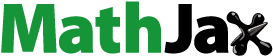
ABSTRACT
Uncontrolled hazardous wastes sites have the potential to adversely impact human health and damage or disrupt ecological systems and the greater environment. Four decades have passed since the Superfund law was enacted, allowing increased exposure time to these potential health hazards while also allowing advancement of analysis techniques. Florida has the sixth highest number of Superfund sites in the US and, in 2016, Florida was projected to have the second largest number of new cancer cases in the US. We explore statewide cancer incidence in Florida from 1986 to 2010 to determine if differences or associations exist in counties containing Superfund sites compared to counties that do not. To investigate potential environmental associations with cancer incidence; results using spatial and nonspatial mixed models were compared. Using a Poisson–Gamma mixture model, our results provide some evidence of an association between cancer incidence rates and Superfund site hazard levels, as well as proxy measures of water contamination around Superfund sites. In addition, results build upon previously observed gender differences in cancer incidence rates and further indicate spatial differences for cancer incidence. Heterogeneity among cancer incidence rates were observed across Florida with some mild association with Superfund exposure proxies.
Introduction
In the past 20 years, spatial analysis techniques have greatly advanced. The software capabilities for these techniques have greatly expanded as well. These new analytic tools allow more precise estimation methods and analyses that produce higher quality modeling results. In particular, these advanced techniques can be used to improve upon the existing body of literature that investigates associations between cancer incidence and environmental exposures. Most of the previous research investigating these relationships was conducted in the 1980s and early 1990s. Only a few recent studies have further explored these relationships, none of which have investigated nonpediatric cancer incidence. In addition, a longer time period of recorded cancer incidence and exposure data allow better characterization of exposures for the known long latency period prior to cancer incidence (Nadler Citation2014). Additional historical data and current advanced analysis techniques available to researchers provides an opportunity to restudy these relationships and consider trends over a longer time period.
When considering adverse environmental exposures, “Superfund” is probably one of the most recognized terms to describe the scale and extent of these exposure types. On December 11, 1980, U.S. President Jimmy Carter signed into law the Comprehensive Environmental Response, Compensation and Liability Act (CERCLA), known informally as Superfund. Once enacted, the Superfund law provided the U.S. Environmental Protection Agency (EPA) the legal authority to conduct clean-up efforts for uncontrolled hazardous waste sites and spills (EPA Citation2014). According to the EPA (Citation2014), these sites include those “abandoned, accidentally spilled, and illegally dumped hazardous wastes that are determined to pose current or future threats to humans or the environment.” Long-term remediation efforts are also authorized by the Superfund law and conducted in areas where hazardous waste release occurred “through years of inadequate or illegal waste management” (EPA Citation2014).
There are multiple environmental factors to cancer risk and these include indoor and outdoor air pollution, soil contamination, and drinking water contamination (Boffetta and Nyberg Citation2003). In contrast to the large amount of work on air pollution and health risk, particularly lung cancer (Raaschou-Nielsen et al. Citation2013; Hamra et al. Citation2014), our focus for this study is on soil contamination and drinking water contamination exposures, common avenues of exposure for hazardous wastes, using Superfund sites as the environmental factor of interest. A comprehensive review of the literature on implications of hazardous waste was published (Johnson and DeRosa Citation1997) and concluded that in spite of inherent study limitations, some studies have detected associations between cancer and exposure to materials similar to those that are found at hazardous waste sites. Older studies from the 1980s found increased frequency of gastrointestinal, esophageal, stomach, colon, lung, bladder, large intestine, and rectal cancers in counties containing hazardous waste sites (Najem et al. Citation1983; Budnick et al. Citation1984; Griffith et.al. Citation1989). More current studies have continued to explore cancer incidence or other health impacts of hazardous waste exposure on the national or local level (Johnson Citation1995; Davies Citation2006; Florida Department of Health Citation2012a). Statewide cancer impacts and hazardous waste site exposure in Florida have previously been explored in one study, which focused on childhood cancers; results indicated some evidence of spatial clustering focused within 5–10 miles (8–16 km) of Superfund sites and not in areas in closer proximity to the Superfund site, although health data were aggregated to the geographic centroid of each census tract and may not reflect the real cluster location (Kearney Citation2008). A series of studies have been published that explore the pediatric cancer incidence in Florida and potential spatial clustering (Heaton Citation2014; Amin et al. Citation2014; Wang and Rodríguez Citation2014; Lawson and Rotejanaprasert Citation2014; Zhang, Lim, and Maiti Citation2014). For this series of studies, data were obtained from the Florida Association of Pediatric Tumor Program (FAPTR) and explored only pediatric onset cancers. Results indicate evidence of spatial clustering of pediatric cancer incidence; however different areas were identified as potential clusters across studies. Further, in Heaton (Citation2014), spatial differences in pediatric cancer incidence were observed by race but this was not observed in the other studies in this series.
