ABSTRACT
Perfetti (2007) proposed that the quality of lexical representations affects reading. We investigated the role of lexical quality in decoding. Eighty-four adults read aloud words and pseudowords with dense and sparse neighbourhoods in a masked form-priming experiment. Individual-difference measures of language and cognitive processes were collected and entered into a principal component analysis (PCA). Compared to a non-overlapping control prime, we observed greater facilitatory form-priming for word targets with sparse neighbourhoods than those with dense neighbourhoods. A PCA component related to orthographic precision affected form-priming: people with low orthographic precision showed greater facilitation for words with sparse neighbourhoods, primed by pseudowords, than those with dense neighbourhoods. People with high orthographic precision demonstrated the converse, only when primed by words. For pseudoword reading, word primes facilitated more than pseudoword primes in people with low orthographic precision. People with high orthographic precision showed the opposite pattern.
One of the main subcomponents in reading is recognising an orthographic input as a word. Visual word recognition is fast, efficient, and relatively effortless in most adults, suggesting that this aspect of word processing is largely similar across adults (e.g. LaBerge & Samuel, Citation1974). However, individual variation has been shown to affect each stage of visual word recognition, including letter-sound correspondence and access to the mental lexicon (e.g. Andrews & Hersch, Citation2010; Katz et al., Citation2012; see review by Perfetti, Citation2007), and not all neurotypical children become skilled readers (see review by Castles et al., Citation2018).
The Lexical Quality Hypothesis (LQH; Perfetti, Citation1992, Citation2007; Perfetti & Hart, Citation2001) asserts that individual differences in reading skill amongst adult skilled readers arise from differences in the individual quality of readers’ lexical representations. The quality of lexical representations is defined by two principles: precision and redundancy (Perfetti, Citation2007). Precision is the specificity and completeness of the information stored in the lexical representation, containing letter identity and letter position, as well as the sounds defining a specific word. Redundancy is the extent to which information in any one representation (e.g. orthography) of a word is predictable from another representation (e.g. phonology and/or semantics). Consistent and partially redundant patterns (e.g. strong grapheme-phoneme correspondences) produce coherent and synchronous activation between the different levels of representation to define a word’s identity (Ehri, Citation2005; Perfetti & Hart, Citation2002). Such redundancies create stronger links between the different levels of representation of a word so that, for example, if the orthographic form of the word is encountered, the phonological and semantic representations are immediately activated, and vice versa (Ehri, Citation2005; Perfetti & Hart, Citation2002). The development of a precise and redundant lexical representation gradually becomes stronger through reading experience (Share, Citation1995). As a consequence, skilled readers with well-defined lexical representations can more readily discriminate between a target word and its orthographically and phonologically similar neighbours (e.g. bane and bake; i.e. words that differ from the target word by one letter or sound; Andrews & Hersch, Citation2010; Coltheart et al., Citation1977). In turn, this enables fast word identification, that can operate in a more context-independent manner, supporting effective reading comprehension (Andrews, Citation2012, Citation2015; Perfetti & Hart, Citation2001). In contrast, less skilled readers possess lower-quality lexical representations in that they are less specified and integrated, with more variable bonds between representations. This makes the process of grapheme-phoneme conversion (i.e. decoding; Gough & Tumner, Citation1986; Katz et al., Citation2012) more laborious and effortful, leading to poor reading comprehension. When a target word is presented, less skilled readers are assumed to struggle to suppress its neighbours, and therefore take longer to recognise the target.
Most of the studies that have investigated individual differences in visual word recognition have used a lexical decision task (LDT; i.e. word/nonword decision in response to a letter string) and concluded that orthographic (e.g. Andrews & Hersch, Citation2010; Andrews & Lo, Citation2012; Meade et al., Citation2018) or phonological (e.g. Elsherif et al., Citation2022) precision contribute to the development of skilled reading. However, word recognition processes are influenced by the nature of the task used. For example, there is evidence that the LDT and visual word naming may measure different stages of visual word recognition – more precisely that LDT taps into later stages of processing, while visual word naming taps into earlier stages (e.g. Schilling et al., Citation1998). A number of studies showed that lexical and semantic predictors contribute more to reaction times in the LDT than in visual word naming, whereas the converse holds for orthographic, phonological and articulatory predictors (Brysbaert & Cortese, Citation2010; Cortese & Khanna, Citation2007; Ferrand et al., Citation2011). For example, word length contributes more to visual word naming than LDT, while imageability has been found to contribute more to LDT than visual word naming (Cortese et al., Citation2018). In addition, word frequency accounts for 40% of the variance in the response latencies in visual LDT, while the predictor of initial phoneme onset describes 2% of the variance of LDT performance (Brysbaert & Cortese, Citation2010; Cortese & Khanna, Citation2007; Ferrand et al., Citation2011). In contrast, initial phoneme onset accounts for 40% of the variance for word naming, while word frequency describes less than 10% of the variance (Cortese & Khanna, Citation2007; Ferrand et al., Citation2011). The aim of the current study is to investigate individual differences in the early stages of word recognition, and we therefore employed a visual word naming paradigm. Here, we report two experiments using word and pseudoword (i.e. pronouncable nonwords) naming that are identical to the LDT previously reported in Elsherif et al. (Citation2022, described in more detail below), apart from the task being changed to naming.
Similar to many studies of visual word recognition, we employed the masked form-priming paradigm (e.g. Davis & Lupker, Citation2006; Rastle & Brysbaert, Citation2006). Critically there is also evidence that the masked form-priming of lexical decision and naming yields differing effects. Phonological priming is not always observed in the LDT but is consistently demonstrated in visual word naming studies (see review by Rastle & Brysbaert, Citation2006). Interestingly, masked form-priming in visual word naming usually has a facilitatory effect on response times, with orthographic/phonological overlap between primes and targets speeding spoken word onset. In contast, in the LDT, inhibitory, facilitatory or no priming effects of form overlap have been observed (Rastle & Brysbaert, Citation2006).
Masked form-priming in LDT is known to be modulated by prime and target lexicality (word or pseudoword): Latencies to word targets are inhibited by form related word primes but facilitated by pseudoword primes (e.g. Davis & Lupker, Citation2006). This pattern is explained in terms of facilitation due to orthographic overlap between pseudowords and targets and inhibition due to lexical competition between word primes and targets (Davis & Lupker, Citation2006; Forster & Davis, Citation1991; Forster & Veres, Citation1998). The effect of prime-target lexicality has received less scrutiny in visual word naming experiments. However, two studies have compared the effects of word and pseudoword form-primes on visual word naming. A Spanish masked priming study presented word targets such as CURVA (curve) preceded by onset related (e.g. campo) and unrelated (e.g. fondo) word primes and related (e.g. coslo) and unrelated (e.g. foszi) pseudoword primes (Dimitropoulou et al., Citation2010). Naming latencies were faster for target words preceded by related word and pseudoword primes compared to matched controls. The observed facilitation was attributed to speech planning processes. In another naming study conducted in English, Mousikou et al. (Citation2015) used pseudoword targets (e.g. BLUP) preceded by related onset (e.g. bist) and unrelated (e.g. trin) pseudowords and observed facilitatory priming for pseudoword targets. Mousikou et al. proposed that the facilitatory priming effect arose due to overlap in grapheme-phoneme conversion. On presentation of the prime, the reader computes the phonology of the prime based on grapheme-phoneme correspondence rules. This pre-activates the processing of related targets (following Forster & Davis, Citation1991), leading to facilitatory priming in naming. Mousikou et al. also tested word targets (e.g. BANISH) preceded by related word onset primes (e.g. beetle), and pseudoword primes (e.g. bellap). Compared to matched unrelated words and pseudowords, facilitatory priming was observed following both prime types but the facilitation was larger following pseudoword than word primes. They proposed that lexical competition occurs between word prime and word targets, thus reducing facilitatory priming, while pseudoword primes, which lack a lexical representation, do not compete.
Interestingly, both of the above studies failed to show the inhibitory effect of word primes that has been observed in the LDT, although lexical competition was proposed to account for the difference between word and pseudoword primes in the Mousikou et al. (Citation2015). However, neither study used neighbour primes or manipulated neighbourhood density, and the overlap was restricted to the onset. This makes it difficult to identify the nature, and the size, of lexical competition effects. Form-priming effects in LDT are known to be modulated by NHD, such that word targets from dense neighbourhoods (i.e. many neighbours; e.g. BEAD has BEAR, BEAT, READ, DEAD) tend to show inhibitory priming effects while those from sparse neighbourhoods (i.e. few neighbours; e.g. VEIN has only VEIL and REIN) tend to show facilitation (Davis & Lupker Citation2006). To our knowledge, the only study that has assessed the relationship between NHD and form-priming in visual word naming, using related neighbour primes, yielded the opposite pattern. Forster and Davis (Citation1991) tested participants in a masked form-priming paradigm and asked them to name the target words. In the experimental condition, participants named word targets from either dense or sparse neighbourhoods, which were always preceded by a pseudoword prime (e.g. gord-GOLD), whereas in the control condition the target always followed a word prime (e.g. soil-GOLD). The naming latencies in the experimental condition were subtracted from the naming latencies in the control condition to determine a priming effect. A larger facilitatory priming effect was observed for word targets from dense neighbourhoods than for those from sparse neighbourhoods, which is the reverse of what is found in visual LDT (e.g. Andrews & Hersch, Citation2010; Davis & Lupker, Citation2006; Elsherif et al., Citation2022). They argued that the facilitation was due to shared words onsets aiding the preparation of the spoken response (with more onset overlap in dense neighbourhoods). When the word primes also shared onset consonants (e.g. goat-GOLD, Experiment 2) the effect of NHD observed was more similar to LDT with no facilitation for words targets with dense neighbourhoods but significant facilitation for targets from sparse neigbourhoods.
