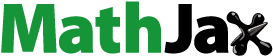
Abstract
In December 2020, Texas filed a motion to the U.S. Supreme Court claiming that the four battleground states: Pennsylvania, Georgia, Michigan, and Wisconsin did not conduct their 2020 presidential elections in compliance with the Constitution. Texas supported its motion with a statistical analysis purportedly demonstrating that it was highly improbable that Biden had more votes than Trump in the four battleground states. This article points out that Texas’s claim is logically flawed and the analysis submitted violated several fundamental principles of statistics.
1 Introduction
On December 7, 2020, Texas filed a motion requesting the U.S. Supreme Court to allow it to file a bill of complaint against the states of Pennsylvania, Georgia, Michigan, and Wisconsin. The complaint would assert that the defendant states “will appoint electors based on unconstitutional and deeply uncertain election results”Footnote1 and ask the Court to enjoin them from certifying the electors pledged to vote for President-elect Biden. In support of its motion, Texas presented two probability calculations stating that the probability Vice President Biden won the four states was less than one in a quadrillion.
On December 11, 2020, the Court rejected Texas’s attempt to deny the voters of the four defendant states their chosen electors.Footnote2 Because the analysis presented to the Court is logically flawed and violated basic principles of statistical reasoning, it is worthwhile pointing out the erroneous reasoning offered to the Court, so courts can reject such “statistical analyses” at an early phase of a case.
The article is organized as follows: the statistical analyses referred to in the motion, the expert’s affidavit, Pennsylvania’s reply, and Texas’s response are summarized in Section 2. Section 3 shows how the analyses reviewed in Section 2 are logically flawed and violate several basic principles of statistical reasoning.
2 The Statistical Arguments Presented by the States of Texas and Pennsylvania
2.1 Texas’s Statistical Argument
The motion filed by Texas described why the four defendant states did not conduct their elections appropriately. The motion supported its claim with two probability calculations.
The first was based on the fact that during the vote count, then-President Trump had an early lead, however, the later votes were sufficient to make President Biden the ultimate winner. The analysis said that “The probability of former Vice President Biden winning the popular vote in the four Defendant States—Georgia, Michigan, Pennsylvania, and Wisconsin—independently given President Trump’s early lead in those States as of 3 a.m. on November 4, 2020, is less than one in a quadrillion, or 1 in 1,000,000,000,000,000.”Footnote3
The second analysis compared the numbers and percentages of votes for President Biden in 2020 with those of Secretary of State Clinton in 2016. The expert’s declaration concluded “the statistical improbability of Mr. Biden winning the popular vote in these four States collectively is 1 in 1,000,000,000,000,000.”Footnote4
These probabilities were based on the Z-scores of standard statistical tests comparing the total votes and vote percentages between Clinton and Biden, and early versus later tabulations in the four battleground states. Those Z-scores are reported in .
Table 1 Z-scores in the four battleground states.
For example, for the state of Georgia, the expert first tested the hypothesis that the performance of Biden and Clinton were statistically similar by comparing both the total votes and voting percentages, using standard statistical tests. The resulting Z-scores are 396.3 and 108.7, respectively, enabling the expert to “reject the hypothesis many times more than one in a quadrillion times that the two outcomes were similar.”Footnote5 The expert also compared the percentage of voters counted by 3 a.m. on November 4th (48.91% for Biden and 51.09% for Trump) with the final results (50.14% for Biden and 49.86% for Trump) announced by the state on November 18th.Footnote6 Then he tested the equality of the percentage of the votes counted by 3 a.m. (early) that Biden received to his percentage of the votes counted after 3 a.m. (late). The Z-score of the two time periods is 1891 and consequently the two time periods “could not remotely plausibly be random samples from the same population of all Georgia ballots tabulated.”Footnote7
The expert further notes that Georgia had counted 95% of all votes by 3 a.m. and that comparable figures for the initial phase of counting in Pennsylvania, Wisconsin, and Michigan were 75%, 89%, and 69%, respectively, and they “are large enough to expect comparable percentages and vote margins for random selections of ballots to tabulate early and later.”Footnote8
Besides the above probability calculations, the expert also showed that early votes increased significantly in 2020 relative to 2016 in all four battleground states. For the state of Georgia, the expert also showed that a much smaller percentage of absentee ballots were rejected in 2020 (0.3634%) than in 2016 (6.42%). The motion claimed that the modifications in the state’s treatment of absentee ballots made in March of 2020 led to the large decline in rejected absentee ballots.Footnote9
2.2 The Reply of Pennsylvania and Texas’s Response
The reply brief of Pennsylvania addressed Texas’ probability calculations. For the early versus late comparison, Pennsylvania pointed out that the expert’s calculation assumed early and late votes were randomly drawn from the same population, however, those votes were clearly not “randomly drawn from the same population of votes.”Footnote10 For the comparison between Biden to Clinton, Pennsylvania noted that the calculation was based on the assumption that voters in a state would vote the same way in two consecutive elections. Because the elections were separate events, any analysis based on this assumption is worthless.Footnote11
In its response brief, Texas questioned the criticisms in Pennsylvania’s brief.Footnote12 Concerning the comparison of early versus late votes, Texas claimed that their expert “did take into account the possibility that votes were not randomly drawn in the later period” but he was unaware of any data that would support such an assertion. With regard to the comparison of Biden to Clinton, the brief refers to a subsequent analysis by its expert that showed that Biden underperformed Clinton in the Top-50 urban areas in the Country by 1.4%,Footnote13 but received a larger percentage of votes in the four of the five urban areas in the defendant states. The expert claimed that this pattern was unusual and deserves more scrutiny.Footnote14
3 How the Analysis Submitted by Texas is Logically Flawed and Violates Some Basic Statistical Principles
This section explains why the logic and statistical reasoning underlying Texas’s analysis are incorrect.
