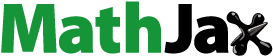
Abstract
The measurement of the disparate impact of a particular de facto discriminatory policy on a minority or otherwise legally protected group has been of importance since passage of the Civil Rights Act of 1964. When the data available for the measurement of disparate impact, as embodied in the so-called “disparity ratio,” come from samples, a statistical approach naturally suggests itself. This article reviews both the law and statistics literature with regard to statistical inference applicable to the disparity ratio and related measures of disparate impact. From that review, three primary approaches are evaluated, the difference in so-called “rejection” rates for the protected and non-protected groups, their ratio (the disparity ratio), and the natural logarithm of the disparity ratio. For various reasons, the direct ratio estimator is recommended for use in all but small samples, where the log-ratio approach is to be preferred. The main points are illustrated with two fair housing examples, one being the possible discriminatory effect by race owing to a landlord’s refusal to accept Section 8 housing vouchers in lieu of cash rent, and the other being the effects of occupancy restrictions on families with children. Various methodological issues that arise in the application of these three estimation approaches are addressed in the context of the more complex sample designs that underlie the data utilized.
1 Introduction
Since passage of the Civil Rights Act of 1964, plaintiffs have been able to mount legal actions under Title VII in order to mitigate the effects of de facto discriminatory policies in employment matters, while the Fair Housing Act of 1968 (FHA) prohibits discrimination in residency preferences, the use of screening devices in housing, exclusionary zoning, mortgage practices, home insurance standards, and occupancy restrictions (Murray and Cornelius Citation2014; Glassman and Verna Citation2016; Schwemm and Bradford Citation2016).Footnote1 First, the plaintiff must prove that the defendant’s policy caused a sufficiently large disparate impact on a racial minority or other legally-protected group.Footnote2 The types of claims covered must involve a pervasive policy, not a single act or decision. Once disparate impact is established, then it remains to establish causation, essentially isolating the offending policy from other factors that could have resulted in the observed inequality. Even if defendant establishes a legitimate reason for its policy, plaintiff can still prevail if it shows that a less discriminatory policy would have served.
The approaches used to adjudicate disparate impact claims evolved in a somewhat uneven way, in particular, where erroneous or inconsistent techniques were used [Schwemm and Bradford Citation2016 (hereafter S&B), p. 690]. After the Supreme Court’s 2015 decision in the case of Texas Department of Housing and Community Affairs vs. the Inclusive Communities Project Inc. (“Inclusive Communities”), a set of guidelines emerged for demonstrating disparate impact.Footnote3
There are four guidelines involved:Footnote4
Plaintiff’s statistical evidence must focus on the subset of the population affected by the challenged policy. The affected population will vary by case, and could be quite limited (e.g., persons residing at a particular housing complex) or broad (e.g., a landlord’s screening device for applicants or a municipality’s blocking of a proposed development.) The affected population could vary even in a single case if the policy has both a future impact and a backward-looking impact.
Within the affected population, the statistical evidence must focus on appropriate comparison groups and must show “disparate impact,” not only that the protected class was harmed but that others were less harmed.
The statistical comparison should show relative, not absolute (number of persons who were harmed), impact.
The disparate impact should be “sizeable.”
The data involved should, in most cases, focus on the local market or metro area. For housing cases, this involves “applicant flow” information which, unlike employment application data, may not exist. The definition of the “local market” also may or may not be obvious.
The usual way to measure disparate impact is through the “disparity ratio.” The disparity ratio shows the percentage of households (or individuals) in a protected class that are impacted by a policy divided by the percentage of households (or individuals) not in that protected class which are impacted (e.g., the percentage of households with children with three or more persons divided by the percentage of households without children with three or more persons).Footnote5 The disparity ratio thus, is defined as a relative measure. It does not reflect the numbers of individuals affected by a policy, which also may be of interest. For example, if 4% of the protected class is impacted while only 1% of the non-protected class is, the disparity ratio is 4.0, and 3 percentage points more individuals in the protected class are affected. Another policy applied to the same underlying populations may impact 88% of the protected class and 80% of the non-protected class, which yields a much smaller disparity ratio (1.1) but has an impact on many more individuals (8 percentage points).
In this article we concentrate on statistical approaches for establishing disparate impact using sample data. First, we summarize and critique the extant approaches for measuring disparate impact and establishing its statistical significance from a review of the law literature. It is shown how two commonly used approaches to establishing disparate impact based on a comparison of the “rejection” rate for the protected class to the rejection rate for the non-protected class are related and why estimation and inference using the disparity ratio is a more appropriate way to proceed.
Next, we turn to a review of the relevant statistics literature. Much of it stems from the measurement of disparate impact in employee selection processes. In this context, the ratio of “selection” rates is called the “adverse impact ratio.” Of particular interest is the construction of confidence intervals for the adverse impact ratio based on its logarithmic transformation as opposed to the direct ratio estimator itself. Much relevant work has also been done on the sample size requirements for the efficacy of both approaches, since the distributional assumptions they rest on are only approximate.
We go on to illustrate the main points gleaned from these reviews via two examples drawn from the history of fair housing litigation. The first involves Section 8 housing vouchers, whereby the refusal to accept them in partial payment of rent on the part of a landlord can have discriminatory implications by race owing to differences in income distributions. The second example involves occupancy limitations in apartment rentals and their impact on families with children. Here we introduce the idea of “incremental disparity” as a way to measure the possible disparate impact of a particular occupancy policy relative to federal or state guidelines. These applications involve datasets that incorporate household weights indicative of the more complex sample designs used in their source, the American Community survey.
The technical details are relegated to an appendix. A second appendix is devoted to an example of choice of significance level for a test of the disparity ratio based on the relative hazards associated with Type I and Type II decision errors.
Our primary goal is to aid practitioners (lawyers and their statistical consultants) by first critically evaluating what has been done in the past to provide statistical evidence in disparate impact cases and then to set out improved statistical methods for assessing disparate impact, particularly in fair housing cases.
2 Statistical Tests for the Disparity Ratio: The Law Literature
In their comprehensive review of the use of the disparity ratio in various litigation contexts, S & B (p. 699) define the disparity ratio as the relative percentages of protected (P1) versus non-protected class members (P2) affected by a policy. They define different measures that correspond to whether the impact of a policy is beneficial or harmful.
“Selection” rates correspond to a beneficial outcome, such as comparing the pass rate on a job test for protected class members (which we denote as Q1) to the pass rate for non-protected class members (which we denote as Q2). This gives rise to the 4/5ths Rule for use in Title-VII-impact cases: that a policy will only be judged to have a disparate impact if the (presumed beneficial) selection rate of members of the protected class is 80 percent or less than the selection rate of non-protected class members. In contrast, “rejection” rates correspond to a policy with harmful impacts. S&B focus on housing discrimination cases, which typically involve rejection rates.Footnote6 Here disparate impact is judged to occur if this ratio is 1.25 or above, as a rule of thumb. In the early days of litigation involving either Title VII or the Fair Housing Act, before plaintiffs employed statistical analysis of any sort, these so-called “actionable” values were recognized by the courts as encompassing a variety of uncertainties, including statistical uncertainty.
Following S&B’s terminology for consistency, it is useful to discuss the relationship between the ratio of rejection rates relevant to fair housing cases—the disparity ratio R P1/P2—and its counterpart based on selection rates, namely, the adverse impact ratio R*
Q1/Q2, where, for example, Q
P1). Importantly, it is not the case that R = 1/R*, even though it is true that
.Footnote7 The algebraic relationship between R and R* is shown in (A.3a) and (A.3b) of Appendix A.
Whatever actionable value of the disparity ratio is chosen, when sample data are used to evaluate the question of whether it has been equaled or exceeded, techniques of statistical inference need to be employed. In their very comprehensive article on the Inclusive Communities case and its offshoots, S&B recommend two different approaches. First, they suggest comparing the two-sided confidence intervals for the numerator and denominator of the disparity ratio to see if they overlap. But they note that a statistical test of the difference between the numerator and denominator proportions is the “most common” approach. While these two approaches are similar, algebraically they are not identical, as demonstrated in Appendix A, (A.8) and (A.9a).
The nonoverlapping confidence intervals test compares the lower bound of the confidence interval for the numerator (P1) to the upper bound of the confidence interval for the denominator (P2). This appears intended to test that the difference between the numerator and denominator in R P1/P2 is equal to or greater than zero, and hence that the disparity ratio is equal to or greater than one. A direct test of the difference (or a confidence interval for it) has the same interpretation but will not yield identical results. In particular, at a given confidence level, the nonoverlapping confidence intervals approach may fail to reject the null hypothesis that the disparity ratio is equal to one when a direct test of the null hypothesis of no difference or a confidence interval for the difference would approximation to the reject the null hypothesis because the lower bound in (A.9a) is always greater than the lower bound in (A.8).Footnote8 More importantly, the direct test of difference and its confidence interval counterpart are consistent with the Normal difference between two binomials. Thus, the direct test of the difference or confidence interval for the difference is preferable. However, no other value for the disparity ratio than R
can be evaluated with either of these approaches. It is to be noted that the use of a two-sided confidence interval is inconsistent with the implied one-sided test of the disparity ratio being equal to or exceeding a pre-specified value. The implication of this error is that the nominal level of significance from using the two-sided confidence interval for testing purposes is actually smaller than indicated.Footnote9 Note also that an estimated positive difference, (p1–p2) > 0, is consistent with many disparity ratios greater than one.Footnote10 Take, for instance, an observed difference of 0.05. If p
and p
, then the observed disparity ratio r
p1/p
. But if p
and p
, then r
.
