal vision, no history of neurological and/or psychiatric disorders, and acquisition of German from birth. Informed written consent was obtained from all participants. All study procedures were in line with the Helsinki declaration on human subject testing.
Materials
Self-paced reading task
The experimental stimuli consisted of 40 sets of sentence stems, a subset of the 48 experimental items used in Haeuser and colleagues (Citation2020). Each sentence stem was completed either with its most predictable article–noun combination (operationalised as the article–noun combination with the highest cloze probability in prior ratings, see below), and an unpredictable article–noun combination with a different grammatical gender (a continuation that was never produced as possible ending in the cloze ratings), yielding a total of 80 experimental sentences.
To determine the cloze probability of the most predictable continuations, a norming study was conducted with 37 participants who did not participate in the main experiment. Candidate sentence frames were 74 items, truncated before the definite article (e.g. Als Paul endlich seinen Führerschein erhalten hatte, fuhr er ständig mit … // When Paul finally got his driver’s license, he was always driving around with … , predictable continuation dem Auto // the masculine car). Participants were asked to complete each sentence frame with the most sensible ending, and to also provide a second-best alternative. Upon collecting the ratings, the experimenters calculated separate cloze probabilities for the most predictable definite article and the most predictable noun. Items were excluded from the set when the nouns and definite articles had a cloze probability lower than 0.6 and 0.5, respectively. In the final set of 40 sentences, predictable articles had a mean cloze probability of 0.84 (range: 0.54–1.0) and predictable nouns had a mean cloze probability of 0.84 (range: 0.62–1.00).
The experimenters then chose unpredictable endings for each sentence stem, making sure that (a) the unpredictable noun for each sentence stem had a different grammatical gender than the most predictable noun for that stem, and (b) that the unpredictable items were never produced as first- or second-guess completions in the cloze ratings. Here, the goal was to select nouns that were unexpected, but possible and semantically plausible given the sentence context. For example, the unpredictable completion for the sentence Als Paul endlich seinen Führerschein erhalten hatte, fuhr er ständig mit … (predictable completion: dem masculine Auto von Freunden) was the German article-noun combination der feminine Gruppe von Freunden.
By definition then, unpredictable nouns had zero cloze probabilities, but the cloze probabilities of unpredictable articles varied, depending on the fraction of participants who produced a given unpredictable article in the cloze ratings. In the final stimulus set, unpredictable articles had an average cloze probability of 0.04 (range: 0–0.25). shows histograms of article and noun cloze probabilities.
We also conducted a plausibility pre-test on predictable and unpredictable sentences. This test was run through SoSciSurvey, an online survey tool, on 60 native speakers of German who did not participate in the main experiment or in the cloze ratings. Participants were presented with the experimental sentences and asked to rate the plausibility of each item on a five-point Likert scale (1 meaning an item was not plausible at all, 5 meaning an item was very plausible). In this pre-test, unpredictable nouns were rated as significantly less plausible given their sentence contexts (M = 2.20, SD = 0.78) than predictable nouns (M = 4.82, SD = 0.16), t(78) = 20.74, p < .001.
To avoid that sentence-final wrap-up effects during the reading confounded RTs on the noun, the sentences were padded with identical words which continued them plausibly (e.g. … fuhr er ständig mit dem Auto predictable / der Gruppe unpredictable von Freunden auf den Landstraßen herum; English: When Paul finally got his driver’s license, he was always driving around wit the car predictable / the group unpredictable of friends on the roads).
In order to account for spill-over from the definite article onto subsequent words of the sentence during reading, the experimenters inserted three additional words between the definite article and the noun, mostly adverbs and adjectives, for example, … mit dem alten aber zuverlässigen Auto von Freunden, mit der alten aber zuverlässigen Gruppe von Freunden (English: … with the old but reliable car from friends, with the old but reliable group of friends). Here, the goal was to choose modifiers that were semantically compatible with, and did not differently bias, the predictable vs unpredictable continuation of the phrase. Frequency and length characteristics of the three spill-over words are presented in . Independent t-tests that estimated length- and frequency differences between adjectives depending on returned non-significant results for all words (see ).
Table 1. Stimuli characteristics for the three adjectives inserted after the article.
Across sentences, article gender (feminine, masculine and neuter) was counterbalanced over both predictable and unpredictable conditions, and gender types appeared equally frequently in sentences that confirmed semantic expectations and in sentences that violated semantic expectations. Predictable and unpredictable nouns were matched in frequency, based on the Zipf scale from the Subtlex-DE database (Welch’s t(78) = 0.27, p = .78; predictable items: M = 2.80, SD = 0.7; unpredictable items: M = 2.76, SD = 0.67). However, unpredictable nouns were slightly longer than predictable nouns (7 vs 6 characters, respectively), a barely significant difference, Welch’s t(78) = −1.75, p = .08. Note that we added word length as a control variable to all statistical models reported below.
Finally, the 80 sentences were evenly distributed on two experimental lists (npredictable items = 20, nunpredictable items = 20) so that one subject only got to see one experimental version of each item during testing. In order to make sure that, despite the large number of unpredictable sentence continuations, participants continued to make predictions during reading (Brothers, Swaab, & Traxler, Citation2017), 38 moderately predictable sentences from the Potsdam sentence corpus were used as fillers, yielding a total of 78 sentences per list. Comprehension questions (yes/no questions) were created for 25% of all sentences to make sure that participants read the sentences for content. These comprehension questions targeted some aspect or piece of information from the sentence contexts and were designed so that they could not be responded to correctly based on mere world knowledge. Examples of the comprehension questions are presented in . Experimental items and fillers were randomly distributed on each list, with two constraints: (1) No more than four unpredictable items in a row, and (2) no more than three items with comprehension questions in a row. Finally, to prevent trial-order effects, the experimenters created a reversed version of each list, yielding a total of four experimental lists for the self-paced reading task.
Table 2. Examples of comprehension questions that were used in the self-paced reading experiment.
Word recognition task
Experimental items for the recognition task were the 20 predictable and 20 unpredictable words from the self-paced reading task, and 40 new words that did not appear in the self-paced reading task (neither as an experimental noun, nor as any other noun in any sentence that was presented during self-paced reading). The 40 new words were selected from the SUBTLEX-DE data base and had the same frequency and length range and as the experimental (“old”) nouns. All new nouns were also concrete (since virtually all experimental nouns in the self-paced reading task were concrete). Stimuli for the recognition task were then distributed on two lists. During testing, recognition lists were assigned depending on which list a participant had seen during self-paced reading (i.e. when a participant saw item 23 in its unpredictable version with the noun “group” during encoding, they were presented with “group” during the recognition task).
Procedure
The experimental session consisted of the self-paced reading task (∼20 min), followed by the (surprise) word recognition task (∼10 min), and a test battery of standard cognitive tests that assessed working memory capacity, inhibition and context maintenance (∼30 min; not reported here).