3.1 The Comparison of Total Votes and Vote Percentages between Biden and Clinton
The expert found that in all the four battleground states, the increase in total votes and percentages of votes for Biden over Clinton are “statistically incredible if the outcomes were based on similar populations of voters supporting the two Democrat candidates,” thereby raising doubts about the 2020 election outcomes.Footnote15 These comparisons between Clinton and Biden are logically wrong. As stated later in this section, the circumstances in 2016 and 2020 were substantially different, therefore, one expects different numbers of voters and different percentages of them favoring the Democrats and Republicans in the two years, that is, the populations of voters supporting the Democratic candidate were not expected to be similar. The statistical significance of the test of the equality of the percentages voting for Biden and Clinton simply confirms the fact that the political preferences of voters differed in the two years and does not raise any doubt about the vote count of the 2020 election.
Following the principles of Mallows (Citation1998), there should be a reason for conducting a study. However, there is no political or historical justification for assuming the political preferences for voters in 2020 and 2016 should be similar. Not only were Biden and Clinton different candidates with different histories and styles, the state of the nation differed substantially in 2020 compared to 2016. The 2020 election occurred in the midst of the worst pandemic the nation had experienced in 100 years. The national unemployment rate was 6.9%,Footnote16 much higher than the 4.6% in November 2016.Footnote17 Furthermore, a much larger number of voters participated in the 2020 election than in 2016. According to the United States Elections Project, the turnout rates as a percentage of voting-eligible population was 59.2%Footnote18 in 2016, and 66.7%Footnote19 in 2020, a 7.5% increase. The fact that over 22 million Americans moved to a different state between 2017 and 2019 also affected the pool of eligible voters in the states.Footnote20 Moreover, even if the populations of voters in the two elections were nearly identical, political preferences can certainly change even in a two-year period. For example, while both President Obama and President Trump started their terms with a majority of House of Representatives being from their respective parties, after the mid-term elections the opposing party became the majority of the House.
Historically, when a president runs for reelection, the percentages of voters who voted for their opponents usually are not the same in both elections. lists the percentages of the popular votes for their opponents for those Presidents who ran for reelection, since President McKinley in 1896. Even when the President ran against the same candidate in both elections, for example, President Eisenhower ran against Stevenson both times, the percentages of votes the opponent received changed, due to changes in the social, political, economic conditions occurring between the elections, the current President’s approval ratings and in some cases, the existence of a serious third party candidate in one of the elections. The differences between the opponents’ percentages in the second and first elections range from –5.2% to 18.7%. In fact, shows that in the 16 pairs of elections where an incumbent ran for reelection, there were only three where the percentages of votes the opponent received were within 1%.Footnote21 Because the historical data clearly contradict the idea that the percentages of votes received by candidates from the same party should be the same in successive elections, the hypothesis tested by the expert has no subject matter justification.
Table 2 Comparison of the opponents’ percentages of the popular votes in the first and second elections when U.S. Presidents Ran for Reelection.
In addition to the logical flaw in the Biden versus Clinton comparison, the application of the standard statistical hypothesis tests is questionable for several reasons.