Other authors have promoted the direct test of difference in the two proportions as the most appropriate method. Paetzold and Willborn (Citation2011) opine that either the test of difference (in their case, in selection rates) alone or the 4/5ths Rule alone could establish disparate impact, under the notion that the use of 0.80 as the ceiling already contains a fudge factor for statistical uncertainty. But, of course, a difference that is meaningful in magnitude (yielding a disparity ratio of 0.8 or lower) does not guarantee it will be statistically significant. In Peresie (Citation2009), the same ideas are pursued. To her credit, she recognizes that a one-sided test of the difference should be used and she discusses an important aspect of statistical testing, namely the choice of a significance level and the tradeoff between Type I and Type II errors, a topic we address in Appendix B. What is highlighted there is how the relative costs of decision errors in the context of disparate impact can be accounted for in the tradeoff between the “significance” and “power” of statistical tests involving the disparity ratio.Footnote11
In practice, it is commonplace to use the difference test to argue that P P2 if the hypothesis P
P
is rejected and then to discuss whether the observed “effect,” namely, the observed disparity ratio, is large enough to establish prima facie discrimination. This approach has been codified for the adverse impact ratio in the most recent guidelines issued by the U.S. Department of Labor’s Office of Federal Contract Compliance Programs (OFCCP 2020), where it is said that to be prima facie evidence of discrimination the (absolute) difference in selection rates between the protected and non-protected groups must be at least twice its standard error, or the so-called “p-value,” which is the area under a standard Normal distribution between the ratio of this difference to its standard error (the so-called “Z-statistic”) and infinity must be 0.05 or less.Footnote12
The OFCCP guidelines also discuss “practical significance” once statistical significance has been established. They concentrate on two measures: the size of the observed adverse impact ratio and the magnitude of the percentage point difference between q2 and q1. They create “sliding scales” to convey the likelihood that a finding of practical significance would be made in each case. For instance, in the case of the adverse impact ratio, it would be “unlikely” or “very unlikely” for OFCCP to conclude there was discrimination in a practical sense if r* , the infamous 4/5ths Rule. If r* lies between 0.7 and 0.8, a conclusion of discrimination would be “likely,” and for r* < 0.7, “very likely.”Footnote13 Yet without performing a statistical test for R* < 0.8 or forming a one-sided confidence interval for R*, a defendant might argue that the observed value was simply due to chance.Footnote14
As mentioned in the previous section, the disparity and adverse impact ratios are relative measures. They do not reflect the numbers of individuals or households impacted by a policy or process. The OFCCP guidelines address this issue by adding a second level of “practical significance,” namely, the percentage point difference between q2 and q1. Again, a sliding scale is used, where with a difference of 2 percentage points or less, OFCCP would be “unlikely” or “very unlikely” to act, whereas with a difference of 2–5 percentage points it would be “likely” to act and with a difference of greater than 5 percentage points it would be “very likely” to act. The use of this measure would come into play, according to the OFCCP, when selection rates are low, which can give rise to situations where r* < 0.8 but very few applicants are impacted.Footnote15 OFCCP is quick to add, however, that though it has established these two sets of benchmarks, it will still exercise discretion in issuing “pre-enforcement notices” according to the “facts and circumstances of individual cases” (OFCCP 2020, p. 71560).Footnote16 A recent paper by Gastwirth et al. (Citation2021) sets out an improved set of guidelines.
While the context for OFCCP is employment discrimination and thus the adverse impact ratio is the relevant measure, the issues just presented translate directly to our situation, which focuses on R, the disparity ratio.
The regulatory background is U.S. Department of Housing and Urban Development (US HUD) (2013, 2020), especially the sections regarding the so-called “burden-shifting” test for proving a claim of liability for discriminatory impact under the FHA. This consists of three parts:Footnote17
“…the plaintiff bears the burden of proving its prima facie case that a particular practice results in, or would predictably result in, a discriminatory effect on the basis of a protected characteristic.
“If the …plaintiff proves a prima facie case, the burden of proof shifts to the …defendant to prove that the challenged practice is necessary to achieve one or more of its substantial, legitimate, nondiscriminatory interests.
“If the …defendant satisfies this burden, then the …plaintiff may still establish liability by proving that the substantial, legitimate, nondiscriminatory interest could be served by a practice that has less discriminatory effect.”
“Discriminatory effect” is defined as (a) resulting in a disparate impact on a protected class, or (b) has the effect of creating, perpetuating, or increasing segregated housing patterns. Part (a) is illustrated with reference, in particular, to the disparity ratio. But, unlike with the OFCCP, no technical guidelines covering such things as choice of significance level or practical significance are included.Footnote18
Accordingly, with focus on the disparity ratio we now proceed to approximate the variance of the direct ratio estimator r p1/p2 using standard methods. The resulting formula is (A.13a) in Appendix A. Once calculated, then a confidence interval for R or a statistical test for a specific value of R being equaled or exceeded can be developed, as shown in Appendix A, (A.14) and (A.15).Footnote19 Formulas are given for simple random sampling and for simple random sampling with weights, as well as the formula for use with other sample designs, since the data used in our examples contain household-specific weights, reflecting unequal inverse selection probabilities.
3 Statistical Tests for the Disparity Ratio: The Statistics Literature
The direct ratio estimator has a venerable history in epidemiology as a measure of association between an exposure (say, to a carcinogenic substance) and a binary outcome (say, life or death). In this context, it is called the “risk ratio.” The NIH Library of Medicine’s National Center for Biotechnical Information cites many articles relating to its estimation and use in cohort studies. For example, the review paper by Cummings (Citation2009) explores the relative merits of the risk ratio versus the odds ratio OR (P1/Q1)/(P2/Q2) and concludes that unless the outcome is rare (when the odds ratio closely approximates the risk ratio), the risk ratio is to be preferred, since it has “…a useful interpretation as the ratio change in average risk due to exposure.”Footnote20 While the odds ratio does have the advantage of symmetry with regard to outcome definitions, for a variety of reasons the risk ratio is the preferred measure.Footnote21
Estimating the so-called “crude” risk ratio is identical to direct ratio estimation. But because in epidemiological applications there are many confounding factors that impact the association between exposure and outcome (e.g., age, sex), estimation approaches that can control for these confounding factors are used, such as the Mantel-Haenzel Method that averages the risk ratio over strata defined by a confounding factor (Newman Citation2001) or via regression analysis (Robbins, Chao, and Fonseca Citation2002). It is recognized that the usefulness of the risk ratio is enhanced when a corresponding confidence interval is reported as well (Viera Citation2008).Footnote22
An early paper by Katz et al. (Citation1978) is somewhat relevant for our purposes because it addresses the relative merits of the construction of one-sided confidence bounds for the risk ratio among three competing approaches, one derived from the odds ratio, one derived from the approximate Normal distribution of (p1–Rp2) and the third from ln (p1/p2). These authors conclude that the first and third methods produce confidence intervals that more reliably achieve their target () confidence levels for
, 0.05, and 0.10, especially in small samples.Footnote23 It is to be noted that the second method is not equivalent to a confidence interval based on our (A.14), so their comparison is of limited value. In the one application they present, the lower bound computed for the second method is greater than that computed for the log-ratio method, a result opposite to a general finding we report on comparing the direct ratio estimator to the log-ratio method.
As an early precursor to the OFCCP guidelines, the U.S. Equal Employment Opportunity Commission (EEOC) issued its guidelines on employee selection procedures in 1978.Footnote24 Among them was the so-called “4/5ths Rule” which stated that “a selection rate for any race, sex, or ethnic group which is less than four-fifths (4/5, or 80%) of the rate for the group with the highest rate will generally be regarded by the Federal enforcement agencies as evidence of adverse impact, while a greater than four-fifths rate will generally not be regarded as evidence of adverse impact” (Equal Employment Opportunity Commission et al 1978, p. 38297). The guidelines go on to say that smaller differences may nevertheless be considered to indicate adverse impact if they are shown to be statistically and practically significant, while greater differences may not be considered so if they are not statistically significant or if it can be demonstrated that the particular sample pool of applicants is atypical in some meaningful way from the “normal” pool of applicants. In an earlier case, the courts established the criterion for statistical significance at , though this is not specifically mentioned in the EEOC guidelines.
The introduction of the 4/5ths Rule as a substitute for a formal statistical approach spawned numerous critical articles in the statistics literature. Greenberg (Citation1979) demonstrates that blind adherence to the 4/5ths Rule is likely to lead to larger false positive probabilities than 0.05 but without dramatically lowering the probabilities of false negatives. Boardman (Citation1979) replicates Greenberg’s analysis for the case where the number of people selected is not predetermined and his conclusions are similar to Greenberg’s. Neither of these articles considers the broader question of actually estimating the adverse impact ratio and evaluating its statistical significance. But there are many papers in the adverse impact statistics literature and a few in the disparate impact statistics literature that do.
A particularly useful compendium in the adverse impact statistics literature is the book edited by Morris and Dunleavy (Citation2017). The papers therein range from an introduction to the measurement of adverse impact in the EEOC context, to statistical issues of measurement and inference, to practical significance and perspectives on the evolution of case law regarding statistical evidence in adverse impact litigation. For our purposes we focus on papers in the Morris-Dunleavy volume that address measurement and statistical inference, plus papers published elsewhere that relate to the use of direct ratio estimation in disparate impact settings.
As mentioned in the previous section, a common approach in both adverse impact and disparate impact cases has been to test whether Q Q2 (adverse impact) or P
P2 (disparate impact) using the difference test and then to argue whether the observed ratio is significant from a practical point-of-view. In Morris and Lobsenz (Citation2000) the authors first promote the idea of estimating adverse impact with r* and then doing either a statistical test on a hypothetical value or range of values for R*, or reporting a confidence interval based on the logarithmic transformation of r*. They do an extensive study of the efficacy of this approach and the difference method, and conclude that at the conventional level of significance,
, both approaches have low power. Increasing α and/or using a one-tailed test are suggested. But their primary proposal is to estimate the adverse impact ratio and then construct a confidence interval around R*, which is precisely what we are proposing for the disparity ratio.
