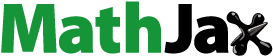
Abstract
In several problems of decision-making under uncertainty, it is necessary to study the sign of the covariance between marginal utilities. All of the works that study the covariance signs are based on Chebyschev’s integral inequality. However, this inequality requires that both functions be monotonic. There are many cases, originated basically by new alternative theories, which assume that the marginal utilities of interest are non-monotonic. Thus, we cannot use Chebyschev’s result as it relies on monotonic functions. In this article, I derive some new covariance inequalities for utility functions which have non-monotonic marginal utilities. I also apply the theoretical results to two problems in economics: First, I study some properties of the indiference curve in the mean-variance space for Prospect Theory and for Markowitz utility functions. Second, I analyze the asset hedging policies of a bank that behaves as predicted by Prospect Theory.
Public Interest Statement
In this article, I find new covariance inequalities for non-monotonic functions. These findings help me to explain why the investors trade-off between risk and return still hold under non-expected utility theories. Additionally, the results show that hedging policies of banks that use S-shaped could not very different from those that use the traditional concave utility function.
1 Introduction
Many problems of choice under uncertainty involve studying the sign of a covariance. In particular, many times it is necessary to determine the sign of the covariance of two real functions and
of a random variable
:
(1)
(1) The sign of (1) is deduced with the following argument: If these two functions are increasing (or both are decreasing), its sign is non-negative, while if one function is increasing and the other is decreasing the sign is non-positive (cf. e.g. Gurland, Citation1967; Lehmann, Citation1966; McEntire, Citation1984; Schmidt, Citation2014). This argument relies on Chebyshev’s integral inequality (cf. e.g. Mitrinovic & Vasic, Citation1970; Simonovits, Citation1995).
We shall see that there are some economic problems where or
is a marginal utility function. For instance, suppose that
is an increasing and concave utility function, then setting
and
Equation (1) can be written as follows
(2)
(2) In this particular case, as
is an increasing function and
is a decreasing function (given the concavity of
), the sign of (2) is non-positive. This particular case of (1) has been used in many papers in economics. For instance, Sandmo (Citation1971) studies the sign of covariance (2) to characterize the conditions under which a competitive firm, facing an uncertain price, would produce more or less than under certainty. Similarly, Batra and Russell (Citation1974) use this tool to analyze the effect of international price uncertainty over the social welfare of a small country with two goods. While Mossin (Citation1968) uses this covariance sign to show that full insurance is optimal at an actuarial fair price and partial insurance is optimal if the premium includes a positive loading.
Nevertheless, Chebyshev’s integral inequality crucially depends on the monotonic behavior of both functions. Sometimes this assumption does not hold. For instance, Wagener (Citation2006) studies the sign of an expression similar to (2), which involves a non-monotonic function, to derive some results of comparative statics under uncertainty. Besides, other types utility functions have non-monotonic marginal utilities. For instance, prospect theory (Kahneman & Tversky, Citation1979) proposes an S-shaped utility function, which means the marginal utility is non-monotonic. On the other hand, Markowitz (Citation1952) proposes a utility function that, in its simplest case, is reverse S-shaped (RS-shaped), implying that the marginal utility is also non-monotonic. Therefore, Cheyshev’s integral inequality does not work for marginal utilities of S-shaped or RS-shaped utility functions.
This paper contributes in the following. First, I derive some new covariance inequalities involving non-monotonic functions. In particular, I study the sign of covariance (2) for S-shaped and RS-shaped utility functions. Second, I apply these new results to two problems in economics. In the first application, I study the shape of the mean-variance indifference curves for S-shaped and RS-shaped utility functions. In this application, I study whether the monotonicity of the indifference curve in the continues to hold for these types of utility functions. Finally, I establish the optimal strategies for hedging asset price risk within prospect theory. Specifically, I examine the optimal strategy for an enterprise that behaves according to prospect theory.
The paper continues as follows. In the next section, I give a brief view of non-monotonic marginal utility functions. In Section 3, I present some previous covariance inequalities. In Section 4, I derive the main results of the paper. In Section 5, I present the applications noted above. Section 6 provides concluding remarks.
2 Non-monotonic marginal utility functions
In this section, I give a brief introduction to different types of non-monotonic marginal utility functions. As we have seen, a utility function, can take on various shapes: concave, convex, S-shaped, and reversed S-shaped, among others. For a discussion of different forms of the utility function, I refer to Alghalith (Citation2010) and Gillen and Markowitz (Citation2009).
Friedman and Savage (Citation1948) are among the first to propose an utility function different from the classical Bernoulli utility type. Why individuals buy lotteries (reflecting risk seeking) and purchase insurance (reflecting risk aversion) at the same time.