3.1.1 Assumptions of the Statistical Tests are Violated
The calculations of the Z-scores in the expert declaration assume the data are random samples from a common population,Footnote22 but the expert’s affidavit provides no justification for this assumption. Indeed, it is likely that voters are more interested or have stronger political views than nonvoters, so the population of voters differs from the population of eligible voters. Consequently, the assumption underlying the standard statistical tests that the sample (those who actually voted) are randomly chosen members of the population of eligible voters is false. Meng (Citation2018) showed that seemingly small violations of randomness can seriously bias results, which implies that the expert’s conclusions are unreliable.
3.1.2 The Conclusions from the Hypothesis Tests are Wrong
Even under the questionable assumptions made by the expert, the large Z values reported in the affidavit simply shows that the total votes and percentages of those votes cast for Biden and Clinton are significantly different. As noted earlier, the two populations of voters being compared are different, and the large Z-scores simply confirm this. They do not cast doubt about the election results.
3.1.3 Other Factors that Might Influence Voters’ Preference are Not Controlled for
Since the economic and public health circumstances were substantially different at the time of the 2016 and 2020 elections, the analysis should include a control population, for example, all the other states or possibly a subset of other states where the vote was expected to be close.Footnote23 Therefore, the simplistic analysis only comparing Biden to Clinton in the four battleground states is meaningless. Because the statewide elections are of primary importance, changes in voter turnout as well as the Biden versus Clinton comparison in all 50 states and the District of Columbia will be reviewed.
Biden versus Clinton:
in the Appendix shows that the percentage of individuals who voted for Biden in 2020 were higher than those for Clinton in 2016 in all 50 states and the District of Columbia, not just in the four battleground states. Even in states that Trump won, for example, Kansas, Idaho, and Utah, Biden received at least 5% more votes than Clinton.
Table A1 Clinton versus Biden comparison.
Voting for Other Candidates:
In all 50 states and the District of Columbia, the percentages of voters who voted for other candidates on the ballot were lower in 2020 than in 2016 (see in the Appendix).
Voter Turnout:
in the Appendix shows that in all 50 states and the District of Columbia, the voter turnout rates as a percentage of the voting-eligible population in 2020 are higher than those in 2016. The turnout rates in 2020 in the four battleground states, Georgia, Pennsylvania, Wisconsin and Michigan, were 8.6%, 7.4%, 6.3%, and 9.2% higher than the 2016 turnout in those states. These are similar to the national increase of 7.5%.
Table A2 Voter Turnout rates for 2020 and 2016.
Because the Biden versus Clinton comparisons in the four battleground states were similar to the same comparisons in other states, the fact that Biden received more votes and a higher percentage of votes than Clinton in the four battleground states does not raise a credible doubt about the election results in those four states. Indeed, had the expert used a control group, which is standard statistical practice, he would have seen that this pattern occurred in all 50 states and the District of Columbia.
3.1.4 Texas’s Additional Analysis in its Response
In the reply filed by Texas, the expert claimed that, after removing the four battleground states, Clinton outperformed Biden by 1.4% in the top-50 cities, but Biden won four out of five major urban counties in the battleground states. He infers that this conflict is unusual and justifies further investigationFootnote24. The expert’s declaration does not report or cite the source of the data used to support the claim. In fact, the claim is wrong. Biden outperformed Clinton in a majority of the largest urban areas. The National Review examined the data in 36 of the top-50 urban areas and found that in 29 of them Biden received a higher percentage of the votes than Clinton.Footnote25 This was known in November, 2020 before the motion was filed.
3.2 Comparing Early and Subsequent Tabulations
The expert reported that Trump led the vote count before 3 a.m. on November 4, 2020. When additional ballots were included, Biden won all the four battleground states. The expert tested the hypothesis that the percentages of votes for Trump tabulated in the two time periods were equal. From the result that they were statistically significantly different, he concluded that the votes counted in the two periods “could not remotely plausibly by random samples from the same population of all Georgia ballots tabulated,”Footnote26 which cast doubts on the election results in those four states. The logic of this conclusion is wrong.