In Morris (Citation2001), the author generates sample sizes for the test of adverse impact either by the difference method or by the log-ratio method in order to achieve a predetermined level of power. The results are based on a very specific situation, namely, where the so-called “minority” and “majority” groups come from a common pool of applicants and the overall selection rate is known. As such, they are not transferable to our disparity ratio analysis. In Collins and Morris (Citation2008), the analysis is focused on alternative approaches for testing (Q Q
, and the 4/5ths Rule. As such, it is also of limited value for our purposes, although it does explore the efficacy of the difference approach based on the Normal approximation to the distribution of (q
q2) to other approaches that are more clearly suited for use in small samples. They conclude that the continued use of the difference approach is “reasonably well justified,” which is a useful finding for us going forward.Footnote25
Miao and Gastwirth (Citation2013) also focus on the test of difference in pass rates, the 4/5ths Rule and related tests in the pursuit of a prima facie case of discrimination. They do not consider the adverse impact ratio per se. Nor does Gastwirth (Citation2017) but he does raise (and analyze) some important issues in the debate over statistical and practical significance, which includes consideration of the adverse impact and odds ratios. He also bemoans the fact that the risk and benefits associated with case outcomes are generally ignored and beseeches the courts and/or Congress to address this shortcoming, which has implications for the choice of significance level.Footnote26 This is a particularly noteworthy paper owing to its depth of analysis and pertinency.
Fleiss, Levin, and Park (Citation2003) also cover the efficacy of the odds ratio based on the non-central hypergeometric distribution and an approximate method that relies on a normality assumption and results in a Chi-squared test. They note in their examples that the Chi-squared statistic for testing OR > 1 is always somewhat larger than the Chi-squared statistics for testing ln OR > 0, and thus the corresponding confidence intervals for OR based on the approximate method are somewhat wider than those based on ln OR. This is akin to our findings that the confidence interval for R based on the direct ratio estimator is wider than that based on ln R.
Finally, the efficacy of the direct ratio estimator, r, depends on sample size with regard to bias, the approximation to its variance, and its asymptotic Normal distribution. Fortunately, all three issues are addressed by Cho (Citation2013) in his development of an asymptotic confidence interval for r. He also produces tables that show the minimum sample sizes required to produce a confidence interval of a specific width for 90% and 95%, using various assumptions about the true value of R and the relationship between n1 and n2 defined by . A general rule of thumb emerges: A more precise interval is generated when a larger sample is taken from the “rare” population (smaller rejection rate). Fortunately, in disparate impact cases the sample sizes for the non-protected class (n2) are usually larger than for the protected class (n1).
We now move to discuss two examples of disparate impact with regard to housing discrimination. The first is taken from S & B from among the many such examples they consider. It involves the disparate impact on black households of the denial of Section 8 housing vouchers. We use this example to explore the relationships among the difference method, the direct ratio method and the log-ratio method for analyzing disparate impact. Next, we consider the matter of occupancy policy, specifically, whether a particular occupancy policy discriminates against families with children. This example uses data from San Diego County, California, and involves the introduction of the concept of “incremental disparity” as a way to evaluate a specific occupancy policy relative to either state or federal guidelines.
4 A Fair Housing Example
S&B cover a very comprehensive set of applications for the disparity ratio, many of which involve sample data and are therefore relevant for our purposes here.
One such application involves the analysis of income data by race, as one approach to determine whether a landlord’s “No Section 8” screening policy for potential renters is discriminatory. Under the federal Housing Choice Voucher (HCV) program, households apply for a voucher from their local public housing authority and, if they qualify, they are issued a HUD-funded voucher that covers their rent in excess of 30% of household income. While federal law bars discrimination against voucher holders in most government-assisted housing, it does not cover other landlords, and many have refused to honor vouchers.
One of the methods S&B use for analyzing the discriminatory impact of a “No Section 8” policy is to compare the groups of black and white households that are eligible for vouchers, to compute the corresponding disparity ratios, and to assess their statistical significance.Footnote27 An illustration in their article involves household income by race for the District of Columbia in 2014 using sample data from the American Community Survey (ACS).
shows the income distribution and disparity ratios by race for D.C. in 2014 below $50,000, which S&B assert is the relevant range, since at that time the “very low income” (VLI) limit of eligibility for four-person households was $53,500. The other relevant eligibility limit is “extremely low income” (ELI), which was $31,100 for four-person households at that time.Footnote28 The disparity ratios for these income intervals are all above 1.25.Footnote29 S&B also comment that one could compute confidence intervals for (P1–P2) in each stratum (which they don’t do) or first group the income strata to fit the general HUD income-eligibility limits and then proceed (which they do). We have done both, but we have used $10,000 width intervals.Footnote30
Table 1 District Of Columbia Income Ranges for Black and White households, 2014, with 95% one-sided confidence interval lower bounds.
The other columns of display the lower 95% confidence bounds for the test of difference, the direct ratio approach, and the log-ratio method. Since sample sizes are large (n ; n
), one would expect consistent results, and that’s what we have here: The difference method produces all positive lower bounds, signaling P
P2, and both ratio methods suggest R > 1. It is to be noted that while the two ratio methods produce similar values, the lower bounds from the log-ratio method are always greater than for the direct ratio method, which can be established mathematically.Footnote31
We have done a similar analysis for all 50 states, covering six values for α from 0.005 to 0.30 and the five income ranges in . This yields 1500 cases. We find that in 1.4% (21) of these cases, the difference approach and the log-ratio method give conflicting results (P P2 is implied while R > 1 is as well), whereas in none of those same cases is the direct ratio method inconsistent with the difference approach, including 71% of them (15/21 cases) where a negative bound is computed that would be set to zero. As expected, in all 1500 cases the lower bound derived from the log-ratio method is greater than that derived from the direct ratio method, but they are relatively close.
Sample sizes for these anomalous cases range from 10 to 52, so they are all fairly small. Most are below 30 and do not meet even the rule of thumb often used to establish the adequacy of the Normal approximation to the difference between two binomials.Footnote32 In these small sample situations it is also the case that the direct ratio estimator often behaves erratically vis-à-vis the log-ratio method, but even so, the direct ratio method is always consistent with the difference method. Without an extensive comparative study of the accuracy of the two ratio approaches as a function of sample size, Á la Cho (Citation2013), we are left in a quandary as to which approach to recommend except for large enough samples where, from a legal perspective, emphasis is placed on the more conservative of two competing approaches, and thus use of the direct ratio estimator is indicated.Footnote33 But because of the erratic behavior of the direct ratio estimator in small samples, say, less than or equal to 50, use of the log-ratio method would be prudent in such situations.
While S&B discuss the available data sources for use in evaluating FHA-impact claims, including the ACS, in their analysis of the D.C. income data they ignore that fact that each household in the sample has an associated weight that reflects how many households in the population are represented by it, that is, an inverse selection probability indicator. To incorporate such weights into the analysis is straightforward. The requisite formulas for the weighted estimator of a population proportion and its sampling variance are given in Appendix A, (A.10) and (A.12). These quantities are then incorporated into the formulas for confidence intervals for the difference approach (A.9c), the direct ratio approach (A.13c), and the log-ratio approach (A.16c). The results are shown in .
Table 2 District Of Columbia Income Ranges for Black and White households, 2014, with 95% one-sided confidence interval lower bounds.
What is immediately apparent is that the disparity ratios are all smaller with weights being incorporated, as are all the lower bounds. This latter observation reflects the fact that the sampling variances of the weighted estimators are always equal to or greater than the sampling variances of their unweighted counterparts. This manifests itself more in a comparison of the lower bounds for the direct ratio and log-ratio approaches in and than it does for the difference approach. But the actual sample design for the income data is somewhat more complex than merely a simple random sample of Bernoulli variables with weights.Footnote34 This can result in even larger variances.
For their published surveys, the Census Bureau provides margins of error (MOEs) for basic survey measures, like counts, and guidelines for the computation of MOEs for a variety of so-called “user-derived” proportions and ratios.Footnote35 But for our purposes published MOEs are not available and, moreover, sampling weights are involved. We used the statistical package Stata for our calculations, which relies on a procedure due to Demnati and Rao (Citation2004) for deriving the linearized variances for functions of survey data that are themselves continuous functions of the sampling weights. Essentially, variances for the weighted proportions involved are used in conjunction with the Appendix A formulas (A.9c) for the difference approach, (A.13c) and (A.14) for the direct ratio estimator, and (A.16c) and (A.17) for the log-ratio estimator. A further refinement to these more general variance estimators is available for a number of published estimates from the ACS using an approach called “variance replicate estimates,” whereby 80 pseudo-estimates for a given measured characteristic are used to produce a sampling variance. This approach does not depend on the variance approximations we rely upon. However, it represents a logical leap from the methodological path we have used herein, and so we have chosen not to develop it.Footnote36
The results are shown in . We observe that all the lower bounds are equal to or smaller than those for the more straightforward method that relies on the assumption of Bernoulli-generated data with weights, but the differences are all quite small, suggesting that the sample design underlying the income data is quite similar or at least not very impactful as regards the resulting sampling variances.
Table 3 District Of Columbia Income Ranges for Black and White households, 2014, with 95% One-Sided confidence interval lower bounds.
Whether using the Stata-generated variances or the more straightforward method when analyzing these results for the 50 states, there are more inconsistencies among the three approaches than we observed using the unweighted data, in particular between the difference approach and the log-ratio approach, but still the incidence of such cases is relatively small. While the difference approach and the direct ratio approach yield consistent inferences, for small samples the direct ratio approach behaves somewhat erratically, as before.
Moving on to the analysis of the Section 8 grouped income data, reproduces the relevant table in S&B where there are three income groups, <$25,000, <$35,000, and <$50,000, but where we have substituted the lower bounds from (A.9a) for their Z-scores and added results for the direct ratio and log-ratio approaches, for the 95% level of significance. Once again, the results are consistent, and remain so for a range of confidence levels from 99.5% to 70%. The log-ratio method still yields a slightly higher lower bound than the direct ratio method in all cases.
Table 4 District of Columbia Income Ranges for Black and White households, 2014, with 95% one-sided confidence interval lower bounds.