Attributing pediatric cancers to environmental causes is difficult because pediatric cancer is rare but also because the causal linkage between environmental exposure pediatric cancer is unclear (National Cancer Institute Citation2016). However, environmental causes can be considered for adult cancers because the older age supports the longer latency needed. Nadler and Zurbenko (Citation2014) provided a nice description of the approximate latency period from cancer initiation to diagnosis for 34 cancer types. Cancer latency periods range from 2.2 years for chronic lymphocytic leukemia to 56.8 years for ascending colon cancer. Unfortunately because location history is problematic to obtain, inference is still difficult.
Uncontrolled hazardous waste sites have the potential to adversely impact human health and damage or disrupt ecological systems and the greater environment. Specifically, improperly stored or damaged containers of hazardous wastes can contribute to overall toxic exposures through the food chain or through intakes as part of a water supply for human or agricultural consumption (Hendryx et al. Citation2012). The reasons for identifying potential Superfund sites are those designated by the Superfund law as (i) either causing or contributing to an increase in mortality or irreversible or incapacitating illness, (ii) posing a substantial present or potential hazard to human health, or (iii) when materials hazardous to humans or to the environment were improperly treated, stored, transported, disposed of, or otherwise managed (TOXMAP Citation2012a). More than 800 materials are designated as hazardous and nearly 100 additional materials are identified as potentially hazardous (TOXMAP Citation2012b, Citation2012c).
Four decades have passed since the Superfund law was enacted, which has allowed for more historical data to be collected regarding these potential health hazards. During this same time frame, analysis techniques—particularly geographic information system techniques (GIS)—have advanced and allow a more detailed investigation of these relationships. In this study, the association between all-cause cancer incidence in Florida and locations of Superfund sites are explored, using proxy measures of exposure to hazardous chemicals and various demographic variables as predictors.
Methods
Data
A publically available information database for cancer incidence in Florida, called the STAT CD, was requested from the Florida Cancer Data System (FCDS) and available demographic covariates were age, sex, and county of residence (FCDS (Florida Cancer Data System) Citation2012). Because of the overwhelming white and non-Hispanic cancer incidence numbers in these data (>90% for both), these analyses did not separate out race and ethnicity demographics. Pediatric cancers were excluded from analysis because of the likely nonenvironmental cause; it is widely believed that children would not have the needed prolonged exposure to environmental toxins or chemicals to cause environmentally induced cancers (National Cancer Institute Citation2016). Age at the time of cancer incidence was categorized into groups, while, due to identifiability concerns, diagnosis year was grouped into 5-year intervals for the years between 1986 and 2010, inclusive. Due to privacy concerns and limited data availability, only county-level information was considered in this manuscript. University of Florida Health Science Center and University of Missouri Institution Review Board approval was obtained and nearly 2.4 million records were used for analysis ( and ).
Table 1. Raw total, all-cause cancer incidence counts for Florida from 1986 to 2010, in 5-year intervals and all-cause cancer incidence rates using population totals averaged across the 5-year intervals.
Table 2. Raw total, all-cause cancer incidence counts by age grouping and gender, 1986–2010. Total Rate refers to the rate per 100,000.
Table 3. Direct adjusted cancer incidence rates per 100,000 people, adjusted by age and gender, for each Florida county.
We adjust the raw, total, all-cause cancer incidence counts to account for the total Florida population. There are two main methods of adjustments: the direct method and the indirect method (Naing Citation2000). The direct method of adjustment applies strata (here the 5-year intervals or age groups) observed in the population to a reference or standard population, here the 2000 US Census numbers for comparison to the Florida Cancer Data System rates (Florida Cancer Data System Citation2016). The direct method for adjustment results in the number of expected cancers in the reference population. The indirect method for adjustment is similar but observed rates are compared to the populations of interest, to result in the observed number of deaths in each population of interest. The direct method was used here to compare rates easily to results from the Florida Cancer Data System ().
We aggregated data for Florida Superfund sites and hazards (TOXMAP Citation2012a), Florida shape files for GIS techniques (U.S. Census Bureau Citation2008), socioeconomic status data (Florida CHARTS Citation2015), census data (U.S. Census Bureau Citation2008; Florida Department of Economic and Demographic Research Citation2016), and data from water reservoirs (ESRI Citation2012).
