Abstract
Increased intraindividual variability (IIV) has been linked to outcomes such as cognitive decline and dementia, suggesting IIV might add valuable diagnostic information beyond traditional neuropsychological interpretation. We explored whether a subtype of IIV, dispersion, can provide additional information for dementia diagnosis. In a sample of memory clinic patients, three cognitive status groups were identified: subjective cognitive impairment (SCI; n = 85), amnestic mild cognitive impairment (a-MCI; n = 16), and dementia due to Alzheimer’s disease (AD; n = 48). Dispersion was computed as intraindividual standard deviations across multiple neuropsychological measures within three cognitive domains (executive functioning; immediate and delayed memory) and was compared for each diagnostic group using profile analysis. Patients with AD and a-MCI demonstrated less dispersion than patients with SCI in delayed memory. Results support existing theoretic perspectives on cognitive variability and age-related cognitive decline but suggest floor effects underlie suppression of dispersion in amnestic cognitive presentations. Questions remain about the contribution of IIV beyond impressions of impairment versus no impairment in these constrained representations of cognitive domains. Future investigations should investigate variability in SCI groups against controls to examine whether observed dispersion similarities between SCI and a-MCI or AD in immediate memory and executive functioning are meaningful.
Introduction
Currently, over 564,000 Canadians live with dementia, with this number expected to approach 1 million in the next 15 years (Alzheimer’s Society of Canada, Citation2016), placing large demands on the individuals involved in patient care (Carretero et al., Citation2009; Etters et al., Citation2008; Zarit et al, Citation1980). Diagnosis is critical, with evidence suggesting timely identification improves patient quality of life (Alzheimer’s Disease International, Citation2011). Conversely, diagnostic delays are associated with hospitalization and premature long-term care placement (Gaugler et al., Citation2005; McCloskey et al., Citation2014). We explored whether intraindividual variability (IIV) within a cognitive domain (i.e., immediate memory, delayed memory, and executive functioning) would provide novel diagnostic information by examining individuals with subjective cognitive impairment (SCI), amnestic mild cognitive impairment (a-MCI), and dementia due to Alzheimer’s Disease (AD).
Dementia due to Alzheimer Disease (AD) can produce non-uniform declines in the cognitive domain of memory with short term (secondary) memory functions dissociating from long term (primary) memory as the disease progresses (Becker, Citation1988; Baddeley et al., Citation1991; Becker, Lopez, & Wess, Citation1992). This non-linear decline can produce distinct memory profiles (e.g., Becker, et al., Citation1992; Mayes & Roberts, Citation2001; Shepard, Citation1967); individuals with similar disease severity indexed different weaknesses in memory across neuropsychological tests (Stopford et al, Citation2007). In addition to the distinct memory profiles observed with AD, the disease can impact an individual’s cognitive profile at the level of executive functioning (EF). EF dysfunction is a disease indicator and potential predictor of functional status for individuals with dementia (Logie et al., Citation2004; Kim et al., Citation2017). Using verbal fluency tasks, it has been consistently demonstrated that there is differential impact of AD on EF. Semantic fluency suffers to a greater degree than phonemic fluency (Monsch, et al., Citation1994; Murphy et al., Citation2006), with the disparity increasing as the disease progresses (Salmon et al., Citation1999). Clearly, AD pathology generates distinct profiles of neuropsychological dysfunction.
This research into aging and cognitive impairment has traditionally focused on tracking performance on single tasks or single cognitive domains across time, an approach that assumes individual variability is noise surrounding the true measure of interest (e.g., MacDonald & Stawski, Citation2015). More recently, however, intraindividual variability (IIV) has become of interest. IIV can be conceptualized in several ways such as inconsistency and dispersion. Both subtypes have been tied with the cognitive aging process independent of raw test performance, with older adults scoring higher on all types (Hultsch et al., Citation2002; MacDonald & Stawski, Citation2020). IIV has also been demonstrated as more sensitive to central nervous system vulnerability than mean differences on neuropsychological testing (MacDonald & Stawski, Citation2020).
Given IIV’s ties to central nervous system integrity, there is also research suggesting connections between IIV neuropathology such as dementia. For instance, increases in IIV have been found to be a potential preclinical marker of AD (e.g., Gorus et al., Citation2008), with the potential to improve prediction of the disease (Holtzer et al., Citation2008). Additionally, baseline IIV has been linked to incident dementia in a population sample (Kochan et al., Citation2016). Further, increased IIV was associated with AD as well as Parkinson’s Dementia (PD) (Burton et al., Citation2006), indicating ties to decreased central nervous system integrity. Additionally, this study indicated differences in IIV between AD and PD groups, suggesting the presence of information relevant to the determination of disease etiology. Another study supports this assertion, with IIV magnitude differing across dementia subtypes (Murtha et al., Citation2002). Specifically, individuals with frontal-lobe dementia exhibited increased variability in Stroop tests relative to those with AD.
Changes in IIV are also relevant to mild cognitive impairment (MCI). For example, increased IIV has been found to be predictive of MCI diagnosis (Dixon et al., Citation2007), with higher baseline IIV increasing longitudinal risk (Bielak et al., Citation2010; Cherbuin et al., Citation2010). Elevated within-person variability has also been shown on tasks of complex attention tasks requiring inhibition and switching (Strauss et al., Citation2007) as well as visual search tasks (McLaughlin et al., Citation2010; Phillips et al., Citation2013) for individuals with MCI. As well, IIV has been suggested as a unique predictor of MCI group membership (versus control), over and above performance accuracy (Troyer et al., Citation2016). This elevated variability may hold relevance for conversion from MCI to dementia, with investigations suggesting high IIV relates to an increased probability that an individual with a-MCI will progress to dementia within 2–4 years (Bayer et al., Citation2014; Tales et al., Citation2012).
IIV in the context of SCI—a putative prodrome of MCI and AD (Jessen et al. Citation2014)—is less understood. By its nature, SCI is a disorder in which ostensibly healthy individuals report self-perceived impairment in cognition, usually memory, in the absence of objective evidence upon formal neuropsychological evaluation (Jessen et al., Citation2014). As a result, potential functional decline in daily life and other areas of wellness may be underestimated or undetected by clinicians (Tales et al., Citation2014). One study of SCI suggests that for some individuals, perceived memory changes in can also be associated with slowed and/or more variable information processing (Jenkins et al., Citation2019). This finding supports previous literature suggesting relevant diagnostic information (e.g., functional information) can be missed in those with SCI (Tales et al., Citation2014). As well, in a sample of older adults, cross-domain IIV was associated with declining functional status (Rapp et al., Citation2005). This may be implicated in deleterious aging, given the functional emphasis in dementia diagnosis (e.g., Gauthier et al., Citation2012). Synthesizing these findings, there is an imperative to better understand IIV within this understudied population and how it is related to more developed pathology such as MCI and AD. This is especially true considering evidence suggesting IIV possesses utility in both delineating levels of impairment (Dixon et al., Citation2007; Strauss et al., Citation2007) when used alongside traditional scores (Tractenberg & Pietrzak, Citation2011), and in predicting cognitive change across domains (Haynes et al., Citation2017).