Summarizing the state-specific results for these income groupings, there are relatively fewer anomalous results () and all of them are situations where the difference method indicates P
P2, the direct ratio method suggests R < 1, and the log-ratio method indicates R > 1. Sample sizes range from 10 to 81, with 8/9 of them below 30. As expected, the lower bound derived from the log-ratio method is always slightly greater than that derived from the direct ratio method.
and display, respectively, comparable results for these calculations under the assumption of simple random sampling with weights and the actual ACS sample design with weights incorporated. Again, the disparity ratios are considerably lower when weights are used as are the lower bounds for both the direct ratio and log-ratio approaches. These same lower bounds are lower still for the ACS sample design than for simple random sampling with weights, but the differences are small.
Table 5 District of Columbia Income Ranges for Black and White households, 2014, with 95% one-sided confidence interval lower bounds.
Table 6 District of Columbia Income Ranges for Black and White households, 2014, with 95% one-sided confidence interval lower bounds.
5 Analyzing Housing Occupancy Restrictions
In order to illustrate our methodological approach further, we use an example involving rental housing in San Diego County, California, in 2014. Suppose an apartment complex we will refer to as the Torrey Pines or “TP” project has established occupancy restrictions as follows: Occupancy is limited to the number of bedrooms plus one. Thus, for a one-bedroom apartment the limit is two persons; for a two-bedroom apartment, three; and for a three-bedroom apartment, four. The question is whether households with children (the protected group) are differentially impacted by this policy compared to households without children.Footnote37 The analysis applies to multi-family apartment buildings, defined as having two or more units for rent.
Having considered the implications of using formulas based on simple random sampling when the underlying data are clearly derived from a more complex sample design in the previous example, here we proceed directly to an analysis based on weighted data. shows the weighted proportions needed for evaluating the TP occupancy policy, were it applied to households in San Diego Co. The disparity ratios are all quite large and they show the expected ordering: As household size increases, so does disparity.Footnote38 But rather than trying to determine whether they are also statistically significant, it is important to recognize that any occupancy restriction is likely to generate disparity between the two groups since households with children tend to be larger than those without. As a result, both the federal government and the states have adopted occupancy guidelines and it is with respect to them that any particular occupancy policy should be compared.
Table 7 Sample percentages, disparity ratios and sample sizes for evaluating the TP occupancy policy, San Diego Co., Multifamily Buildings, 2014.
At the federal level, the Fair Housing Act allows local governments to restrict the number of occupants in a rental unit so long as it is “reasonable,” does not discriminate against families, and is applied uniformly without regard to what constitutes a “family.” A common and “reasonable” occupancy limit in most circumstances is two persons per bedroom, and that is the standard we adopt here.Footnote39
Likewise, the California Employment and Housing Act prohibits discrimination against families with minor children in many circumstances.Footnote40 The related Uniform Housing Code specifies an occupancy limit in most circumstances of two times the number of bedrooms plus one, somewhat less restrictive than the federal guidelines.Footnote41 One approach to analyzing the TP occupancy policy relative to either federal or State guidelines is based on the data contained in . The idea is to compare statistically the disparity ratio R to either RF
or R
for one-bedroom, two-bedroom and three-bedroom units.
Table 8 Sample Percentages, disparity ratios and sample sizes for evaluating the TP occupancy policy relative to Federal and California guidelines, Multifamily Buildings, San Diego Co., 2014.
For one-bedroom units, the TP occupancy policy is identical to the Federal guidelines but is more restrictive than the California guidelines. But R is greater than R
, an anomaly. For two-bedroom units, the TP policy is more restrictive than either the Federal or California guidelines. Again, we have an anomalous situation in that R
RF
, but since R
R
, that case could be analyzed. Likewise, for three-bedroom units, where R
R
R
. The statistical analysis is to test whether R
/RF
or R
/R
is greater than one. Since R
/R
is akin to an odds ratio, the standard approach would be to test ln R
–ln R
= 0 versus ln R
ln R
. This is possible but, unlike the case of an odds ratio, the numerator and denominator are correlated, leading to the variance formula shown by (A.20a) in Appendix A for weighted data.
A more straightforward approach is based on the data of , which shows, for the Federal and California guidelines, the disparity ratios attributed to those family sizes that exceed the TP occupancy policy but meet the Federal and/or California guidelines. We label these “incremental disparity” and denote them by RI
. We then compare the relevant RI
to either RF
or R from . This is somewhat simpler than the usual approach based solely on the data from , since RI
is uncorrelated with R
. Here we either test ln RI
–ln R
versus ln RI
–ln R
or we compute the corresponding confidence interval. In this instance, the formula for the approximate variance of ln rI
–ln r
is given by (A.19c) in Appendix A for weighted data. We proceed with the incremental disparity approach first, recognizing that the two approaches should yield similar results.
Table 9 Sample percentages, disparity ratios and sample sizes for evaluating the TP occupancy policy relative to Federal and California guidelines, Multifamily Buildings, San Diego Co., 2014.
The results are reported in and , where we see that in two of the three cases considered, incremental disparity is statistically insignificant compared to the respective Federal or California guideline, whether we use simple random sampling with weights as the analytical approach or the more general ACS design approach, whose methodological differences were discussed in the previous section. From a legal perspective, in those instances we would conclude that the TP policy is not overtly discriminatory. For the third case, which involves three-bedroom rental units, the TP incremental disparity is significantly different from the California guideline at the level based on and p = 0.024 based on , but neither of these meets the level required when accounting for multiple comparisons with the simple Bonferroni correction.Footnote42 The preferred approach controls for the expected proportion of errors among rejected hypotheses via the so-called False Discovery Rate (FDR), in which event the third case is significant with an FDR of slightly less than 0.08.Footnote43 In all these cases, sample sizes are quite large, so we have assurance that both the Normal approximation and the approximate variance formulas are reliable.
Table 10 Test statistics and 95% confidence intervals for Comparing the TP occupancy policy to Federal and California guidelines, Multifamily Buildings, San Diego Co., 2014, using the incremental disparity approach, simple random sampling with weights.
Table 11 Test statistics and 95% confidence intervals for comparing the TP occupancy policy to Federal and California guidelines, Multifamily Buildings, San Diego Co., 2014, using the incremental disparity approach, ACS design with weights.
The corresponding lower bounds are 1.19 and 1.21, respectively. We note that the lower confidence bounds for the Stata/ACS approach are all slightly tighter than for simple random sampling with weights, but they are very close. This is the result of most (but not all) of the component sampling variances for the weighted proportions being slightly smaller for the latter approach, just what we found in the Fair Housing example.Footnote44
and contain a matching set of numbers using the more conventional approach of comparing R to R
.
Table 12 Test statistics and 95% confidence intervals for Comparing the TP occupancy policy to Federal and California guidelines, Multifamily Buildings, San Diego Co., 2014, using the conventional approach, simple random sampling with weights.
Table 13 Test statistics and 95% confidence intervals for Comparing the TP occupancy policy to Federal and California guidelines, Multifamily Buildings, San Diego Co., 2014, using the conventional approach, ACS design with weights.
Considering first a comparison between the two tables, the conclusion is similar to what we found in and , namely that the lower confidence bounds (and the Z-statistics) for the Stata/ACS approach are all slightly higher than for simple random sampling with weights, but here they are very close in all three cases.
Comparing the results between and , and and , the incremental disparity approach results in slightly lower Z-statistics and lower bounds whether we use calculations based on simple random sampling with weights or Stat/ACS, but they are very close.
6 Conclusions
There has been a wealth of academic work devoted to statistical inference for the adverse impact ratio in equal employment cases, much of which is relevant to the use of the disparity ratio in fair housing cases.
The law literature conveys two ways of testing for disparate impact, both of which are based on the difference between the numerator and denominator of the disparity ratio. We show how these two approaches are related and focus on one of them, namely, the traditional test of the difference between two binomial variates based on its approximate Normal distribution. While this test (or confidence interval) can be used to examine whether the numerator “rejection” rate is larger than its denominator counterpart, thereby establishing an indication of prima facie discrimination (or not), a more direct approach is to estimate the disparity ratio and put a confidence interval around it, primarily because the typical approach in case law and within government anti-discrimination guidelines is to use the difference test in order to establish statistical significance and then to use the observed disparity ratio as a measure of practical significance. There are circumstances, however, in which both the difference in rejection rates and their ratio are of interest, namely, where this disparity ratio is large but the absolute difference is small and, conversely, where the absolute difference is large in practical terms but the ratio is relatively small (close to one).
The modern statistics literature begins with the book by Fleiss, Levin, and Park (Citation2003), the first edition of which appeared in 1973. This work is devoted to statistical methods for rates and proportions, including various tests for the difference and the odds ratio. Of particular interest in this and several related papers in the areas of biostatistics and adverse impact are comparisons of the direct ratio estimator and tests or confidence intervals for it, to its (natural) logarithmic transformation. In particular, it is suggested that the log transformation be used in small samples.
These findings are consistent with our own work in a section devoted to an analysis of possible discrimination in a landlord’s refusal to honor Section 8 housing vouchers based on differences in black and white household incomes. We also demonstrate something that has been hinted at in the statistics literature, namely, that the confidence intervals based on the log transformation are generally narrower than those based on the direct ratio estimator. In addition, there can be conflicting results with the difference method in assessing statistical significance using the log transformation. However, the direct ratio estimator can behave erratically in small samples. Fortunately, the paper by Cho (Citation2013) provides guidance in determining what sample sizes are required for its efficacy, and we provide some additional guidance based on our experience with the income data. Importantly, we also incorporate household weights into the analysis.
Finally, in a section devoted to an analysis of the possible discriminatory impact on families with children of occupancy restrictions, we introduce the idea of “incremental disparity” by considering the additional disparity invoked by the particular occupancy policy relative to either federal or state guidelines. Again, weighted data are used. While there is no substantive difference between the results obtained from this approach and those obtained from the more conventional approach that compares the subject occupancy policy directly to government-imposed guidelines, the incremental disparity approach does have the slight advantage of relying on a somewhat less complicated approximate variance formula.