However, a direct comparison of related and unrelated conditions in this study is problematic as prime lexicality differed. As discussed above, pseudoword primes have been shown to produce more facilitation than word primes (e.g. Mousikou et al., Citation2015) and to our knowledge no study of visual word naming has manipulated both prime-target lexicality and NHD. As reviewed above, both of these factors arguably affect the interplay between the faciliatory effects of form overlap and the resulting inhibitory effects of lexical competition. One of our aims was therefore to disentangle the effects of NHD, prime lexicality and form relatedness in a task that taps into the early stages of word recognition.
The current study used the same materials as Elsherif et al. (Citation2022) to investigate visual word naming. Elsherif et al. (Citation2022) report a masked form-priming study of LDT in which we manipulated prime-target lexicality and NHD. As the focus of this study was on lexical competition, word primes were higher in frequency than words targets to maximise the primes’ ability to suppress neighbours. Previous studies have shown that latencies to word targets are inhibited by high-frequency word primes but can facilitated by low-frequency word primes (e.g. Segui & Grainger, Citation1990). Consistent with previous LDT findings reviewed above (e.g. Davis & Lupker, Citation2006), form-priming of word targets was inhibitory when the primes were also words but facilitatory when the primes were pseudowords. Elsherif et al. (Citation2022) also included a large suite of individual differences to examine their contribution to competition resolution in LDT. We found that the inhibitory form-priming was modulated by a component relating to phonological precision. These results suggest that the component of phonological precision is linked to lexical competition for word recognition (but see Andrews and Hersch, Citation2010; Andrews & Lo, Citation2012, who showed similar effects but for individual differences in spelling). However, little is known about individual differences in reading skill relating to the earlier stages of visual word recognition. As argued above, evidence of lexical competition is indicative of access to the mental lexicon, while facilitatory form-priming reflects orthographic and/or phonological overlap between the prime and target. Access to orthographic representations necessarily occurs prior to access to the mental lexicon, and there is evidence that phonological representations are also accessed in the early stages of word recognition (e.g. Rastle & Brysbaert, Citation2006; Wheat et al., Citation2010). The process of reading aloud also entails grapheme-phoneme conversion (e.g. Cortese & Khanna, Citation2007). Therefore the skills emphasised by the visual word naming task may be different to those for the LDT. An additional aim of the present study was to determine the role of individual differences in aspects of reading skills to visual word naming.
According to the self-teaching theory (Share, Citation1995), learning and applying the mapping of graphemes to phonemes must be fluent before detailed orthographic representations are formed, which can then be used in word recognition. This requires increased reading experience, which improves word reading abilities. It is possible that different word recognition strategies are used by readers with different levels of reading experience. For example, Martens and De Jong (Citation2008) assessed the influence of repeated word and pseudoword reading on direct and indirect word reading regarding word length. The length effect was seen as an index of sublexical letter-sound conversion. They argued that the disappearance of the length effect after repeated word reading would indicate a shift from a letter-sound correspondence to a whole word route. The authors found that after 16 repeated word readings, the length effect disappeared, in average and good readers, but persisted for the poor readers in fourth and fifth grades. The authors concluded that poor readers depend on the letter-sound correspondence route for reading for longer than average readers and good readers. In addition, Adelman et al. (Citation2014) asked 100 17-to-55 year old participants to read 592 monosyllabic words aloud. They found that word targets with dense neighbourhoods were named more quickly than those with sparse neighbourhoods. In addition, naming latencies were shorter for high-frequency words than low-frequency words. These effects became smaller with increasing age. They concluded that older participants have more reading experience and a larger vocabulary, and therefore possess high-quality lexical representations (it should be noted though that vocabulary size and print exposure were not independently assessed). Taken together, these studies support the notion that the development of a precise lexical representation depends on reading experience and vocabulary, which makes phonological decoding fluent and results in more stable orthographic representations.
In sum, the current study extends our previous work (Elsherif et al., Citation2022) to visual word naming and investigates which aspects of reading ability affect the magnitude of priming in naming. We manipulated NHD and prime-target lexicality on masked form-priming in a visual word naming task. We predict that the effects of form-priming will be facilitatory overall, as visual word naming taps into the early stages of visual word recognition, which involves the preactivation of the target by overlapping segments of the prime (Rastle & Brysbaert, Citation2006). The effect of NHD observed will be dependent on the extent to which lexical competition affects naming performance. According to Forster and Davis (Citation1991), facilitatory form-priming should be larger for word targets with dense neighbourhoods than sparse neighbourhoods, as the former possess more shared phonological segments between the prime and target, thus facilitating spoken word production. In contrast, the findings of Mousikou et al. (Citation2015) suggest that weak effects of lexical competition can modulate form-priming in visual word recognition. In this case, facilitatory form-priming should be reduced for word targets with dense neighbourhoods compared to sparse neighbourhoods due to increased lexical competition. In order to further assess the effects of sublexical overlap, we include pseudoword primes and targets, as they have no lexical representations (but will of course activate related lexical representations). Pseudword stimuli should therefore demonstrate effects of sublexical overlap, but not effects of lexical competition.
Finally, we investigate the effect of individual differences in components of reading skill. We use a suite of individual difference measures to assess which component of phonology, orthography, and/or semantics modulate lexical retrieval. However, given that more skilled readers are better at reading than less skilled readers, as measured by print exposure, vocabulary size, reading comprehension, and phonological processing (e.g. Acheson et al., Citation2008; Burt & Fury, Citation2000; review by Huettig et al., Citation2018; Martin-Chang & Gould, Citation2008), it becomes more difficult to assess which components of reading (e.g. orthography, phonology and semantics) modulates lexical retrieval, as these individual differences measures are likely to be collinear. We therefore use a Principal Component Analysis (PCA) to group together the individual difference tests. As the same participants were tested in this study, we use the same components produced by the PCA from Elsherif et al. (Citation2022), which are: phonological precision, orthographic precision, and semantic coherence. In the LDT we found that phonological precision modulated form-priming effects. However if visual word naming taps into earlier processes in word recognition it is possible that orthographic precision could play a greater role in this task as orthographic processes precede access to phonology in reading (e.g. Grainger et al. Citation2006).
The effects of individual differences in reading skill on visual word naming are more difficult to predict as no previous studies have examined this relationship. In the LDT we observed that for readers with greater phonological precision, the direction of priming for word targets with sparse neighbourhoods was facilitatory, while the direction for those with dense neighbourhoods was inhibitory. In contrast, people with low phonological precision showed a non-significant pattern in the opposite direction. This pattern is consistent with the proposal that greater phonological precision results in the fast and effective inhibition of lexical competitors (Andrews & Hersch, Citation2010; Andrews & Lo, Citation2012: Elsherif et al. Citation2022). However as reviewed above, the extent to which lexical competition contributes to visual word naming latencies is a matter of debate. It is possibile that more skilled readers again show evidence of rapid inhibition of competitors, leading to reduced facilitation of words from dense neighbourhoods, whereas naming in less-skilled readers will be supported by the activation of larger neighbourhoods. Pseudoword processing should provide a measure of the benefits of form overlap independent of lexical competition. Alternatively, following Forster and Davis (Citation1991), lexical competition may not play a role in visual word naming. Instead, more skilled readers may be better able to benefit from form overlap than less skilled readers, due to faster grapheme-to-phoneme conversion processes which facilitate spoken word production.
Method
Participants
We aimed to detect a previously revealed effect size (i.e. the main effect of relatedness) from Forster and Davis’ (Citation1991) study. A post-hoc power analysis, using G*Power 3.1.9.4. (Faul et al., Citation2009), indicated that our sample size exceeded the number required to reach the desired level of power of 0.95 (minimum of 15 participants recommended, while we included the data from 84 participants in the analyses; see the Supplementary Material for the parameters used in the power analysis).Footnote1 Ninety-one monolingual British undergraduate students with normal or corrected-to-normal vision participated in the current study. All participants had participated in Elsherif et al. (Citation2021, Citation2022) and signed a consent form. Seven participants withdrew from the study and the data from a further two participants were removed because they performed below 2SD in individual difference measures that assessed phonology, reading fluency and spelling, a level of performance which may be indicative of dyslexia.Footnote2 The remaining 84 undergraduate students (77 females and 7 left-handers) aged 19–23 years (M = 20.18 ± 1.04 years) from the University of Birmingham, participated in the study for course credits. All participants were British English speakers, monolingual and had a similar level of education. The experiment was conducted in accordance with British Psychological Society ethical guidelines and was approved by the University of Birmingham’s ethical committee (ERN_15-1236).
Tests
General procedure for the tests. Each participant completed all components of the study over three sessions. Each session lasted approximately an hour (See for an overview of the study). All participants completed the tests in the same order. Participants were assessed on several measures of orthography, phonology, reading fluency, semantics, non-verbal intelligence and inhibitory control,Footnote3 which are described in detail in Elsherif et al. (Citation2021; see ). To provide a broad assessment of lexical quality, the tests selected included three measures of orthographic processing: spelling production, author recognition test and title recognition test; three tests of semantic processing: expressive vocabulary, receptive vocabulary and a passage comprehension test; four reading fluency tests: test of regular word reading, irregular word reading, pseudoword reading and rapid letter naming; and finally, four tests of phonological processing: phoneme elision, phoneme reversal, nonword repetition and memory for digits (see supplementary materials for more details). They were also given a demographic questionnaire that included questions of age, gender and handedness (see supplementary Material).
Figure 1. An overview of the three experimental sessions. From “Phonological precision for word recognition in skilled readers,” by M.M.Elsherif, L.R.Wheeldon, and S.Frisson, 2022, Quarterly Journal of Experimental Psychology, 75(6), p. 1025. CC BY-NC-ND.