To complement IIV’s associations with deleterious aging, there is considerable empirical support for the position that IIV is related to the integrity of underlying neural systems (e.g., Costa et al., Citation2019; Haynes et al., 2017; MacDonald et al., Citation2006, Citation2009). It is therefore likely that these findings are relevant to explorations of clinical subgroups. For instance, IIV has been implicated in frontal changes gray matter, white matter, or neurotransmitters (MacDonald et al., Citation2006), as well as prefrontal volume changes (Lövdén et al., Citation2013). Further, elevations in IIV have been associated with increased activity in left and right middle frontal regions (Bellgrove et al., Citation2004). This increased activation was related to heightened demand for executive control to maintain task performance (see also Strauss et al., Citation2007).
IIV is thus somewhat involved with frontal and prefrontal systems which may have utility differentiating clinical subgroups in aging. In light of previously described evidence that different types of dementia may harbor different variability profiles (Burton et al., Citation2006; Murtha et al., Citation2002), IIV may be able to help systematically differentiate clinical presentations (e.g., between frontal dementias and other phenotypes). As well, executive functioning is often disrupted in MCI (e.g., Marshall et al., Citation2011). Thus, detecting fluctuations in IIV may hold promise for uncovering MCI as well as currently subclinical cases (i.e., SCI). An additional consideration is IIV’s association to disruptions in medial temporal regions (Troyer et al., Citation2016), which may provide information relevant to MCI and AD diagnoses. These regions are involved in AD related decline, specifically difficulties in encoding and storage of new information (e.g., Peña-Casanova et al., Citation2012). Accordingly, IIV elevations may function as a red flag for early detection of the AD disease process.
As demonstrated above, increasing IIV has been linked to deleterious age-related outcomes such as cognitive decline and dementia, but much of this work focusses on IIV conceptualized as inconsistency. Dispersion is typically described in the context of cognitive or neuropsychological tasks, capturing the amount of variability across a battery of performance measures for a given individual and point in time (Christensen et al., Citation1999; Hultsch et al., Citation2002). It is sensitive to age (Christensen et al., Citation1999) with older adults indexing higher dispersion, and may be reflective of developmental phenomena (Mella et al., Citation2016). Further, it has been correlated with cognitive performance (Hultsch, Citation2002; Hilborn et al., Citation2009) and is potentially predictive of incident dementia (Holtzer et al., Citation2008). Dispersion has also been employed within domains of cognitive functioning, with differences in variability patterns being observed across reaction time tasks and working memory tasks in a lifespan sample (Mella et al., Citation2015). From this evidence emerges the rationale for the present study. The above literature demonstrated the presence of distinct cognitive profiles in AD, alongside evidence that IIV holds associations with dementia. In combining these lines of research with the relative dearth of information on dispersion, there is an opportunity to explore variability patterns at the domain level as they relate to AD and its prodromes.
The present objective was to explore the dispersion profiles of individuals with AD, a-MCI, and subjective cognitive impairment (SCI; those who were worried about their cognition but demonstrated no objective cognitive impairment) across a battery of neuropsychological tasks spanning several theoretical domains of cognitive functioning. Domains of interest were immediate memory, delayed memory, and executive functioning. Presently, executive functioning is explored as a single domain defined as mental functions associated with the ability to engage in purposeful, organized, self-regulated, and goal-directed behaviors (McCloskey et al., Citation2009) and integrate, synthesize, and organize other cognitive processes (Wecker et al., Citation2000). Dispersion variability estimates were calculated in the distinct domains of executive functioning, immediate memory, and delayed memory. These cognitive domains align with previous literature suggesting vulnerability to the AD process at the level of score-based analysis (Logie et al., Citation2004; Welsh et al., Citation1991).
Method
Participants
Archival data from 149 consecutive individuals assessed at the Rural and Remote Memory Clinic (RRMC) between approximately 2013 and 2019 were analyzed. Assessments were interdisciplinary; participants attended a one-day session involving a physical therapist, registered nurse, neurologist, and neuropsychologist (Morgan et al., Citation2009). Inclusion criteria were based on completion of the neuropsychological battery, as well as provision of consent for data to be used for research. The sample included persons diagnosed with AD (n = 48; 12 male, 36 female), a-MCI (n = 16; 10 male, 6 female), and SCI (n = 85; 43 male, 42 female). Sample demographics are outlined in , with education level determined using the guidelines in detailed by Strauss et al. (Citation2006). Individuals diagnosed with SCI were referred for subjective complaints but received a diagnosis of no cognitive impairment based on a lack of objective evidence and no functional impairment. Diagnoses were made independently by the neurologist and neuropsychologist, who then discussed to reach clinician consensus. Diagnoses were consistent with guidelines for diagnosing cognitive impairment and dementia (4th and 5th Annual Canadian Consensus Conference on the Diagnosis and Treatment of Dementia; Gauthier et al., Citation2012; Ismail et al., Citation2020). Of specific note, diagnoses of a-MCI were by the determined presence of cognitive impairment (i.e., less than 5th percentile on a given test) in one or more domains, as well as the absence of functional impairment (Albert et al., Citation2011).
Table 1. Demographics characteristics for the diagnostic subgroups.
Table 2. Mean z-scores for neuropsychological tests across the three cognitive classifications.
Measures
Short form California Verbal Learning Test-Second edition
The nine-item Short Form of the California Verbal Learning Test Second Edition (CVLT-II SF; Delis et al., Citation2000) was used to assess verbal episodic memory. The task required four learning trials comprised of 9 words in three categories (fruits, clothing, and tools). Subsequently two delayed free recall trials (30-s and 10-min delays), a delayed cued recall trial (10-min delay) and a recognition trial (10-min delay) were completed. The reliability of the parent CVLT-II appears acceptable, with internal consistency values ranging from .78 to .94, across all ages (Delis et al., Citation2000), alongside acceptable test-retest reliability of the short form (Woods et al., Citation2006). In addition, there is support for the criterion validity of the CVLT-II in patients with various neurological conditions (Baldoet al., Citation2002; Delis et al., Citation2005; Donders & Nienhuis, Citation2007).
Brief Visuospatial Memory Test-Revised
The Brief Visuospatial Memory Test-Revised (BVMT-R; Benedict, Citation1997) was used to assess visuospatial episodic memory. There are three learning trials, in which a stimulus page containing six geometric shapes was presented for 10 s. The examinee was then asked to draw as many as they could remember in the correct place on a response page (i.e., immediate recall), and this procedure is completed a total of 3 times. A delayed recall trial is administered after 25 min. Finally, a recognition trial is administered where the respondent was asked to identify which of 12 shapes were included among the originals. Investigations of the psychometric properties of the BVMT-R point to adequate reliability and validity (Benedict, Citation1997; Benedict et al., Citation1996; Kane & Yochim, Citation2014). Further, it has demonstrated utility in detection of memory deficits arising of various etiologies (e.g., Belanger et al., Citation2009; Benedict & Zivadinov, Citation2007; Strober et al., Citation2009).