Before any hazardous waste site can be classified as a Superfund site, it must first be placed on the National Priorities List (NPL) in the U.S. Federal Register. To be placed on the NPL, sites contaminated by hazardous wastes must be proposed by the EPA, the state in which the site is located, or concerned citizens. Once placed on the NPL, site status is designated as “Proposed,” “Deleted,” and “Final.” The EPA identifies proposed sites as candidates for cleanup activities because they pose a risk to human health and/or the environment. Deleted sites are those that have been deleted from the NPL by the EPA, with state concurrence. Sites are given deleted status when cleanup goals have been met and no further hazardous waste response is necessary. Final sites are those determined to pose a real or potential threat to human health and the environment. Final status is received after completion of the hazardous ranking score screening—a method that triages hazardous waste sites—and public solicitation of comments about the proposed site. For these analyses, the number of proposed and final Superfund sites in each county was compiled and included as one exposure variable to indicate a higher hazard for those that have not been remediated. In addition, the number of deleted Superfund sites in each county was also included, as a method to determine if there may be an association with cancer incidence and either previous exposure or continued exposure after remediation. We assume that possible confounding influences that contribute to cancer incidence are relatively homogenous across Florida.
Seventy-seven Superfund sites with a final, proposed, or deleted status are located in the state of Florida in 22 different counties (). The EPA defines a hazardous ranking score (HRS) using a numerical value from 0 to 100, with larger values indicating a larger potential health hazard to humans or the environmental. The HRS is assigned by the EPA to each Superfund site, regardless of site status, and characterizes the combination of the potential hazard of the location, the possibility that a site has released or has the potential to release hazardous substances into the environment, characteristics of the waste (e.g., toxicity and waste quantity), and people or sensitive environments that could be affected by a release. Exposures to hazardous wastes for each Florida county were assigned using ordinary kriging of the HRS. Ordinary kriging (OK) is a method that is widely used for prediction of geostatistical data and assumes a constant mean in the neighborhood of each prediction. Values are predicted based on the known values at collected points. The reader is referred to supplementary materials for more details on OK. Ordinary kriging produces spatial predictions of the HRS and these predictions were located at each county's centroid. For the spatial models, these spatial predictions were used as the HRS value for each county (). For nonspatial models, total county-level HRS was computed by summing each HRS score for all Superfund sites located in a county. Both of these measures can be considered a proxy for the level of hazard associated with Superfund site(s) within and around each county.
Figure 1. (a) Locations of Superfund sites in Florida with the spatial prediction for county-level hazardous ranking score (HRS). (b) Locations of surface water reservoirs and National Weather Service (NWS) regions in Florida.
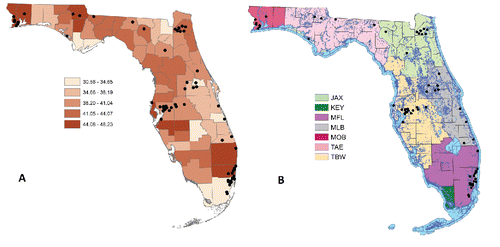
An important factor when considering hazardous waste and toxic materials is their ability to travel from the original waste site; one such method of travel is through water sources (EPA Citation1992). Because of the higher potential for hazardous wastes in Florida to travel through water—due to Florida's swampy, wet environment—the proportion of surface water contained within a 1 km area around each Superfund site was calculated and rescaled. As shown in , most Superfund sites (black dots) in Florida have some type of surface water reservoir located nearby (blue areas). For Superfund sites near county lines, this proportion of surface water was considered part of the county in which the Superfund site was located. The aggregated rescaled proportion for all Superfund sites located within a county's borders was used as the proxy for water exposure of hazardous wastes for the entire county.
Nonspatial Models
Cancer incidence counts were modeled using a Poisson–Gamma mixture model. The Poisson–Gamma mixture model is equivalent to the negative binomial generalized linear model and allows a more flexible model fit because it accounts for overdispersion. The negative binomial model is formulated as a mixture of the Poisson and gamma distributions and has the following form:
where Y is the response of interest that can either be count data or rate data. Models with count data must include an offset term with total population counts; this ensures that the counts are scaled to the total counts. To ensure the required flexibility for comparison to the standard Poisson model, Z is assumed to have a gamma distribution as previously defined.