The ballots counted in the two time periods are not random samplesfrom all votes in each state, that is, the assumption that they are random samples on which the standard statistical tests are based is violated. Most jurisdictions count the ballots cast by absentee and early voters after they count the votes cast in person on Election Day.Footnote27 According to New York Times,Footnote28 mail ballots tend to take longer to process than in-person votes, and millions more people voted by mail in 2020 than ever before. Since a higher proportion of registered Democrats requested absentee ballots, this trend combined with the expected delays in tabulating absentee ballots in several battleground states, implies that the in-person vote counted soon after polls closed on Election Day were likely to show early Republican leads, and the absentee votes tallied later were likely to show Democrats gaining ground.Footnote29
Even if the assumptions underlying the tests were satisfied, the statistically significant results would only mean that the percentages of the total vote Trump received in the two time periods were different. They do not imply that the early and later votes are not random samples from the same population. On the contrary, only when the two samples are randomly selected can one use the Z-score to test whether the candidate preferences in the votes counted early or later are statistically similar, that is, the fact that the data are random samples is one of the assumptions required for the validity of the statistical test, not an inference or conclusion.
Furthermore, the expert’s declaration stated that “Georgia had tabulated 95% of the ballots cast by 3 a.m. EST. The comparable initial tabulations in Pennsylvania, Wisconsin, and Michigan were 75%, 89% and 69%. These are large enough to expect comparable percentages and vote margins for random selections of ballots to tabulate early and late.”Footnote30 When comparing the percentages of votes counted early and later received by the candidates, the fact that ballots counted early were a large proportion of all the votes does not imply that they were randomly selected from all ballots cast. The votes counted in the two time periods reflected the different preferences of in person votes and mail-in votes. Indeed, it was known before Election Day that the percentages of votes favoring either candidate in the two voting options would not be similar.
Because the expert’s declaration discussed the situation in Georgia in detail and the state had to carry out two recounts, it is worthwhile to examine the data in detail. reports the way Georgians cast their ballots in the election.
Table 3 Georgia votes by type of vote in 2020.
The data in demonstrate that Biden’s strong majority of the absentee by mail ballots were essential to his winning the state. Because these votes were counted after the ballots cast on Election Day, the votes tabulated earlier in the process favored Trump, while the absentee votes reversed his lead. Vote counting was also delayed in Fulton County (Atlanta, Georgia) because a pipe burst in State Farm Arena just above the room where the absentee ballots were being counted.Footnote31 Thus, absentee ballots from Atlanta, which is predominantly Democratic were counted later. On December 2nd, the Secretary of State who oversees the voting process announced that a second recount confirmed that President Biden received more votes.Footnote32
3.3 Percent Increase in Early Ballots between 2016 and 2020
The expert showed that the four battleground states had a significant increase in early balloting in 2020 compared to 2016.Footnote33 Even thoughan explicit conclusion was not drawn from these increases, reporting them in only the four battleground states suggests they were unusual situations, thereby casting doubt about the election results in those four states.
The increase in early ballots in 2020 was expected because many states gave voters more opportunity to vote absentee or early in response to the pandemic. in the Appendix lists the early ballots for 2016 and 2020 for all 50 states and the District of Columbia along with the 2020/2016 ratio, expressed as a percentage. The table is arranged in descending order of the 2020/2016 ratio and shows that 2020 early ballots increased in all 50 states and the District of Columbia.Footnote34 The ratios of the four battleground states are ranked 4, 16, 17, and 31 among the 50 states and District of Columba and are in line with those of other states. If one desires to draw a sound statistical conclusion from the 2020/2016 early ballot ratios for the four battleground states, one should compare them to the ratios of the other states.
Table A3 Early ballots for 2020 and 2016.
4 Final Remarks and Conclusion
This article points out that the analysis of the 2020 election submitted by Texas is logically flawed and violated several major statistical principals. First, the populations being compared, 2016 and 2020 votes, are by definition different. Historical data also showed that the percentage of votes received by a party’s candidate in one election usually differed from the succeeding election. Second, one cannot draw a sound inference by examining only data in the four battleground states. Only when the trends in the four battleground states differ from those of comparable states can one believe those trends are meaningful. This is especially true in the present context as the economic and health situations in 2020 were dramatically different from 2016 throughout the nation.
Third, a major assumption in testing the equality of two binomial proportions is that both proportions were obtained by random sampling. The individuals who voted in 2016 and those who voted in 2020 are not random samples from all eligible voters. Thus, the large Z-scores and the corresponding extremely small p-values reported in the expert declaration are calculated under an erroneous assumption and conclusions drawn from them are not reliable.
Fourth, the p-values do not convey information on whether or not the samples are random. With respect to the 2020 election, several sourcesFootnote35 show that the tabulated votes at the two time frames were not random samples and hence, once cannot expect comparable percentages from the two time periods. Thus, the expert’s conclusions from the early versus late comparison are not sound.
Acknowledgments
The authors thank the referees and Associate Editor for many helpful comments and suggestions.