Delis-Kaplan Executive Function System
The Delis-Kaplan Executive Function System (DKEFS; Delis et al., Citation2001) is a set of tests designed for the assessment of executive functioning, both verbal and nonverbal. Tests administered included the Trail Making Test, Verbal Fluency Test, and the Color-Word Interference Test. The Trails test consisted of a collection of connect-the-circle tasks as well as a cancellation/visual scanning task. The Verbal Fluency Test measures fluid word generation in an effortful phonemic condition, a category based (i.e., semantic) condition and a category switching condition requiring the examinee to alternate between semantic categories. The Color-Word Interference Test indexed inhibition of an overlearned response (i.e., reading the word on the page) by producing an atypical response by indicating the color of the ink in which the words are printed. An inhibition/switching condition further indexes inhibition as well as cognitive flexibility (Swanson, Citation2005). The DKEFS has been suggested to have an overall moderate test-retest reliability given the difficulties in assessing executive function (Shunk et al., Citation2006), and has been shown to possess comparable reliability coefficients to other neuropsychological tests (e.g., Wisconsin Card Sorting Task & Wechsler Memory Scale Third Edition; Delis et al., Citation2004). Evidence for the DKEFS’s validity has been provided in terms of appropriate sensitivity of the tests in the detection of frontal brain damage and in the ability of the tests to measure areas of higher-level executive functions (Delis et al., Citation2001). Numerous studies have demonstrated that D-KEFS tests are sensitive to the assessment of executive-function deficits in numerous clinical populations (see Delis et al., Citation2004 for a list).
Wechsler Memory Scale Fourth Edition
Select subtests from the Wechsler Memory Scale Fourth Edition (WMS-IV; Wechsler, Citation2009) were administered to further assess verbal episodic memory. Logical memory I & II were both administered to assess free recall of two verbally presented short stories. The examinee was asked to recall details from the stories immediately, and then were given another opportunity following a 20- to 30-min delay. A yes/no recognition trial was administered after the respective delayed recall trials. Reliability evidence for the WMS-IV has been described in the test manual (Wechsler, Citation2009), as well as interpretive texts (Drozdick et al., Citation2011). In the clinical standardization sample, average split half reliabilities range from 0.86 to 0.97 at the subtest level (Drozdick et al., Citation2011). Aditionnaly, both the test manual (Wechsler, Citation2009) and various publications (e.g., Carlozzi et al., Citation2013; Hoelzle et al., Citation2011) provide convergent evidence for validity of the WMS-IV.
Dispersion profiles
To index IIV across dispersion profiles, we computed intraindividual standard deviations (ISDs) score, reflecting within-person performance fluctuations across the neuropsychological battery. Dispersion estimates were derived through a regression technique that computes ISDs from standardized test scores (Christensen et al., Citation1999; Halliday et al., Citation2018; Hultsch, MacDonald & Dixon, Citation2002). Normatively adjusted scores (i.e., from published test norms) were first converted into z-scores and regressed on age to control for group differences. Although normatively adjusted scores are corrected for age and sex, there were cognitive performance differences across age groups, with larger variances typically to be related to greater means (Stawski et al., Citation2019; Halliday et al., Citation2018). The resulting residuals for each test score were then transformed to T-scores (i.e., M = 50, SD = 10; Halliday et al., Citation2018) to eliminate negative values and facilitate interpretation. Subsequently, the standard deviation of three collections of residualized test scores was calculated, rendering an ISD for each of three dispersion profiles in each individual (i.e., three profiles per person); the composition of each profile is described in more detail below. The result was a common metric for dispersion. Each ISD indexed the amount of IIV across an individual’s three profiles relative to the group average level of performance. Higher values reflected greater IIV in neuropsychological test performance.
Three theoretically derived dispersion profiles were constructed using this residualization technique. Profiles were constrained by the existing RRMC test battery. The executive functioning profile consisted of seven scores: Number-Letter Switching from the Trail Making Test; Letter Fluency, Category Fluency, Category Switching Total, and Category Switching Accuracy from the Verbal Fluency Test; and Inhibition and Inhibition Switching from the Color-Word Interference Test. The immediate memory profile consisted of three scores: Logical Memory I from the WMS-IV; Total Immediate Recall from the BVMT-R; and the total of trials 1–4 from the CVLT-II SF. Lastly, the delayed memory profile consisted of three scores: Logical Memory II from the WMS-IV; Delayed Recall from the BVMT-R; and Long Delay Free Recall from the CVLT-II SF. Cued recall measures were not included in the profiles because they were deemed inappropriate estimates of target memory ability in this context.
Statistical analysis
A profile analysis was conducted using SPSS 26 to explore the domains of immediate memory, delayed memory, and executive functioning of AD, a-MCI, and SCI individuals. Profile analysis is a technique comparable to a repeated-measures analysis of variance (ANOVA; Biernat & Wortman, Citation1991) but for multiple dependent measures. The technique tested three main hypotheses (see Tabachnick & Fidell, Citation1996 for detailed treatment). Firstly, it examines whether the profiles of the dependent variables are parallel. This is analogous to the test for an interaction, between diagnostic grouping and dispersion within each cognitive domain. Next, it assesses whether the profiles are horizontal (the flatness hypothesis). This is comparable to a within-subjects main effect in repeated measures ANOVA which measures whether dispersion was similar across each cognitive domain. Finally, profile analysis probes whether profiles possess equal levels, allowing determination of whether groups are statistically different. When possible, the most robust statistic is reported (i.e., Pillai’s trace) in an effort to protect against assumption violations present (e.g., unequal cell size) in the clinical data (Pillai, Citation1955; Tabachnick & Fidell, Citation1996). Unequal cell size occurred as scores on all tests comprising the profiles were necessary for inclusion, and low completion rates of tests (e.g., executive tests; Schmitt et al., Citation2010) are common among dementia samples (Enright et al., Citation2015).
Results
When between-subjects effects from the profile analysis were examined, there was a significant difference in dispersion across the diagnostic groups averaged across all theoretical domains, F(2, 146) = 7.312, p = 0.001 (see ). This suggested a difference in levels (i.e., group membership), and post-hoc testing indicated that dispersion in delayed memory was lower than for immediate memory or executive function (F(2, 146) = 12.528, p < 0.001; see , results persisted after Bonferroni correction; Dunn, Citation1961). The profiles departed significantly from flatness across the domains of executive functioning, immediate memory and delayed memory, Pillai’s trace = 0.41, F(2, 145) = 3.114, p = 0.047 (see ), and the interaction between diagnostic classification and theoretical domain suggested different patterns of results for each level of cognitive classification, Pillai’s trace = 0.122, F(4, 292) = 4.733, p = 0.001 (see and ). All diagnostic groups demonstrated similar dispersion in their performance across tests measuring immediate memory and executive function. Persons with SCI did not differ in their dispersion scores across cognitive domains, but those with a-MCI and those with AD demonstrated less dispersion in their delayed memory performance, and those with AD demonstrated less dispersion in delayed memory relative to persons with a-MCI.
Figure 1. Visual representation of the mean ISD score across each profile indexed for SCI, a-MCI and AD individuals respectively. Error bars reflect the standard error.
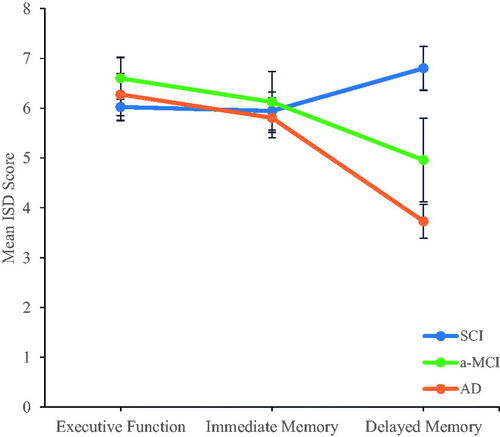
Table 3. Test of the effect of diagnostic classification averaged over executive functioning, immediate memory and delayed memory profiles; testing the levels hypothesis.