As defined, the marginal distribution of Y has the probability mass function:
(1)
(1)
For integer values α = m, the mass function (1) simplifies to the negative binomial mass function:
For fixed m (or α), the model follows the standard generalized linear equation framework with the link function, g(E[Y]). The linear predictor has the form η = g(E[Y]) = XTβ, where XT is the transposed vector of predictors and β is the vector of model coefficients. For arbitrary α, the mean and variance have the form:
This model was fit (see the supplementary materials) using a population offset from the 2000 U.S. Census (U.S. Census Bureau), and thus all interpretations will be in terms of cancer incidence rates. Using the 2000 U.S. Census numbers, rather than the 2010 U.S. Census numbers, was intended to provide uniform comparison to the Florida Cancer Data System rates, which use the 2000 U.S. Census numbers (Florida Cancer Data System Citation2016). For the nonspatial analysis, the 67 counties within Florida could not be used as a spatial proxy due to the large number of counties, which when included as an indicator variable, produced model instability due to the large number of parameters. However, the National Weather Service (NWS) defines seven different weather regions within Florida, which are defined using county boundaries within Florida (). These NWS regions were used as spatial proxies in all nonspatial analyses and are composed of the Jacksonville region (JAX), Keys region (KEY), Miami region (MFL), Melbourne region (MLB), Mobile region (MOB), Tallahassee region (TAE), and the Tampa Bay region (TBW). The summary information for Superfund site locations by NWS region is provided in .
Table 4. Superfund status by NWS region and aggregated HRS.
Categorical covariates included in the nonspatial model were age group, gender, diagnosis period, presence of Superfund indicator, and NWS region. Continuous predictor and covariates were median income, the number of proposed and final Superfund sites, number of deleted Superfund sites, total county-level HRS, and county-level water exposure. Median income was used to control the potential confounding from socioeconomic status. Demographic covariates, such as gender and age group, were included to account for the known differences in cancer incidence by gender and age (e.g., cancer incidence increases for older age groups). Diagnosis period was also included in the analysis as it can be considered a measure of latency or proxy for medical care during this large time frame.
Spatial Models
A spatial simultaneous autoregressive error model (SAR Error Model) was used for spatial modeling. These model equations had the form:
Or equivalently,
Here, W was the matrix of the boundary weights, Y was the response vector, X is the matrix of predictors, I is the identity matrix, and ε is the vector of normally distributed errors. The parameters λ and β were estimated (see supplementary materials). The county-level spatial structure for Florida was described using a boundary matrix W of dimension 67 by 67 representing the number of Florida counties—using two different types of spatial weights to represent the spatial relationships between counties: (1) binary 0-1 queen weights and (2) standardized queen weights. Binary 0-1 queen weights for a given county are stored in a single row of the weights matrix. The weight value is equal to 1 if counties have at least one shared boundary point and 0 otherwise. Standardized queen weights are obtained from binary 0-1 queen weights by dividing each element in the row by the sum of all elements in that row. The individual elements of W are denoted by wij for i = 1, 2, …, N and j = 1, 2, …, N where N = 67 corresponds to the number of counties in Florida. Since spatial models are constructed so that only a single rate can be used for each spatial element (here, county), age and diagnosis year were included in these spatial models by adjusting the cancer incidence rates for each county by both age and diagnosis year, using US Census Data for Florida from 2000 as previously discussed. County-level exposure variables included in the models included the ordinary kriged HRS, county-level water exposure, the number of proposed and final Superfund sites for the given county and the number of deleted Superfund sites.
To investigate spatial heterogeneity, the Global Moran's I statistic was calculated for the spatial models to determine the presence of any spatial autocorrelation (spatial relationship) between the cancer incidence rate and county. Global Moran's I is sensitive to global spatial autocorrelation and has the form:
Here, Xi arethe values for the spatial objects and wij are the weights from the 67 × 67 weight matrix, W, for i = 1, 2, …, 67 and j = 1, 2, …, 67. The value corresponds to the average value of Xi across all spatial elements, that is,
. The reader is referred to supplementary materials for more details on the Global Moran's I statistic.
Although similar to the Global Moran's I, Geary's C statistic was also used to focus on relationships at the local level. The statistic has the form
The reader is referred to supplementary materials for more details on Geary's C statistic.
To further investigate the observed spatial relationships, local Getis-Ord general G and local Moran's I tests were conducted. Local Gettis-Ord Gi are used to identify clusters with high and low values. Local Gettis-Ord Gi statistics for each spatial object i have the form:
Anselin Local Moran's Ii statistics were introduced to detect local spatial autocorrelation. The formula for each spatial object i has the form:
The local Getis-Ord general G tests for local clustering of cancer incidence rates will indicate any difference from expected cancer incidence rates. In addition, the Local Moran's I statistic helps to identify if a county has similarly high or low cancer incidence rates with the surrounding counties and can be helpful to indicate potential clusters of either high or low cancer incidence rates. Used together with Getis-Ord general G, results can identify potential clusters and cancer incidence rates that could be considered “outliers” compared to rates in surrounding counties. The reader is referred to the supplementary materials for more details on Local Gettis-Ord Gi and Anselin Local Moran's Ii statistics.
