Abstract
Recent application studies of deep reinforcement learning (DRL) in power electronic systems have successfully demonstrated its superiority over conventional model-based control design methods, stemming from its adaption and self-optimisation capabilities. However, the inevitable gap between offline training and real-life application presents a significant challenge for practical implementation, owing to its insufficient robustness. With this in mind, this paper proposes a novel robust DRL controller by fusing an extended state observer (ESO) for the DC–DC buck converter system feeding constant power loads (CPLs). To be specific, the mismatched lumped terms are reconstructed by an ESO in real time, and then fed forward into the agent's action, aiming to improve the adaptability to parameter variations of the real-life converter systems. By carefully conducting simulation and experimental tests, the robustness enhancement ability of the proposed framework compared with model-free DRL and conventional PI controllers are clearly verified.
Disclosure statement
No potential conflict of interest was reported by the authors.
Additional information
Funding
Notes on contributors
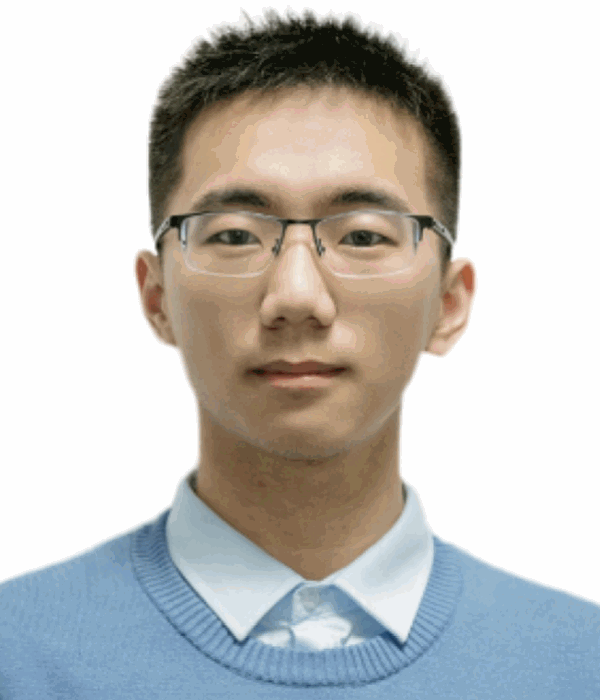
Tianxiao Yang
Tianxiao Yang received the B.E. degree in electrical engineering from Shenyang University of Technology, Shenyang, China, in 2020. He is currently working toward the Master's degree in automatic control from Shanghai University of Electric Power. His research interests include deep reinforcement learning theory and its applications for microgrid and power systems.
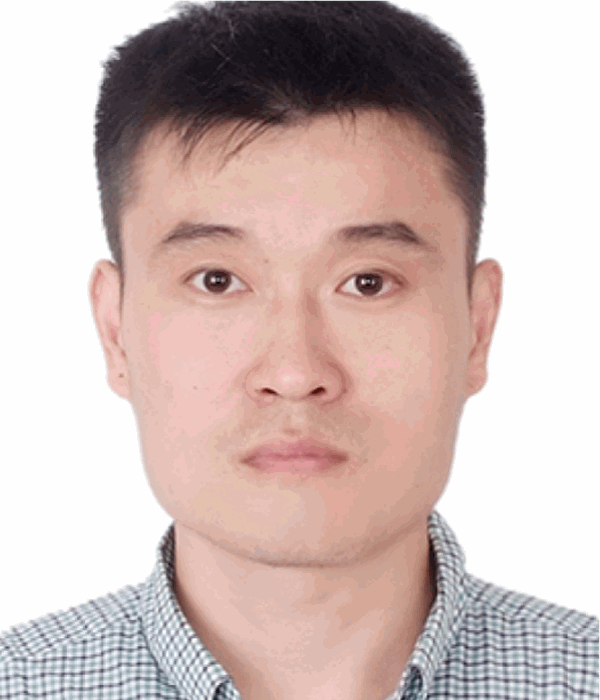
Chengang Cui
Chengang Cui received the B.E. degree in automation engineering from Jilin University, China, in 2004, the Ph.D. degree in control theory and control from Zhejiang University, China, in 2010. He worked at Shanghai Institute for Advanced Studies, Chinese Academy of Sciences, engaged in energy management and optimal scheduling from 2012 to 2015. He has been with the School of Automation, Shanghai University of Electric Power, where he is currently a associate professor. His research interests cover the control and schedule of renewable energy systems and microgrid.