Table 4. Follow-up analysis to determine where the differences in dispersion profile levels occurred averaged across diagnostic classification employing a One-way ANOVA.
Table 5. Examination of the flatness and parallel hypothesis through profile analysis.
Discussion
The aim of the present study was to explore the dispersion profiles of individuals with AD, a-MCI, and SCI across a battery of neuropsychological tasks spanning executive functioning, immediate memory, and delayed memory. The delayed memory profile had less dispersion based on diagnosis, which is consistent with previous data demonstrating dissociation of episodic memory with progression from a-MCI to dementia (e.g., Mayes & Roberts, Citation2001). A probable explanation for our finding of reduced dispersion in delayed memory for the groups diagnosed with dementia due to AD and a-MCI is a floor effect within the neuropsychological battery. This can occur when a set of tests possess a distinct lower bound, and many participants score near this limit (Hessling, Schmidt, & Traxel, Citation2004). Suppression of variability in the AD profile was not surprising; individuals living with dementia tend to have lower scores on cognitive assessments, limiting variability (Drozdick et al., Citation2013) and likely increasing susceptibility to floor effects. Further, the relatively circumscribed a-MCI group also is typically expected to perform poorly on tests of delayed memory (e.g., free recall; Dubois & Albert, Citation2004) which would leave these individuals susceptible to variability suppression. Additional support for the occurrence of a floor effect in the test battery can be found from examining the mean z-scores for each neuropsychological test (). Here, a clear demonstration of low range performance permits little room for variability for the group living with AD. Although cognitive test scores were a part of the interdisciplinary diagnostic process, the dispersion estimate was not used in diagnosis, and may not have added additional diagnostic information in the current setting.
The finding of depressed dispersion due to likely floor effects for individuals with AD versus those with a-MCI or SCI supports prior work suggesting the value of IIV as a marker of impairment with population-based samples or large-scale initiatives such as the Kungsholman Project (MacDonald, et al., Citation2012). In this project, broad scale dispersion for those with AD was elevated, but suppressed variability was observed in episodic memory. In contrast, the Alzheimer’s Disease Neuroimaging Initiative (Tractenberg & Pietrzak, Citation2011) and results from the Berlin Aging study (Lövdén et al., Citation2007) report increases in IIV in relation to declines in cognitive function. These kinds of studies survey a large number of individuals lending increased power for the detection of nuanced variability. Further, the samples of older adults (e.g., Christensen et al., Citation1999; Halliday et al., Citation2018; Hilborn et al., Citation2009) are often not being investigated upon referral to a specialty memory clinic with suspected impairment. This implicates IIV and dispersion as potentially useful in non-specialty contexts, including identifying those at risk for developing a neurodegenerative disease or who are in the prodromal and early stages (Kälin et al., Citation2014).
We found dispersion similarities in immediate memory and executive functioning between SCI and a-MCI/AD groups which may hold meaning but are difficult to interpret without the use of a control group. It has been noted in previous investigations that IIV for apparently normal older adults has value in determining who will evidence pathologic decline in the future (Christensen et al., Citation1999; Holtzer et al., Citation2008; MacDonald et al., Citation2008). For instance, an investigation using the general public reported IIV changes on a reaction time task for some individuals with SCI at the within-group level (Jenkins et al., Citation2019). The authors suggest that for some with SCI, there is an association with slowed and/or more variable information processing which may indicate more burden on daily living. As increasing evidence suggests the presence of SCI may increase risk for dementia (e.g., Mitchell et al., Citation2014), it is pertinent to understand whether noted IIV changes in this group are relevant to incipient dementia. As such, future research would benefit from comparing individuals diagnosed with SCI in a clinical setting to normal controls to discern whether IIV changes in these individuals are a by-product of normal aging.
This analysis contains several limitations. Firstly, given that the dispersion indices were constructed from neuropsychological tests which helped inform each individual’s diagnosis, there is a degree of criterion contamination. It is important to stress that dispersion as an analytic method was not used in the diagnostic process, and neuropsychological testing was only one part of the diagnostic process. An external standard (i.e., a second neuropsychological battery; Bangen et al., Citation2019) would be required to avoid any form of criterion contamination. In addition, certain rates of dispersion would be expected based on the way the groups were classified (e.g., more truncated range in the AD group because of greater levels of impairment), but the present study only demonstrated reduced dispersion for delayed memory, not other assessed domains. It is possible, however, that the low number of tests contributing to the delayed memory domain contributed to the finding of reduced dispersion. The number of tests where dispersion was computed for the delayed memory domain was low compared to previous research (e.g., Bangen et al., Citation2019; De Felice & Holland, Citation2018). This leaves the possibility that group differences in memory dispersion were an artifact of the low number of contributing memory tests, but we would have expected to see this artifact demonstrated for all diagnostic groups and we did not. Additionally, the relatively small and unequal cell sizes contribute to a limitation of power to detect effects, particularly with respect to the a-MCI group. A small sample size could influence the size and reliability of the dispersion estimate and may have contributed to the larger standard error for the a-MCI group (). This could explain the lack of group differences in executive functioning. A final limitation is that our indices of memory are intertwined with language. Given that some linguistic change may also indicate the onset of a-MCI or AD (e.g., Emery, Citation2000) it is a possibility that different findings would emerge if the memory profiles relied more heavily on less language dependent memory tests.
Despite these limitations, this work has value. For instance, it explores whether dispersion related work should be pursued in clinical settings. It is a necessary precursor before clinicians can decide whether it would be worthwhile to undertake the investment of constructing an additional battery for an external standard of comparison as has been done in research settings (Bangen et al., Citation2019). It remains possible that dispersion related information holds use in the triangulation of clinical information (e.g., Campbell & Fiske, Citation1959). For example, is possible to conceptualize the suppression effect where there was a lack of dispersion for tests of delayed memory as an additional strand of convergent evidence for a clinical decision (e.g., a diagnosis of dementia).
Decline due to dementia is an issue of global importance understood to produce impairment in various domains of cognition such as memory and executive functioning, such that distinct profiles emerge (e.g., Stopford et al., Citation2007; Salmon, et al., Citation1999). The results of this study tentatively suggest that although these profiles are constructible in a clinical sample, they may have limited utility due to a floor effect. Nevertheless, the picture remains unclear whether dispersion is diagnostically useful beyond giving impressions of impairment versus no impairment in individuals subject to clinical neuropsychological assessment.
Disclosure statement
No potential conflict of interest was reported by the author(s).
Additional information
Funding
References
- Albert, M. S., DeKosky, S. T., Dickson, D., Dubois, B., Feldman, H. H., Fox, N. C., Gamst, A., Holtzman, D. M., Jagust, W. J., Petersen, R. C., Snyder, P. J., Carrillo, M. C., Thies, B., & Phelps, C. H. (2011). The diagnosis of mild cognitive impairment due to Alzheimer's disease: Recommendations from the National Institute on Aging-Alzheimer's Association workgroups on diagnostic guidelines for Alzheimer's disease . Alzheimer's & Dementia, 7(3), 270–279. https://doi.org/10.1016/j.jalz.2011.03.008
- Alzheimer’s Disease International. (2011). World Alzheimer report 2011. London.