In the self-paced reading task, participants read sentences on a screen word-by-word. Each trial started with the presentation of the first word of the sentence, next to a number of underscores, separated by spaces, indicating the number of words to follow (i.e. “moving window” format). By pushing the space bar with their dominant hand, participants proceeded to the next word, and the letters of the previous word were replaced with underscores. Participants were instructed to read the sentences as fast as possible, and to answer all true/false comprehension questions as accurately as possible by pushing the “J” (Yes, correct) and “N” (No, incorrect) bars on the keyboard. Trials were separated by a 500 ms fixation cross.
In the recognition task, participants were presented with the 80 nouns (40 old, 40 new), displayed on a screen one after another. Participants were instructed to indicate, by pressing the J and N bars after each word, whether they “remembered reading that word in the previous task of the study”. Target words stayed on the screen until a response was made. Trials were separated by a 500 ms blank screen.
All sentences and target nouns were presented on a Fujitsu Siemens P-19-2 monitor with a screen resolution of 1280 × 1024 pixels, using a Courier New 18 pt font on a white background. All tasks (including the cognitive test battery) were controlled using the E-Prime 2.0 software (Psychology Software Tools, Pittsburgh, PA).
Results
General note on statistical data analysis
We present separate analyses for recognition accuracy in the noun recognition task, and reading times (RTs) in the self-paced reading (SPR) task. In order to more directly relate encoding RTs to subsequent recognition rates, we also present an exploratory step-wise regression analysis.
For the word recognition task, the dependent variable was response accuracy, a binary variable. For SPR, the dependent variable was reading times (log-transformed to avoid skewing; Gelman & Hill, Citation2007) on all words in the critical region (e.g. the | old | but | reliable | car | of). In both analyses, fixed effects were predictability (a factor with two levels: predictable vs unpredictable; dummy coded with predictable items as the reference category) and z-transformed Pr scores from the word recognition task that reflect discrimination rates between old and new items during word recognition (see below, for details on how this score was computed).
We constructed separate linear mixed-effects models for each dependent variable as implemented in the lme4 library (Bates, Mächler, Bolker, & Walker, Citation2015; version 1.1-19) in R (R Development Core Team, Citation2018; version 3.5.2). To protect against anti-conservative model estimates, all models were initially fit with random intercepts for subjects and items, and random slope adjustments for all corresponding within-subject and within-item effects warranted by the design, including their interactions (i.e. a fully maximal random-effects structure; see Barr et al., Citation2013). Since the maximally specified models frequently did not converge, we simplified each model progressively using the least variance approach until convergence was achieved (following the guidelines established in Barr et al., Citation2013). P-values were estimated using the Satterthwaite degrees of freedom method, as implemented in the R package lmerTest (Kuznetsova et al., Citation2017).
All statistical models reported below were fit with word length and trial number as control variables, scaled to reduce multicollinearity.Footnote1 Note that, for RTs on the gender-marked article, word length was not included as a control variable because it was constant across conditions (three characters). For RT analyses, the corresponding graph is shown using residual RT (i.e. with effects of length partialled out). Prior to analysis, and based on visual inspection, RT data from SPR were trimmed minimally by excluding RTs > 1500 ms for pre-nominal target words, and by excluding RTs > 3000 ms for nominal and post-nominal target words. Altogether, this exclusion rate retained more than 98% of all data points.
Word recognition task
On average, participants correctly recognised 69% of the “old” words in the word recognition task, and false-alarmed to an average of 9% of the new words. These rates are similar in value to prior studies (e.g. Corley et al., Citation2007; Federmeier et al., Citation2007; Wlotko, Federmeier, & Kutas, Citation2012).
To obtain an estimate of participants’ overall recognition performance, we computed the probability of true recognition score (Snodgrass & Corwin, Citation1988) for each individual, by subtracting subject-wise false alarms from subject-wise hits. Lower Pr scores represent poorer discrimination rates between old and new items, thereby indicating lower levels of accurate recognition overall. In contrast, higher Pr scores correspond to better discrimination rates between old and new items, and, therefore, indicate better recognition overall. For illustration purposes only, participants were then divided into groups of Low Recognisers (n = 35) and High Recognisers (n = 35) based on a median split of all Pr scores.
In a first step, in order to estimate recognition rates depending on noun predictability, we conducted a trial-by-trial analysis on accuracy values for predictable vs unpredictable nouns. The corresponding glmer-model was fit with additional control variables for noun frequency (based on the log-transformed SUBTLEX-DE estimates in Brysbaert et al., Citation2011) and scaled noun plausibility. As an additional control variable, we also added log-transformed reaction times for the memory response in each trial, in order to ascertain that there were no speed-accuracy tradeoffs.Footnote2 The lme4-formula for models in this section was glmer(accuracy ∼ condition + log(RT) + scale(plausibility) + log(frequency) + (1+condition|subject) + (1 + condition|item), data = data, family = binomial). The condition was entered into the model as a dummy-coded variable, with predictable items set as the reference category. Variance inflation factors (VIFs) for the final model were moderately high for condition and plausibility (VIF = 6.2), which could potentially indicate multicollinearity between the two predictors (VIFs for word RTs and frequency were not problematic: 1 and 1, respectively). In order to assess whether multicollinearity was, indeed, a cause of concern for our model, we followed the procedure described in Tomaschek et al. (Citation2018), which suggests running a stripped model, with only one of the predictors, and a full model, with both predictors. When the sign of the effect changes while comparing the stripped to the full model, or when the z-value increases dramatically, this might be indicative of collinearity. Indeed, when we ran the two models (which were run without the control predictors for word frequency and trial RT since they were diagnostic in nature), the sign of the condition effect stayed the same, but the z-value of the condition effect moderately increased from z = −2.00 in the stripped model to z = −2.49 in the full model. Therefore, in order to gauge the independent contributions of our condition variable and word plausibility, we ran separate models for each of the predictors, without including the other one as a control predictor.
In the condition model, we found a significant, negative-going simple effect of condition (b = –.50, SE = 0.22, z = −2.28, p = .02), suggesting that, overall, recognition memory was less accurate for unpredictable vs predictable nouns (see ). There was also a marginally significant effect for the control variable noun frequency. Specifically, as word frequency increased, recognition memory decreased (in other words, recognition memory was more accurate for low-frequency as compared to high-frequency nouns; b = –.03, SE = 0.15, z = −1.93, p = .05).
Figure 2. Accuracy rates during word recognition depending on encoding condition (predictable vs unpredictable). Black triangles indicate average values per condition.
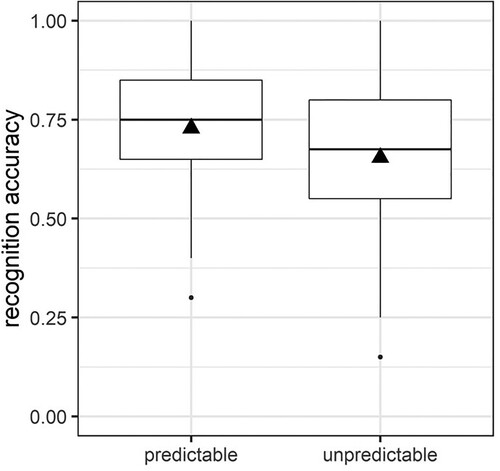
Crucially, the model for the effect of noun plausibility showed no further effects of interest (effect of plausibility: b = .13, SE = 0.11, z = 1.22, p = .22), except for a marginally significant effect of noun frequency that was similar in magnitude and direction to the one in the condition model (b = −0.26, SE = 0.15, z = −1.70, p = .08).