Markowitz (Citation1952) criticizes Friedman and Savage’s proposal and posits an alternative model that modifies the shape of the utility function. In particular, he proposes a utility function in which the domain is all the real line. This utility function starts convex, then turns concave with an inflection point at the origin, becoming convex and finishing concave. For simplicity, many authors have used a reverse-shaped type utility function with only one inflection point at the origin, (cf. e.g. Egozcue, Fuentes García, Wong, & Zitikis, Citation2011; bib155). Although Markowitz’s proposal is appealing, there is mixed empirical evidence to support this theory (e.g. Hershey & Schoemaker, Citation1980; Loubergé & Outreville, Citation2001; Post & Levy, Citation2005; Reilly, Citation1986).
Egozcue et al. (Citation2011) use a power function to represent an RS-shaped utility function given by(3)
(3) where
.
The marginal utility is decreasing for negative values of and increasing for positive values of
. This corresponds with the assumption that the decision maker is risk averse in its negative domain and is risk seeker in its positive domain.
Prospect theory is one of the most famous alternative theories to expected utility theory (Kahneman & Tversky, Citation1979; Tversky & Kahneman, Citation1992). It serves to explain a wide range of phenomena that are not explained within the traditional expected utility framework. It is used in different fields such as: economics, finance, marketing, and psychology (cf. e.g. Barberis, Huang, & Santos, Citation2001; Dalal, Citation1983; Pennings & Smidts, Citation2003; and references therein).
Prospect theory proposes an S-shaped utility function. A formal definition of this type of function is found in Neilson (Citation2002):
Definition 1
A continuous strictly non-decreasing function is called S-shaped if there is a point
such that the function is non-positive and convex to the left of
and non-negative and concave to the right of
. The point
, that separates losses from gains, is frequently called the reference point, or the status quo. (Throughout the paper, I set
.
Specifically, Kahneman and Tversky (Citation1979) propose the following power function(4)
(4) where
is the degree of loss aversion, and
and
are degrees of diminishing sensitivity.
al-Nowaihi, Bradley, and Dhami (Citation2008) prove that (4) with is a proper S-shaped function that accounts for preference homogeneity and loss aversion. Nonetheless, this utility function has a mathematical tractability limitation, which is that its first derivative does not exist at
(cf. e.g. Wakker, Citation2010). I shall consider this limitation in the main result.
Nevertheless, other different types of S-shaped utility functions have been proposed. For instance, De Giorgi and Hens (Citation2006) suggest using the following S-shaped function:(5)
(5) with parameters
and
.
Since we are interested in analyzing the marginal utility of an S- shaped utility function, then we can write:
(i) For Kahneman and Tversky (Citation1979)Footnote1(7)
(7) and (ii) for De Giorgi and Hens (Citation2006) as follows
(8)
(8) Naturally, the marginal utility function
is non-negative because it is generally assumed that the underlying utility function
is not a decreasing one. Furthermore,
in many situations is non-monotonic on the entire real line.
Nevertheless, other types of S-shaped utility functions have been little explored in the literature. For instance, Berhold (Citation1973) and LiCalzi (Citation2000) propose the use of cumulative distribution functions to represent S-shaped utility functions. In particular, Broll, Egozcue, Wong and Zitikis (Citation2010) and LiCalzi (Citation2000) use an S-shaped utility function of the form,(9)
(9) where
is the cumulative distribution function of a symmetric random variable. For example, setting
, where
is the standard normal distribution function, then
has an S-shaped form, with a reference point at the origin.
One of the innovative features of prospect theory is loss aversion. The basic idea of loss aversion is that losses loom larger than gains of the same quantity. It can be defined in different ways (cf., Köbberling & Wakker, Citation2005; Neilson, Citation2002). I present a brief summary of the most well known definitions of loss aversion.
Definition 2
Let be an S-shaped utility function with
. Then
exhibits loss aversion if it fulfills one of the following conditions:
(1) |
| ||||
(2) |
| ||||
(3) |
| ||||
(4) | If | ||||
(5) | If |
Some authors also define loss aversion as for all
, which is a particular case of the third condition in Definition 2. Hereafter, I shall consider utility functions that posses this last particular characterization of loss aversion, and I will also use those utility functions with loss aversion as defined in condition (5).
Nonetheless, evidence of the presence of loss aversion has received mixed empirical support (cf. e.g. Harinck, Van Dijk, Van Beest, & Mersmann, Citation2007; McGraw, Larsen, Kahneman, & Schkade, Citation2010; Rozin & Royzman, 2001, and the references therein).
Indeed, although the idea of loss aversion is appealing, recent studies have found evidence of opposite effects. For instance, Harinck et al. (Citation2007) and McGraw et al. (Citation2010) find evidence that for small outcomes, loss aversion is reversed, and individuals weigh more heavily gains than losses, which is referred as reverse loss aversion. In order to make the analysis as general as possible, I will also consider the case of reversed loss aversion (i.e. gains loom larger than losses). I shall call reverse loss aversion utility functions, those with the condition for all
. Note that for power utility functions as presented in Definition 2 condition (4), reversed loss aversion implies that
. For further features of prospect theory functionals, the interested reader is referred to Wakker (Citation2010).