Results
Results from both the spatial and nonspatial models indicated that spatial differences are observed in adult cancer incidence in Florida from 1986–2010. Further, nonspatial models indicated that increased county-level HRS, county-level water exposure for hazardous wastes and being male were associated with increased rates for adult cancer incidence.
Nonspatial Models
The nonspatial model fit results are provided in . In the nonspatial Poisson–Gamma mixture model using NWS regions as spatial proxies, each age group category was observed to be significantly associated with cancer incidence (p < 0.010). This significance reflects the higher cancer incidence observed as a population ages. Being female was inversely associated with cancer incidence, with p value equal to 0.0508, indicating a potential protective effect against cancer incidence for females, or reduced exposure. Of the exposure variables, county-level water exposure for hazardous wastes and total county-level HRS were positively associated with cancer incidence (p = 0.028 and p = 0.009, respectively). The number of proposed and final Superfund sites were also significantly associated with cancer incidence (p = 0.0025), indicating that counties with a greater number of proposed and final sites were related to increasing cancer incidence. However, the direct opposite relationship that counties with fewer Superfund sites—of all types—also related to increased cancer incidence. In Kearney (Citation2008), most Superfund sites near a cancer cluster were located within 5–10 miles rather than within 0–1 mile; perhaps that study, as well as this study, are observing effects of the spatial misalignment problem–data are not measured at the same spatial level and the misalignment propagates error throughout the analysis.
Table 5. The results of the Poisson-Gamma mixture model fit.
In addition, evidence of extreme spatial heterogeneity among cancer incidence rates was observed between the seven NWS geographic regions. Because of the larger size and few Superfund sites, the Tallahassee region (TAE) was used as the reference region for all nonspatial analyses. Of the remaining six regions, all other regions demonstrated strong positive association with increased cancer incidence rates in comparison to Tallahassee region (TAE) region (p < 0.05), with the exception of the Miami region (MFL). The strongest associations were observed in the Jacksonville region (JAX), the Melbourne region (MLB), and the Tampa Bay region (TBW). These relationships likely reflect that these regions contain the most Superfund sites. The covariate for median income was on the boundary of significance (p = 0.060).
Spatial Models
Spatial models were constructed separately for each gender and similar results were obtained using each type of spatial weight. However, no evidence of a significant association between environmental exposures and cancer incidence rates was observed.
Because of the observed regional differences using the nonspatial models, the Global Moran's I and Geary's C statistics were calculated for the spatial data, to determine the presence of any spatial autocorrelation between the cancer incidence rate and county. Both Moran's I and Geary's C presented evidence of spatial autocorrelation with p values ranging from p < 0.0001 (Moran's I, males, standardized queen weights) to p = 0.07 (Geary's C, females, binary queen weights). The results depended on the test, gender, and weights used. The tests for global spatial autocorrelation were significant for both males and females; smaller p values were observed for males with the largest value, p = 0.003.
To investigate further, the local Getis-Ord general G and local Moran's I tests were conducted. Both tests produced similar patterns for males and females, with the exception of a few counties (). Similar to the conclusions from Global Moran's I and Geary's C, these results indicate that cancer incidence rates for males have stronger spatial heterogeneities. That provides evidence that cancer rates are indeed different between counties and identifies the patterns in the data. Considering the Local Moran's I test together with the Getis-Ord general G, overall higher geographic variability was observed for male rates indicating spatial differences in cancer incidence rates by gender ().
Discussion
Using nonspatial analysis techniques, our results suggest a potential association between increased HRS score and increased proxy water exposure of hazardous wastes around Superfund sites with increased adult cancer incidence rates. Further, presence of a Superfund site in a county was associated with an increase in cancer incidence rates. Relationships between gender and age covariates, specifically being older and male, are associated with increased cancer incidence. Results from this research that show associations with cancer incidence rates between age and the male sex are not unexpected based on the typical longer latency period needed for cancer onset (Nadler Citation2014) and established gender differences in cancer incidences (Cook et al. Citation2009; Edgren et al. Citation2012). These results are important as almost 37% of the total population in Florida is 50 years of age or older and the resident median age for Florida is 41.2 years old, higher than the national median age of 37.4 years old (U.S. Census Bureau Citation2015a). Unexpectedly, higher median income was associated with a higher cancer incidence rate but this is perhaps an indication that diagnostic procedures might be more frequent or of a higher quality for people or families with higher income. This result may reflect that the NWS regions that contain the largest numbers of Superfund sites are also those regions with both wealthy and urban populations.