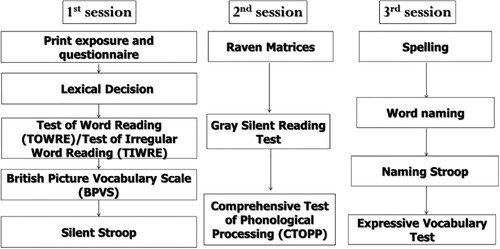
Table 1. The individual difference measures used in the current experiment and their groupings. From “Phonological precision for word recognition in skilled readers,” by M.M.Elsherif, L.R.Wheeldon, and S.Frisson, 2022, Quarterly Journal of Experimental Psychology, 75(6), p. 1025. CC BY-NC-ND.
Materials for masked priming
The stimuli are described in detail in Elsherif et al. (Citation2022).
Word target set. The CELEX database (Baayen et al., Citation1995), using Davis’s (Citation2005) N-Watch, was used for item selection and to obtain all frequency and neighbourhood values for the 80 monosyllabic word targets. The 40 words with a sparse neighbourhood had on average 5.3 orthographic neighbours (SD = 1.90; range = 2-8), 9.3 phonological neighbours (SD = 2.92; range = 8-15), whereas the 40 words with dense neighbourhoods had on average 13.0 orthographic neighbours (ON; SD = 2.05, range = 10-18) and 23.2 phonological neighbours (PN; SD = 5.90, range = 14-36). The low NHD had 3.5 phonographic neighbours (PgN; SD = 1.76, range = 1-8), while the high NHD had 7.03 phonographic neighbours (SD = 2.78, range = 2-12). Both sets differed significantly from each other (ON: t(78) 17.72, p < .001, d = 4.01;PN: t(78) 15.26, p < .001, d = 3.46; PgN: t(78) = 6.64, p < .001, d = 1.50). In addition, we used CLEARPOND (Marian et al., Citation2012) to calculate neighbourhood frequency between dense and sparse NHD and did not observe a significant difference between dense (M = 8.35, SD = 8.05) and sparse NHD (M = 6.18, SD = 13.85) in terms of neighbourhood frequency (t < 1). The word targets were matched between groups (dense vs. sparse) in terms of word length (number of letters) and word frequency. Statistical tests showed that the groups did not differ significantly on these measures (word frequency t < 1; log frequency; t (78) 1.84, p = .07, d = 0.42; SUBTLEX-UK frequency (van Heuven et al., Citation2014) t(78) 1.91, p = .06, d = 0.43; word length: number of graphemes; t < 1).Footnote4 The high and low NHD target sets differed in length on average by less than one phoneme, however, this difference was significant (t(78) 9.35, p < .001, d = 2.11).Footnote5 See for descriptives. See Appendix A1 for word targets.
Table 2. Descriptive statistics for word target characteristics.
Pseudoword target set. For the purpose of pseudoword naming, 80 nonwords were created that matched the word targets in word length (t < 1) and orthographic NHD (dense NHD: t(78) 1.22, p = .23, d = 0.28; sparse NHD: t(78) 1.67, p = .10, d = 0.38). The targets were divided into two equal sets differing in orthographic NHD. The high NHD pseudoword set had eight or above orthographic neighbours, while the low NHD pseudoword set had between two and seven orthographic neighbours. Both sets were significantly different (t(78) 12.31, p < .001, d = 2.8). See for descriptives. All pseudowords conformed to the English spelling rules and were pronounceable using the grapheme-phoneme conversion rules. See Appendix A2 for pseudoword targets.
Table 3. Descriptive statistics for pseudoword target characteristics.
Prime set. All primes were monosyllabic and shared the same number of letters as their targets. The primes were differentiated by word and pseudoword primes (see and ). The related primes were re-ordered for each NHD set with an additional criterion of no orthographic overlap (i.e. no letter in the same position) between prime and target (e.g. vire-PEEK/ ploq-FUNK) to create the unrelated word primes. The related and unrelated prime conditions did not differ from each other in terms of word frequency, word length, number of phonemes, orthographic and phonological NHD (all ts < 1).
Target words had a lower word frequency than their related and unrelated word primes (all ps < .001). However, the prime and target did not differ in measures of orthographic, phonological and phonographic NHD (all ts < 1) and word length (t < 1). The word prime sets for the dense and sparse NHD did not significantly differ in terms of word frequency (word and pseudoword target: t < 1). Within each NHD set, the number of phonemes did not differ significantly between prime and target (dense and sparse NHD: t < 1). However, even though the number of phonemes for the dense and sparse NHD prime sets only differed on average by less than 1/10th, this difference was significant (t(78) 7.1, p < .001, d = 1.61).
Design of the masked priming experiment.
The masked priming experiment design involved an orthogonal manipulation of a 2 (prime lexicality: word versus pseudoword) x 2 (NHD: dense versus sparse) x 2 (related versus unrelated) nested within-subject design for each between-item factor. Two lists were created such that each item occurred twice in each list in related and unrelated conditions but in a different prime lexicality in each list. Assignment of targets was counterbalanced across lists such that half of the targets in each list were primed by words and half by nonwords. Each list was then divided into 4 sections of 40 targets with equal numbers of items per condition and no repetition of target items. All targets were separated by an intervening section. The order of presentation of these sections was rotated across participants who saw one list only.
Procedure
Masked priming. Participants were informed that they would be presented with an existing or novel word. Participants were instructed to read it aloud as fast as possible without compromising accuracy into a microphone connected to a Sony DAT recorder (PCM-M1) for future offline analysis of the naming data. E-prime (E-Prime 2.0) software was used to create the experiment and collect the responses. All stimuli were written in Arial font size 34. No mention was made of the primes. No feedback was provided.
A trial of the masked priming task had the following sequence: a forward mask (#####) was presented for 500 ms, which was followed by a prime stimulus in lower case for 50 ms and finally, the target stimulus in upper case for 1500 ms. Participants had to respond within 1500 ms. Following the participant’s response, there was an inter-trial interval of 1500 ms. Participants first completed 10 practice trials with a similar structure to the experimental trials. The experiment started after the practice trials. After every 80 trials, participants had a short break. The naming task was presented a approximately four months after the LDT. Participants who were given List 1 in the LDT, were presented with List 2 in the visual word naming task, and vice versa.
Results
Cognitive and language tests
Our participants were homogeneous in their demographics. All 84 participants were monolingual English speakers. All participants had a similar level of educational experience (i.e. 83 undergraduate students and 1 graduate student). Results from the individual difference measures can be found in .
Table 4. Means and standard deviation of all measures.
Correlation
Following Elsherif et al. (Citation2022), we contructed robust composíte measures of different aspects of reading ability by averaging the standard scores for the specific measures which assessed a similar process. A z-transformation was conducted on the scores for the suite of individual difference measures to standardise the units in order to compare these measures. A Pearson’s correlation was computed to evaluate the correlation between these measures in the entire sample. The factors going into the composite measures were highly collinear, which, if entered separately, can cause the PCA to be unstable and produce biased estimates (Field, Citation2009). The composite measures were formed by averaging the standard scores for the relevant measures.
A composite measure of vocabulary (ZVocab) was formed by averaging the standard scores of the vocabulary measures (i.e. BPVS and EVT, which were strongly correlated: r = .51) to provide a more comprehensive measure of vocabulary ability. To form a composite measure of phonological working memory, ZMemory, the two highly correlated measures of phonological working memory (i.e. nonword repetition and memory for digits; r = 0.43) were combined. In addition, we included three highly correlated measures of reading fluency (TOWRE word reading and Rapid Letter Naming; r = .47, TOWRE phonemic decoding and Rapid Letter Naming; r = .56 and TOWRE word reading and phonemic decoding; r = .56) as one averaged measure to offer a detailed assessment of reading fluency, ZReadingFluency. Finally, two strongly related measures of print exposure (Author Recognition Test and Title Recognition Test; r = .77) were aggregated to create a measure of print exposure, ZPrintexposure. summarises the correlations between the composite standard scores with the other individual difference measures. The correlations reflect relationships shown in previous studies, including the relationship between print exposure and reading comprehension (e.g. Acheson et al., Citation2008). Importantly, the collinearity between these individual difference measures is relatively high (rs ≥ .3), thus it is appropriate to use a multi-variate approach such as PCA. A PCA was therefore computed to isolate common constructs between the remaining measures and its composite variables (see Andrews & Lo, Citation2012; Elsherif et al., Citation2022; Holmes et al., Citation2014).
Table 5. Correlations between tasks.
Principal component analysis
We conducted the PCA analysis to determine statistical clustering of the individual difference measures. This analysis was conducted with the GPA rotation package (Bernaards & Jenrich, Citation2005) in the R statistical programming open code software (R Development Core Team, Citation2017). The data from was placed into a PCA with CTOPP phoneme elision being dropped, as it correlated less than .3 with any other variable. The Kaiser–Meyer–Olkin measure of sampling adequacy was .68, above the commonly recommended value of .50 (Field, Citation2009). The Bartlett’s test of sphericity was significant (χ2 (28) 113.47, p < .001). This showed the correlations between the remaining eight variables were appropriate for PCA.
We calculated both varimax and oblique rotations. presents the results of the varimax analysis. The analysis produced three components in the current study. The eigenvalues were greater than Kaiser’s criterion of 1, explaining a total of 62% of variance in reading behaviours. Irrespective of rotation, component loadings were similar (except that the variable Zvocab was only noted in varimax rotation). The highest inter-component correlation produced by an oblique rotation was .26 between the second and third components. A vari-max rotation was used, as the components did not corelate with each other above .32 (Tabachnick et al., Citation2007). Only variables with loadings of higher than 0.45 were considered. Based on the loadings, these three components were assigned construct names indicative of their component variables and are listed in the order of variance explained in . Components show positive or negative loadings. Positive loadings give inclusionary criteria and describe the underlying construct of the component. Negative loadings provide exclusionary criteria and show an inverse relationship to the construct of the component.