3 Literature review
In this section, I present a brief review of some well-known covariance inequalities. I begin with the celebrated Chebyshev’s integral inequality, which can be stated in its integral (original) form as follows:
Theorem 1
Let ,
be two real functions from
and
. Then we have:
(1) | If | ||||
(2) | If one of the functions is increasing and the other is decreasing, then the inequality is reversed. |
It is common to see this inequality in its probabilistic notation. This can easily be done, assuming as a probability density function. Then, Chebyshev’s integral inequality in Equation (10) can be written as follows
Therefore, Theorem 1, can be expressed in its most usual probabilistic form as follows.
Theorem 2
Let be a continuous random variable defined on
, with well defined expectations. Consider two real functions
and
then:
(1) | If | ||||
(2) | If one function is non-decreasing and the other one is non-increasing, then we have |
We note that if the random variable is non-degenerate and both functions are strictly monotonic then the inequalities in Theorem 2 are strict.
The following Lemma plays an important role in proving the Chebyschev’s inequality.
Lemma 1
Let and
be two continuous real functions and
be a continuous random variable defined on
. Then
where
is such that
Remark 1
This result follows directly from applying the Second Mean Value Theorem for integrals (cf. e.g. Sahoo & Riedel, Citation1998), and using the definition of the covariance (see e.g. Gurland, Citation1967; Schmidt, Citation2014). Notice that Theorem 2 can be proved invoking Lemma 1. For instance, assume both functions are increasing, then (i) If then as
and
are increasing then
is non-negative. (ii) On the other hand, if
then
and
, which yields the same result.
As I have noted earlier, there is an important limitation of the Chebyshev’s integral inequality. It requires that both functions must be monotonic. This strong assumption might be violated on several occasions. Hence, studying the sign of (1) by relaxing the monotonicity assumption is not only a problem of pure mathematical interest, but it is also of interest in applied mathematics.
Some results, relaxing the monotonicity assumption, have already been proved. For example, Steffensen (Citation1925) proposes a non-monotonic version of Chebyshev integral inequality. However, one of the functions must hold a special condition. For future reference, since the proof is difficult to find in the literature, we present a simple proof expressed with probabilistic notation.Footnote2 Additionally, we extend Steffensen’s Theorem considering some other cases.
Theorem 3
Let and
, be differentiable real functions. Consider a random variable
with the density function
, the cumulative density function
and support on
Assume the expectations exist. If
(11)
(11) where
is the conditional expectation operator, then:
(1) | If | ||||
(2) | if |
Proof
We prove only the first case, the other cases can be proved with the same argument. Using the weighted average mean value theorem for integrals (Sahoo & Riedel, Citation1998), there is such that
The condition
for all
is equivalent to
where
is the indicator function, which is equal to
whenever
and equal to
otherwise. First, notice that
Now, we can express
(12)
(12) Integrating by parts in Equation (12), we obtain
Since
and
for all
we get
We note that if is increasing then inequality (11) holds. However, the opposite is not necessarily true. Nevertheless, the non-monotonic function must hold a special condition, which is expressed with a conditional expectation inequality.
Egozcue, Fuentes Garcıa, & Wong (Citation2009), Egozcue et al. (Citation2011) derive some new covariance inequalities relaxing the assumption of monotonicity, but their results work only for symmetric random variables. Actually, some of their results are a special case of Steffensen’s theorem, which we proceed to prove after presenting its statement.
Theorem 4
Let be a random variable symmetric about zero with support on
and with a density function
. Consider two continuous real functions
and
. Assume that
is an odd function with
for all
. We have
(1) | if | ||||
(2) | if |
Proof
The first case; the others can be proved similarly. Note that because is an odd function and
is symmetric, then
Let
then
Now, applying the Leibniz’s rule, we get
We therefore deduce that
for
and
for
. Thus, we have Steffensen’s theorem condition,
Hence, as
is increasing we apply Theorem 3.
This result relaxes the monotonicity assumption of one function, but it requires symmetry with the random variable. Naturally, as one assumption is relaxed it requires an additional assumption to get consistent results.
In the next theorem, Egozcue et al. (Citation2009) relax the monotonicity assumption of both random variables.
Theorem 5
Let be a random variable symmetric about zero. Consider two real functions
and
. Let
be an odd function of bounded variation with
for all
.We have that
(1) | if | ||||
(2) | if |
Broll et al. (Citation2010) extend this result considering S-shaped utility function. They show that the mean has an important role to determine the covariance sign for a particular type of S-shaped utility functions, as we can see in the following theorem.
Theorem 6
Let be symmetric around its mean
. If
is an S-shaped function, with
for all
Then, we have the following statements:
(1) | If | ||||
(2) | If |
Theorem (6) characterizes the sign of the covariance (2) for a non-monotonic marginal utility. However, it works only for a utility function that does not consider strict loss aversion, as it is defined in Definition (2). In the next section, I present a general result of this theorem that includes discussion for an S-shaped utility function with loss aversion and reversed loss aversion as well. I also extend this theorem considering reverse S-shaped utility functions.