Notes
1 State of Texas v. Commonwealth of Pennsylvania, State of Georgia, State of Michigan and State of Wisconsin, docket number 220155 (available at www.scotusblog.com, which has all of the briefs filed in the case).
2 Texas v. Pennsylvania 592 U.S._ (2020). The decision was based on legal considerations.
3 Page 8 of State of Texas v. Commonwealth of Pennsylvania, State of Georgia, State of Michigan and State of Wisconsin, citing expert’s declaration in the Appendix at 4a–7a, 9a.
4 Id at 8.
5 Expert Declaration, appendix 3a.
6 Expert declaration 4a. These percentages exclude third-party candidates.
7 Expert Declaration, appendix 5a.
8 Expert Declaration, appendix 7a.
9 The compromise and settlement of the lawsuit, Democratic Party of Georgia v. Raffensperger, No. 1:19-cv-5028-WMR (N.D. Ga.), stipulated that before a signed ballot would be rejected, three registrars would need to examine it and compare it to the one on file.
10 Opposition to Motion for Leave to File Bill of Complaint, December 10, 2020, No. 22O155, at pages 7 and 8.
11 Id. at 8.
12 Reply in Support of Motion for Leave to File, No. 22O155, December, 11, 2020, pages 2–3.
13 Id. at 156a. The expert removed cities in the four battleground states in determining the top-50 cities.
14 Id. at 157a.
15 Expert declaration at 4a.
17 According to https://www.bls.gov/opub/ted/2016/unemployment-rate-declines-to-4-point-6-percent-in-november-2016.htm, the unemployment rate in November 2016 was 4.6%.
19 http://www.electproject.org/2020g. The website also contains the voter turnout rates for each state.
20 Derived from Table K200701 from the U.S. Census Bureau’s data on geographical mobility obtained from the Bureau’s website. The data indicate that about 2.2%–2.3% of the population moves each year.
21 McKinley (1896, 1900), Reagan (1980, 1984), and Bush (2000, 2004).
22 The Declaration does not state this explicitly, but when drawing conclusions from standard statistical tests using p-values, the expert implicitly assumed random samples from the same population. See expert’s original declaration, pages 3a–7a.
23 The need for an appropriate control or comparator is noted in equal employment cases, for example, Crawford v. Ind. Harbor Belt R.R. Co. 461 F.3d 844 (7th. Cir. 2006) and in trademark infringement cases (Jacoby Citation2002; Diamond and Franklyn Citation2014).
24 Page 156a of the expert’s Supplemental Declaration.
25 Dan McLaughlin: No, Joe Biden Did Not Only Improve in Four Major Swing-State Cities. National Review (November 16, 2020) (available at https://www.nationalreview.com/corner/no-joe-biden-did-not-only-improve-in-four-major-swing-state-cities).
26 Pages 4a and 5a of the expert’s original declaration.
27 Delays in Verifying Mail-In Ballots Will Slow Election Tally, by Anthony Izaguirre, Associate Press on October 4, 2020.
28 We Have Never Had Final Results on Election Day, New York Times, by Maggie Astor, updated on November 3.
29 Record-Setting Turnout: Tracking Early Voting in the 2020 Election, by Lazaro Gamio, John Keefe, Denise Lu and Rich Harris, New York Times, updated November 12, 2020.
30 Page 7a of expert declaration.
31 Brash, B. (2020). Fulton County election results delayed after pipe bursts. The Atlanta Journal-Constitution (November 3rd).
32 Biden to Carry Georgia After Second Recount: State Election Official https://www.usnews.com/news/top-news/articles/2020-12-02/biden-will-carry-georgia-after-second-recount-secretary-of-state.
33 Table 1 on page 5a of expert’s original declaration.
34 The increase was noticeable except in the three states that mailed ballots to registered voters in both 2016 and 2020.
35 See footnotes 27 and 28.
References
- Diamond, S. S., and Franklyn, D. J. (2014), “Trademark Surveys: An Undulating Path,” Texas Law Review, 92, 2029–2073.
- Jacoby, J. (2002), “Experimental Design and the Selection of Controls in Trademark and Deceptive Advertising Surveys,” The Trademark Reporter, 92, 890–895.
- Mallows, C. (1998), “The Zeroth Problem,” The American Statistician, 52, 1–9.
- Meng, X.-L. (2018), “Statistical Paradises and Paradoxes in Big Data (I): Law of Large Populations, Big Data Paradox, and the 2016 U.S. Presidential Election,” Annals of Applied Statistics, 12, 685–726.