In addition, heterogeneity of cancer incidence was identified in all NWS regions without exception and NWS regions with the largest numbers of Superfund sites (JAX, MLB, TBW) had the strongest associations. Confirming results from the nonspatial model, Global Moran's I provided evidence of spatial autocorrelation for both types of spatial weights and sex. Similar results were observed using Geary's C statistic indicating that similar cancer incidence rates cluster spatially. The Gettis-Ord G and Local Moran's I results indicated that there is stronger spatial heterogeneity among males, although one high-high cluster was observed in one county for both males and females.
The spatial models did not find evidence of a significant association. This result could be due to the structure of the spatial model, as only one response was allowed for each spatial unit (e.g. county). The direct rate adjustment could have diluted a signal in the data, the signal that was observed in the non-spatial model. One suggestion is that future studies consider techniques to better ameliorate spatial misalignment in the data, such as using block kriging for county level estimates.
Although previous research has indicated an association between gender and increased cancer incidence (Cook et al. Citation2009), results from this analysis indicate that in addition, there are spatial differences between genders for cancer incidence. These results build on the spatial differences observed in Goli et al. (Citation2013) by identifying these spatial differences for a Western population while also investigating their associations with environmental exposures related to Superfund site locations. Spatial heterogeneity is important for future epidemiological work into identifying environmental causes of cancer clusters as well as to inform environmental regulations and policy.
A perplexing result from the nonspatial analysis is the observed relationship between decreasing numbers of proposed and deleted Superfund sites and increased cancer incidence, while a protective relationship is observed when considering all types of Superfund sites (proposed, deleted, and final) and cancer incidence. In addition, counties with small numbers of Superfund sites were found to have high cancer incidence rates. This could be due to location or material characteristics of the final Superfund sites. For example, Miami-Dade county has experienced massive immigration from inside and outside the US, in the past few decades. This has caused the population in this area to dramatically increase. Because of this population inflow, environmental exposures to toxic chemicals in this area is less likely for the new residents. In addition, the influx of new residents increased total population counts, possibly confounding cancer rates for this area. During the same time frame, immigration to the other parts of Florida (especially North Florida) was more moderate.
As in most research studies, limitations of the data and analysis are problematic. The demographics in the cancer incidence database for Florida contains an overwhelming number of white, non-Hispanic persons (>90%). These values are different from the overall population of Florida which is more diverse with 55% white, non-Hispanic and nearly 25% white, Hispanic (US Census Bureau Citation2015b). Another limitation of the analysis is the privacy concern motivating aggregation to the county level. Although beyond the scope of this analysis, to investigate overall all-cause cancer incidence, further investigation into different categories of cancer incidence may reveal more about possible explanations for these observed spatial differences. For example, differences in cancer incidence could be attributed to differences in occupation, residential location, risk behaviors such as tobacco use, or lifestyle differences such as staying indoors. Although further analysis and more detailed data, particularly exposure histories, are needed to better attribute what may be driving these spatial differences, the range of possible factors makes this a daunting task. The recent advances in spatial analysis techniques and software will become useful to further investigate cancer incidence and environmental hazardous waste exposure, when exposure history data are recorded and made available for study.
Conclusions
Results indicated potential association with environmental exposures related to Superfund sites and cancer incidence rates for Florida. In addition, evidence of spatial differences by gender for county-level cancer incidence rates in Florida were observed. More research is recommended on this topic to further identify and define these differences. Data availability creates the biggest challenge to further the science. Within the United States, cancer registries are defined and run by each state and they do not routinely include geographical or exposure histories that indicate length of residence at the time of cancer diagnosis, a thorough residential history, or an extremely detailed smoking and drinking exposure history. However, these exposure histories are essential to thoroughly investigate the effect of environmental exposures on cancer.
Abbreviations | ||
ACS | = | American Cancer Society |
ArcGIS | = | Geographic information system software produced by Environmental Systems Research Institute |
CERCLA | = | Comprehensive Environmental Response, Compensation and Liability Act |
EPA | = | U.S. Environmental Protection Agency |
FAPTP | = | The Florida Association of Pediatric Tumor Program |
FCDS | = | Florida Cancer Data System |
FDOH | = | Florida Department of Health |
GIS | = | Geographic Information System |
GLM | = | Generalized Linear Model |
HRS | = | Hazardous Ranking Score |
NPL | = | National Priorities List |
NRC | = | National Research Council |
NWS | = | National Weather Service |
SAR Model | = | Spatial Autoregressive Model |
USPP_1267599_Supplemental_Files.zip
Download Zip (1.2 MB)Acknowledgments
The Florida cancer incidence data used in this report were collected by the Florida Cancer Data system under contract with the Florida Department of Health. The views expressed herein are solely those of the author(s), and do not necessarily reflect those of the contractor of the Florida Department of Health.