4 Main results
In this section, I present the main results of this paper. We have seen that S-shaped utility functions have non-monotonic marginal utilities. We have also seen that for some S-shaped utility functions, (e.g. (4)), the marginal utility does not exist at the reference point
. Nevertheless, there other S-shaped utility functions with more mathematically tractable behavior such as (5) or (9). First, I shall state a general theorem where the marginal utility exists in all the real line. Second, I shall relax the assumption of existence of the marginal utility at the origin.
First, in the next result, I extend Broll et al. (Citation2010) findings considering general S-shaped and RS-shaped utility functions. The novelty of this result is that I shall consider loss aversion and reversed loss aversion as well.
Theorem 7
Let be a symmetric random variable about its mean
. Let
be a differentiable utility function.
(1) | If
| ||||||||||||||||
(2) | If
|
Proof
First, I prove case (1a). Define the random variable Therefore,
is symmetric about zero with
. Thus, we rewrite the covariance as follows
(13)
(13) There are two cases to consider: (i) Let
. Since
implies
and
is non-increasing on
, we have that
(14)
(14) (ii) Now, assume that
. Since
and
, we therefore have that
. Consequently, using the assumption of
for all
we have that
and thus
(15)
(15) We exploit the fact that the right-hand side of bound (15) is non-positive because
is non-increasing on
and
. Consequently,
and thus
(16)
(16) Therefore, together from (14) and (16), we conclude that
(17)
(17) Multiplying in both sides of (17) by
, taking expectations in both sides and using equality (13), we obtain that
This finishes the proof of part 1(a).
Now, the proof of part (2b). Starting with equality (13), we have, again, two cases:
(i) Assume , then (since
and
), we have
. And, since we are in the negative domain of
it is concave. Therefore, we conclude that
(ii) Now, assume that
. Since we have that
for all
then
. So that,
Notice that
, thus we are in the positive domain of
, implying that it is convex. Thus,
, therefore, we conclude that
At the end, we conclude that for all
and
we have that
Following the same steps as in the proof of part 1(a), we conclude that
This ends the proof of part (2b). The other cases could almost certainly be adapted in the same way.
The extension of Theorem 7 to other S-shaped such as (4) can be done considering certain types of symmetric random variables. For example, Theorem 7 could almost certainly be adapted to those random variables, , such as
almost surely. I present this extension in the next theorem.
Theorem 8
Let be a random variable symmetric about its mean
, and such that
almost surely. Suppose
is an S- shaped utility function, as defined in (4), then we have the following two statements:
(1) | If | ||||
(2) | If |
Proof
I only prove the first case; the other case can be proved in the same way. The proof mimics the proof of Theorem 7. First, with the notation , we rewrite the covariance
as the expectation
. Since
by assumption, we have also
by the symmetry of
. Consequently,
is equal to
, and thus
(18)
(18) I skip the rest of the proof, since it is similar to that of Theorem 7.
5 Applications
This section shows some applications of the main results of the paper. The range of applications of our findings is broad, but I restrict the analysis to two cases. First, I study the monotonicity condition of the mean-variance indifference curve for an S-shaped utility function and RS-shaped utility function. Second, I apply the findings to the hedging policies of an enterprise that behaves according to prospect theory.
5.1 Mean-variance indifference curves for S-shaped and RS-shaped utility functions
The expected utility approach and the mean-variance approach, which is known as criterion, are in general two different approaches for decision-making under uncertainty. The expected utility approach says that
is preferred to
if and only if
(19)
(19) where
is a concave utility function. On the other hand, the mean-variance approach (sometimes also called mean-variance rule) was introduced by Markowitz (Citation1952) and states that choice
is preferred over choice
if
with at least one strict inequality. Here,
and
denote the mean of
and
and
and
denote their respective standard deviations. The idea is that decision-makers use only the mean and variance to make decisions. This is a common tool used by practitioners in finance (Shefrin, Citation2008). However, it has strong theoretical limitations. For example, it does not satisfy the expected utility independence axiom (e.g. Hens & Rieger, Citation2010, p. 50).
Many scholars study when both approaches are equivalent. Tobin (Citation1958) shows that the two approaches are compatible under normally distributed assets or quadratic utility functions. Moreover, under the normal distribution assumption, the mean-variance rule also coincides with the expected utility approach (Hanoch and Levy, Citation1969). Sinn (Citation1983) and Meyer (Citation1987) show the equivalence of these approaches when the distributions differ only by a location and scale parameters. That is, suppose that has a distribution that belongs to a class
, then
where,
and
also belongs to that class of distribution
In other words, if the distribution of
is
then the distribution of
is equal to
. Some distributions that satisfy the location scale condition are, among others: the elliptical distributions; the normal distribution; the uniform distribution; the Cauchy distribution; and the Student’s t distribution.