- Alzheimer’s Society of Canada. (2016). Dementia numbers in Canada. Retrieved April 15, 2020, from http://www.alzheimer.ca/en/About-dementia/What-is-dementia/Dementia-numbers.
- Baddeley, A. D., Bressi, S., Della Sala, S., Logie, R., & Spinnler, H. (1991). The decline of working memory in Alzheimer's disease. A longitudinal study. Brain, 114(Pt 6), 2521–2542. https://doi.org/10.1093/brain/114.6.2521
- Baldo, J. V., Delis, D., Kramer, J., & Shimamura, A. P. (2002). Memory performance on the California Verbal Learning Test-II: Findings from patients with focal frontal lesions. Journal of the International Neuropsychological Society, 8(4), 539–546. https://doi.org/10.1017/s135561770281428x
- Bangen, K. J., Weigand, A. J., Thomas, K. R., Delano-Wood, L., Clark, L. R., Eppig, J., Werhane, M. L., Edmonds, E. C., & Bondi, M. W. (2019). Cognitive dispersion is a sensitive marker for early neurodegenerative changes and functional decline in nondemented older adults. Neuropsychology, 33(5), 599–608. https://doi.org/10.1037/neu0000532
- Bayer, A., Phillips, M., Porter, G., Leonards, U., Bompas, A., & Tales, A. (2014). Abnormal inhibition of return in mild cognitive impairment: Is it specific to the presence of prodromal dementia? Journal of Alzheimer's Disease, 40(1), 177–189. https://doi.org/10.3233/JAD-131934
- Becker, J. (1988). Working memory and secondary memory deficits in Alzheimer's disease. Journal of Clinical and Experimental Neuropsychology, 10(6), 739–753. https://doi.org/10.1080/01688638808402811
- Becker, J. T., Bajulaiye, O., & Smith, C. (1992). Longitudinal analysis of a two-component model of the memory deficit in Alzheimer's disease. Psychological Medicine, 22(2), 437–445. https://doi.org/10.1017/s0033291700030385
- Becker, J. T., Lopez, O. L., & Wess, J. (1992). Material-specific memory loss in probable Alzheimer's disease. Journal of Neurology, Neurosurgery, and Psychiatry, 55(12), 1177–1181. https://doi.org/10.1136/jnnp.55.12.1177
- Belanger, H. G., Kretzmer, T., Yoash-Gantz, R., Pickett, T., & Tupler, L. A. (2009). Cognitive sequelae of blast-related versus other mechanisms of brain trauma. Journal of the International Neuropsychological Society, 15(1), 1–8. https://doi.org/10.1017/S1355617708090036
- Bellgrove, M. A., Hester, R., & Garavan, H. (2004). The functional neuroanatomical correlates of response variability: Evidence from a response inhibition task. Neuropsychologia, 42(14), 1910–1916. https://doi.org/10.1016/j.neuropsychologia.2004.05.007
- Benedict, R. H. B. (1997). Brief Visuospatial Memory Test – Revised: Professional manual. Psychological Assessment Resources, Inc.
- Benedict, R. H. B., Schretlen, D., Groninger, L., Dobraski, M., & Shpritz, B. (1996). Revision of the Brief Visuospatial Memory Test: Studies of normal performance, reliability, and validity. Psychological Assessment, 8(2), 145–153. https://doi.org/10.1037/1040-3590.8.2.145
- Benedict, R. H. B., & Zivadinov, R. (2007). Reliability and validity of neuropsychological screening and assessment strategies in MS. Journal of Neurology, 254(S2), II22–II25. https://doi.org/10.1007/s00415-007-2007-4
- Bielak, A. A. M., Hultsch, D. F., Strauss, E., MacDonald, S. W. S., & Hunter, M. A. (2010). Intraindividual variability in reaction time predicts cognitive outcomes 5 years later. Neuropsychology, 24(6), 731–741. https://doi.org/10.1037/a0019802
- Biernat, M., & Wortman, C. B. (1991). Sharing of home responsibilities between professionally employed women and their husbands. Journal of Personality and Social Psychology, 60(6), 844–860. https://doi.org/10.1037//0022-3514.60.6.844
- Burton, C. L., Strauss, E., Hultsch, D. F., Moll, A., & Hunter, M. A. (2006). Intraindividual variability as a marker of neurological dysfunction: A comparison of Alzheimer's disease and Parkinson's disease. Journal of Clinical and Experimental Neuropsychology, 28(1), 67–83. https://doi.org/10.1080/13803390490918318
- Campbell, D. T., & Fiske, D. W. (1959). Convergent and discriminant validation by the multitrait-multimethod matrix. Psychological Bulletin, 56(2), 81–105.
- Carlozzi, N. E., Grech, J., & Tulsky, D. S. (2013). Memory functioning in individuals with traumatic brain injury: An examination of the Wechsler Memory Scale-Fourth Edition (WMS-IV). Journal of Clinical and Experimental Neuropsychology, 35(9), 906–914. https://doi.org/10.1080/13803395.2013.833178
- Carretero, S., Garces, J., Rodenas, F., & Sanjose, V. (2009). The informal caregiver's burden of dependent people: Theory and empirical review. Archives of Gerontology and Geriatrics, 49(1), 74–79. https://doi.org/10.1016/j.archger.2008.05.004
- Cherbuin, N., Sachdev, P., & Anstey, K. J. (2010). Neuropsychological predictors of transition from healthy cognitive aging to mild cognitive impairment: The PATH through life study. The American Journal of Geriatric Psychiatry, 18(8), 723–733. https://doi.org/10.1097/jgp.0b013e3181cdecf1
- Christensen, H., Mackinnon, A., Korten, A., Jorm, A., Henderson, A., & Jacomb, P. (1999). Dispersion in cognitive ability as a function of age: A longitudinal study of an elderly community sample. Aging, Neuropsychology, and Cognition (Neuropsychology, Development and Cognition: Section B), 6(3), 214–228. https://doi.org/10.1076/anec.6.3.214.779
- Costa, A. S., Dogan, I., Schulz, J. B., & Reetz, K. (2019). Going beyond the mean: Intraindividual variability of cognitive performance in prodromal and early neurodegenerative disorders. The Clinical Neuropsychologist, 33(2), 369–389. https://doi.org/10.1080/13854046.2018.1533587
- De Felice, S., & Holland, C. A. (2018). Intra-individual variability across fluid cognition can reveal qualitatively different cognitive styles of the aging brain. Frontiers in Psychology, 9, 1973. https://doi.org/10.3389/fpsyg.2018.01973
- Delis, D. C., Kaplan, E., & Kramer, J. H. (2001). Delis-Kaplan Executive Function System (D- KEFS). The Psychological Corporation.
- Delis, D. C., Kramer, J. H., Kaplan, E., & Holdnack, J. (2004). Reliability and validity of the Delis-Kaplan Executive Function System: An update. Journal of the International Neuropsychological Society, 10(2), 301–303. https://doi.org/10.1017/S1355617704102191
- Delis, D. C., Kramer, J. H., Kaplan, E., & Ober, B. A. (2000). The California Verbal Learning Test-Second Edition. Psychological Corporation.