The authors thank Dr. Xiaohui Xu and Dr. Jonathan D. Sugimoto for the project guidance and critical review of the analysis performed. In addition, the authors thank Dr. Wendell P. Cropper, Jr. for general guidance and early, critical review of the manuscript. The authors contributed equally to this article.
Funding
This research project was not funded by grant or any other source.
Conflict of Interest Statement
The authors state that they have no competing financial interests or conflict of interests in conducting this research.
Supplementary Materials
The supplementary materials contain detailed background information on the statistics and models used as well as a reference map of the 67 counties in Florida.
References
- Amin, R., Hendryx, M., Shull, M., and Bohnert, A. (2014), “A Cluster Analysis of Pediatric Cancer Incidence Rates in Florida: 2000–2010,” Statistics and Public Policy, 1, 69–77.
- Boffetta, P., and Nyberg, F. (2003), “Contribution of Environmental Factors to Cancer Risk,” British Medical Bulletin, 68, 71–94.
- Budnick, L., Sokal, D., Falk, H., Logue, J., and Fox, J. (1984), “Cancer and Birth Defects Near the Drake Superfund Site, Pennsylvania,” Archives of Environmental & Occupational Health, 39, 409–413.
- Cook, M. B., Dawsey, S. M., Freedman, N. D., Inskip, P. D., Wichner, S. M., Quraishi, S. M., Devesa, S. S., and McGlynn, K. A. (2009). “Sex Disparities in Cancer Incidence by Period and Age,” Cancer Epidemiol Biomarkers Preview, 18, 1174–1182.
- Davies, K. (2006). “Economic Costs of Childhood Diseases and Disabilities Attributable to Environmental Contaminants in Washington State, USA,” EcoHealth, 3, 86–94.
- Edgren, G., Liang, L., Adami, H. O., and Chang, E. T. (2012). “Enigmatic Sex Disparities in Cancer Incidence,” European Journal of Epidemiology, 27, 187–196.
- EPA (Environmental Protection Agency) (1992), “Hazard Ranking System Guidance Manual: Chapter 3, the Hazardous Ranking System Scoring Process,” available at http://nepis.epa.gov/Exe/ZyPDF.cgi/2000IS27.PDF?Dockey=2000IS27.PDF
- EPA (Environmental Protection Agency) (2014), “Superfund,” Available at http://www.epa.gov/superfund
- ESRI (2012), Environmental Systems Research Institute. Available at http://esri.com
- FCDS (Florida Cancer Data System) (2012), “FCDS Variables (available upon request and approval),” available at https://fcds.med.miami.edu/inc/datarequest.shtml
- Florida Cancer Data System (2016), FloridaCHARTS (2015) Median Household Income, “Statistics,” available at https://fcds.med.miami.edu/inc/statistics.shtml
- Florida CHARTS (2015), available at http://www.floridacharts.com/charts/OtherIndicators/NonVitalIndRateOnlyDataViewer.aspx?cid=0293
- FDOH (Florida Department of Health) (2012a) “Alachua County Report, Review of Cancer Rates for Census Tract 3 (Containing Stephen Foster Neighborhood),” available at http://www.alachuacounty.us/Depts/EPD/Pollution/Documents/1658_FDOH%20March%202012%20Ca-ncer%20Study%20Stephen%20Foster%20%20Koppers%20Neighbor-hood%20%206-6-2012.pdf
- Goli, A., Oroei, M., Jalalpour, M., Faramarzi, H., and Askarian, M. (2013), “The Spatial Distribution of Cancer Incidence in Fars Province: A GIS-Based Analysis of Cancer Registry Data,” International Journal of Preventive Medicine, 4, 1122–1130.
- Griffith, J., Duncan, R., Riggan, W., and Pellom, A. (1989), “Cancer Mortality in U.S. Counties with Hazardous Waste Sites and Ground Water Pollution,” Archives of Environmental Health, 44, 69–74.
- Hamra, G. B., Guha, N., Cohen, A., Laden, F., Raaschou-Nielsen, O., Samet, J. M., Vineis, P., Forastiere, F., Saldiva, P., Yorifugi, T., and Loomis, D. (2014), “Outdoor Particulate Matter Exposure and Lung Cancer: A Systematic Review and Meta-Analysis,” Environmental Health Perspectives, 122, 906–912.
- Heaton, M. J. (2014), “Wombling Analysis of Childhood Tumor Rates in Florida,” Statistics and Public Policy, 1, 60–67.
- Hendryx, M., Conley, J., Fedorko, E., Luo, J., and Armistead, M. (2012), “Permitted Water Pollution Discharges and Population Cancer and Non-Cancer Mortality: Toxicity Weights and Upstream Discharge Effects in US Rural-Urban Areas,” International Journal of Health Geographics, 11, 9–23.