Sinn (Citation1983) and Meyer (Citation1987) derive several properties of the indifference curve in a space, generated by a general risk averse von Neumann-Morgenstern utility function. In particular, these studies prove that these indifference curves, represented as a function
, are increasing and convex. These conditions are useful when the indifference curve is maximized over convex feasible sets. They explained, among other things, issues such as the existence of the CAPM equilibrium, as elucidated by Ormiston and Schlee (Citation2001).
It is important to study the monotonicity of function . An increasing function means that the investor is willing to take more risk in exchange for more expected return. This is a crucial assumption of portfolio theory, since larger returns are associated with higher risk. Therefore, as an application of the main results, I will study whether the monotonicity property still holds for S-shaped and RS-shaped utility functions.
To keep the analysis as simple as possible, I do not consider transformations of the distribution function as prospect theory suggests (Kahneman & Tversky, Citation1979; Tversky & Kahneman, Citation1992). Hereafter, I assume that the random return belongs to the location-scale family
, where
is a random variable with mean
and variance
, and whose distribution function
does not depend on
and
. Hence, the expected utility
defines a two-argument function
(20)
(20) Various properties of
, its partial derivatives
and especially of
have been extensively investigated in the literature (see, for example, Meyer, Citation1987; Sinn, Citation1983).
The quantity has played a particularly prominent role. For instance, it can be viewed as the derivative with respect to the standard deviation
of the indifference function
, which, for a given constant
, can be viewed as the curve
drawn in the
-plane.
Hence, if is positive, then the indifference function
is increasing, whereas if
is negative, then the indifference function is decreasing. Assuming that the utility function
is differentiable and some integrability conditions are satisfied, we have the equations
(21)
(21) where
. Since
, we therefore have
(22)
(22) We may view
as the expected marginal utility or, in other words, the slope of the expected utility
with respect to
. Likewise, we may view
as the expected marginal utility
with respect to
. Finally, we may view
as the slope of the indifference function
.
This indifference curve and its various properties (e.g. monotonicity, convexity, concavity, and so forth) have received considerable attention in the literature. As we have noted above, some of the properties follow from the corresponding ones of the indifference function . In particular, the following general property is well known (see, for example, Eichner, Citation2008; Eichner & Wagener, Citation2009; Meyer, Citation1987; and references therein).
Theorem 9
If the distribution of with mean
and variance
belongs to a location and scale family, and the twice differentiable utility function
is increasing on its domain of definition, then we have the following two statements:
(1) | If the utility function | ||||
(2) | If the utility function |
It is now natural to extend formulas (21)–(23) to the case of general marginal utility functions and random variables
. As before, I use the notation
and
.
Determining the sign of (23) is obviously related to the sign of . When the marginal utility is monotonic, then we know that
for every non-decreasing
and
for every non-increasing
. However, the marginal utility may be non-monotonic, as noted earlier. To cover such functions, I establish the following theorem that studies the monotonicity of the indifference curve generated by S -shaped utility functions.
Theorem 10
Suppose the utility is an S-shaped function. Let
be a random variable where
is a symmetric random variable with zero mean and unit variance. Assume that the location-scale condition holds.
(1) | If | ||||
(2) | If |
Proof
I only prove Part (1) of the theorem by considering the case We have seen that the slope of the indifference function
is determined by the sign of
Since
is symmetric about zero, then
is also symmetric about
. Therefore, invoking the first part of Theorem 7, we deduce that
and thus
which implies that the assertion in Part (1) of Theorem 10 holds. Part (2) can be proved in the same way.
Next, I study the monotonicity property of the indifference curve for RS-shaped utility functions.
Theorem 11
Consider the utility function as defined in (3), in which case, the utility is an RS-shaped function. Let be a random variable where
is a symmetric random variable with zero mean and unit variance.
(1) | If | ||||
(2) | If |
Proof
The proof is analogous to the one in Theorem 10, but now invoking the results of the second part of Theorem 7.
5.2 Hedging policies within prospect theory
In this application, I follow the Broll and Wahl (Citation2006) hedging model of a firm with one-period planning horizon.
The model setup is as follows. The enterprise that has risky assets with random returns, (future spot price) The assets are financed partially with external funds (deposits), denoted by
, which pays a certain return (price)
The enterprise also finances its assets with a fixed equity
. Therefore, we can write the firm’s balance sheet constraint as follows,
(23)
(23) There are operational costs that depend on the deposits level. We represent these costs with a function
which we assume to be increasing and convex. In part of the uncertainty, the risky assets,
, can be hedged in the forward market at a certain price
. Let
denote the amount of the hedged assets that is determined at the beginning of the period. When
is positive means that the firm is selling assets in the futures market. On the other hand, if
is negative, it means that firm is purchasing assets in the futures market. It is said that speculation is involved if
; otherwise, the assets are hedged without speculation. For instance,
it means that the firm is purchasing assets in the forward market, while
means that the firm selling an amount greater in the futures market than its current assets.
Since is known, in this scenario, the next period enterprise’s profit function is given by
(24)
(24) The firm’s profit is uncertain and its mean is given by
(25)
(25) As we shall see, the value of the mean, for the reasons studied in the previous section, has an important role in determining the optimal hedging decision.