- Delis, D. C., Wetter, S. R., Jacobson, M. W., Peavy, G., Hamilton, J., Gongvatana, A., Kramer, J. H., Bondi, M. W., Corey-Bloom, J., & Salmon, D. P. (2005). Recall discriminability: Utility of a new CVLT-II measure in the differential diagnosis of dementia. Journal of the International Neuropsychological Society, 11(6), 708–715. https://doi.org/10.1017/S1355617705050812
- Dixon, R. A., Garrett, D. D., Lentz, T. L., MacDonald, S. W. S., Strauss, E., & Hultsch, D. F. (2007). Neurocognitive markers of cognitive impairment: Exploring the roles of speed and inconsistency. Neuropsychology, 21(3), 381–399. https://doi.org/10.1037/0894-4105.21.3.381
- Donders, J., & Nienhuis, J. B. (2007). Utility of CVLT-II recall discriminability indices in the evaluation of traumatic brain injury. Journal of the International Neuropsychological Society, 13(02), 354–358. https://doi.org/10.1017/S1355617707070439
- Drozdick, L. W., Holdnack, J. A., & Hilsabeck, R. C. (2011). Essentials of WMS-IV Assessment (Vol. 85). John Wiley & Sons.
- Drozdick, L. W., Holdnack, J. A., Salthouse, T., & Cullum, C. M. (2013). Chapter 9: Assessing Cognition in older adults with the WAIS-IV, WMS-IV, and ACS. In J. A. Holdnack, L. W. Drozdick, L. G. Wiess, G. L. Iverson (Eds.), WAIS-IV, WMS-IV and ACS, advanced clinical interpretation (pp. 407–471). Academic Press.
- Dubois, B., & Albert, M. L. (2004). Amnestic MCI or prodromal Alzheimer's disease? The Lancet Neurology, 3(4), 246–248. https://doi.org/10.1016/S1474-4422(04)00710-0
- Dunn, O. J. (1961). Multiple comparisons among means. Journal of the American Statistical Association, 56(293), 52–64. https://doi.org/10.1080/01621459.1961.10482090
- Emery, V. O. B. (2000). Language impairment in dementia of the Alzheimer type: A hierarchical decline? The International Journal of Psychiatry in Medicine, 30(2), 145–164. https://doi.org/10.2190/X09P-N7AU-UCHA-VW08
- Enright, J., O'Connell, M., Mackinnon, S., & Morgan, D. (2015). Predictors of completion of executive-functioning tasks in a memory clinic dementia sample. Applied Neuropsychology. Adult, 22(6), 459–464. https://doi.org/10.1080/23279095.2014.992070
- Etters, L., Goodall, D., & Harrison, B. E. (2008). Caregiver burden among dementia patient caregivers: A review of the literature. Journal of the American Academy of Nurse Practitioners, 20(8), 423–428. https://doi.org/10.1111/j.1745-7599.2008.00342.x
- Gaugler, J., Kane, R., Kane, R., & Newcomer, R. (2005). Early community-based service utilization and its effects on institutionalization in dementia caregiving. The Gerontologist, 45(2), 177–185. https://doi.org/10.1093/geront/45.2.177
- Gauthier, S., Patterson, C., Chertkow, H., Gordon, M., Herrmann, N., Rockwood, K., Rosa-Neto, P., & Soucy, J.-P. (2012). Recommendations of the 4th Canadian Consensus Conference on the Diagnosis and Treatment of Dementia (CCCDTD4). Canadian Geriatrics Journal, 15(4), 120–126. https://doi.org/10.5770/cgj.15.49
- Gorus, E., De Raedt, R., Lambert, M., Lemper, J. C., & Mets, T. (2008). Reaction times and performance variability in normal aging, mild cognitive impairment, and Alzheimer's disease. Journal of Geriatric Psychiatry and Neurology, 21(3), 204–218. https://doi.org/10.1177/0891988708320973
- Halliday, D. W. R., Stawski, R. S., Cerino, E. S., DeCarlo, C. A., Grewal, K., & MacDonald, S. W. S. (2018). Intraindividual variability across neuropsychological tests: Dispersion and disengaged lifestyle increase risk for Alzheimer's disease. Journal of Intelligence, 6(1), 12. https://doi.org/10.3390/jintelligence6010012
- Haynes, B. I., Bauermeister, S., & Bunce, D. (2017). A systematic review of longitudinal associations between reaction time intraindividual variability and age-related cognitive decline or impairment, dementia, and mortality. Journal of the International Neuropsychological Society : JINS, 23(5), 431–445. 10.1017/S1355617717000236
- Hessling, R., Schmidt, T., & Traxel, N. (2004). Floor effect. In M. S. Lewis-Beck A. Bryman & T. F. Liao (Eds.), The SAGE encyclopedia of social science research methods (Vol. 1, pp. 391–391). SAGE Publications, Inc. https://doi.org/10.4135/9781412950589.n344
- Hilborn, J. V., Strauss, E., Hultsch, D. F., & Hunter, M. A. (2009). Intraindividual variability across cognitive domains: Investigation of dispersion levels and performance profiles in older adults. Journal of Clinical and Experimental Neuropsychology, 31(4), 412–424. https://doi.org/10.1080/13803390802232659
- Hoelzle, J. B., Nelson, N. W., & Smith, C. A. (2011). Comparison of Wechsler Memory Scale-Fourth Edition (WMS-IV) and Third Edition (WMS-III) dimensional structures: Improved ability to evaluate auditory and visual constructs. Journal of Clinical and Experimental Neuropsychology, 33(3), 283–291. https://doi.org/10.1080/13803395.2010.511603
- Holtzer, R., Verghese, J., Wang, C., Hall, C. B., & Lipton, R. B. (2008). Within-person across-neuropsychological test variability and incident dementia. JAMA, 300(7), 823–830. https://doi.org/10.1001/jama.300.7.823
- Hultsch, D. F., MacDonald, S. W. S., & Dixon, R. A. (2002). Variability in reaction time performance of younger and older adults. The Journals of Gerontology. Series B, Psychological Sciences and Social Sciences, 57(2), P101–115. https://doi.org/10.1093/geronb/57.2.p101
- Ismail, Z., Black, S. E., Camicioli, R., Chertkow, H., Herrmann, N., Laforce, R., Jr., Montero‐ Odasso, M., Rockwood, K., Rosa‐Neto, P., Seitz, D., Sivananthan, S., Smith, E. E., Soucy, J., Vedel, I., & Gauthier, S. (2020). Recommendations of the 5th Canadian Consensus Conference on the diagnosis and treatment of dementia. Alzheimer's & Dementia, 16(8), 1182–1195. https://doi.org/10.1002/alz.12105
- Jenkins, A., Tree, J. J., Thornton, I. M., & Tales, A. (2019). Subjective cognitive impairment in 55-65-year-old adults is associated with negative affective symptoms, neuroticism, and poor quality of life. Journal of Alzheimer's Disease, 67(4), 1367–1378. https://doi.org/10.3233/JAD-180810
- Jessen, F., Amariglio, R. E., van Boxtel, M., Breteler, M., Ceccaldi, M., Chételat, G., Dubois, B., Dufouil, C., Ellis, K. A., van der Flier, W. M., Glodzik, L., van Harten, A. C., de Leon, M. J., McHugh, P., Mielke, M. M., Molinuevo, J. L., Mosconi, L., Osorio, R. S., Perrotin, A., … Wagner, M. (2014). A conceptual framework for research on subjective cognitive decline in preclinical Alzheimer's disease. Alzheimer's & Dementia, 10(6), 844–852. https://doi.org/10.1016/j.jalz.2014.01.001