In a second step, we added the continuous variable of subject-wise Pr scores as an interaction variable to the model. The goal of this model was to check whether the condition effect that emerged in model 1 (i.e. better recognition for predictable vs unpredictable words) was driven by all subjects alike or whether subgroups of participants (low vs high recognisers) were more likely to show condition effects. In that model, the continuous variable for Pr scores was scaled (i.e. centred around its mean) to reduce multicollinearity. As control variables we again entered log word frequency and log trial RTs. The lme4-formula for this model was glmer(accuracy ∼ condition × scale(Pr_score) + log(RT) + log(frequency) + (1+condition|subject) + (1 + condition:scale(Pr_score)|item), data = data, family = binomial). We found a significant interaction between predictability condition and recognition performance (i.e. Pr scores, b = .19, SE = 0.09, z = 2.06, p = .04). The boxplot of this interaction (see ) suggests that the effects of reduced recognition accuracy for unpredictable items were predominantly driven by the group of low recognisers, since only in this group, recognition accuracy was lower for unpredictable vs predictable items. High recognisers, in contrast, seemed to recognise predictable and unpredictable nouns equally correctly.
Figure 3. Accuracy rates during word recognition depending on encoding condition (predictable vs unpredictable) and subject-wise recognition scores (reflecting the discrimination between old and new items). Black triangles indicate average values per condition.
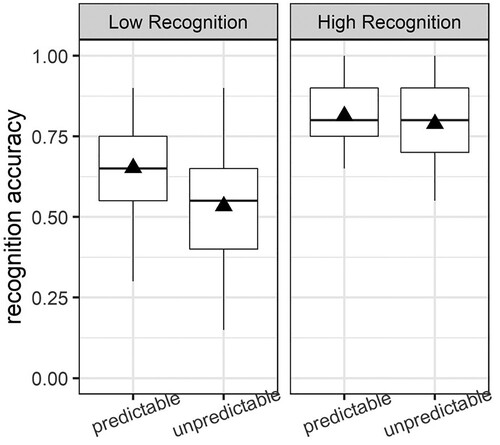
Hence, whereas recognition memory for previously predictable nouns was relatively high across the board in all subjects, intact recognition of previously unpredictable nouns was found only in a subgroup of subjects, that is, those who showed better discrimination between old and new items.
In sum, recognition memory varied as a function of word predictability. Irrespective of memory Pr scores, we found that word predictability increased word recognition performance, that is, predictable nouns were recognised more correctly during word recognition than unpredictable nouns. When we took into account subject-wise Pr scores, however, we found that the reduced recognition rates for unpredictable nouns were predominantly driven by the group of low recognisers, that is, those individuals who showed lower discrimination ability between “old” and “new” items during word recognition across the board. In contrast, high recognisers performed equally correctly in recognising predictable and unpredictable nouns. The question that arises is, whether these diverging recognition rates can be traced back to differences in sentence encoding during the self-paced reading task. It is with this question in mind that we now turn to the analysis of the self-paced reading results.
Self-paced reading task
Bevavioural accuracy
Accuracy on the comprehension questions was high overall (M = 0.98, SD = 0.03), with no significant comprehension differences between low (M = 0.98) and high recognisers (M = 0.98), t(68) = 1.06, p = .3. Similarly, subject-wise accuracy rates did not differ between predictable (M = 0.98, SD = 0.05) and unpredictable (M = 0.98, SD = 0.05) items, t(69) = −0.90, p = 0.38. These results suggest that participants were attentive during the experiment and understood the sentences they were reading. All RT analyses below were conducted, and all results are reported, with incorrect responses removed.
Reading times
Of critical interest to our research question is whether predictability effects during word recognition are related to predictability effects during the encoding phase, especially on critical words before the noun (i.e. pre-nominally), as these constitute strongest evidence in favour of prediction (see Pickering & Gambi, Citation2018; Urbach et al., Citation2020). Since prenominal prediction effects during reading could emerge on any of the four words preceding the critical noun (e.g. thearticle oldspill 1 butspill 2 reliablespill 3), we adjusted the p-value threshold for statistical significance for these analyses using the Bonferroni correction to p < .0125. (corresponding to .05/4). For later-emerging predictability effects (e.g. at the noun), we corrected the p-value threshold to .025, since we analysed condition effects at the noun (i.e. car) and the subsequent spill-over word after the noun (i.e. of [friends]). shows the length-corrected RTs for encoding RTs of the critical target words, split out by low and high recognisers. and show the corresponding model outputs; asterisks indicate statistical significance when applying the correction for multiple comparisons as reported above ( and ).
Figure 4. Residual (length-corrected) RTs for target words in predictable and unpredictable items, split out by high and low Pr recognition scores (note: all models were run with the scaled continuous variable). Words displayed correspond to the example item, When Paul finally got his driver’s license, he was always driving around with the old but reliable car/group of friends.
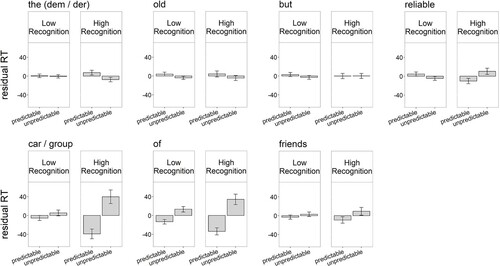
Table 3. Effect sizes (b), standard errors (SE), and t-values for models estimating the effects of condition and recognition scores on log-transformed RTs of the article, and the first and second word in the spill-over region (e.g. thearticle oldword 1 butword 2 reliable word 3 car/group).
Table 4. Effect sizes (b), standard errors (SE), and t-values for models estimating the effects of condition and recognition Pr scores on log-transformed RTs of the the third spill-over word, the noun, and the noun spill over region (e.g. … . reliable spill-over 3 car/group noun of noun spill-over 1).
Closer inspection of suggests that low and high recognisers demonstrated remarkable differences with respect to the time course of sentence encoding. Whereas high recognisers seemed to show strong predictability effects at the level of the noun and the spill-over word after the noun, low recognisers showed predictability only at the level of the spill-over word after the noun. In addition, in low recognisers, predictability effects seemed less pronounced overall compared to high recognisers.
These observations were confirmed by a series of models on log RTs which estimated condition effects in interaction with recognition performance (i.e. the scaled Pr scores for recognition performance; see for outputs).Footnote3 Again, these models were run on trial-by-trial unaggregated data. The lme4-formula for models in this section was log(RT) ∼ condition * scale(Pr_score) + scale(word_length) + scale(trial) + (1+condition|subject) + (1 + condition*scale(Pr_score)|item), data = data.