Table 6. Components produced by the PCA.
The first component, describing most of the variance, was a composite including a composite measure of phonological working memory and a composite measure of reading fluency, phoneme reversal and TIWRE (all positive components). These positive loadings indicate that higher phonological working memory and phonological awareness might benefit from redundant word-specific phonology and context sensitive grapheme-phoneme phonology, leading to more efficient access to the phonological representation (Perfetti, Citation2007). This component was argued to be analogous to a measure of phonological precision.
The second component included a composite measure of print exposure, a composite measure of vocabulary and spelling, thus was labelled as a general measure of orthographic precision. The positive loadings of the recognition test variables, along with spelling and vocabulary, indicate that the larger vocabulary size might benefit from stronger and richer resonance between the sublexical representations, allowing more efficient bottom-up processing, which is consistent with the term of orthographic precision (Perfetti, Citation2007), in spite of the vocabulary measures not being orthographic. Put simply, orthographic precision is the connection between orthography or form to higher-level semantic knowledge.
Finally, the third component involved Gray Silent Reading Comprehension and a composite measure of vocabulary, together with a composite score of reading fluency, which was negatively related to reading comprehension and vocabulary. The lower the reading fluency scores, the higher the scores for vocabulary and reading comprehension. This could be therefore interpreted as an index of the semantic coherence facet of the Lexical Quality Hypothesis (LQH). The negative loading of ZReadingFluency is surprising, as reading fluency contributes to reading comprehension in younger readers (Silverman et al., Citation2013). However, this contribution weakens with increasing age (Language and Reading Research Consortium, Citation2015). In addition, Jackson (Citation2005) found that the measures that form our ZReadingFluency composite did not to correlate with text comprehension. Most importantly, ZReadingFluency plays a small role on the semantic coherence component and this component does not interact with any of the naming results.
General linear mixed effect model (GLMM)
Statistical analyses were conducted using general linear mixed effects models with the statistical package R (R Core Team, Citation2017) with the lme4 package (Bates et al., Citation2010). These analyses were conducted on the naming latencies for word and pseudoword targets. The naming latencies were log-transformed. The GLMM models included three fixed effects: NHD (sum coded with sparse neighbourhood as intercept), relatedness (sum coded with unrelated as intercept) and prime lexicality (sum coded with pseudoword prime as intercept). In addition, the three components from the PCA were included into the model as fixed effects and analysed as a continuous variable. The components were centred. If any interactions between the components from the PCA and any other fixed effects arose, then the continuous PCA data were logged as binary variables (high vs. low). The recoding was done by splitting the data from a variable into two sets so that the number of data points per set was as closely matched as possible. In all cases, the maximal random structure model included the interactions of all three conditions with both subjects and items (Barr et al., Citation2013). A fully random model was used whenever possible. If convergence did not ensue, the random structure was simplified by removing interactions before main effects in the order of least variance explained until model convergence was reached (Veldre & Andrews, Citation2014).
We started with a full model, and performed a step-wise reduction procedure (using the drop1 function in R) to remove fixed effects and to locate the minimal model using Bayesian Information Criterion (BIC) to find the lowest BIC, indicating better goodness of fit (Schwarz, Citation1978). The difference between the full model and reduced model formed ΔBIC; a positive ΔBIC indicates that the reduced model is better than the null model. In addition, Using the formula (exp(ΔBIC/2); Raftery, Citation1995), wecalculated approximate Bayes factor (BF) to compare the relative evidence between the full model and reduced models. For instance, a BF value of 10 suggests that the reduced model is 10 times more likely than the full model to occur. These measures were used to create a minimal model and provide the best fit for our data. In general, the higher both ΔBIC and BF, the more likely the reduced model can explain the data in comparison to the full model. We used an absolute t value greater than 2.00 to suggest that the variable was significant at the α = .05 level (Baayen et al., Citation2008). When interactions were observed, each fixed effect was logged as a binary variable and p values were calculated using the afex package (Singmann et al., Citation2020). Finally, effect sizes were calculated using Cohen’s d = ΔM/ σ for the within-group comparisons. These were computed with estimated marginal means (for calculation of ΔM) and total variance from the covariance model estimates (for standardisation of σ; Cohen, Citation1988; see review by Westfall et al., Citation2014, for calculation).
Word targets
The naming latencies data for each participant in each condition was subjected to a ± 2.5 standard deviation trim. Any naming latencies below 200 ms were also removed. Only RTs for correct responses were used in the analyses. One word item, “BASS”, produced more than 50% of errors and was removed from the analyses, leaving 39 target words per condition. In total, 6.18% of the data was removed prior to analyses. Average RTs, SDs and the proportion of correct responses for each condition, are shown in .
Table 7. Mean response times and proportion correct for each prime lexicality, relatedness and NHD condition for word naming.
Accuracy was high for all conditions, which was also the case for the pseudoword targets. Since the models for accuracy did not converge, we will not discuss accuracy further (see supplementary materials).
For reaction times, the general pattern was facilitatory priming effects for word targets, with smaller effects for words with dense neighbourhoods than those with sparse neighbourhoods.
The model for the word naming task did not converge until the item-slope was removed, leaving only a random item-intercept, and the three-way interaction was reduced to NHD and relatedness as individual factors by themselves in the random structure of the subject (see Appendix B for the final model code). The minimal model with the same random structure is shown in .
Table 8. The minimal model output for RTs in the word naming task.
Although the reduced model was not significantly different from the full model (Full model: AIC: −8498.0, BIC: −7939.9; reduced model = AIC; −8533.7, BIC: −8347.7, p = .08, ΔBIC: 407.79, Approx. BF > 10,000), the reduced model produced an approximate Bayes factor above 10,000 and a higher ΔBIC value than the full model, suggesting that the removal of these variables improved the model fit and that the reduced model is more likely to occur at least more than 10000 times than the full model. The final model is therefore based on the reduced model. In the reduced model, there was a four-way interaction between orthographic precision, neighbourhood density, prime lexicality and relatedness (). For people with high orthographic precision, word targets with dense neighbourhoods showed less facilitation from prime words than those with sparse neighbourhoods. A similar pattern occurred for pseudoword primes, although the facilitation was less than for word primes overall. For people with low orthographic precision, word targets with dense neighbourhoods showed more facilitation than targets with sparse neighbourhoods for word primes. The opposite pattern occurred for pseudoword primes.
Figure 2. Reaction time (RT) priming effects (in ms) for high- and low-N targets preceded by word and pseudoword primes and separated by the orthographic precision component. Positive priming effects reflect facilitation for targets preceded by related primes, relative to unrelated primes. Error bars represent 95% confidence interval for each condition.
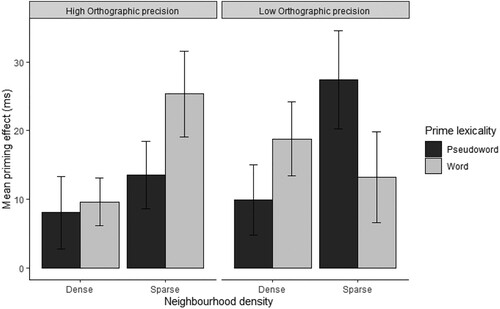
The full model was split into two sub-models: high orthographic precision and low orthographic precision models. Orthographic precision was removed from the equation and the same procedures for the analyses and random structure from the full model were applied to the sub-models. In the high orthographic precision model, there was an interaction of NHD, prime lexicality and relatedness that approached significance (b = 0.028, t = 1.76, p = .08). As can be seen in , the effect of prime lexicality is greater for word targets with sparse neighbourhoods than for dense neighbourhoods. We therefore split the high orthographic precision model split into two sub-models: word primes and pseudoword primes. Prime lexicality was removed from the equation and the same procedures for the analyses and random structure from the full model were applied to the sub-models. For word targets preceded by word primes, there was an interaction of relatedness and NHD (b = 0.04, t = 3.14, p = .002). In word targets following pseudoword primes, there was no significant interaction (b = .008, t = .69, p = .50), nor a significant effect of NHD (b = −0.012, t = −1.22, p = .23), though there was a significant effect of relatedness (b = 0.022, t = 2.50, p = .01).
For the sub-model of people with low orthographic precision, an interaction between NHD, prime lexicality and relatedness was found (b = −0.038, t = −2.03, p = .042). We therefore split the low orthographic precision model into two sub-models: word primes and pseudoword primes. Prime lexicality was removed from the equation and the same procedures for the analyses and random structure from the full model were applied to the sub-models. For word targets preceded by a word prime, there was no significant interaction (b = .006, t = .50, p = .62) and no significant effect of NHD (b = −0.001, t = −0.92, p = .93), but there was a significant effect of relatedness (b = 0.040, t = 3.95, p < .001). In word targets following pseudoword primes, there was an interaction of relatedness and NHD (b = 0.03, t = 2.40, p = .02).
With regard to the individual components, the model output showed that there was a significant effect of phonological precision on log RT: the higher the value of the of phonological precision component, the shorter the reaction times.
Pseudoword targets
The reaction times (RTs) were trimmed in the same way as for word targets, leading to 6.38% of the data being removed in total. Average RTs, SDs, and the proportion correct responses for each condition, are shown in . For reaction times, the priming effects for the pseudoword targets were all facilitatory. The priming effects were smaller for pseudowords with dense neighbourhoods than those with sparse neighbourhoods.
Table 9. Mean response times and proportion correct for each prime lexicality, relatedness and NHD condition for pseudoword naming.
The model for the pseudoword naming task did not converge until the item-slope was removed, leaving only a random item-intercept, and the three-way interaction was reduced to NHD and relatedness as individual components by themselves in the random structure of the subject (see Appendix B for the final model code). The minimal model with the same random structure is shown in .
Table 10. The minimal model output for RTs in the pseudoword naming task.