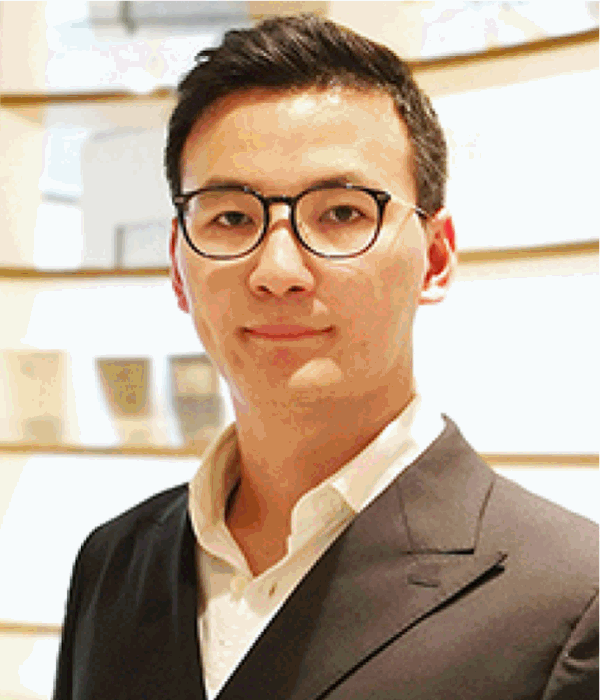
Chuanlin Zhang
Chuanlin Zhang received the B.S. degree in mathematics and the Ph.D. degree in control theory and control engineering from the School of Automation, Southeast University, Nanjing, China, in 2008 and 2014, respectively. He was a Visiting Ph.D. Student with the Department of Electrical and Computer Engineering, University of Texas at San Antonio, USA, from 2011 to 2012; a Visiting Scholar with the Energy Research Institute, Nanyang Technological University, Singapore, from 2016 to 2017; a visiting scholar with Advanced Robotics Center, National University of Singapore, from 2017 to 2018. Since 2014, he has been with the College of Automation Engineering, Shanghai University of Electric Power, Shanghai, where he is currently a Professor. His research interests include nonlinear system control theory and applications for power systems.
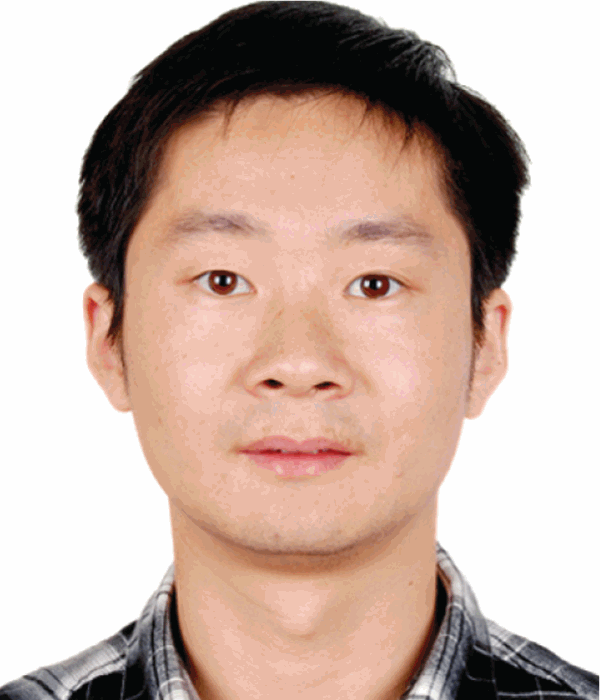
Jun Yang
Jun Yang received the B.Sc. degree in automation from the Department of Automatic Control, Northeastern University, Shenyang, China, in 2006, and the Ph.D. degree in control theory and control engineering from the School of Automation, Southeast University, Nanjing, China, in 2011. He joined the Department of Aeronautical and Automotive Engineering at Loughborough University from 2020 as a senior lecturer. His research interests include disturbance estimation and compensation, and advanced control theory and its application to flight control systems and motion control systems. He is a fellow of IET.