At the level of the noun, there was a significant condition by recognition interaction (b = .05, SE = 0.01, t = 4.12, p < .0001), suggesting that only high, and not low, recognisers showed comprehension difficulties when reading unpredictable nouns. In the model for RTs on the spill-over word after the noun, however, the condition by recognition interaction was not significant (b = .02, SE = 0.01, t = 1.90, p = .06); instead, there was a significant effect for condition (b = .07, SE = 0.02, t = 4.21, p < .0001), suggesting integration difficulties for unpredictable items in both subject groups. Notably, though, predictability effects during encoding were less pronounced in low compared to high recognisers, as follow-up models showed: At the noun spill-over word after the noun, the parameter estimates (b’s) for the effect of condition were numerically lower for low recognisers (b = .06) compared to high recognisers (b = .09), even though in both models, the condition effect was significant (low recognisers: t = 4.40, p < .0001; high recognisers: t = 5.27, p < .0001).
Hence, high recognisers showed comprehension difficulties when reading unpredictable nouns. In low recognisers, this effect seemed to emerge somewhat later in the sentence (only at the level of the spill-over word after the noun), and in addition, the effect was less pronounced overall.
Of crucial interest here is whether there were pre-nominal prediction effects, in the form of condition effects before the noun. Notably, it seems that this was the case in high recognisers: suggests that these participants showed prolonged RTs when encoding the pre-nominal target word in unpredictable items (see , panel “reliable”), whereas low recognisers seemed to read pre-nominal target words equally fast, irrespective of whether they were presented in a predictable and unpredictable condition.
Again, these observations were confirmed by lmer models (see for the summary) whose p values were adjusted for multiple comparisons. At the level of the pre-nominal target word that immediately preceded the noun (e.g. reliable in the old but reliable car/group), there was a significant condition by recognition interaction (b = 0.02, SE = 0.01, t = 2.62, p = .009). Follow-up models on untransformed RTs that were run in order to estimate the size of the predictability effect in raw RTs showed that the predictability effect in high recognisers amounted to 17 ms (a marginally significant effect; b = 16.66, SE = 8.62, t = 1.93, p = .05), whereas in low recognisers the predictability effect was −6 ms (i.e. numerically faster RTs for unpredictable target words, a non-significant effect; b = −5.99, SE = 6.56, t = –.91, p = .4). There were no condition effects on any other pre-nominal target word (see ), neither with nor without the correction for multiple comparisons.Footnote4
To sum up the effects during word encoding, when reading unpredictable sentences that violated semantic expectancies, only high recognisers showed early, pre-nominal effects of having predictions disconfirmed. In addition, high recognisers demonstrated later-emerging comprehension difficulties at the level of the noun and the spill-over word after the noun. For low recognisers, in contrast, there were no prenominal effects of predictability, instead, low recognisers only showed late predictability effects at the level of the spill-over word after the noun. The question that emerges is whether these RT differences during online encoding can be related more directly to recognition rates during subsequent recognition. It is with this question in mind that we now turn the results of the stepwise regression analysis.
Exploratory step-wise regression analysis
The step-wise regression analysis presented below was exploratory in nature, as it was motivated by the findings reported above. The goals of this analysis were two-fold. First, one potential concern about the self-paced reading results presented above could be that high recognisers showed prolonged encoding RTs compared to low-recognisers on all target words in the critical region (see ). Because of this, it is unclear whether the superior recognition rates in high recognisers were actually driven by their general reading speed (or some mechanisms of general attention during the experiment).
A second goal of the step-wise regression analysis was to determine whether recognition accuracy was driven by relatively early vs later predictability effects during reading. Specifically, we asked whether early, pre-nominal prediction costs or relatively later emerging noun comprehension costs were better predictors of recognition memory. The pre-nominal prediction cost was computed by residualizing (i.e. length-correcting) the RT difference between unpredictable vs predictable items on the third spill-over word after the article, later emerging noun comprehension cost scores. The later emerging noun comprehension cost was computed by residualizing the RT difference for unpredictable vs predictable items at the noun, that is, car/group.
The dependent variable in this analysis was subject-wise hit rates for “old” (i.e. previously seen) items. As predictor variables, we entered the subject-wise residual RT cost scores for the third spill-over word after the article (i.e. the old but reliable car), calculated as the difference between unpredictable and predictable items, as well as subject-wise residual RT cost scores for the RT difference between unpredictable and predictable nouns (i.e. the old but reliable car/group). As a third predictor variable, we entered the subject-wise average residual RTs of the third, fourth and fifth word of each sentence during encoding. This latter variable was added to the model in order to target the concern that general reading speed across individuals drove recognition performance.Footnote5 We chose relatively sentence-initial words for this predictor variable, because sentence-early words are arguably least confounded by effects of increasing constraint that generally reduce reading rates in the course of reading/encoding a sentence (see Staub, Citation2015). We chose a grand average of three words (as opposed to one word only) because the sentence-early words in our target sentences were not controlled for along any psycholinguistic variable such as frequency or concreteness, so it seemed the safer choice to average over multiple words.
All predictors were entered into the regression as scaled continuous variables (i.e. with M = 0 and SD = 1) to reduce multi-collinearity. Variance inflation factors (VIFs) for the three model predictors were <1, which is way below values deemed problematic even under more strict accounts of multicollinearity (see Tomaschek et al., Citation2018, for discussion).
In what follows, we used a stepwise multiple regression approach to compare an intercept-only model (i.e. a model that predicted hit rates by the grand mean) to a full model that included the intercept, the residual prenominal prediction cost scores, the residual integration cost scores, and the average residual encoding RTs per subject as predictors. The model fitting procedure was forward and backward, that is, new predictors were only added to the model if they improved model fit, and old predictors were discarded after each step if they became irrelevant after the adding of new variables.
The results showed that the best-fitting model (determined by means of lowest AIC) was one that included general RTs and the prenominal prediction costs, but not the integration costs at the noun. In that model, scaled general RTs as well as the scaled prediction costs were both significant predictors of recognition rates (b = 0.05, SE = 0.02, t = 3.1, p = .01; b = 0.04, SE = 0.02, t = 3.0, p = .04, respectively; see , for correlation plots).
Figure 5. Correlation plots and Pearson correlation coefficients, illustrating the relationship between residualized reading times during encoding (left panel: average RTs per subject for sentence-early words; right panel: prediction cost at word spill 3 (i.e. reliable) per subject) and recognition memory.
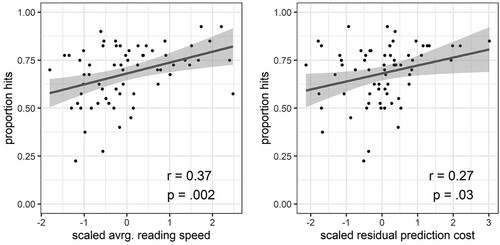
Hence, recognition memory for previously seen items was related to general reading speed during encoding and to the size of the prediction error subjects experienced during encoding. Of relevance here is that prediction cost scores explained a significant amount of variance in recognition memory, over and above individual differences in reading speed. In contrast to prenominal prediction costs, integration costs at the noun did not drive recognition accuracy. We return to this point in the general discussion.