Although the reduced model was not significantly different from the full model (Full model AIC: –6972.1, BIC: −6414.1; reduced model = AIC: −7019.8, BIC: −6871.0, p = .23, ΔBIC: 456.85, Approx. BF > 10,000), the reduced model produced an approximate Bayes factor above 10,000 and a higher ΔBIC values than the full model, indicating that removing these variables improved the fit of the model, with the reduced model being more likely to occur than the full model. In the reduced model (see ), there was a significant three-way interaction between orthographic precision, relatedness and prime lexicality (see ). In people with high orthographic precision the facilitation for pseudoword targets preceded by word primes was larger than that following pseudoword primes. The opposite pattern emerged for people with low orthographic precision.
Figure 3. Reaction time (RT) priming effects (in ms) for pseudword targets preceded by word and pseudoword primes and separated by the orthographic precision component. Positive priming effects reflect facilitation for targets preceded by related primes, relative to unrelated primes. Error bars represent 95% confidence interval for each condition.
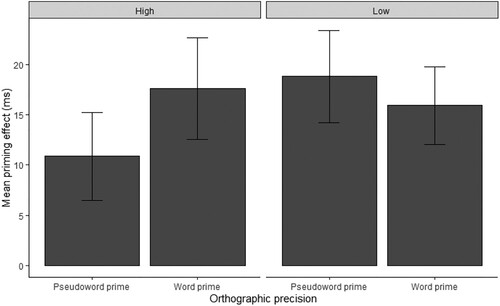
The full model was split into two sub-models: high orthographic precision and low orthographic precision models. Orthographic precision was removed from the equation and the same procedures for the analyses and random structure from the full model were applied to the sub-models. In the high orthographic precision model, there was no interaction of prime lexicality and relatedness (b = 0.011, t = 1.4, p = .17), and no main effect of prime lexicality (b = −0.002, t = −0.40, p = .72). There was a main effect of relatedness (b = 0.022, t = 3.5, p < .001). For the participants with low orthographic precision, no interaction was observed between prime lexicality and relatedness (b = −0.012, t = - 1.19, p = .23), and the effect of prime lexicality was also not significant (b = 0.0048, t = 0.69, p = .49). However, there was an effect of relatedness (b = 0.035, t = 4.79, p < .001).
The full model also produced a significant interaction of phonological precision with relatedness. Participants with high phonological precision produced larger facilitatory priming (i.e. 15 ms) than those with low phonological precision (i.e. 11 ms). The full model was split into two sub-models: high phonological precision and low phonological precision. Phonological precision was removed from the equation and the same procedures for the analyses from the full model were applied to the sub-models. A significant effect of relatedness was shown for both the high phonological precision model (b = 0.04, t = 7.85, p < .001, d = 0.17) and the low phonological precision model (b = 0.02, t = 3.96, p < .001, d = 0.09).
The full model also produced a significant interaction of NHD with relatedness (). As can be seen in , pseudowords with dense neighbourhoods showed less facilitation than those with sparse neighbourhoods. The full model was split into two sub-models: pseudoword targets with dense neighbourhoods and pseudoword targets with sparse neighbourhoods. NHD was removed from the equation and the same procedures for the analyses from the full model were applied to the sub-models. The effect of relatedness was significant for both pseudoword targets with dense neighbourhoods (b = 0.020, t = 3.93, p < .001, d = 0.09) and with sparse neighbourhoods (b = 0.036, t = 7.67, p < .001, d = 0.15).
With regard to the individual components, there was a significant effect of phonological precision and orthographic precision on log RT. Unsurprisingly, the higher the components of phonological precision and orthographic precision, the shorter the reaction times.
General discussion
Summary of findings
The current study used a suite of individual difference measures to assess which facets of LQH modulate lexical retrieval in production. In order to investigate sublexical facilitation effects during visual word naming and pseudoword naming, we manipulated NHD and prime and target lexicality in a masked form-priming naming experiment. We reasoned that visual word and pseudoword naming taps into an earlier, sublexical stage of reading, compared to LDT (Schilling et al., Citation1998). If visual word and pseudoword naming reflect the earlier (sublexical) stages of reading, then the direction of priming effects for visual word naming and visual pseudoword naming should be facilitatory in nature. The results are consistent with this hypothesis. Following Forster and Davis (Citation1991), one would predict that because word and pseudoword targets with dense neighbourhoods activate more words with shared phonological segments than those with sparse neighbourhoods, there should be more facilitation between naming similar primes and targets in the former than the latter. However, in contrast to Forster and Davis (Citation1991) we observed that both word and pseudoword targets with dense neighbourhoods showed less facilitatory form-priming than those with sparse neighbourhoods. A possible explanation for why our results differ from Forster and Davis (Citation1991), is that they primed word targets with a related pseudoword prime and an unrelated word prime, whereas our study was designed to disentangle the role of prime lexicality and relatedness. Mousikou et al. (Citation2015) argued that word primes are more likely to produce lexical competition in word naming, whereas pseudoword primes should facilitate grapheme-phoneme conversion. Our finding of decreased facilitation for targets with dense neighbourhoods compared to sparse neighbourhoods is consistent with the occurrence of lexical competition. Overall, our findings are consistent with the claim that visual word naming taps into an earlier stage of word recognition than the LDT. We observed only facilitatory effects of form-priming which are attributable to overlap in the activation of sublexical orthographic and/or phonological representations. The effects we observed that are consistent with lexical competition (i.e. interactions with NHD) are weaker than in the LDT and serve only to modulate the degree of facilitation due to form overlap. Interestingly, NHD also interacted with form-priming for pseudoword targets. However, this was the only effect of NHD observed for pseudoword targets, and was largely driven by reduced facilitation for word primes with dense neighbourhoods (See ), suggesting that lexical competition during word prime processing also affected the processing of pseudowords with dense neighbourhoods, and we return to this possibility below. It is clear that the interplay between the effects of sublexical facilitation and lexical competition during word recognition is complex, and influenced by several factors that determine the speed of access to different levels of representation, and the spread of activation within the mental lexicon.
We also observed effects of individual differences in aspects of reading skill. Similar to the LDT task, reported by Elsherif et al. (Citation2022), the component of phonological precision contributed to naming latencies for both word and pseudoword targets, with higher values of the component related to faster responses. In addition, there was a significant two-way interaction between phonological precision and priming effects only in pseudoword targets, such that people with high phonological precision also showed more facilitatory form-priming than people with low phonological precision. However, unlike in the LDT, the component of phonological precision showed no interactions with NHD or prime lexicality, suggesting that this skill component did not modulate lexical competition in visual word naming. Instead, these effects are consistent with phonological precision facilitating spoken word output processes in this task, especially for individuals with high phonological precision, consistent with the proposal of Forster and Davis (Citation1991). Shapiro et al. (Citation2013) and Moll et al. (Citation2009) have also argued that measures of reading fluency and phonological processing reflect the automaticity of grapheme-phoneme conversion. Participants with high phonological precision have more redundant mappings between orthography and phonology, enabling them to dedicate more resources to other processes, such as articulation. Participants with low phonological precision expend more resources for grapheme-phoneme conversion, leading to slower spoken word output (see also Timmer et al., Citation2012).
In contrast to the LDT, the priming effects we observed for both word and pseudoword targets were modulated by the individual difference component of orthographic precision. However these effects differed for the word and pseudoword targets. For word targets, there was a significant four-way interaction of orthographic precision, prime lexicality, NHD, and relatedness. People with high orthographic precision showed significantly less priming for targets from dense than sparse neighbourhoods, when primed by words. In contrast, facilitation from pseudoword primes was unaffected by NHD. These effects of NHD and prime lexicality are consistent with lexical competition, which reduces facilitation in participants with high orthographic precision. Following Perfetti’s (Citation2007, 2017) Lexical Quality Hypothesis (LQH), this is due to people with high orthographic precision having a more precise and redundant letter-sound correspondence. When the word prime is presented, people with high orthographic precision should process the prime quickly, thus suppressing any neighbouring candidates. Once the target word is shown, competition between the lexical representations of the prime and target ensues. As their sublexical representations are more stable, skilled readers can decode pseudowords efficiently and quickly, and thus are less likely to benefit from the pseudoword primes than readers with low orthographic precision. People with low orthographic precision showed a different pattern. The facilitation from word primes was unaffected by NHD but targets with dense neighbourhoods showed significantly less priming from pseudoword primes than targets with sparse neigbourhoods. According to the LQH, people with low orthographic precision would have less redundant and less precise letter-sound correspondences. They would therefore take longer to process the prime, within the given time frame, resulting in less efficient suppression of lexical competitors, such that only facilitation from shared sublexical and lexical representations is observed. The sublexical facilitation would be most beneficial for the processing of target words with little co-activation from lexical neighbours (i.e. targets from sparse neighbourhoods).
The pseudoword target experiment also showed interactions with orthographic precision. In this experiment, however, NHD did not interact with this factor. Instead, the direction of faciliation for people with high orthographic precision was greater for word than pseudoword primes, while people with low orthographic precision showed the opposite pattern. A possible explanation of this pattern is that the production of pseudowords is facilitated by the activation of shared sublexical representations and that this facilitation is more beneficial for people with low orthographic precision who have less redundant grapheme-phoneme correspondences. In contrast, pseudoword production may be susceptible to interference from activated lexical candidates. As argued above, lexical neighbours would be more rapidly suppressed by people with high orthographic precision, thereby reducing this interference. However, as only the three-way interaction was significant, we do not wish to make strong claims based on this effect, and our explanation is tentative, at best.