Therefore, the firm manager’s problem is to find the optimal hedging that maximizes the expected utility of profits. However, instead of considering a traditional Bernoulli utility function, the firm uses an S-shaped utility function as defined in Definition 1.
Thus, the firm wants to maximize its expected utility of profit(26)
(26) In other words, we want to find the
that maximizes the expected utility of profits. I denote by
the solution of (27). Since
is an S-shaped function, then there is no guarantee that
will be concave respect to
. Therefore, I restrict the analysis to those cases where the first-order condition holds and there is a global solution of (27).
Proposition 1
If the first-order condition of (27) holds then we have(27)
(27)
Proof
Taking the first-order condition of (27), evaluated at , we have
The latter equation can be rewritten as follows:
Now, using the covariance function, we have
(28)
(28) After subtracting (25) from (26), we get
which implies that
(29)
(29) Substituting (30) in the covariance term of (25), we have
Therefore, from Equation (29), we have
(30)
(30)
Finding is generally a complex task. Nevertheless, Equation (31) has important derivations. Since
is strictly positive, the sign of the covariance
determines the sign of the product
. When
is more complexly shaped than being concave, then determining the sign of the covariance
is a challenging task.
The relation between the expected spot price and the futures price will also determine the sign of . When there are more hedgers taking short positions in the futures market than those that are going long then, to reach a balance, speculators must enter the market taking long positions. The speculators will do so, only if
(a condition named normal backwardation). Conversely, if there are more hedgers taking long positions than those that are short, speculators will enter the market if
(a condition named contango).
Notice that using the spot-futures parity relationship (which states that the ratio of return on perfectly hedged stocks equals the risk-free interest rate), we can write, where
is the risk free interest rate,
the required rate of return and
is the number of periods (Bodie, Kane, & Marcus, Citation1996 p. 708). Thus,
will be less than
whenever
(i.e. whenever the asset has a positive beta). When the expected price equals the forward price, then the price is unbiased, which is the case when
. Finally,
whenever
(i.e. whenever the asset has a negative beta).
Next, I present an application of Theorem 7 that studies the sign of the covariance in (28).
Proposition 2
Let the distribution of be symmetric around its mean
. Let
be an S-shaped utility function, with loss aversion defined as
for all
. If
then
Proof
The proof follows directly by invoking Theorem 7.
We see that plays a decisive role in determining the sign of the covariance
When
, note that we have the following expressions for the mean:
Using Proposition 2, we obtain the following corollary, which guides the firm in deciding whether to speculate or not. More precisely, it will tell us whether
is smaller or greater than
, depending on whether the expected price
is smaller or greater than the forward rate
.
Corollary 1
Let the distribution of be symmetric around its mean
. Let
be an
-shaped utility function such that
for all
. Assume that
is a solution of (31) such that
, then we have the following statements:
(1) | If | ||||
(2) | If |
Proof
Now, I prove the first part. The third part can be proved similarly. From the first-order condition of (27) we have. Since
using Proposition 2 then
Therefore, the sign of
is the opposite to the sign of
Therefore, since
and
we have
This result has the following intuition.Footnote3 In the first case, if the forward price is less than the expected spot price, then the firm could hedge an amount less than its current assets. However, if the gap between the forward price and the expected prices is large enough, then the firm could purchase assets in the futures market. The firm will expect to sell at a greater price in the future. In the second case, if the forward price is greater than the expected price, then the firm will speculate selling an amount greater than its assets, expecting to purchase the additional assets in the future at a lower price.
These are well known results for decision makers with strict risk aversion (Benninga, Eldor, & Zilcha, Citation1983; Feder, Just, & Schmitz, Citation1980; Holthausen, Citation1979; Meyer & Robison, Citation1988). At the end, under these conditions, the enterprise hedging policies with an S-shaped utility are similar to those if it has an increasing and concave utility function.
6 Concluding remarks
In this paper, I establish new covariance inequalities with non-monotone functions. In particular, I derive new results to study the sign of , when the marginal utility is non-monotonic. This is the case when the utility function is according to either utility, as presented by Markowitz, or as in prospect theory. I show that the sign depends on the mean of the random variable and on the degree of loss aversion.
Two applications illustrate the main results of this paper. First, I study the monotonicity properties of the indifference curves on the -plane for
-shaped and
-shaped utility functions. My results show that the indifference curve of S-shaped utility is increasing when there is loss aversion and
Similar results are derived considering reverse loss aversion and using RS-shaped utility functions as well. Finally, I study hedging policies of a firm that uses a utility function as postulated by prospect theory. I examine the behavior of a firm whose utility function varies with gains and losses in firm’s profits. Even though the analysis with prospect theory is more complex than one that assumes risk aversion, I demonstrate that similar behavior hold for symmetric random variables.
This work can be extended in several directions. For instance, it would be interesting to generalize Theorems 7 and 8 considering skewed distributions and especially for skewed-normal distribution (Azzalini, Citation1985). This remains a task for future studies.