Summary
Our self-paced reading and subsequent word recognition tasks showed four key findings.
First, recognition memory was generally higher for predictable nouns, that is, contextually expected words that continued a sentence context plausibly.
Second, relatively intact recognition memory for unpredictable (compared to predictable) nouns only emerged in high recognisers, that is, those individuals who showed better discrimination between old and new items during word recognition overall.
Third, during self-paced reading, high recognisers also showed pre-nominal prediction costs, in that they read pre-nominal adjectives in contextually unpredictable items more slowly (compared to adjectives in predictable items).
Fourth, the best predictors for subsequent word memory were early prediction costs, as well as individual differences in general reading speed during encoding. Integration costs at the noun were not predictive of recognition.
Discussion
In this study, we used a self-paced reading (SPR) and subsequent word recognition task to investigate whether prediction error during encoding relates to subsequent retrieval from episodic memory. During SPR, we presented gender-marked German nouns that were predictable or unpredictable based on prior sentence context. Critically, the pre-nominal gender-marked article (i.e. the) and the spill-over region after the article (i.e. old but reliable) could be used as an early cue that indicated whether or not the sentence was continuing as predicted. In the recognition task, participants performed old-new judgments on nouns they had previously encountered in predictable and unpredictable conditions (“old” items), and on new nouns that they had not previously seen (“new” items).
Our results indicate that early prediction error during encoding is related to enhanced memory performance later on. Not only did individuals with higher recognition memory show early (pre-nominal) effects of having predictions disconfirmed in that they showed slower reading for unpredictable items, a finding that did not emerge for low recognisers. Exploratory step-wise regression analyses demonstrated that, across subjects, higher prediction cost scores (i.e. larger RT differences between unpredictable and predictable items) and individual differences in encoding speed, but not later-emerging comprehension difficulty at the noun, were the best predictors of subsequent memory performance.
As such, our data seem to indicate that different temporal characteristics during encoding may give rise to different memory patterns: Whereas high recognisers showed early and late prediction costs that emerged both pre-nominally and nominally, low recognisers only showed relatively late predictability costs (not at, but after the noun), and, crucially, no pre-nominal prediction. Thus, having predictions disconfirmed early on might be related to more successful retrieval from episodic memory, later on, maybe because prediction errors garner memory traces during encoding, and make them more distinct during subsequent memory tests.
In the introduction, we hypothesised that early prediction during sentence reading might enhance memory for encoded words, because the “double take” effect of early prediction error and relatively later emerging noun integration difficulty might furnish memory traces and lead to more distinct representations. On the other hand, the use of predictive processing during encoding might also be detrimental for subsequent memory, because the strict use of a “top down verification” mode (Rommers & Federmeier, Citation2018a; Van Berkum, Citation2010) might be too superficial during encoding to leave long-lasting and robust memory traces. Unfortunately, our data do not clearly support one hypothesis over the over; at best, there seems to be weak evidence for both.
On the one hand, we found that there were early and late prediction costs in individuals who showed enhanced word recognition memory. This may, indeed, advocate that “double take” predictability effects during encoding enhance memory. However, according to our stepwise regression analysis, the late noun comprehension cost was not predictive of memory at all, despite the fact that comprehension difficulties for unpredictable nouns did emerge across subjects during reading. This is important because it indicates that, even though high recognisers showed prediction error prenominally, they did not completely revise their expectations as to the sentence continuation, so that the unpredictable noun still came “as a surprise” to them.
On the other hand, some aspects of our findings also seem to advocate the second hypothesis, that is, that purely context-driven, top-down processing might be detrimental for subsequent memory, at least if we understand this kind of top-down processing as implying superficiality and, maybe, increased speed. With respect to this account, we found that faster encoding (which could be indicative of shallower processing and less attention) seemed to diminish memory, whereas slower reading speed (i.e. deeper processing, more attention) seemed to enhance it. This seems to advocate the “top down” verification mode. Again, however, this interpretation is somewhat clouded by another aspect of our findings, specifically, that contextually predictable nouns were remembered relatively successfully across the board, even though these items were read consistently more quickly during encoding.
Overall then, given our data, we cannot really adjudicate between these two accounts, at least not in a straightforward manner. This might be especially true because top-down sentence verification on the one hand, and reading costs for unpredictable information on the other, may not be mutually exclusive at all. In fact, it is conceivable that readers encode predictable words very quickly (they may even skip reading them altogether; see Staub, Citation2015), but show slow-down and comprehension difficulty when encountering unpredictable information. In fact, one might actually be dependent on the other.
A second question in this study concerned recognition memory for nouns that were previously predictable and unpredictable based on context, and how sentence plausibility affected memory. We found that word predictability enhanced recognition rates across the board, that is, predictable nouns were remembered more successfully than unpredictable nouns. In a separate analysis that probed word recognition based on noun plausibility, and irrespective of predictable or unpredictable condition, no effects emerged. What can we conclude from this? Do these results mean that word plausibility does not matter for recognition? One reason for the lack of an effect could lie in the nature of the (im)plausibility of our experimental sentences. Specifically, our experimental sentences might have been too implausible for the noun to be remembered more successfully. For example, in a prior study, Federmeier and colleagues (Citation2007) showed that recognition memory was enhanced for unpredictable nouns that were somewhat plausible (e.g. He bought her a diamond necklace for her collection, when birthday is predicted), compared to fully predictable-plausible items (i.e. birthday, in this context). In that and other studies, unpredictable (but plausible) nouns elicited a late frontal positivity during encoding, a brain component that has been associated with prediction error and effortful updating, re-analysis or repair (DeLong et al., Citation2014; DeLong & Kutas, Citation2020; Kuperberg, Citation2007; Van Petten & Luka, Citation2012). Crucially, outright implausible or semantically anomalous nouns (e.g. For the snowman’s nose, the children used a groan; DeLong et al., Citation2014) have not been associated with processes of repair and updating during encoding. This might explain why plausibility did not have more of an effect on recognition rates in the present study – we may have used the wrong type of implausibility to find an experimental effect (also see DeLong & Kutas, Citation2020; Quante et al., Citation2018). Current studies conducted in our lab right now target the effects of word plausibility more directly by exploring recognition memory for unpredictable nouns that additionally differ in (im)plausibility, that is, nouns that are mildly and deeply implausible given the sentence context (Van De Meerendonk, Kolk, Vissers, & Chwilla, Citation2010).
A remaining question in our study is whether the diverging encoding characteristics of low and high recognisers were accidental, or whether they emerged as a consequence of different encoding strategies used by the two participant groups. Our data cannot directly speak to this issue, but we know from eye-tracking research that readers integrate different kinds of information during distinct, only partially overlapping time windows during encoding (Rayner et al., Citation2004; Warren & McConnell, Citation2007), and that they can delay their commitment to a certain interpretation of a sentence constituent altogether (Frazier & Rayner, Citation1990; Frisson & Pickering, Citation1999; Sanford & Sturt, Citation2002). We could speculate that the low recognisers among our participants might have used such a delayed processing strategy that somewhat postponed their interpretation of unpredictable items during encoding to a somewhat later portion of the sentence, that is, after the noun. It is, however, unknown, why the low recognisers would have adopted such a strategy, and also, why the high recognisers would have not. Future research might be able to address this issue by probing participants’ awareness of expected and unexpected sentence continuations during different time points of sentence encoding.