The role of LQH in masked priming for word and pseudoword targets
One of the main contributions of the present study is the inclusion of a suite of individual difference measures. These individual difference measures were grouped into different components using PCA. In the current neurotypical population, the PCA distinguished three components relating to phonology, orthography, and semantics. These components reflect processes which are distinct from one another. Perfetti (Citation2007) argued that skilled reading involves integrating these different components during online processing and the current study provides evidence that individuals integrate these components to different extents. Our results indicate that pseudoword and word naming tasks tap into an earlier stage of visual word recognition that is affected by our measure of orthographic precision. Our pattern of results is consistent with the claim that readers with high orthographic precision decode primes more effectively, as a result of more redundant letter-sound correspondence. In contrast to the LDT, Our measure of phonological precision related to naming latencies but did not interact with factors relating to sublexical and lexical activation (i.e. NHD and prime lexicality). This is consistent with phonological precision corresponding to the fluency of output processes in the visual word naming task, as opposed to lexical competition resolution as seen in the LDT.
The present study is the first to include measures of orthographic, phonological and semantic processing in visual word naming and demonstrated that priming effects depended significantly on the component of orthographic precision. NHD interacted with relatedness, prime lexicality and orthographic precision for word naming, but not for pseudoword naming. The current study therefore shows that the priming effects for word targets depend on the component of orthographic precision. This contrasts with what we found for visual word recognition in the LDT (Elsherif et al., Citation2022), where a component involving phonological precision, as opposed to orthographic precision, modulated the priming effects. These findings for visual word naming and visual word recognition might seem counterintuitive, as one would assume that phonology would contribute strongly to visual word naming, whereas orthographic processes would drive the priming effect in visual word recognition. However, we propose that this pattern is related to the different stages of word recognition being assessed in naming and LDT. As stated in the introduction, early processing measures are more strongly correlated with naming times than lexical decision times, while later measures are more strongly related to lexical decision times than naming latencies (Schilling et al., Citation1998). These findings indicate that the LDT primarily taps into the lexical processes of visual word recognition, while naming taps into earlier, more pre-lexical mechanisms of visual word recognition. The current findings are in line with Grainger et al. (Citation2006), who showed that phonology starts to have an influence in visual word recognition at 250 ms and modulates performance around 400 ms, while orthography already appeared around 200 ms. They concluded that orthographic processing arises earlier than phonologic processing.
The present results and the findings of Grainger et al. (Citation2006) are compatible with Grainger and Holcomb’s (Citation2009) bi-interactive activation model, which posits that a printed word stimulus activates a set of perceptual features which, in turn, activate sublexical orthographic codes. The sublexical orthography sends activation not only to the whole-word orthographic representation but also to the sublexical phonological representations via the grapheme-phoneme interface. Finally, orthographic processing and phonological processing converge on the lexical-orthographic representations, and from there on to appropriate semantic representations. Both orthographic and phonological precision produce activation in the lexical representation of the items, though with orthographic information used somewhat earlier than phonological information (see also Frisson et al., Citation2014). Future research should use a subtraction approach to assess the sequential activation processes from LDT to visual word naming and subtract the latencies of visual word naming from the latencies of LDT to provide a phonological access value (Catling & Elsherif, Citation2020; Santiago et al., Citation2000), assess which component affects lexical retrieval, especially when details of the specific item is unidentified or unretrieved (e.g. recognition without identification paradigm; Catling et al., Citation2021), or using a creative destruction approach (i.e. pre-specifying alternative results by competing hypotheses on a complex set of experimental findings; Tierney et al., Citation2020; Tierney et al., Citation2021).
We should be cautious about a few issues.Footnote6 One possibility is that the results could be confounding lexical processes with perceptual mechanisms in visual word naming. Of course, the present findings must also include the effects of perceptual processes. That is, some early and low-level system that notices the visual difference between the prime and target that may be relevant to the attentional system, but not to the lexical system during visual word naming (e.g. Chauncey et al., Citation2008). Importantly, our effects of orthographic precision cannot simply be attributed to an early perceptual effect that is peripheral to word identification. The current study observed that NHD, a lexical predictor, interacted with prime lexicality, orthographic precision and relatedness, which is indicative of lexical competition occurring. This means that it is difficult to explain these results by perceptual mechanisms alone.
Conclusion
In summary, the current study found a significant interaction of orthographic precision, prime lexicality, NHD, and relatedness. In individuals with high orthographic precision, greater facilitation was observed for words with dense neighbourhoods, primed by words, than those with sparse neighbourhoods. The converse was demonstrated for people with low orthographic precision only when primed by pseudowords. In addition, for people with low orthographic precision, greater facilitation was observed when a pseudoword target was primed by word primes than pseudoword primes, whereas the opposite pattern was demonstrated in people with high orthographic precision. Our results indicate that orthographic precision modulates lexical activation and retrieval in visual word naming. Our findings therefore contribute to the development of theoretical models that underlie visual word recognition and its time-course such that visual word naming taps into an earlier stage of lexical retrieval than the LDT. The goal of establishing a link between empirical data concerning individual differences in adult skilled readers and models of skilled reading may shed light on reading ability and further our understanding of fundamental aspects of the adult word recognition system.
Supplemental Material
Download Zip (508.9 KB)Acknowledgements
We thank all the participants for their involvement in the study. This study was part of the first author’s doctoral degree. Sections of this study were presented at the Psycholinguistics in Flanders (Ghent, Belgium, June 2018) and the Experimental Psychological Society (Bournemouth, 2019, London, 2019) conferences, thank you to the anonymous reviewers.
Disclosure statement
No potential conflict of interest was reported by the authors and no source of financial support.
Data deposition
All scripts, data and materials for the experiment are available at the open science framework at https://osf.io/efq5b/.
Notes
1 With 84 participants, 80 words and 80 pseudowords, we obtained a larger number of observations (1680) per condition than the recommended 1600 observations per condition (Brysbaert & Stevens, 2018).
2 The six participants did not differ in demographics and individual standardised test results (all Fs < 1) from the main group and their data were excluded from all further analyses. Following the advice of Woods et al. (Citation2021, 2022), the rate of missingness for gender was 7% but was missing at random.
3 The Raven Matrices, Manual Stroop and Naming Stroop were included as control measures for future research to ensure that the differences between groups did not result from non-verbal intelligence or inhibitory control (see Elsherif et al., Citation2021). These tests were not included in the PCA.
4 As target frequency approached significance, we included target frequency as a covariate in the model, the effect did not influence the interaction of orthographic precision, NHD, prime lexicality and relatedness.
5 As target phoneme length was significant, we included target phoneme length as a covariate in the model, the effect did not influence the interaction of orthographic precision, NHD, prime lexicality and relatedness.
6 We also acknowledge that we are mindful of constraints on the generality of our current paper (Delios et al., Citation2022; Parsons et al., Citation2022; Simons et al., Citation2017). Our study was conducted in English and with a Western, Educated, Industralised, Rich and Democratic (WEIRD) sample (Henrich et al., Citation2010). Our study was also reliant on student participation for data collection. We consider our large dataset to be representative of students within the UK - the population typically used in psycholinguistic research. However, caution should, of course, be used when applying our results to other stimuli (e.g. compound words; Elsherif & Catling, Citation2021) populations and languages.
References
- Acheson, D. J., Wells, J. B., & MacDonald, M. C. (2008). New and updated tests of print exposure and reading abilities in college students. Behavior Research Methods, 40(1), 278–289. https://doi.org/10.3758/BRM.40.1.278
- Adelman, J. S., Sabatos-DeVito, M. G., Marquis, S. J., & Estes, Z. (2014). Individual differences in reading aloud: A mega-study, item effects, and some models. Cognitive Psychology, 68, 113–160. https://doi.org/10.1016/j.cogpsych.2013.11.001
- Andrews, S. (2012). Individual differences in skilled visual word recognition: The role of lexical quality. In J. S. Adelman (Ed.), Visual word recognition: Vol. 2. Psychology Press.
- Andrews, S. (2015). Individual differences among skilled readers: The role of lexical quality. In A. Pollatsek, & R. Treiman (Eds.), The Oxford handbook of reading. Oxford University Press.
- Andrews, S., & Hersch, J. (2010). Lexical precision in skilled readers: Individual differences in masked neighbor priming. Journal of Experimental Psychology: General, 139(2), 299–318. https://doi.org/10.1037/a0018366
- Andrews, S., & Lo, S. (2012). Not all skilled readers have cracked the code: Individual differences in masked form priming. Journal of Experimental Psychology: Learning, Memory, and Cognition, 38(1), 152–163. https://doi.org/10.1037/a0024953.
- Baayen, R. H., Davidson, D. J., & Bates, D. M. (2008). Mixed-effects modeling with crossed random effects for subjects and items. Journal of Memory and Language, 59(4), 390–412. https://doi.org/10.1016/j.jml.2007.12.005
- Baayen, R. H., Piepenbrock, R., & Gulikers, L. (1995). The CELEX lexical database. [CD-ROM]. University of Pennsylvania, Linguistic Data Consortium.
- Barr, D. J., Levy, R., Scheepers, C., & Tily, H. J. (2013). Random effects structure for confirmatory hypothesis testing: Keep it maximal. Journal of Memory and Language, 68(3), 255–278. https://doi.org/10.1016/j.jml.2012.11.001
- Bates, D., Maechler, M., & Dai, B. (2010). lme4: Linear mixed-effects models using S4 classes (R package Version 0.999375-27)[Computer software].