For convenience, all technical details have been relegated to an appendix. This includes the articulation of basic definitions and relationships, plus all the relevant formulas for the tests and confidence intervals used in the text. A second appendix has been devoted to a simple example of the choice of significance level for a test of the disparity ratio. This is important because in most of the cases involving disparate impact and the federal guidelines governing its use, a significance level of is recommended. We argue that accounting for the relative consequences of decision errors in such cases will, in most instances, lead to a different selection. The common choice of
thereby implies a set of relative consequences, via its impact on Type II error probabilities that, in all likelihood, do not reflect the actual costs of false positives (concluding there is no discrimination when it exists) versus false negatives (concluding that discrimination exists when it doesn’t).
In summary, we have provided improved statistical approaches for assessing disparate impact that we hope will be adopted by practitioners in an effort to improve the quality of decisions in fair housing cases.
Acknowledgments
We wish to thank Scott Morris and two anonymous referees for helpful comments on an earlier version.
Disclosure Statement
No potential conflict of interest was reported by the authors.
Notes
1 Specifically, the Fair Housing Act prohibits housing discrimination because of “race, color, national origin, religion, sex, familial status, [and] disability.” See U.S. Department of Housing and Urban Development, Housing Discrimination Under the Fair Housing Act (2023).
2 The disparate impact approach was first introduced in the U.S. Supreme Court case Griggs v. Duke Power Co., in 1971.
3 See also, Callison (Citation2016, pp. 424–428), and Glassman and Verna (Citation2016, p. 12), for a summary of HUD’s 2013 ruling regarding disparate impact liability. The use of disparate impact as an approach for demonstrating de facto discrimination in fair housing cases follows its establishment by the Supreme Court in equal employment opportunity cases.
4 S&B, pp. 698–700.
5 Disparity ratios have also been used or proposed to evaluate disparate impact in contexts that include government contracting (San Francisco BART, 2017; Celec et al. Citation2000), the criminal justice system (The Sentencing Project Citation2016), evictions (Hepburn et al. Citation2020), and placement exams used in undergraduate education (Poe et al. Citation2014).
6 However, a “rejection” rate is hardly an apt description of, say, the proportion of families with minor children that are impacted by maximum occupancy restrictions in an apartment complex. For ease of exposition, unless otherwise specified, the remainder of this article uses disparity ratio to mean the ratio of rejection rates used in fair housing cases.
7 In their Appendix C, S&B devote three pages to exploring this matter, but without the benefit of algebra. As a result, their treatment is somewhat opaque.
8 See the discussion following equation (A.9a) in Appendix A. For example, suppose the observed P1 rejection rate is 0.60, based on a sample of 200, and the observed P2 rejection rate is 0.48, based on a sample of 257. The calculated disparity ratio is 1.25 (). The lower bound of a two-sided 95% confidence interval for P1 is 0.532 and the upper bound of the corresponding interval for P2 is 0.541, so they overlap, and we could not reject the hypothesis that the disparity ratio is one (or less). But the lower bound for the corresponding two-sided 95% confidence interval for the difference (P1–P2) is 0.029, hence, on that basis we would reject the hypothesis that (P1–P
and conclude that the disparity ratio exceeds one.
9 So, in the previous example, the operative level of significance is 0.025, not 0.05. The matter of under what circumstances a two-tailed test is appropriate is considered in depth by Gutman (Citation2017) in his review of the case law relating to adverse impact. See also, Fienberg and Straf (Citation1982).
10 Following conventional statistical notation, a lower-case letter indicates the estimated value of its upper-case counterpart, the unknown parameter.
11 Consideration of Type II errors in statistical work supporting litigation is a fairly recent phenomenon. In their review of federal court cases from 1960 to early 1982, for instance, Fienberg and Straf (Citation1982) found mention of “Type I and Type II errors” only three times (as compared to 688 times for “median”).
12 The exact statement in the OFCCP guidelines is internally inconsistent and incorrect in one aspect (OFCCP 2020, p. 71558). The inconsistency occurs between the first criterion, which states that statistical significance is established when the disparity (difference) is two or more times larger than its standard error, and the other two criteria, whereby the Z-statistic has a value greater than two, or the p-value is less than 0.05. The error arises when the first criterion is described as “a standard deviation of two or more.” With regard to the choice of a significance level in situations where sample sizes are large but the difference is small, Gastwirth, et al. (Citation2021) recommend using instead of
(p. 83).
13 The 4/5ths Rule, which was at the outset used in lieu of statistical analysis, is still used as the “hinge point” for a determination of practical significance. In Isabel v. City of Memphis (2005), the U.S. Court of Appeals upheld a lower court’s decision that prima facie discrimination existed in the administration of a written test for promotion from sergeant to lieutenant in the Memphis police force based on the difference test even though the observed adverse impact ratio was above the 4/5ths Rule cutoff of 0.80.
14 Even though it would be unusual for the difference test to suggest Q Q2 while the ratio test indicated R* > 1, it can happen, as we illustrate in a subsequent section for the disparity ratio.
15 They cite an example from Oswald et al. (Citation2017) where q , q
, so that q
q
, but r*
, which is in the actionable range.
16 In Browne (Citation2017), there is an example where, although 98.60% of minority applicants passed a particular employment test compared to 99.95% for whites (no adverse impact according to the 4/5ths Rule), the failure rate for minority applicants was 28 times that for whites (disparity ratio). But in Black v. City of Akron (1987), the U.S. Court of Appeals dismissed an argument that rejection (or failure) rates should be used in Title VII cases.
17 US HUD (2013), p. 11460. The rule promulgated in US HUD (2020) amends these three “parts” slightly to bring them into closer compliance with the language and meaning of the Inclusive Communities case.
18 An important difference between equal employment opportunity (EEO) and fair housing (FHA) cases that may explain the lack of guidance regarding practical significance in the latter is that for EEO cases the affected party is a relatively small number of individuals rather than an entire class of households.
19 For the example in footnote #8, where the observed disparity ratio is 1.25, the lower bound of a one-sided 97.5% confidence interval based on (A.14) is 1.04. According to Peresie (Citation2009, p. 792), the combination of a calculated ratio in the actionable range along with the result of the difference test rejecting (P P
in favor of (P
P
would provide a prima facie case for disparate impact, but that is not supported by the correct test where, in this example, the lower bound for R is less than 1.25.
20 Cummings (Citation2009), p. 443.
21 See Robbins et al. (Citation2002). A case where the odds ratio is used to evaluate disparate impact is U. S. v. Johnson (2015), which involves alleged bias on the part of the Alamance Co., N. C. Sheriff’s Office in issuing a disproportionate number of traffic citations to Hispanic drivers.
22 In epidemiological applications, a two-sided confidence interval is normally used because “exposures” can have both harmful and beneficial effects.
23 The paper by Koopman (Citation1984) presents a fourth approach based on the Chi-squared distribution that must be calculated via an iterative procedure. Except for situations where p1 is either close to zero or close to one, his proposed method gives results similar to the log-ratio method.
24 A draft version was issued in 1976.
25 Collins and Morris (Citation2008), p. 470.
26 See Gastwirth (Citation2017), p. 199 and our Appendix B.
27 The racial designation of a household is based on the (self-identified) race of the head of household.
28 Subsequently, S&B do similar calculations based on income data for “family households” in order to overcome the obvious problem with assuming that these data accurately reflect incomes for four-person households, but that has its own set of issues. For our purposes, using the undifferentiated (by household size) income data is sufficient.
29 The disparity ratio for incomes above $50,000 drops to 0.54.
30 S&B use $5,000 width intervals above $10,000. We use $10,000 width intervals to economize on the number of cases to be considered in a subsequent analysis involving all 50 states.
31 See the discussion in Appendix A following (A.18). Fleiss, Levin, and Park (Citation2003) and others have observed a similar relationship between confidence intervals for the odds ratio derived by the direct ratio and log-ratio approaches.
32 See Fleiss, Levin, and Park (2016), p. 26 and footnote #46 in Appendix A.
33 With regard to the adequacy of the direct ratio estimator in this case, D.C. is unusual compared to other “states” in that there is a larger percentage of black people compared to whites. (This is still the case, but the percentage black has been declining.) This situation is not covered explicitly by Cho, but his other results suggest that in this instance the minimum sample size requirements for the adequacy of the variance approximation embedded in (A.13a) are less onerous.
34 See U.S. Department of Commerce and Bureau of the Census (2004) for a discussion of the sample design used.
35 U.S. Department of Commerce and Bureau of the Census (2020), Chapters 7 and 8.
36 See U.S. Department of Commerce, Bureau of the Census, Documentation for the 2017-2021 Variance Replicate Estimates Tables, 2022. It is of interest to note, however, that in this application the “variance replicate estimates” approach yields results that are very close to what we have called “ACS Design with Weights.”
37 Households with children are defined as those in which one or more minor children (children under 18 years of age) of the head of household reside.
38 In < i>Rhode Island Commission for Human Rights v. Graul</i> (Citation2015), a case involving precisely the same considerations here, the plaintiffs prevailed solely on the fact that the disparity ratios increased with family size and were large, being “…well above the 1.25…that separates the statistically significant from the insignificant” (an erroneous statement, for sure) and which defines “substantial impact” (the effect size matters too). This case is discussed in S&B, pp. 735–737. A similar case is Gashi v. Grubb & Ellis Property Management Services (2011), but by no means does this constitute an exhaustive list of cases of this sort, which probably number in the hundreds, but not all of which went to trial. It is also worth noting that in HUD’s 2013 standard for discriminatory effects, use of the disparity ratio is illustrated by reference to the disparate impact of occupancy restrictions on families with children.
39 A federal occupancy limit of two persons per bedroom is not specified in the FHA but rather is HUD’s interpretation. HUD “…believes that an occupancy policy of two person in a bedroom, as a general rule, is reasonable under the Fair Housing Act. See U.S. Department of Housing and Urban Development, Office of General Counsel, Memorandum from Frank Keating re: Fair Housing Enforcement Policy: Occupancy Cases (1991).
40 Excluded are elderly housing and housing owned by private clubs or religious organizations.
41 In both the federal and California guidelines, “reasonableness” is circumscribed by things like the size of bedrooms and common areas.