Acknowledgements
I am grateful to two anonymous reviewers for numerous insightful suggestions and critical remarks that have guided my work on revising the paper. Comments by Luis Fuentes García, Hugh Schwartz and Ricardas Zitikis are much appreciated.
Additional information
Funding
Notes on contributors
Martín Egozcue
Professor Egozcue is a Certified Public Accountant and Bachelor of Economics from Facultad de Ciencias Económicas, UdelaR. He also holds a Master and a Doctoral degree in Economics from Facultad de Ciencias Sociales, UdelaR. He teaches Behavioral Economics at Universidad de la República del Uruguay and Finance at Catholic University Damaso Antonio Larrañaga in Uruguay. He also works as a Financial Consultant for several firms in the construction, educational, financial and pharmaceutical industry. His primary research is decision theory, but he is also interested in mathematical inequalities and its applications to social science. In several publications, the author has derived new extensions of Gruss and Chebyschev covariance inequalities. This article continues in the same direction, presenting new covariance inequalities and its potential applications.
Notes
Martín Egozcue, Facultad de Ciencias Sociales, Department of Economics, Universidad de la República, Montevideo 11200, Uruguay.
1 Throughout this work, I use to denote the first derivative of
(when it exists), and the Radon-Nykodym derivative in the absolutely continuous case (when the derivative may not exist). For example, given the marginal utility
as in (4), the utility function
is given by the formula
(6)
(6) Notice, that
in (6) coincides with
in (4). This is in line with the most frequently used in the statistical notion of absolutely continuous distribution functions. For example, the uniform on
density function
is related to the uniform distribution
by the equation
, but
is not differentiable at the points
and
2 Part of this proof was provided to me by Luis Fuentes García.
3 I have left the case as a task for future research, because it is more involved. It is necessary to prove that
implies
, where
and
are real numbers.
References
- Alghalith, M. (2010). The limitations of prospect theory and the expected utility theory: A new theory. Atlantic Economic Journal, 38, 243–244.
- al-Nowaihi A., Bradley I., & Dhami S. (2008). A note on the utility function under prospect theory. Economics Letters, 99, 337-339.
- Azzalini, A. (1985). A class of distributions which includes the normal ones. Scandinavian Journal of Statistics, 12, 171–178.
- Barberis, N., Huang, M., & Santos, T. (2001). Prospect theory and asset prices. Quarterly Journal of Economics, 116(1), 1–53.
- Batra, R. N., & Russell, W. R. (1974). Gains from trade under uncertainty. American Economic Review, 64, 1040–1048.
- Benninga, S., Eldor, R., & Zilcha, I. (1983). Optimal hedging in the futures market under price uncertainty. Economics Letters, 13, 141–145.
- Berhold, M. H. (1973). The use of distribution functions to represent utility functions. Management Science, 19, 825–829.
- Bodie, Z., Kane, A., & Marcus, A. J. (1996). Investments (3rd ed.). Boston, MA: Irwin.
- Broll, U., Egozcue, M., Wong, W. K., & Zitikis, R. (2010). Prospect theory, indifference curves, and hedging risks. Applied Mathematics Research Express, 2010, 142–153.
- Broll, U., & Wahl, J. E. (2006). Banking and the advantage of hedging. Annals of Financial Economics, 1, 24–41.
- Dalal, J. A. (1983). On the use of a covariance function in a portfolio model. Journal of Financial and Quantitative Analysis, 18, 223–227.
- De Giorgi, E., & Hens, T. (2006). Making prospect theory fit for finance. Financial Markets and Portfolio Management, 20, 339–360.
- Egozcue, M., Fuentes Garcıa, L., & Wong, W. K. (2009). On some covariance inequalities for monotonic and non-monotonic functions. Journal of Inequalities in Pure and Applied Mathematics, 10, 1–7.
- Egozcue, M., Fuentes García, L., Wong, W. K., & Zitikis, R. (2011). Do investors like to diversify? A study of Markowitz preferences. European Journal of Operational Research, 215, 188–193.
- Eichner, T. (2008). Mean variance vulnerability. Management Science, 54, 586–593.
- Eichner, T., & Wagener, A. (2009). Multiple risks and mean-variance preferences. Operations Research, 57, 1142–1154.
- Feder, G., Just, R. E., & Schmitz, A. (1980). Futures markets and the theory of the firm under price uncertainty. Quarterly Journal of Economics, 94, 317–328.
- Friedman, M., & Savage, J. L. (1948). The utility analysis of choices involving risk. Journal of Political Economy, 56, 279–304.
- Gillen, B. & Markowitz, H. M. (2009). A taxonomy of utility functions. In J. R. Aronson, H. L. Parmet & R. J. Thornton (Eds.), Variations in economic analysis: Essays in honor of Eli Schwartz. New York: Springer.
- Gurland, J. (1967). The teacher’s corner: An inequality satisfied by the expectation of the reciprocal of a random variable. American Statistician, 21, 24–25.