- Bernaards, C. A., & Jennrich, R. I. (2005). Gradient projection algorithms and software for arbitrary rotation criteria in factor analysis. Educational and Psychological Measurement, 65(5), 676–696. https://doi.org/10.1177/0013164404272507
- Brysbaert, M., & Cortese, M. J. (2010). Do the effects of subjective frequency and age of acquisition survive better word frequency norms? Quarterly Journal of Experimental Psychology, 64(3), 545–559. https://doi.org/10.1080/17470218.2010.503374
- Burt, J. S., & Fury, M. B. (2000). Spelling in adults: The role of reading skills and experience. Reading and Writing, 13(1-2), 1–30. https://doi.org/10.1023/A:1008071802996
- Castles, A., Rastle, K., & Nation, K. (2018). Ending the reading wars: Reading acquisition from novice to expert. Psychological Science in the Public Interest, 19(1), 5–51. https://doi.org/10.1177/1529100618772271
- Catling, J. C., & Elsherif, M. M. (2020). The hunt for the age of acquisition effect: It’s in the links!. Acta Psychologica, 209, 103138. https://doi.org/10.1016/j.actpsy.2020.103138
- Catling, J. C., Pymont, C., Johnston, R. A., Elsherif, M. M., Clark, R., & Kendall, E. (2021). Age of acquisition effects in recognition without identification tasks. Memory (Hove, England), 29(5), 662–674. https://doi.org/10.1080/09658211.2021.1931695
- Chauncey, K., Holcomb, P. J., & Grainger, J. (2008). Effects of stimulus font and size on masked repetition priming: An event-related potentials (ERP) investigation. Language and Cognitive Processes, 23(1), 183–200. https://doi.org/10.1080/01690960701579839
- Cohen, J. (1988). Statistical power analysis for the behavioral sciences. Erlbaum-Drucker.
- Coltheart, M., Davelaar, E., Jonasson, J. F., & Besner, D. (1977). Access to the internal lexicon. In S. Dornic (Ed.), Attention and performance VI (pp. 535–555). Erlbaum.
- Cortese, M. J., & Khanna, M. M. (2007). Age of acquisition predicts naming and lexical-decision performance above and beyond 22 other predictor variables: An analysis of 2,342 words. Quarterly Journal of Experimental Psychology, 60(8), 1072–1082. https://doi.org/10.1080/17470210701315467
- Cortese, M. J., Yates, M., Schock, J., & Vilks, L. (2018). Examining word processing via a megastudy of conditional reading aloud. Quarterly Journal of Experimental Psychology, 71(11), 2295–2313. https://doi.org/10.1177/1747021817741269
- Cunningham, A. E., & Stanovich, K. E. (1990). Assessing print exposure and orthographic processing skill in children: A quick measure of reading experience. Journal of Educational Psychology, 82(4), 733–740. https://doi.org/10.1037/0022-0663.82.4.733
- Davis, C. J. (2005). N-Watch: A program for deriving neighborhood size and other psycholinguistic statistics. Behavior Research Methods, 37(1), 65–70. https://doi.org/10.3758/BF03206399
- Davis, C. J., & Lupker, S. J. (2006). Masked inhibitory priming in English: Evidence for lexical inhibition. Journal of Experimental Psychology: Human Perception and Performance, 32(3), 668–687. https://doi.org/10.1037/0096-1523.32.3.668
- Delios, A., Clemente, E. G., Wu, T., Tan, H., Wang, Y., Gordon, M., Viganola, D., Chen, Z., Dreber Johannesson, M., Pfeiffer, T., Generalizability Tests Forecasting Collaboration, & Uhlmann, E. L. (2022). Examining the generalizability of research findings from archival data. Proceedings of the National Academy of Sciences, 119(30), e2120377119. https://doi.org/10.1073/pnas.2120377119
- Dimitropoulou, M., Duñabeitia, J. A., & Carreiras, M. (2010). Influence of prime lexicality, frequency, and pronounceability on the masked onset priming effect. Quarterly Journal of Experimental Psychology, 63(9), 1813–1837. https://doi.org/10.1080/17470210903540763
- Dunn, L., Dunn, L. M., Whetton, C., & Burley, J. (1997). British Picture Vocabulary Scale ii. GL Assessment.
- Ehri, L. C. (2005). Development of sight word reading: Phases and findings. In M. J. Snowling, & C. Hulme (Eds.), The science of reading: A handbook (pp. 135–154). Blackwell Publishing.
- Elliott, C. D., Smith, P., & McCulloch, K. (1996). British ability scales second edition (BAS II): administration and scoring manual. NFER-Nelson.
- Elsherif, M. M., & Catling, J. C. (2021). Age of acquisition effects on the decomposition of compound words. Journal of Cognitive Psychology, 1–14. https://doi.org/10.1080/20445911.2021.2013246
- Elsherif, M. M., Wheeldon, L. R., & Frisson, S. (2021). Do dyslexia and stuttering share a processing deficit? Journal of Fluency Disorders, 67(1), 105827. https://doi.org/10.1016/j.jfludis.2020.105827
- Elsherif, M. M., Wheeldon, L. R., & Frisson, S. (2022). Phonological precision for word recognition in skilled readers. Quarterly Journal of Experimental Psychology, 75(6), 1021–1040. https://doi.org/10.1177/17470218211046350
- Faul, F., Erdfelder, E., Buchner, A., & Lang, A. (2009). Statistical power analyses using G*power 3.1: Tests for correlation and regression analyses. Behavior Research Methods, 41(4), 1149–1160. https://doi.org/10.3758/BRM.41.4.1149
- Ferrand, L., Brysbaert, M., Keuleers, E., New, B., Bonin, P., Méot, A., Augustinova, M., & Pallier, C. (2011). Comparing word processing times in naming, lexical decision, and progressive demasking: Evidence from chronolex. Frontiers in Psychology, 2(306), 1–10. https://doi.org/10.3389/fpsyg.2011.00306
- Field, A. P. (2009). Discovering statistics using SPSS: (and sex and drugs and rock “n” roll) (3rd ed.). Sage.
- Forster, K. I., & Davis, C. (1991). The density constraint on form-priming in the naming task: Interference effects from a masked prime. Journal of Memory and Language, 30(1), 1–25. https://doi.org/10.1016/0749-596X(91)90008-8
- Forster, K. I., & Veres, C. (1998). The prime lexicality effect: Form-priming as a function of prime awareness, lexical status, and discrimination difficulty. Journal of Experimental Psychology: Learning, Memory, and Cognition, 24(2), 498–514. https://doi.org/10.1037/0278-7393.24.2.498
- Frisson, S., Bélanger, N. N., & Rayner, K. (2014). Phonological and orthographic overlap effects in fast and masked priming. Quarterly Journal of Experimental Psychology, 67(9), 1742–1767. https://doi.org/10.1080/17470218.2013.869614
- Gough, P. B., & Tunmer, W. E. (1986). Decoding, reading, and reading disability. Remedial and Special Education, 7(1), 6–10. https://doi.org/10.1177/074193258600700104
- Grainger, J., & Holcomb, P. J. (2009). Watching the word Go by: On the time-course of component processes in visual word recognition. Language and Linguistics Compass, 3(1), 128–156. https://doi.org/10.1111/j.1749-818X.2008.00121.x
- Grainger, J., Kiyonaga, K., & Holcomb, P. J. (2006). The time course of orthographic and phonological code activation. Psychological Science, 17(12), 1021–1026. https://doi.org/10.1111/j.1467-9280.2006.01821.x
- Henrich, J., Heine, S. J., & Norenzayan, A. (2010). The weirdest people in the world? Behavioral and Brain Sciences, 33(2-3), 61–83. https://doi.org/10.1017/S0140525X0999152X
- Holmes, J., Hilton, K. A., Place, M., Alloway, T. P., Elliott, J. G., & Gathercole, S. E. (2014). Children with low working memory and children with ADHD: Same or different? Frontiers in Human Neuroscience, 8, 976. https://doi.org/10.3389/fnhum.2014.00976
- Huettig, F., Lachmann, T., Reis, A., & Petersson, K. M. (2018). Distinguishing cause from effect – many deficits associated with developmental dyslexia may be a consequence of reduced and suboptimal reading experience. Language, Cognition and Neuroscience, 33(3), 333–350. https://doi.org/10.1080/23273798.2017.1348528
- Jackson, N.E. (2005). Are university students' component reading skills related to their text comprehension and academic achievement?. Learning and Individual Differences, 15(2), 113–139. https://doi.org/10.1016/j.lindif.2004.11.001
- Katz, L., Brancazio, L., Irwin, J., Katz, S., Magnuson, J., & Whalen, D. H. (2012). What lexical decision and naming tell US about reading. Reading and Writing, 25(6), 1259–1282. https://doi.org/10.1007/s11145-011-9316-9
- Laberge, D., & Samuels, S. J. (1974). Toward a theory of automatic information processing in reading. Cognitive Psychology, 6(2), 293–323. https://doi.org/10.1016/0010-0285(74)90015-2
- Language and Reading Research Consortium. (2015). Learning to read: Should we keep things simple? Reading Research Quarterly, 50, 151–169. https://doi.org/10.1002/rrq.99
- Marian, V., Bartolotti, J., Chabal, S., & Shook, A. (2012). CLEARPOND: cross-linguistic easy-access resource for phonological and orthographic neighborhood densities. PLoS ONE, 7(8), e43230. https://doi.org/10.1371/journal.pone.0043230
- Martens, V. E., & De Jong, P. F. (2008). Effects of repeated reading on the length effect in word and pseudoword reading. Journal of Research in Reading, 31(1), 40–54. https://doi.org/10.1111/j.1467-9817.2007.00360.x
- Martin-Chang, S. L., & Gould, O. N. (2008). Revisiting print exposure: Exploring differential links to vocabulary, comprehension and reading rate. Journal of Research in Reading, 31(3), 273–284. https://doi.org/10.1111/j.1467-9817.2008.00371.x
- Meade, G., Grainger, J., Midgley, K. J., Emmorey, K., & Holcomb, P. J. (2018). From sublexical facilitation to lexical competition: ERP effects of masked neighbor priming. Brain Research, 1685, 29–41. https://doi.org/10.1016/j.brainres.2018.01.029
- Moll, K., Fussenegger, B., Willburger, E., & Landerl, K. (2009). Ran is not a measure of orthographic processing. Evidence from the asymmetric German orthography. Scientific Studies of Reading, 13(1), 1–25. https://doi.org/10.1080/10888430802631684
- Mousikou, P., Rastle, K., Besner, D., & Coltheart, M. (2015). The locus of serial processing in reading aloud: Orthography–to–phonology computation or speech planning? Journal of Experimental Psychology: Learning, Memory, and Cognition, 41(4), 1076–1099. https://doi.org/10.1037/xlm0000090
- Parsons, S., Azevedo, F., Elsherif, M. M., Guay, S., Shahimet, O. N., Govaart, G., Norris, M., O’Mahony, A., Parker, A. J., Todorovic, A., Pennington, C. R., Garcia-Pelegrin, E., Lazić, A., Robertson, O., Middleton, S. L., Valentini, B., McCuaig, J., Baker, B. J., Collins, E., … Aczel, B. (2022). A community-sourced glossary of open scholarship terms. Nature Human Behaviour, 6(3), 312–318. https://doi.org/10.1038/s41562-021-01269-4
- Perfetti, C. (2007). Reading ability: Lexical quality to comprehension. Scientific Studies of Reading, 11(4), 357–383. https://doi.org/10.1080/10888430701530730
- Perfetti, C. A. (1992). The representation problem in reading acquisition. In P. B. Gough, L. C. Ehri, & R. Treiman (Eds.), Reading acquisition (pp. 145–174). Erlbaum.