- Johnson, B. (1995), “Nature, Extent, and Impact of Superfund Hazardous Waste Sites,” Chemosphere, 31, 2415–2428.
- Johnson, B. L., and DeRosa, C. T. (1997), “The Toxicologic Hazard of Superfund Hazardous-Waste Sites,” Reviews on Environmental Health, 12, 235–251.
- Kearney, G. (2008), “A Procedure for Detecting Childhood Cancer Clusters Near Hazardous Wastes Sites in Florida,” Journal of Environmental Health, 70, 29–34.
- Lawson, A. B., and Rotejanaprasert, C. (2014), “Childhood Brain Cancer in Florida: A Bayesian Clustering Approach,” Statistics and Public Policy, 1, 99–107.
- Nadler, D. L., and Zurbenko, I. G. (2014), “Estimating Cancer Latency Times using a Weibull Model,” Advanced Epidemiology. doi: 10.1155/2014/746769. Available at https://www.hindawi.com/archive/2014/746769/abs/.
- Naing, N. N. (2000), “Easy Way to Learn Standardization: Direct and Indirect Methods,” Malaysian Journal of Medical Sciences, 7, 10–15.
- Najem, G., Thind, I., Lavenhar, M., and Louria, D. (1983), “Gastrointestinal Cancer Mortality in New Jersey Counties, and the Relationship with Environmental Variables,” International Journal of Epidemiology, 12, 276–289.
- National Cancer Institute (2016), “Childhood Cancers,” Available at https://www.cancer.gov/types/childhood-cancers
- Raaschou-Nielsen, O., Andersen, Z. J., Beelen, R., Samoli, E., Stafoggia, M., Weinmayr, G., Hoffmann, B., Fischer, P., Nieuwenhuijsen, M. J., Brunekreef, B. Xun, W. W., Katsouyanni, K., Dimakopoulou, K., Sommar, J., Forsberg, B., Modig, L., Oudin, A., Oftedal, B., Schwarze, P. E., Nafstad, P., De Faire, U., Pedersen, N. L., Östenson, C., Fratiglioni, L., Penell, J., Korek, M., Pershagen, G., Eriksen, K. T., Sørensen, M., Ane Tjønneland, A., Ellermann, T., Eeftens, M., Peeters, P. H., Meliefste, K., Wang, M., Bueno-de-Mesquita, B., Key, T. J., de Hoogh, K., Concin, H., Nagel, G., Vilier, A., Grioni, S., Krogh, V., Tsai, M., Ricceri, F., Sacerdote, C., Galassi, C., Migliore, E., Ranzi, A., Cesaroni, G., Badaloni, C., Forastiere, F., Tamayo, I., Amiano, P., Dorronsoro, M., Trichopoulou, A., Bamia, C., Vineis, P., and Hoek, G. (2013), “Air Pollution and Lung Cancer Incidence in 17 European Cohorts: Prospective Analyses from the European Study of Cohorts for Air Pollution Effects (ESCAPE),” The Lancet Oncology, 14, 813–822.
- TOXMAP: Environmental Health e-Maps (2012a), “U.S. National Library of Medicine,” available at http://toxmap.nlm.nih.gov/toxmap/main/index.jsp
- ——— (2012b), “What Kinds of Chemicals Are Found at Superfund Sites?” Available at http://toxmap.nlm.nih.gov/toxmap/faq/2009/08/what-kinds-of-chemicals-are-found-at-superfund-sites.html
- ———. (2012c), “Superfund Chemicals in TOXMAP,” available at http://toxmap.nlm.nih.gov/toxmap/main/sfChemicals.jsp
- U.S. Census Bureau (2008), “2008 TIGER/Line® Shapefiles,” available at http://www.census.gov/cgi-bin/geo/shapefiles2008/national-files
- ——— (2015a), “Community Facts Florida,” available at https://factfinder.census.gov/faces/nav/jsf/pages/community_facts.xhtml
- ——— (2015b), “Quick Facts: Florida, 2015,” Available at http://www.census.gov/quickfacts/table/PST045215/12
- Wang, H., and Rodríguez, A. (2014), “Identifying Pediatric Cancer Clusters in Florida Using Log-Linear Models and Generalized Lasso Penalties,” Statistics and Public Policy, 1, 86–96.
- Zhang, Z., Lim, C. Y., and Maiti, T. (2014), “Analyzing 2000–2010 Childhood Age-Adjusted Cancer Rates in Florida: A Spatial Clustering Approach,” Statistics and Public Policy, 1, 120–128.