- Kälin, A. M., Pflüger, M., Gietl, A. F., Riese, F., Jäncke, L., Nitsch, R. M., & Hock, C. (2014). Intraindividual variability across cognitive tasks as a potential marker for prodromal Alzheimer’s disease. Frontiers in Aging Neuroscience, 6, 147. https://doi.org/10.3389/fnagi.2014.00147
- Kane, K. D., & Yochim, B. P. (2014). Construct validity and extended normative data for older adults for the Brief Visuospatial Memory Test, Revised. American Journal of Alzheimer's Disease and Other Dementias, 29(7), 601–606. 10.1177/1533317514524812
- Kim, M.-Y., Park, J.-H., Kim, J. B., & Park, H. Y. (2017). Relationship between executive function components and activities of daily living in elderly with mild dementia. Archives of Physical Medicine and Rehabilitation, 98(10), E100–E101. https://doi.org/10.1016/j.apmr.2017.08.324
- Kochan, N. A., Bunce, D., Pont, S., Crawford, J. D., Brodaty, H., & Sachdev, P. S. (2016). Reaction time measures predict incident dementia in community-living older adults: The Sydney Memory and Ageing Study. The American Journal of Geriatric Psychiatry, 24(3), 221–231. https://doi.org/10.1016/j.jagp.2015.12.005
- Logie, R. H., Cocchini, G., Delia Sala, S., & Baddeley, A. D. (2004). Is there a specific executive capacity for dual task coordination? Evidence from Alzheimer's disease. Neuropsychology, 18(3), 504–513. https://doi.org/10.1037/0894-4105.18.3.504
- Lövdén, M., Li, S.-C., Shing, Y. L., & Lindenberger, U. (2007). Within-person trial-to-trial variability precedes and predicts cognitive decline in old and very old age: Longitudinal data from the Berlin Aging Study. Neuropsychologia, 45(12), 2827–2838. https://doi.org/10.1016/j.neuropsychologia.2007.05.005
- Lövdén, M., Schmiedek, F., Kennedy, K. M., Rodrigue, K. M., Lindenberger, U., & Raz, N. (2013). Does variability in cognitive performance correlate with frontal brain volume? NeuroImage, 64, 209–215. https://doi.org/10.1016/j.neuroimage.2012.09.039
- MacDonald, S., Brewster, P., Laukka, E., Fratiglioni, L., & Bäckman, L. (2012). Intraindividual variability across neuropsychological tasks is associated with risk of Alzheimer’s disease. Alzheimer's & Dementia, 8(4S_Part_10), P370–P370. https://doi.org/10.1016/j.jalz.2012.05.1016
- MacDonald, S. W., Hultsch, D. F., & Dixon, R. A. (2008). Predicting impending death: Inconsistency in speed is a selective and early marker. Psychology and Aging, 23(3), 595–607. https://doi.org/10.1037/0882-7974.23.3.595
- MacDonald, S. W., Nyberg, L., & Bäckman, L. (2006). Intra-individual variability in behavior: Links to brain structure, neurotransmission and neuronal activity. Trends in Neurosciences, 29(8), 474–480. https://doi.org/10.1016/j.tins.2006.06.011
- MacDonald, S. W. S., Li, S.-C., & Bäckman, L. (2009). Neural underpinnings of within-person variability in cognitive functioning. Psychology and Aging, 24(4), 792–808. https://doi.org/10.1037/a0017798
- MacDonald, S. W. S., & Stawski, R. S. (2015). Intraindividual variability—An indicator of vulnerability or resilience in adult development and aging? In M. Diehl, K. Hooker, & M. J. Sliwinski (Eds.), Handbook of intraindividual variability across the life span. Routledge. https://doi.org/10.4324/9780203113066.ch13
- MacDonald, S. W. S., & Stawski, R. S. (2020). Longitudinal changes in response time mean and inconsistency exhibit predictive dissociations for risk of cognitive impairment. Neuropsychology, 34(3), 264–275. https://doi.org/10.1037/neu0000608
- Marshall, G. A., Rentz, D. M., Frey, M. T., Locascio, J. J., Johnson, K. A., & Sperling, R. A. (2011). Executive function and instrumental activities of daily living in mild cognitive impairment and Alzheimer’s disease. Alzheimer's & Dementia, 7(3), 300–308. https://doi.org/10.1016/j.jalz.2010.04.005
- Mayes, A. R., & Roberts, N. (2001). Theories of episodic memory. Philosophical Transactions of the Royal Society of London. Series B, Biological Sciences, 356(1413), 1395–1408. https://doi.org/10.1098/rstb.2001.0941
- McCloskey, G., Perkins, L., & Divner, B. (2009). Assessment and intervention for executive function difficulties. Routledge Taylor & Francis Group.
- McCloskey, R., Jarrett, P., Stewart, C., & Nicholson, P. (2014). Alternate level of care patients in hospitals: What does dementia have to do with this? Canadian Geriatrics Journal, 17(3), 88–94. https://doi.org/10.5770/cgj.17.106
- McLaughlin, P. M., Borrie, M. J., & Murtha, S. J. (2010). Shifting efficacy, distribution of attention and controlled processing in two subtypes of mild cognitive impairment: Response time performance and intraindividual variability on a visual search task. Neurocase, 16(5), 408–417. https://doi.org/10.1080/13554791003620306
- Mella, N., Fagot, D., & de Ribaupierre, A. (2016). Dispersion in cognitive functioning: Age differences over the lifespan. Journal of Clinical and Experimental Neuropsychology, 38(1), 111–126. https://doi.org/10.1080/13803395.2015.1089979
- Mella, N., Fagot, D., Lecerf, T., & Ribaupierre, A. D. (2015). Working memory and intraindividual variability in processing speed: A lifespan developmental and individual-differences study. Memory & Cognition, 43(3), 340–356. https://doi.org/10.3758/s13421-014-0491-1
- Mitchell, A. J., Beaumont, H., Ferguson, D., Yadegarfar, M., & Stubbs, B. (2014). Risk of dementia and mild cognitive impairment in older people with subjective memory complaints: Meta-analysis. Acta Psychiatrica Scandinavica, 130(6), 439–451. https://doi.org/10.1111/acps.12336
- Monsch, A., Bondi, M., Butters, N., Paulsen, J., Salmon, D., Brugger, P., & Swenson, M. (1994). A comparison of category and letter fluency in Alzheimer’s disease and Huntingdon’s disease. Neuropsychology, 8(1), 25–30. https://doi.org/10.1037/0894-4105.8.1.25
- Morgan, D. G., Crossley, M., Kirk, A., D'Arcy, C., Stewart, N., Biem, J., Forbes, D., Harder, S., Basran, J., Dal Bello-Haas, V., & McBain, L. (2009). Improving access to dementia care: Development and evaluation of a rural and remote memory clinic. Aging & Mental Health, 13(1), 17–30. https://doi.org/10.1080/13607860802154432
- Murphy, K. J., Rich, J. B., & Troyer, A. K. (2006). Verbal fluency patterns in amnestic mild cognitive impairment are characteristic of Alzheimer’s type dementia. Journal of the International Neuropsychological Society, 12(4), 570–574. https://doi.org/10.1017/S1355617706060590