- Perfetti, C. A. (2017). Lexical quality revisited. In E. Segers, & P. van den Broek (Eds.), Developmental perspectives in written language and literacy: In honor of ludo verhoeven (pp. 51–68). John Benjamins.
- Perfetti, C. A., & Hart, L. (2001). The lexical bases of comprehension skill. In D. S. Gorfien (Ed.), On the consequences of meaning selection (pp. 67–86). American Psychological Association.
- Perfetti, C. A., & Hart, L. (2002). The lexical quality hypothesis. In L. Vehoeven, C. Elbron, & P. Reitsma (Eds.), Precursors of functional literacy (pp. 189–213). John Benjamins.
- Raftery, A. E. (1995). Bayesian model selection in social research. In P. V. Marsden (Ed.), Sociological methodology 1995 (pp. 111–163). Blackwell.
- Rastle, K., & Brysbaert, M. (2006). Masked phonological priming effects in English: Are they real? Do they matter? Cognitive Psychology, 53(2), 97–145. https://doi.org/10.1016/j.cogpsych.2006.01.002
- Raven, J. C. (1960). Guide to using the standard progressive matrices. Lewis.
- R Development Core Team. (2017). r: a language and environment for statistical computing. R Foundation for Statistical Computing, Vienna, Austria URL http://www.R-project.org.ISBN3-900051-00-3
- Reynolds, C. R., & Kamphaus, R. (2007). Tiwre: Test of Irregular Word Reading efficiency. Psychological Assessment Resources.
- Santiago, J., MacKay, D. G., Palma, A., & Rho, C. (2000). Sequential activation processes in producing words and syllables: Evidence from picture naming. Language and Cognitive Processes, 15(1), 1–44. https://doi.org/10.1080/016909600386101
- Schilling, H. E., Rayner, K., & Chumbley, J. I. (1998). Comparing naming, lexical decision, and eye fixation times: Word frequency effects and individual differences. Memory & Cognition, 26(6), 1270–1281. https://doi.org/10.3758/BF03201199
- Schwarz, G. (1978). Estimating the dimension of a model. The Annals of Statistics, 6(2), 461–464. https://doi.org/10.1214/aos/1176344136
- Segui, J., & Grainger, J. (1990). Priming word recognition with orthographic neighbors: Effects of relative prime-target frequency. Journal of Experimental Psychology: Human Perception and Performance, 16(1), 65–76. https://doi.org/10.1037/0096-1523.16.1.65.
- Shapiro, L. R., Carroll, J. M., & Solity, J. E. (2013). Separating the influences of prereading skills on early word and nonword reading. Journal of Experimental Child Psychology, 116(2), 278–295. https://doi.org/10.1016/j.jecp.2013.05.011
- Share, D. L. (1995). Phonological recoding and self-teaching: Sine qua non of reading acquisition. Cognition, 55(2), 151–218. https://doi.org/10.1016/0010-0277(94)00645-2
- Silverman, R.D., Speece, D.L., Harring, J.R., & Ritchey, K. D. (2013). Fluency Has a Role in the Simple View of Reading. Scientific Studies of Reading, 17(2), 108–133. https://doi.org/10.1080/10888438.2011.618153
- Simons, D. J., Shoda, Y., & Lindsay, D. S. (2017). Constraints on generality (COG): A proposed addition to all empirical papers. Perspectives on Psychological Science, 12(6), 1123–1128. https://doi.org/10.1177/1745691617708630
- Singmann, H., Bolker, B., Westfall, J., Aust, F., & Ben-Shachar, M. S. (2020). afex: Analysis of factorial experiments (Version 0.28-0) [Computer software]. Comprehensive R Archive Network. https://CRAN.R-project.org/package=afex
- Stanovich, K. E., & West, R. F. (1989). Exposure to print and orthographic processing. Reading Research Quarterly, 24(4), 402–433. https://doi.org/10.2307/747605
- Stroop, J. R. (1935). Studies of interference in serial verbal reactions. Journal of Experimental Psychology, 18(6), 643–662. https://doi.org/10.1037/h0054651
- Tabachnick, B.G., Fidell, L.S., & Ullman, J.B. (2007). Using multivariate statistics. Pearson.
- Tierney, W., Hardy, J., Ebersole, C. R., Viganola, D., Clemente, E. G., Gordon, M., Hoogeveen, S., Haaf, J., Dreber, A., Johannesson, M., Pfeiffer, T., Huang, J. L., Vaughn, L. A., DeMarree, K., Igou, E. R., Chapman, H., Gantman, A., Vanaman, M., Wylie, J., … Uhlmann, E. L. (2021). A creative destruction approach to replication: Implicit work and sex morality across cultures. Journal of Experimental Social Psychology, 93, 104060–18. https://doi.org/10.1016/j.jesp.2020.104060
- Tierney, W., Hardy, J. H., Ebersole, C. R., Leavitt, K., Viganola, D., Clemente, E. G., Gordon, M., Dreber, A., Johannesson, M., Pfeiffer, T., Hiring Decisions Forecasting Collaboration, & Uhlmann, E. L. (2020). Creative destruction in science. Organizational Behavior and Human Decision Processes, 161, 291–309. https://doi.org/10.1016/j.obhdp.2020.07.002.
- Timmer, K., Vahid-Gharavi, N., & Schiller, N. O. (2012). Reading aloud in Persian: ERP evidence for an early locus of the masked onset priming effect. Brain and Language, 122(1), 34–41. https://doi.org/10.1016/j.bandl.2012.04.013
- Torgesen, J. K., Rashotte, C. A., & Wagner, R. K. (1999). Towre: Test of word reading efficiency. Pro-ed.
- van Heuven, W. J., Mandera, P., Keuleers, E., & Brysbaert, M. (2014). SUBTLEX-UK: A new and improved word frequency database for British English. Quarterly Journal of Experimental Psychology, 67(6), 1176–1190. https://doi.org/10.1080/17470218.2013.850521
- Veldre, A., & Andrews, S. (2014). Lexical quality and eye movements: Individual differences in the perceptual span of skilled adult readers. Quarterly Journal of Experimental Psychology, 67(4), 703–727. https://doi.org/10.1080/17470218.2013.826258
- Wagner, R. K., Torgesen, J. K., & Rashotte, C. A. (1999). Comprehensive test of phonological processing: CTOPP. Pro-ed.
- Westfall, J., Kenny, D. A., & Judd, C. M. (2014). Statistical power and optimal design in experiments in which samples of participants respond to samples of stimuli. Journal of Experimental Psychology: General, 143(5), 2020–2045. https://doi.org/10.1037/xge0000014
- Wheat, K. L., Cornelissen, P. L., Frost, S. J., & Hansen, P. C. (2010). During visual word recognition, phonology Is accessed within 100 ms and May Be mediated by a speech production code: Evidence from magnetoencephalography. Journal of Neuroscience, 30(15), 5229–5233. https://doi.org/10.1523/JNEUROSCI.4448-09.2010
- Wiederholt, J. L., & Blalock, G. (2000). Gsrt: Gray Silent Reading tests. Pro-Ed.
- Williams, K. T. (2007). EVT-2: Expressive vocabulary test. Pearson Assessments.
- Woods, A. D., Davis-Kean, P., Halvorson, M. A., King, K. M., Logan, J. A. R., Xu, M., Bainter, S., Brown, D., Clay, J.M., Cruz, R.A., Elsherif, M.M., Gerasimova, dD., Joyal-Desmarais, K., Moreau, D., Nissen, J., Schmidt, K., Uzdavines, A., Van Dusen, B., & Vasilev, M. R. (2021). Missing Data and Multiple Imputation Decision Tree. https://doi.org/10.31234/osf.io/mdw5r
- Woods, A. D., Gerasimova, D., Van Dusen, B., Nissen, J., Bainter, S., Uzdavines, A., Davis-Kean, P., King, K. M., Logan, J. A. R., Xu, M., Vasilev, M.R., Clay, J.M., Moreau, D., Joyal-Desmarais, J., Cruz, A.R., Brown, D., Schmidt, K., & Elsherif, M. M. (2022). Best practices for addressing missing data through multiple imputation. https://doi.org/10.31234/osf.io/uaezh.
- Ziegler, J. C., & Goswami, U. (2005). Reading acquisition, developmental dyslexia, and skilled reading across languages: A psycholinguistic grain size theory. Psychological Bulletin, 131(1), 3–29. https://doi.org/10.1037/0033-2909.131.1.3