42 The Bonferroni correction for multiple comparisons aims to adjust the significance level of each of m tests to compensate for the fact that as the number of comparisons increases at a given α-level, it becomes more likely that a Type I error will be made. In our case, m = 3, so that
43 For the FDR calculation, one computes the critical p-values according to p* (i/m) D, where i is the rank of the p-value in question (from smallest to largest) and D is the FDR. In situations where the cost of a false negative is high (for us, this means that a discriminatory policy goes undetected and the burden continues to be borne by the protected class), the FDR should be set fairly high—say, 0.10 to 0.25. Not even with D
would our other two cases reach significance, however. The seminal paper on FDR is Benjamini and Hochberg (Citation1995).
44 One might expect the Stata/ACS approach to produce larger variances because it is not making a distributional assumption, but that seems to have had no effect.
45 A general rule of thumb for when the normal approximation to the binomial is adequate is and
. (See Fleiss, Levin, and Park Citation2003, p. 26.) If p = 0.5, for example, then both conditions are met if
. However, if p = 0.1, then we have
from the first condition and
from the second. Hence, both conditions are met only if
.
46 Since the sum or difference of two normally distributed random variables is also normally distributed, the adequacy of the normal approximation in this application should be judged by applying the rule of thumb in the previous footnote to p1 and p2, and taking the largest of the two minimum sample sizes as the threshold for evaluating whether n1 and n2 are of adequate size.
47 In both (A.9a) and (A.9b), α is used as the generic symbol for the level of significance of the one-sided test. The confidence probability associated with (A.9a) is therefore (). For a direct comparison to (A.8), the specific level of significance should be
.
48 See, for example, Kendall and Stuart (Citation1958), esp. p. 232, eq. (10.17).
49 The rule of thumb in footnote #46 suggests that both n1 and for adequacy of the Normal approximation for the difference (p1–p2), much less stringent.
50 Morris (Citation2001, p. 16) states that the log transformation results in a sampling distribution that is “more closely normal” than its direct ratio counterpart and cites a 1994 paper by Fleiss. Nowhere in Fleiss’ later book (2003) is this mentioned but he does provide evidence that the log-method is generally superior to the difference approach, as do Morris and Lobsenz (Citation2000), based on power considerations. No comparisons are made to the direct ratio estimator.
51 A third approach considered in the Katz et al. paper is based on the odds ratio or , which can also be manipulated to produce a lower bound for R. A follow-up paper by Koopman (Citation1984) compares the log-method to an approach he develops based on the Chi-squared distribution that requires an iterative solution.
52 The proof rests on the inequality 1–x < e for 0 < x < 1.
53 Fleiss, Levin, and Park (Citation2003, eq. (10.16), p. 239).
54 This approach was used in the Rhode Island case, as one example, and permeates the many cases covered in S&B’s comprehensive article.
55 S&B on p. 709 state: “Courts generally accept a confidence level of 95% as being statistically ‘significant”’. Much of Peresie’s (Citation2009) work focuses on this confidence level as well, but ultimately she recommends using 90%. In the litigation support literature, confidence intervals are preferred over p-values to quantify a reasonable degree of uncertainty for the quantity of interest. See Kaye and Freedman (Citation2011).
56 This calculation is based on (A.12), using Z and v(r)
, with p
, p
, n
, and n
. The test is done assuming R
. If the null hypothesis is rejected at level of significance α, then it will be rejected for any value of R < 1.20.
57 This happens because the calculated variance of R depends on them.
58 Collins and Morris (Citation2008, p. 470).
59 The law journal article by Peresie (Citation2009) computes sample sizes for the difference test to detect an “actionable value” for the ratio of selection rates, namely 0.80, and then to also achieve power of 0.80, but the assumptions used to accomplish this are “unrealistic” by her own admission (footnote #90, p. 788). She does not consider either the direct ratio method or the log-ratio method.
60 Gastwirth et al. (Citation2021), p. 83.
61 Gastwirth (Citation2017) bemoans the fact that the risk and benefits associated with case outcomes are generally ignored in adverse impact cases, a shortcoming that applies equally well to disparate impact cases.
62 “Hazard” is defined to be the probability that a particular consequence occurs times the cost of that consequence.
63 In all these examples, it is assumed that the consequences themselves do not depend on R. One can easily imagine, however, that the damages assessed for a landlord’s discriminatory practices would depend on how egregious was the disparity, as measured by R.
64 Among the relevant articles on this subject are Hora and Kelley (Citation1983), which presents Bayesian procedures for constructing confidence intervals for the odds ratio and the risk (disparity) ratio, and Agresti and Min (Citation2005), who evaluate the relative performance of Bayesian confidence intervals for the difference method, the risk (disparity) ratio, and the odds ratio.
References
- Agresti, A., and Min, Y. (2005), “Frequentist Performance of Bayesian Confidence Intervals for Comparing Proportions in 2 x 2 Contingency Tables,” Biometrics, 61, 515–523. DOI: 10.1111/j.1541-0420.2005.031228.x.
- Benjamini, Y., and Hochberg, Y. (1995), “Controlling the False Discovery Rate: A Practical and Powerful Approach to Multiple Testing,” Journal of the Royal Statistical Society, Series B, 57, 289–300. DOI: 10.1111/j.2517-6161.1995.tb02031.x.
- Black v. City of Akron, Ohio, U.S. Court of Appeals, Sixth Circuit, October 15, 1987.
- Boardman, A. E. (1979), “Another Analysis of the EEOCC ‘Four-Fifths Rule’,” Management Science, 25, 770–776. DOI: 10.1287/mnsc.25.8.770.
- Browne, K. R. (2017), “Pernicious P-Values: Statistical Proof of Not Very Much,” University of Dayton Law Review, 42, 113–163.
- Callison, J. W. (2016), “Inclusive Communities: Geographic Desegregation, Urban Revitalization, and Disparate Impact under the Fair Housing Act,” University of Memphis Law Review, 46, 421–434.
- Celec, S. E., Voich, D., Jr, Nosari, E. J., and Stith, M. T. Sr., (2000), “Measuring Disparity in Government Procurement: Problems with Using Census Data in Estimating Availability,” Public Administration Review, 60, 134–142. DOI: 10.1111/0033-3352.00072.
- Cho, H. (2013), “Approximate Confidence Limits for the Ratio of Two Binomial Variates with Unequal Sample Sizes,” Communications for Statistical Applications and Methods, 20, 347–356. DOI: 10.5351/CSAM.2013.20.5.347.
- Collins, M. W., and Morris, S. B. (2008), “Testing for Adverse Impact When Sample Size is Small,” The Journal of Applied Psychology, 93, 463–471. DOI: 10.1037/0021-9010.93.2.463.
- Cummings, P. (2009), “The Relative Merits of Risk Ratios and Odds Ratios,” Archives of Pediatrics & Adolescent Medicine, 163, 438–445. DOI: 10.1001/archpediatrics.2009.31.
- Demnati, A., and Rao, J. N. K. (2004), “Linearization Variance Estimators for Survey Data,” Statistics Canada, 30, 17–26.
- Department of Labor, Office of Federal Contract Compliance Programs. (2020), “Nondiscrimination Obligations of Federal Contractors: Procedures to Resolve Potential Employment Discrimination,” Federal Register, 85, 71553–71578.
- Equal Employment Opportunity Commission, et al. (1978), “Uniform Guidelines on Employee Selection Procedures,” Federal Register, 43, 38290–38315.
- Fienberg, S. E., and Straf, M. L. (1982), “Statistical Assessments as Evidence,” Journal of the Royal Statistical Society A, 145, 410–421. DOI: 10.2307/2982094.
- Fleiss, J. L., Levin, B., and Park, M. C. (2003), Statistical Methods for Rates and Proportions (3rd ed.), Hoboken NJ: Wiley.
- Gashi v. Grubb & Ellis Property Services, U.S. District Court, Connecticut, 2011.
- Gastwirth, J. L. (2017), “Some Recurrent Problems in Interpreting Statistical Evidence in Equal Employment Cases,” Law, Probability and Risk, 16, 181–201. DOI: 10.1093/lpr/mgx017.
- Gastwirth, J. L., Miao, W., and Pan, Q. (2021), “On the Interplay between Practical and Statistical Significance in Equal Employment Cases,” Law, Probability and Risk, 20, 69–87. DOI: 10.1093/lpr/mgac002.
- Glassman, A. M., and Verna, S. (2016), “Disparate Impact One Year after Inclusive Communities,” Journal of Affordable Housing, 25, 11–24.
- Greenberg, I. (1979), “An Analysis of the EEOCC ‘Four-Fifths’ Rule,” Management Science, 25, 762–769. DOI: 10.1287/mnsc.25.8.762.
- Griggs v. Duke Power Co., Supreme Court of the United States, 401 U.S. 424.
- Gutman, A. (2017), “Case Law Interpretations of Statistical Evidence regarding Adverse Impact,” in Adverse Impact Analysis, eds. S. B. Morris, and E. M. Dunleavy, pp. 349–362, New York: Routledge.
- Hepburn, P., Louis, R., and Desmond, M. (2020), “Racial and Gender Disparities among Evicted Americans,” Sociological Science, 7, 649–662. DOI: 10.15195/v7.a27.
- Hora, S. C., and Kelley, G. D. (1983), “Bayesian Inference on the Odds and Risk Ratios,” Communications in Statistics - Theory and Methods, 12, 725–738. DOI: 10.1080/03610928308828491.
- Isabel v. City of Memphis, U.S. Court of Appeals, Sixth Circuit, April 11, 2005.
- Katz, D., Baptista, J., Azen, S. P., and Pike, M. C. (1978), “Obtaining Confidence Intervals for the Risk Ratio in Cohort Studies,” Biometrics, 34, 469–474. DOI: 10.2307/2530610.
- Kaye, D. H., and Freedman, D. A. (2011), “Reference Guide on Statistics,” in Reference Manual on Scientific Evidence, pp. 211–302, Washington, D.C: National Academies Press.