A more pressing question given our data is whether can explain our recognition effects by means of some encoding characteristic other than early prediction. For example, during the self-paced reading task, high recognisers showed longer reading times than low recognisers overall (see ). One may argue that the low recognisers were simply not paying much attention to the sentences, and that it was these lapses of attention that drove their lower memory rates for critical nouns, not the fact that they showed no effects of early prediction during encoding. Based on our data, we believe not, at least not entirely. First, proportions of correct responses to the comprehension questions during encoding were generally high for both high and low recognisers, so it is unlikely that low recognisers were not paying attention to the sentences. This argument gains even more credibility by the fact that the comprehension questions were designed to probe details about the previously read sentences (see ) and could not be responded to based on mere world or event knowledge. Second, results from the exploratory stepwise regression directly targeted this concern, by showing that prediction cost explained a significant amount of variance over and above the variance explained by subject-wise differences in reading speed. Hence, individual differences in reading speed between high and low recognisers, even though they definitely existed, cannot fully explain our results. Nevertheless, there are proxies for attention that might be able to address this concern more directly in future studies. For example, one possibility could be to introduce comprehension questions on every trial during encoding, not only on 25%, as done in the present study. Another alternative could be to probe recollection in the memory task more strictly, by asking participants for their confidence when making recognition judgments, or by probing source memory of events. This latter point could be accomplished by asking participants not only whether they remembered seeing a particular noun, but also whether it was preceded by a certain verb.
Another open question in the context of the memory task remains why cost scores at predictable and unpredictable nouns were not predictive of recognition rates. This conclusion was suggested not only by the step-wise regression analysis presented above, but also by an additional follow-up analysis (suggested during review) which used comprehension accuracy among subjects as a measure for general attention during the experiment. The results from both analyses converged in showing that noun cost scores did not significantly predict recognition rates. This finding seems somewhat strange, since the noun, as the phrase head, was in a prominent position in our experimental sentences, so it should have affected recognition. In addition, it is conceivable that the adjectives and adverbs inserted before the noun rather increased than decreased people’s expectation as to the noun completion of the phrase, which should have made the noun stand out even more in memory. However, one potential explanation for this finding could be the serial position of the noun in the sentence, that is, the fact that the adjective always came before the noun. If the pre-nominal adjective was already perceived as prediction-inconsistent during sentence encoding (at least by some readers), it is conceivable that, for these individuals, the following noun could not take more of an effect. One argument against this hypothesis, however, is the fact that unpredictable nouns still elicited substantial comprehension difficulties in participants, even in those who demonstrated slowed reading at unpredictable adjectives that preceeded the noun. Consequently, based on our data we cannot really answer that question, but future studies might be able to explore this question more directly by additionally calculating subsequent memory effects (SMEs) for forgotten and remembered items.Footnote6 SMEs are often used in the memory literature to relate first-pass encoding to subsequent retrieval from memory, as they allow for separate analyses for subsequently remembered and forgotten items (see, e.g. Wagner et al., Citation1998; Otten & Rugg, Citation2001). Such an approach might be helpful in answering that question because it allows researchers to examine the fate of remembered vs forgotten words during the encoding phase more directly. The relatively low number of items in the present study (n = 40) prevented us from using this strategy in a follow-up analysis (as it resulted in an unbalanced design and massively inflated singular-fit warnings in model outputs), but SMEs might be a fruitful avenue for future studies.
In sum, our data lend support to accounts positing that memory is driven by both schema congruency and novelty. On the one hand, our data are in line with the view that schema congruency supports memory, since we found that contextually predictable sentences were recognised successfully in all participants. On the other hand, our data also indicate that novelty, or prediction mismatch during encoding, can enhance memory. Finally, with respect to linguistic prediction more generally, our findings suggest that language users can generate very specific expectations about upcoming linguistic information during language processing. Crucially, they appear to do so with sufficient granularity as to predict the grammatical gender of nouns. Nevertheless, early predictability effects on prediction-inconsistent gender-marked words during reading might be relatively small in size (only 17 ms in the present study; a rate comparable to for example, Van Berkum et al., Citation2005, who found an effect of 21 ms), compared to the integration difficulties that emerge at unpredictable nouns (in this study, 60 ms in raw RTs). In addition, there was no main prediction effect for the whole sample of subjects, but only in a subset of participants. The attentive reader might wonder whether this finding supports accounts arguing that the use of prediction in the language is limited in scope (e.g. Huettig & Guerra, Citation2019), and indeed, whether prediction matters that much for language processing at all. We believe it does. For example, we know that the sample of younger adults tested here showed prediction effects that not only depend on the predictability of items (as investigated here), but also on balancedness of items, that is, whether items created balanced or biased expectations towards multiple or few sentence continuations (see Haeuser et al., Citation2020). Based on these and other data, we believe that prediction does matter for language comprehension. Put differently, why would humans not draw on and use all sources of information that can make language comprehension faster and easier?
Conclusion
In sum, although there is a clear evidence that predictive processing can aid language processing in the moment, the long-term consequences of prediction remain less clear. This study demonstrated initial evidence that early prediction error during encoding can act in enhancing subsequent recognition memory for words. We believe this might be the case because prediction error may furnish memory traces and renders them more distinct for later retrieval. Future studies seeking to corroborate and extend our results could investigate how sentence plausibility, over and above predictability, affects memory for words, and whether effects obtained using word recognition tasks hold up or change when using, e.g., direct or cued recall.
Acknowledgments
The authors wish to thank all students and RAs who helped with stimulus construction and data acquisition for this study.
Disclosure statement
No potential conflict of interest was reported by the author(s).
Additional information
Funding
Notes
1 We included trial number in order to account for effects of fatigue or general customization with the experiment. Word length was included because our items were not controlled for along this variable (see “Materials” section).
2 Based on visual inspection of the data, trials with RTs longer than 6000 ms were removed, a procedure that retained 98% of all data points, a justifiable rate according to Ratcliff (Citation1993).
3 Of note, we replicated all findings reported below when using inverse RTs and raw, untransformed RTs, as dependent variable.
4 A reviewer asked whether the condition by Pr score interaction on the third spill-over word might be caused by non-linearities in the PR score variable. We checked for this by means of residual plots, where Pearson's residuals are expected to be approximately uniform in the y direction if the model is correctly specified. The resulting plots did not raise concerns about a non-linear relationship for this variable.