- Murtha, S., Cismaru, R., Waechter, R., & Chertkow, H. (2002). Increased variability accompanies frontal lobe damage in dementia. Journal of the International Neuropsychological Society, 8(3), 360–372. https://doi.org/10.1017/s1355617702813170
- Peña-Casanova, J., Sánchez-Benavides, G., de Sola, S., Manero-Borrás, R. M., & Casals-Coll, M. (2012). Neuropsychology of Alzheimer's disease. Archives of Medical Research, 43(8), 686–693. https://doi.org/10.1016/j.arcmed.2012.08.015
- Phillips, M., Rogers, P., Haworth, J., Bayer, A., & Tales, A. (2013). Intra-individual reaction time variability in mild cognitive impairment and Alzheimer's disease: Gender, processing load and speed factors. PLoS One, 8(6), e65712. https://doi.org/10.1371/journal.pone.0065712
- Pillai, K. C. S. (1955). Some new test criteria in multivariate analysis. The Annals of Mathematical Statistics, 26(1), 117–121. https://doi.org/10.1214/aoms/1177728599
- Rapp, M. A., Schnaider-Beeri, M., Sano, M., Silverman, J. M., & Haroutunian, V. (2005). Cross-domain variability of cognitive performance in very old nursing home residents and community dwellers: Relationship to functional status. Gerontology, 51(3), 206–212. https://doi.org/10.1159/000083995
- Salmon, D. P., Heindel, W. C., & Lange, K. L. (1999). Differential decline in word generation from phonemic and semantic categories during the course of Alzheimer's disease: Implications for the integrity of semantic memory. Journal of the International Neuropsychological Society, 5(7), 692–703. https://doi.org/10.1017/s1355617799577126
- Schmitt, A. L., Livingston, R. B., Smernoff, E. N., Waits, B. L., Harris, J. B., & Davis, K. M. (2010). Dichotomous scoring of Trails B in patients referred for a dementia evaluation. Perceptual and Motor Skills, 110(2), 429–441. https://doi.org/10.2466/PMS.110.2.429-441
- Shepard, R. N. (1967). Recognition memory for words, sentences, and pictures. Journal of Verbal Learning and Verbal Behavior, 6(1), 156–163. https://doi.org/10.1016/S0022-5371(67)80067-7
- Shunk, A. W., Davis, A. S., & Dean, R. S. (2006). TEST REVIEW: Dean C. Delis, Edith Kaplan & Joel H. Kramer, Delis Kaplan Executive Function System (D-KEFS), The Psychological Corporation, San Antonio, TX, 2001. $415.00 (complete kit). Applied Neuropsychology, 13(4), 275–227. https://doi.org/10.1207/s15324826an1304_9
- Stawski, R. S., MacDonald, S. W., Brewster, P. W., Munoz, E., Cerino, E. S., & Halliday, D. W. (2019). A comprehensive comparison of quantifications of intraindividual variability in response times: A measurement burst approach. The Journals of Gerontology. Series B, Psychological Sciences and Social Sciences, 74(3), 397–408. https://doi.org/10.1093/geronb/gbx115
- Stopford, C. L., Snowden, J. S., Thompson, J. C., & Neary, D. (2007). Distinct memory profiles in Alzheimer's disease. Cortex, 43(7), 846–857. https://doi.org/10.1016/S0010-9452(08)70684-1
- Strauss, E., Bielak, A. A. M., Bunce, D., Hunter, M. A., & Hultsch, D. F. (2007). Within-person variability in response speed as an indicator of cognitive impairment in older adults. Neuropsychology, Development, and Cognition. Section B, Aging, Neuropsychology and Cognition, 14(6), 608–630. https://doi.org/10.1080/13825580600932419
- Strauss, E., Sherman, E. M. S., & Spreen, O. (2006). A compendium of neuropsychological tests: Administration, norms, and commentary (3rd ed.). Oxford University Press.
- Strober, L., Englert, J., Munschauer, F., Weinstock-Guttman, B., Rao, S., & Benedict, R. H. B. (2009). Sensitivity of conventional memory tests in multiple sclerosis: comparing the Rao Brief Repeatable Neuropsychological Battery and the Minimal Assessment of Cognitive Function in MS. Multiple Sclerosis (Houndmills, Basingstoke, England), 15(9), 1077–1084. 10.1177/1352458509106615
- Swanson, J. (2005). The Delis-Kaplan executive function system: A review. Canadian Journal of School Psychology, 20(1–2), 117–128. https://doi.org/10.1177/0829573506295469
- Tabachnick, B. G., & Fidell, L. S. (1996). Using multivariate statistics. Harper Collins College Publ.
- Tales, A., Leonards, U., Bompas, A., Snowden, R. J., Philips, M., Porter, G., Haworth, J., Wilcock, G., & Bayer, A. (2012). Intra-individual reaction time variability in amnestic mild cognitive impairment: A precursor to dementia? Journal of Alzheimer's Disease, 32(2), 457–466. https://doi.org/10.3233/JAD-2012-120505
- Tales, A., Wilcock, G. K., Phillips, J. E., & Bayer, A. (2014). Is there more to subjective cognitive impairment than meets the eye? A perspective. Journal of Alzheimer's Disease, 41(3), 655–661. https://doi.org/10.3233/JAD-132414
- Tractenberg, R. E., & Pietrzak, R. H. (2011). Intra-Individual Variability in Alzheimer's disease and cognitive aging: Definitions, context, and effect sizes. PLoS One, 6(4), e16973. https://doi.org/10.1371/journal.pone.0016973
- Troyer, A. K., Vandermorris, S., & Murphy, K. J. (2016). Intraindividual variability in performance on associative memory tasks is elevated in amnestic mild cognitive impairment. Neuropsychologia, 90, 110–116. https://doi.org/10.1016/j.neuropsychologia.2016.06.011
- Wechsler, D. (2009). Wechsler Memory Scale-Fourth Edition (WMS-IV): Technical and interpretive manual. Pearson.
- Wecker, N. S., Kramer, J. H., Wisniewski, A., Delis, D. C., & Kaplan, E. (2000). Age effects on executive ability. Neuropsychology, 14(3), 409–414. https://doi.org/10.1037//0894-4105.14.3.409
- Welsh, K., Butters, N., Hughes, J., Mohs, R., & Heyman, A. (1991). Detection of abnormal memory decline in mild cases of Alzheimer's disease using CERAD neuropsychological measures. Archives of neurology, 48(3), 278–281. https://doi.org/10.1001/archneur.1991.00530150046016
- Woods, S., Delis, D., Scott, J., Kramer, J., & Holdnack, J. (2006). The California Verbal Learning Test-second edition: Test-retest reliability, practice effects, and reliable change indices for the standard and alternate forms. Archives of Clinical Neuropsychology, 21(5), 413–420. https://doi.org/10.1016/j.acn.2006.06.002
- Zarit, S. H., Reever, K. E., & Bach-Peterson, J. (1980). Relatives of the impaired elderly: Correlates of feelings of burden. The Gerontologist, 20(6), 649–655. https://doi.org/10.1093/geront/20.6.649