- Kendall, M. G., and Stuart, A. (1958), The Advanced Theory of Statistics (Vol. 1), London: Griffin.
- Koopman, P. A. R. (1984), “Confidence Intervals for the Ratio of Two Binomial Proportions,” Biometrics, 40, 513–517. DOI: 10.2307/2531405.
- Miao, W., and Gastwirth, J. L. (2013), “Properties of Statistical Tests Appropriate for the Analysis of Data in Disparate Impact Cases,” Law, Probability and Risk, 12, 37–61. DOI: 10.1093/lpr/mgs032.
- Morris, S. B., and Dunleavy, E. M., eds., (2017), Adverse Impact Analysis, New York: Routledge.
- Morris, S. B. (2001), “Sample Size Required for Adverse Impact Analysis,” Applied HRM Research, 6, 13–32.
- Morris, S. B., and Lobsenz, R. E. (2000), “Significance Tests and Confidence Intervals for the Adverse Impact Ratio,” Personnel Psychology, 53, 89–111. DOI: 10.1111/j.1744-6570.2000.tb00195.x.
- Murray, I. V., and Cornelius, J. (2014), “Promoting ‘Inclusive Communities’: A Modified Approach to Disparate Impact under the Fair Housing Act,” Louisiana Law Review, 75, 212–258.
- Newman, S. C. (2001), Biostatistical Methods in Epidemiology, New York: Wiley.
- Oswald, F. L., Dunleavy, E. M., and Shaw, A. (2017), “Measuring Practical Significance in Adverse Impact Analysis,” in Adverse Impact Analysis, eds. S. B. Morris, and E. M. Dunleavy, pp. 92–112, New York: Routledge.
- Paetzold, R. L., and Willborn, S. L. (2011), The Statistics of Discrimination: Using Statistical Evidence in Discrimination Cases, Eagan, MN: West Publishing.
- Peresie, J. L. (2009), “Toward a Coherent Test for Disparate Impact Discrimination,” Indiana Law Journal, 84, 773–802.
- Poe, M., Elliot, N., Cogan, J. A., Jr,., and Nurudeen, T. G. Jr, (2014), “The Legal and the Local: Using Disparate Impact Analysis to Understand the Consequences of Writing Assessment,” College Composition and Communication, 65, 588–611.
- Rhode Island Commission for Human Rights v. Graul, U.S. District Court, Rhode Island, August 13, 2015.
- Robbins, A. S., Chao, S. Y., and Fonseca, V. P. (2002), “What’s Relative Risk? A Method to Directly Estimate Risk Ratios in Cohort Studies of Common Outcomes,” Annals of Epidemiology, 12, 452–454. DOI: 10.1016/s1047-2797(01)00278-2.
- Ruggles, S., Flood, S., Foster, S., Goeken, R., Pacas, J., Schouweiler, M., and Sobek, M. (2021), IPUMS USA: Version 11.0 [Dataset], Minneapolis, MN: IPUMS.
- San Francisco Bay Area Rapid Transit District Disparity Study Volume I, January 12, 2017. Available at https://www.bart.gov/sites/default/files/docs/VI.BART%20Final%20Report.Volume%20I.1.12.2017_0.pdf
- Schwemm, R. G., and Bradford, C. (2016), “Proving Disparate Impact in Fair Housing Cases after Inclusive Communities,” NYU Journal of Legislation and Public Policy, 19, 685–770.
- Texas Department of Housing and Community Affairs vs. The Inclusive Communities Project, Supreme Court of the United States, 576 U.S. 519, 2015.
- The Sentencing Project. (2016), “Reducing Racial Disparity in the Criminal Justice System: A Manual for Practitioners and Policymakers,” (2nd ed.). Available at https://www.sentencingproject.org/wp-content/uploads/2016/01/Reducing-Racial-Disparity-in-the-Criminal-Justice-System-A-Manual-for-Practitioners-and-Policymakers.pdf.
- U.S. Department of Commerce, Bureau of the Census, (2004), PUMS Accuracy of Data (2003), pp. 10–12, Washington DC: U.S. Government Printing Office.
- U.S. Department of Commerce, Bureau of the Census. (2020), Understanding and Using American Community Survey Data: What All Data Users Need to Know, available at https://www.census.gov/content/dam/Census/library/publications/2020/acs/acs_general_handbook_2020.pdf.
- U.S. Department of Commerce, Bureau of the Census. (2022), Documentation for the 2017-2021 Variance Replicate Estimates Tables, available at https://www2.census.gov/programs_surveys/acs/replicate_estimates/2021/documentation/5-year/2017-2021_variance_replicate_table_documentation.pdf.
- U.S. Department of Housing and Urban Development. (1991), “Office of General Counsel, Memorandum from Frank Keating re: Fair Housing Enforcement Policy: Occupancy Cases,” March 20, available at https://www.hud.gov/sites/documents/DOC_7780.pdf.
- U.S. Department of Housing and Urban Development. (2013), “Implementation of the Fair Housing Act’s Discriminatory Effects Standard,” Federal Register, 78, 11459–11482.
- U.S. Department of Housing and Urban Development. (2020), “Implementation of the Fair Housing Act’s Disparate Impact Standard,” Federal Register, 85, 60288–60333.
- U.S. Department of Housing and Urban Development. (2023), Housing Discrimination Under the Fair Housing Act, available at https://hud.gov/program_offices/fair_housing_equal_opp/faiir_housing_act_overview.
- United States v. Johnson, U.S. District Court, North Carolina, August 7, 2015.
- Upton, G. J. G. (1982), “A Comparison of Alternative Tests for the 2x2 Comparative Trial,” Journal of the Royal Statistical Society, 145, 86–105. DOI: 10.2307/2981423.
- Viera, A. J. (2008), “Odds Ratios and Risk Ratios: What’s the Difference and Why Does It Matter?,” Southern Medical Journal, 101, 730–734. DOI: 10.1097/SMJ.0b013e31817a7ee4.
Appendix A.
Formulas and Relationships
A.1. Definitions of R and R*, and their relationship
Let the numerator proportion of the disparity ratio based on so-called “rejection” rates be denoted P1 and the denominator proportion be denoted P2. Then,
(A.1)
(A.1)
By contrast, the 4/5ths Rule used in employment discrimination cases is defined as
(A.2)
(A.2)
where
and
Their relationship can easily be derived algebraically. The results are:
(A.3a)
(A.3a)
and
(A.3b)
(A.3b)
A.2. Nonoverlapping Confidence Intervals
In this approach, one compares the upper bound of a two-sided confidence interval for P2 to the lower bound of a two-sided confidence interval for P1. This could be done using binomial distributions, but for consistency in what follows we employ the normal approximation.
For sample 1, we denote sample size as n1 and the observed rejection rate as p1. The estimated variance of p1 is
(A.4)
(A.4)
where
, and the two-sided confidence interval around the true value P1 is
(A.5)
(A.5)
where
is the standard normal ordinate that corresponds to a cumulative probability of
in the tail of the normal distribution.Footnote45
Similarly, for sample 2, the analogous confidence interval is
(A.6)
(A.6)
Comparing the lower bound in (A.5) to the upper bound in (A.6), the two confidence intervals do not overlap if:
(A.7a)
(A.7a)
or
(A.7b)
(A.7b)
consistent with the hypothesis that the disparity ratio is at least one. But because only one side of each confidence interval is involved, the confidence probability associated with (A.7b) is actually
, not
. Since the two samples are assumed to be independent, a confidence interval equivalent of (A.7b) is
(A.8)
(A.8)
being a one-sided confidence interval for the difference
with probability
.
A.3. Confidence Interval for (
)
However, this is not the correct confidence interval for the difference , which uses the sum of the variances, namely:Footnote46
(A.9a)
(A.9a)
It can be shown algebraically that the variance term in (A.9a) is always less than or equal to the variance term in (A.8) and so the lower bound (A.9a) is always greater than or equal to (A.8).
But for testing the hypothesis the following variant is typically used, namely:
(A.9b)
(A.9b)
where
.Footnote47
In effect, a common variance is assumed for the estimated difference because, under .
A.4. Confidence Interval for (
) Using Weights
In a simple random sample of independent Bernoulli variables , each with mean P, variance
, and known weights wi
, the weighted sample proportion is
(A.10)
(A.10)
where the weights are normalized to add to one. Under these assumptions, the sampling variance of
is
(A.11)
(A.11)
Since P is unknown, we use the estimate of it, , to get:
(A.12)
(A.12)
in which case (A.9a) becomes:
(A.9c)
(A.9c)
Note that if for all i in (A.10) and (A.12), then
,
, and (A.9c) becomes (A.9a). Also, because
from the Cauchy-Schwarz Inequality, in (A.12),
.
A.5. Confidence Interval for the Disparity Ratio 

The variance of a so-called ratio estimator, in this case , is approximated using standard techniques.Footnote48 This derivation for independent samples produces the following formula for v(r), the estimated variance of r:
(A.13a)
(A.13a)
which, for unweighted data, becomes:
(A.13b)
(A.13b)
and for weighted data,
(A.13c)
(A.13c)
where
and
are given by (A.10) and (A.12), respectively.
A one-sided confidence interval follows easily, and takes the form (for generic r):
(A.14)
(A.14)
For hypothesis testing purposes, one would compare a preselected value for R, say , to the interval in (A.14). The hypothesis R
R0 versus R > R0 is rejected if R0
falls outside (is less than) the interval. The implied level of significance for the test (probability of a Type I error) is at most α.
Expressed as a test of significance, this corresponds to: Reject :
in favor of
:
if
(A.15)
(A.15)
with probability α. Note that if the hypothesis is rejected for
, it will be rejected for any other
. In principle, the difference test
versus
and the ratio test
versus
should yield identical results for large samples. But they rest on somewhat different distributional assumptions and implementation of the ratio test also involves an approximate variance.