5 A reviewer additionally suggested we use subject-wise accuracy averages from the self-paced reading comprehension questions as a predictor for attention. These analyses showed the same overall effects, in that the pre-nominal cost score for the third spill-over word (b = 0.25, SE = 0.12, t = 2.04, p = .05), but not the noun cost score (b = 0.2, SE = 0.12, t = 1.68, p = .1), significantly predicted recognition rates among subjects. Interestingly, comprehension accuracy did not significantly predict recognition accuracy (b = 0.1, SE = 0.12, t = 0.91, p = .4).
6 We would like to thank an anonymous reviewer, for suggesting this to us.
References
- Bar, M. (2009). The proactive brain: Memory for predictions. Philosophical Transactions of the Royal Society B: Biological Sciences, 364(1521), 1235–1243. https://doi.org/10.1098/rstb.2008.0310
- Barr, D. J., Levy, R., Scheepers, C., & Tily, H. J. (2013). Random effects structure for confirmatory hypothesis testing: Keep it maximal. Journal of Memory and Language, 68(3), 255–278. https://doi.org/10.1016/j.jml.2012.11.001
- Bates, D., Mächler, M., Bolker, B., & Walker, S. (2015). Fitting linear mixed-effects models using lme4. Journal of Statistical Software, 67(1), 1–48. https://doi.org/10.18637/jss.v067.i01
- Brod, G., Werkle-Bergner, M., & Shing, Y. L. (2013). The influence of prior knowledge on memory: A developmental cognitive neuroscience perspective. Frontiers in Behavioral Neuroscience, 7, 139. https://doi.org/10.3389/fnbeh.2013.00139
- Brothers, T., Swaab, T. Y., & Traxler, M. J. (2017). Goals and strategies influence lexical prediction during sentence comprehension. Journal of Memory and Language, 93, 203–216. http://doi.org/10.1016/j.cognition.2014.10.017
- Brysbaert, M., Buchmeier, M., Conrad, M., Jacobs, A. M., Bölte, J., & Böhl, A. (2011). The word frequency effect: A review of recent developments and implications for the choice of frequency estimates in German. Experimental Psychology, 58(5), 412–424. https://doi.org/10.1027/1618-3169/a000123
- Chang, F., Dell, G. S., & Bock, K. (2006). Becoming syntactic. Psychological Review, 113(2), 234–272. https://doi.org/10.1037/0033-295X.113.2.234
- Corley, M., MacGregor, L. J., & Donaldson, D. I. (2007). It’s the way that you, er, say it: Hesitations in speech affect language comprehension. Cognition, 105(3), 658–668. https://doi.org/10.1016/j.cognition.2006.10.010
- Craik, F. I., & Tulving, E. (1975). Depth of processing and the retention of words in episodic memory. Journal of Experimental Psychology: General, 104(3), 268–294. https://doi.org/10.1037/0096-3445.104.3.268
- DeLong, K. A., & Kutas, M. (2020). Comprehending surprising sentences: Sensitivity of post-N400 positivities to contextual congruity and semantic relatedness. Language, Cognition and Neuroscience, 35(8), 1044–1063. https://doi.org/10.1080/23273798.2019.1708960
- DeLong, K. A., Quante, L., & Kutas, M. (2014). Predictability, plausibility, and two late ERP positivities during written sentence comprehension. Neuropsychologia, 61, 150–162. https://doi.org/10.1016/j.neuropsychologia.2014.06.016
- DeLong, K. A., Urbach, T. P., & Kutas, M. (2005). Probabilistic word pre-activation during language comprehension inferred from electrical brain activity. Nature Neuroscience, 8(8), 1117–1121. https://doi.org/10.1038/nn1504
- Ehrlich, S. F., & Rayner, K. (1981). Contextual effects on word perception and eye movements during reading. Journal of Verbal Learning and Verbal Behavior, 20(6), 641–655. https://doi.org/10.1016/S0022-5371(81)90220-6
- Federmeier, K. D., Wlotko, E. W., De Ochoa-Dewald, E., & Kutas, M. (2007). Multiple effects of sentential constraint on word processing. Brain Research, 1146, 75–84. https://doi.org/10.1016/j.brainres.2006.06.101
- Frazier, L., & Rayner, K. (1990). Taking on semantic commitments: Processing multiple meanings vs. Multiple senses. Journal of Memory and Language, 29(2), 181–200. https://doi.org/10.1016/0749-596X(90)90071-7
- Frisson, S., & Pickering, M. J. (1999). The processing of metonymy: Evidence from eye movements. Journal of Experimental Psychology: Learning, Memory, and Cognition, 25(6), 1366–1383. https://doi.org/10.1037/0278-7393.25.6.1366
- Gelman, A., & Hill, J. (2007). Data analysis using regression and multilevelhierarchical models (Vol. 1). Cambridge University Press.
- Haeuser, K. I., Kray, J., & Borovsky, A. (2020). Great expectations: Evidence for graded prediction of grammatical gender. In S. Denison, M. Mack, Y. Xu, & B. C. Armstrong (Eds.), Proceedings of the 42nd annual meeting of the Cognitive Science society (pp. 1157–1163). Cognitive Science Society.
- Häuser, K. I., Demberg, V., & Kray, J. (2018). Surprisal modulates dual-task performance in older adults: Pupillometry shows age-related trade-offs in task performance and time-course of language processing. Psychology and Aging, 33(8), 1168–1180. https://doi.org/10.1037/pag0000316
- Höltje, G., Lubahn, B., & Mecklinger, A. (2019). The congruent, the incongruent, and the unexpected: Event-related potentials unveil the processes involved in schematic encoding. Neuropsychologia, 131, 285–293. http://doi.org/10.1016/j.neuropsychologia.2019.05.013
- Huettig, F., & Guerra, E. (2019). Effects of speech rate, preview time of visual context, and participant instructions reveal strong limits on prediction in language processing. Brain Research, 1706, 196–208. https://doi.org/10.1016/j.brainres.2018.11.013
- Kuperberg, G. R. (2007). Neural mechanisms of language comprehension: Challenges to syntax. Brain Research, 1146, 23–49. https://doi.org/10.1016/j.brainres.2006.12.063
- Kuperberg, G. R., & Jaeger, T. F. (2016). What do we mean by prediction in language comprehension? Language, Cognition and Neuroscience, 31(1), 32–59. https://doi.org/10.1080/23273798.2015.1102299
- Kuznetsova, A., Brockhoff, P. B., & Christensen, R. H. (2017). Lmertest package: Tests in linear mixed effects models. Journal of Statistical Software, 82(13), 1–26. https://doi.org/10.18637/jss.v082.i13
- Otten, L. J., & Rugg, M. D. (2001). Electrophysiological correlates of memory encoding are task-dependent. Cognitive Brain Research, 12(1), 11–18. https://doi.org/10.1016/S0926-6410(01)00015-5
- Perry, A. R., & Wingfield, A. (1994). Contextual encoding by young and elderly adults as revealed by cued and free recall. Aging, Neuropsychology, and Cognition, 1(2), 120–139. https://doi.org/10.1080/09289919408251454
- Pickering, M. J., & Gambi, C. (2018). Predicting while comprehending language: A theory and review. Psychological Bulletin, 144(10), 1002–1044. https://doi.org/10.1037/bul0000158
- Popov, V., & Reder, L. M. (2020). Frequency effects on memory: A resource-limited theory. Psychological Review, 127(1), 1–46. https://doi.org/10.1037/rev0000161
- Quante, L., Bölte, J., & Zwitserlood, P. (2018). Dissociating predictability, plausibility and possibility of sentence continuations in reading: Evidence from late-positivity ERPs. PeerJ, 6, e5717. https://doi.org/10.7717/peerj.5717
- R Development Core Team. (2018). R: A language and environment for statistical computing. Version 3.5.1; Feather Spray [Computer Software]. R Foundation for Statistical Computing.