- Hanoch, G., & Levy, H. (1969). The efficiency analysis of choices involving risk. The Review of Economic Studies, 36, 335–346.
- Harinck, F., Van Dijk, E., Van Beest, I., & Mersmann, P. (2007). When gains loom larger than losses reversed loss aversion for small amounts of money. Psychological Science, 18, 1099–1105.
- Hens, T. & Rieger, M. O. (2010). Financial economics: A concise introduction to classical and behavioral finance. Berlin: Springer.
- Hershey, J. C., & Schoemaker, P. J. (1980). Risk taking and problem context in the domain of losses: An expected utility analysis. Journal of Risk and Insurance, 46, 111–132.
- Holthausen, D. M. (1979). Hedging and the competitive firm under price uncertainty hedging and the competitivefirm under price uncertainty. American Economic Review, 69, 989–995.
- Kahneman, D., & Tversky, A. (1979). Prospect theory of decisions under risk. Econometrica, 47, 263–291.
- Köbberling, V., & Wakker, P. P. (2005). An index of loss aversion. Journal of Economic Theory, 122, 119–131.
- Lehmann, E. L. (1966). Some concepts of dependence. Annals of Mathematical Statistics, 37, 1137–1153.
- Levy, H. (2006). Stochastic dominance: Investment decision making under uncertainty (2nd ed.). Berlin: Springer-Verlag.
- LiCalzi, M. (2000). Upper and lower bounds for expected utility. Economic Theory, 16, 489–502.
- Loubergé, H., & Outreville, J. F. (2001). Risk taking in the domain of losses: Experiments in several countries. Journal of Risk Research, 4, 227–236.
- Markowitz, H. (1952). The utility of wealth. Journal of Political Economy, 60, 151–156.
- McEntire, P. L. (1984). Portfolio theory for independent assets. Management Science, 30, 952–963.
- McGraw, A. P., Larsen, J. T., Kahneman, D., & Schkade, D. (2010). Comparing gains and losses. Psychological Science, 21, 1438–1445.
- Meyer, J. (1987). Two-moment decision models and expected utility maximization. American Economic Review, 77, 421–430.
- Meyer, J., & Robison, L. J. (1988). Hedging under output price randomness. American Journal of Agricultural Economics, 70, 268–272.
- Mitrinovic, D. S., & Vasic, P. (1970). Analytic inequalities. Berlin: Springer-Verlag.
- Mossin, J. (1968). Aspects of rational insurance purchasing. Journal of Political Economy, 76, 553–568.
- Neilson, W. S. (2002). Comparative risk sensitivity with reference-dependent preferences. Journal of Risk and Uncertainty, 24, 131–142.
- Ormiston, M. B., & Schlee, E. E. (2001). Mean-variance preferences and investor behaviour. Economic Journal, 111, 849–861.
- Pennings, J. M., & Smidts, A. (2003). The shape of utility functions and organizational behavior. Management Science, 49, 1251–1263.
- Post, T., & Levy, H. (2005). Does risk seeking drive stock prices? A stochastic dominance analysis of aggregate investor preferences and beliefs. Review of Financial Studies, 18, 925–953.
- Reilly, R. J. (1986). The Markowitz utility function and some experimental evidence for small speculative risks. Journal of Risk and Insurance, 53, 724–733.
- Rozin, P., & Royzman, E.B. (2001). Negativity bias, negativity dominance, and contagion. Personality and Social Psychology Review, 5, 296–320.
- Sahoo, P. & Riedel, T. (1998). Mean value theorems and functional equations. Singapore: World Scientific Publishing Company.
- Sandmo, A. (1971). On the theory of the competitive firm under price uncertainty. American Economic Review, 61, 65–73.
- Schmidt, K. D. (2014). On inequalities for moments and the covariance of monotone functions. Insurance: Mathematics and Economics, 55, 91–95.
- Shefrin, H. (2008). A behavioral approach to asset pricing (2nd ed.). Burlington, MA: Elsevier.
- Simonovits, A. (1995). Three economic applications of Chebyshev’s Algebraic Inequality. Mathematical Social Sciences, 30, 207–220.
- Sinn, H.-W. (1983). Economic decisions under uncertainty. Amsterdam: North-Holland.
- Steffensen, J. F. (1925). On a generalization of certain inequalities by Tchebychef and Jensen. Scandinavian Actuarial Journal, (3-4), 137–147.
- Tobin, J. (1958). Liquidity preference as behavior towards risk. Review of Economic Studies, 25, 65–86.
- Tversky, A., & Kahneman, D. (1992). Advances in prospect theory: cumulative representation of uncertainty. Journal of Risk and Uncertainty, 5, 297–323.
- Wagener, A. (2006). Chebyshev’s algebraic inequality and comparative statics under uncertainty. Mathematical Social Sciences, 52, 217–221.
- Wakker, P. P. (2010). Prospect theory: For risk and ambiguity. Cambridge: Cambridge University Press.