For this approach, the adequacy of sample size is even more important than with the test of difference. First, in small samples, r is a biased estimator for R, but the bias disappears asymptotically—that is, as sample size gets larger. Second, as was the case with the normal approximation to the binomial distribution, the approximation for the distribution of r improves as sample size increases. Finally, the efficacy of (A.13b) also depends on sample size, but all three issues are addressed by Cho (Citation2013) in his development of an asymptotic confidence interval for r. He also produces tables that show the minimum sample sizes required to produce a confidence interval of a specific width for 90% and 95%, using various assumptions about the true value of R and the relationship between n1 and n2 defined by .
For example, suppose R = 1.5, with and
. In order to generate a valid 95% confidence interval for R of
with
, we would need a minimum sample size of
(thus,
).Footnote49 These minimums decrease going from 95% to 90% and as the specified margin of error increases. A general rule of thumb emerges: A more precise interval is generated when a larger sample is taken from the “rare” population (in this example, P2). Fortunately, in disparate impact cases the sample sizes for the non-protected class (n2) are usually larger than for the protected class (n1).
In the statistics literature devoted to estimating adverse impact, the complement of disparate impact, however, preference is expressed for first transforming R into ln R, proceeding to develop a confidence interval for it and then taking an antilog at the very end of the process. For this, we first approximate the variance of , which is given by
(A.16a)
(A.16a)
and is estimated by
(A.16b)
(A.16b)
for unweighted data and, for weighted data,
(A.16c)
(A.16c)
with
. and
given by (A.10) and (A.12), respectively.
A one-sided ) confidence interval takes the form (for generic r):
(A.17)
(A.17)
For the corresponding test of significance, we would reject H0: in favor of Ha
:
if
(A.18)
(A.18)
It is to be noted that if ln p is assumed to be normally distributed, then itself is lognormal. As an approximation to the exact distribution of p, the lognormal does have the attractive feature of being bounded below by zero, but otherwise it is not clear which distributional assumption is to be preferred.Footnote50
An early paper by Katz et al. (Citation1978) that is motivated by biometric applications involving the “risk ratio,” compares one-sided confidence intervals based on the ln r approach to one that solves for a lower bound on R using the approximate Normal distribution of (), which is not equivalent to our direct ratio approach.Footnote51 There seems to be no work extant that specifically evaluates the efficacy of the direct ratio estimator for R, as in Cho’s paper, to the log-method, even in the more extensive statistics literature on adverse impact. But, interestingly, it can be shown that if
, then the lower bound in (A.17) is always greater than the lower bound in (A.14).Footnote52
A.6. Incremental Disparity
The analysis of “incremental disparity” involves the quotient of two disparity ratios, and
, estimated by
,
, and
. While
as a direct ratio estimator could be used for this purpose and its approximate variance derived, since it is akin to an odds ratio the more common log-method is used.
With r1 and r2 uncorrelated, the estimated approximate variance of ( is given by:
(A.19a)
(A.19a)
For unweighted data, (A.19a) becomes:
(A.19b)
(A.19b)
(A.19b)
(A.19b)
which is of the same basic form as the approximate variance for the log odds ratio.Footnote53
For weighted data, (A.19a) becomes:
(A.19c)
(A.19c)
(A.19c)
(A.19c)
where
. and
are given by (A.10) and (A.12), respectively.
If r1 and r2 are correlated, however, the estimated approximate variance of ( is
(A.20a)
(A.20a)
For the particular correlation structure described in the text, we have cov ( and cov (
,
. But since
and
, cov (
) and cov (
,
, so that,
For unweighted data, (A.20b) becomes:
(A.20c)
(A.20c)
(A.20c)
(A.20c)
while for weighted data we would use:
(A.20d)
(A.20d)
where
. and
are given by (A.10) and (A.12), respectively.
From these, one could form the test statistic
(A.21)
(A.21)
or form a one-sided (
) confidence interval for
,
(A.22)
(A.22)
expressed for generic r.
Appendix B.
The Choice of Significance Level: A Simple Example
Subsequent to the reliance on an observed disparity ratio of at least 1.25 to show prima facie discrimination in situations involving rejection rates, a common practice was to use the difference method in order to test the significance of an observed disparate impact and then to discuss the practical implications of the disparate “effect” as measured by the disparity ratio.Footnote54 But putting a one-sided confidence interval around the true disparity ratio instead alleviates the need to do the former and refines the disparate “effect” by putting it into a statistical context, as we have recommended. Using the () lower confidence bound as an indicator of the likely minimum for R and to decide whether R > 1 based on it is tantamount to performing a formal statistical test with significance level α, as is well-known.
In the extant case-related literature dealing with statistical testing for the disparity ratio or the 4/5ths Rule, a confidence probability of 95% is usually chosen. This seems to be in accord with legal practice.Footnote55 Used as a device for statistical testing, this implies a level of significance for the corresponding test of 5%, which reflects the probability of committing a so-called Type I error, namely, rejecting the null hypothesis when it is true 5% of the time. In the case of testing H0: R R0 versus Ha
: R > R0, this means there is no actionable disparate impact but we conclude that there is. The consequence of this error falls on the defendant. Presumably, in addition to being required to conform to the law, there would be fines or other damages assessed. While it may be difficult to predict what the precise monetary consequences of this outcome might be in advance, at least there would be publicly known judgments in similar cases to rely on, if only to put a reasonable range on them. The probability of making this sort of error can be easily controlled. But any choice of the level of significance has implications for another decision error (Type II), namely, accepting that R
R0 when it is false. The consequences of this error fall on the plaintiff, whereby there continue to be implications for the protected class from a discriminatory policy, the damages from which would be necessary to assess in order to embark on an analysis of the proper “balance” between the probabilities of making a Type II error (which depend on the true value of R) and the probability of making a Type I error.
Nevertheless, it is instructive to explore these matters, if only with hypothetical data. To begin, we consider the test for the disparity ratio of R R0 versus the alternative hypothesis R > R0 as set forth in (A.12) and the discussion that follows. Using the illustrative data in footnote #10, if R
, then using a 5% level of significance we would reject the hypothesis R
in favor of the alternative R >1.20 if the observed value of R is greater than 1.38.Footnote56 Since r is 1.25, we would not reject the hypothesis.
“Power” is defined as one minus the probability of a Type II error. The power of the test depends on specific values for R that are greater than 1.20. For example, if R , we would calculate the area under the normal distribution with mean 1.25 from 1.38 to infinity. This gives a cumulative probability of 0.115, so in this case our test is not very “powerful.” As R takes on larger values, the test is able to detect the difference between them and R
increasingly well. In order to increase power over the entire range of Ha
: R > R0, a plaintiff would want to increase the significance level. At
, the test still has a difficult time differentiating between values for R that are close to 1.20, but power increases substantially as we move toward larger value for R.
Power can be improved with increases in sample size, but that is not something we can control, as the data used in disparity cases generally come from secondary sources. Power also varies somewhat according to the values of the observed rejection rates that combine to produce the observed disparity ratio, but that is not a choice either.Footnote57 What is under our control is the choice of a significance level for the test. If we increase the significance level, then power improves, as shown in .
In the adverse impact literature, a good deal of work has been done, primarily by Morris and his coauthors, on the matter of power, sample size, etc., both as regards the difference method and the log-ratio method. In Collins and Morris (Citation2008), for instance, the authors evaluate the efficacy of the difference method to Fisher’s Exact Test and a test proposed by Upton (Citation1982) that involves an adjustment to the usual Chi-squared statistic in 2 × 2 contingency tables (which is equivalent to the difference method) for use in small samples. They conclude that the difference method provides a “good balance of maintaining the nominal Type I error rate and maximizing power.”Footnote58 In other papers (Morris Citation2001; Morris and Lobsenz Citation2000), Morris and his coauthors have considered the sample size requirements to meet a pre-specified power level for tests using the log adverse impact ratio, and a comparison of their power vis-à-vis the difference method. In the first instance, the research is not directly transferable to our disparity ratio analysis because it relies on a very specific case, namely where the minority and majority groups come from a common pool of applicants and where the overall selection rate is known. In the second, which is germane, Morris and Lobsenz (Citation2000) find that the log-ratio method has slightly better power than the difference method when the proportion of minority applicants is small, but both approaches produce low power under “common conditions,” which we take to mean modest sample size and a typical level of significance, for example, .Footnote59 In their conclusions, these authors mention the possibility of increasing α in order to provide a better “balance” between Type I and Type II errors but stop there.
Gastwirth et al. (Citation2021) also discuss balancing Type I and Type II errors in the context of employment discrimination, but without regard to the relative costs of these decision errors. But to their credit, they emphasize that “…courts should consider the Type II error in addition to statistical significance at the 0.05 level before relying on a nonsignificant statistical test as a major factor in rejecting a plaintiff’s claim.”Footnote60
Balancing the significance level and the probability of making a Type II error involves two things: an assumed value for R > R0 and the relative consequences of making the two types of error.Footnote61 For example, suppose the consequences are identical in terms of cost and R . Using , it is seen that the two error probabilities are balanced when the significance level is approximately 0.08. Next, suppose the cost of making a Type II error (continuing an unfair housing practice) is judged to be twice as consequential as the cost of making a Type I error (landlord unfairly pays fines or damages). Then one must balance the “hazards” associated with the two decision errors, which is achieved when, at an assumed value for R > R0, the probability of making a Type I error is twice as large as the probability of making a Type II error (1–power).Footnote62 From , for R
this occurs at a significance level of approximately 0.12, where the probability of making a Type II error is 0.06, just about half as large. In this light, the adoption of an arbitrary (though common) level of significance of
implicitly reflects the relative consequences involved. For the numbers shown in , if, for instance, R
, the probability of making a Type II error is 0.427, thus, it is implied that the consequences of making a Type I error must be 8.5 times that of making a Type II error.Footnote63
Of course, the actual value of R is unknown. But there may be prior knowledge as to its value in similar cases and/or a willingness to specify a distribution for it based on prior knowledge, which would allow a Bayesian approach to be used to determine an optimal choice of significant level. But that analysis is beyond the scope of this article.Footnote64
Table B.1 Power versus significance level for the test, R .