- Ramscar, M., Dye, M., & McCauley, S. M. (2013). Error and expectation in language learning: The curious absence of mouses in adult speech. Language, 760–793. https://doi.org/10.1353/lan.2013.0068
- Ratcliff, R. (1993). Methods for dealing with reaction time outliers. Psychological Bulletin, 114(3), 510–532. http://doi.org/10.1037/0033-2909.114.3.510
- Rayner, K. (1998). Eye movements in reading and information processing: 20 years of research. Psychological Bulletin, 124(3), 372–422. https://doi.org/10.1037/0033-2909.124.3.372
- Rayner, K., Warren, T., Juhasz, B. J., & Liversedge, S. P. (2004). The effect of plausibility on eye movements in reading. Journal of Experimental Psychology: Learning, Memory, and Cognition, 30(6), 1290–1301. https://doi.org/10.1037/0278-7393.30.6.1290
- Reggev, N., Sharoni, R., & Maril, A. (2018). Distinctiveness benefits novelty (and not familiarity), but only up to a limit: The prior knowledge perspective. Cognitive Science, 42(1), 103–128. https://doi.org/10.1111/cogs.12498
- Riggs, K. M., Wingfield, A., & Tun, P. A. (1993). Passage difficulty, speech rate, and age differences in memory for spoken text: Speech recall and the complexity hypothesis. Experimental Aging Research, 19(2), 111–128. https://doi.org/10.1080/03610739308253926
- Rommers, J., & Federmeier, K. D. (2018a). Predictability's aftermath: Downstream consequences of word predictability as revealed by repetition effects. Cortex, 101, 16–30. https://doi.org/10.1016/j.cortex.2017.12.018
- Rommers, J., & Federmeier, K. D. (2018b). Lingering expectations: A pseudo-repetition effect for words previously expected but not presented. NeuroImage, 183, 263–272. https://doi.org/10.1016/j.neuroimage.2018.08.023
- Sanford, A. J., & Sturt, P. (2002). Depth of processing in language comprehension: Not noticing the evidence. Trends in Cognitive Sciences, 6(9), 382–386. https://doi.org/10.1016/S1364-6613(02)01958-7
- Schomaker, J., & Meeter, M. (2015). Short- and long-lasting consequences of novelty, deviance and surprise on brain and cognition. Neuroscience & Biobehavioral Reviews, 55, 268–279. https://doi.org/10.1016/j.neubiorev.2015.05.002
- Shing, Y. L., & Brod, G. (2016). Effects of prior knowledge on memory: Implications for education. Mind, Brain, and Education, 10(3), 153–161. https://doi.org/10.1111/mbe.12110
- Smith, N. J., & Levy, R. (2013). The effect of word predictability on reading time is logarithmic. Cognition, 128(3), 302–319. https://doi.org/10.1016/j.cognition.2013.02.013
- Snodgrass, J. G., & Corwin, J. (1988). Pragmatics of measuring recognition memory: Applications to dementia and amnesia. Journal of Experimental Psychology: General, 117(1), 34–50. https://doi.org/10.1037/0096-3445.117.1.34
- Staresina, B. P., Gray, J. C., & Davachi, L. (2009). Event congruency enhances episodic memory encoding through semantic elaboration and relational binding. Cerebral Cortex, 19(5), 1198–1207. https://doi.org/10.1093/cercor/bhn165
- Staub, A. (2015). The effect of lexical predictability on eye movements in reading: Critical review and theoretical interpretation. Language and Linguistics Compass, 9(8), 311–327. https://doi.org/10.1111/lnc3.12151
- Taylor, W. L. (1953). “Cloze procedure”: A new tool for measuring readability. Journalism Quarterly, 30(4), 415–433.
- Tomaschek, F., Hendrix, P., & Baayen, R. H. (2018). Strategies for addressing collinearity in multivariate linguistic data. Journal of Phonetics, 71, 249–267. https://doi.org/10.1016/j.wocn.2018.09.004
- Urbach, T. P., DeLong, K. A., Chan, W. H., & Kutas, M. (2020). An exploratory data analysis of word form prediction during word-by-word reading. Proceedings of the National Academy of Sciences, 117(34), 20483–20494. https://doi.org/10.1073/pnas.1922028117
- Van Berkum, J. J. (2010). The brain is a prediction machine that cares about good and bad – Any implications for neuropragmatics? Italian Journal of Linguistics, 22, 181–208. http://hdl.handle.net/11858/00-001M-0000-0012-C6B0-9
- Van Berkum, J. J., Brown, C. M., Zwitserlood, P., Kooijman, V., & Hagoort, P. (2005). Anticipating upcoming words in discourse: Evidence from ERPs and reading times. Journal of Experimental Psychology: Learning, Memory, and Cognition, 31(3), 443–467. https://doi.org/10.1037/0278-7393.31.3.443
- Van De Meerendonk, N., Kolk, H. H., Vissers, C. T. W., & Chwilla, D. J. (2010). Monitoring in language perception: Mild and strong conflicts elicit different ERP patterns. Journal of Cognitive Neuroscience, 22(1), 67–82. https://doi.org/10.1162/jocn.2008.21170
- Van Kesteren, M. T., Ruiter, D. J., Fernández, G., & Henson, R. N. (2012). How schema and novelty augment memory formation. Trends in Neurosciences, 35(4), 211–219. https://doi.org/10.1016/j.tins.2012.02.001
- Van Petten, C., & Luka, B. J. (2012). Prediction during language comprehension: Benefits, costs, and ERP components. International Journal of Psychophysiology, 83(2), 176–190. https://doi.org/10.1016/j.ijpsycho.2011.09.015
- Wagner, A. D., Schacter, D. L., Rotte, M., Koutstaal, W., Maril, A., Dale, A. M., Rosen, B. R., & Buckner, R. L. (1998). Building memories: Remembering and forgetting of verbal experiences as predicted by brain activity. Science, 281(5380), 1188–1191. https://doi.org/10.1126/science.281.5380.1188
- Warren, T., & McConnell, K. (2007). Investigating effects of selectional restriction violations and plausibility violation severity on eye-movements in reading. Psychonomic Bulletin & Review, 14(4), 770–775. https://doi.org/10.3758/BF03196835
- Wlotko, E. W., Federmeier, K. D., & Kutas, M. (2012). To predict or not to predict: Age-related differences in the use of sentential context. Psychology and Aging, 27(4), 975–988. http://doi.org/10.1037/a0029206