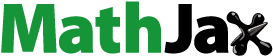
Abstract
This paper’s primary alternative hypothesis is Ha: profitable exchange-traded horserace betting fund with deterministic payoff exists for acceptable institutional portfolio return—risk. The primary hypothesis challenges the semi-strong efficient market hypothesis applied to horse race wagering. An optimal deterministic betting model (DBM) is derived from the existing stochastic model fundamentals, mathematical pooling principles, and new theorem. The exchange-traded betting fund (ETBF) is derived from force of interest first principles. An ETBF driven by DBM processes conjointly defines the research’s betting strategy. Alpha is excess return above financial benchmark, and invokes betting strategy alpha that is composed of model alpha and fund alpha. The results and analysis from statistical testing of a global stratified data sample of three hundred galloper horse races accepted at the ninety-five percent confidence-level positive betting strategy alpha, to endorse an exchange-traded horse race betting fund with deterministic payoff into financial market.
Public Interest Statement
An exchange-traded horserace betting fund with deterministic payoff betting strategy is developed from new and existing mathematical principles and theory. The results from sample testing statistically support a profitable betting strategy to challenge the existing semi-strong efficient market hypothesis (EMH) toward horse racing.
1. Preliminary
The Bank for International Settlements (Citation2007) examined institutional investors, global savings, and asset allocation, and remarked that one important investment decision of insurance companies and pension funds is the selection of an optimal asset mix that best reflects their risk—reward trade-offs. There is little corporate consideration presently toward horserace betting as a mainstream or alternative asset for portfolio input, to support the efficient market hypothesis (EMH) toward horserace betting (Bank for International Settlements, Citation2007; Roca, Wong, and Tularam (Citation2010); Tularam, Roca, & Wong, Citation2010). One conclusion drawn from this hypothesis is that traders are unable to achieve consistently superior returns from either technical parametric or fundamental nonparametric betting strategies. Correspondingly, there is extensive research on optimal stochastic horserace betting systems that forecast horserace outcomes based on both “technical” and “fundamental” analysis techniques to challenge the semi-strong EMH applied to horseracing (Edelman, Citation2007). The concept of an efficient betting market incorporates forecasting future asset prices and future expected return remarked Snyder (Citation1978). Essentially, the horserace bet as suggested by Koning and Van Velzen (Citation2009) is a binary option asset that generates a defined return from the betting market; as argument against the EMH. The questioning of the credibility of the EMH suggests that a profitable deterministic horserace betting strategy may be achievable. The profoundness of a deterministic model is that every set of variable states is uniquely determined by statistics in the model and by sets of previous states of these variables wrote Yang (Citation2008).
2. Rank-order permutation
The foundation for the existing literature written and research conducted on horserace betting models is largely the rank-order permutation of stochastic processes (Critchlow, Fligner, & Verducci, Citation1991). The rank for the outcome of an event expressed by a rater is the result of ranking of a random variable from an underlying parametric or nonparametric distribution (D’Elia, Citation2003). The number of rank-order arrangements in an n racer field is n!, and the subsequent number of arrangements in an n racer field with i associations is (n − i)!, where i denotes the number of associations. The order of finish for a field size of n racers is represented by the general permutation
first to jth ranked racers;
for the n racer field. Permutation group
represents the set of complete and partial rank-order permutations for the n racer field, and
the parametric or nonparametric distribution on permutation
. Plackett’s (Citation1975) first-order permutation is represented by
, whereby
represents the first placed racer and
is the last placed racer from the field.
illustrates Plackett’s second-order permutation for the n racer field (
paired racers). The stochastic technical horserace betting models of parametric distribution revised in this paper utilize the bettor win or place real odds vector,
: first j racer’s odds event i;
, to calculate permutation probability.
3. Permutation probability of parametric distribution
In this paper, and
represent independent, non-identically distributed discrete and continuous random variables, respectively.
represents the rank order for racer i (and associations) with probability density
; and
represents the time taken for the ith rank racer (and associations), with probability distribution
Accordingly,
designate the first ranked racer and time taken by first ranked racer; and subsequently,
represent the first and second ranked racer pair, and the time taken by the winning racer pair, respectively. Stochastic technical model theory has developed from the L-Decomposable and Plackett’s logistic models of distribution
, to models (and their derivatives) based upon the normal, gamma and exponential parametric distributions
. Random variables
are statistically independent when the joint probability density function equals the marginal densities product; i.e.
.
The permutation probability calculated from the conditional product of the choice probabilities is outlined in Equation 3.1;
: probability kth to lth ranked racers from remaining “n − k + 1” racers,
(3.1)
(3.1)
The ranking processes of the L-Decomposable models of Harville (Citation1973), Luce (Citation1959), and Stern (Citation1990) all determine the permutation probability from the individual racer win (or place) odds of r-1 objects as shown by Equation 3.2;
is the set remaining at stage i, with
(3.2)
(3.2)
Plackett’s (Citation1975) logistic model expresses the n!−1 independent permutation probabilities from n objects in terms of a finite set of n!−1 parameters of hierarchical structure . All the parameters of the logistic model are logarithms of probability win ratios, except K (Plackett, Citation1975), and the hierarchical structure is outlined in Equation 3.3.
(3.3)
(3.3)
When equal zero, Plackett’s first-order model is the L-decomposable model; and the permutation probability from a second-order logistic model for n racer field is illustrated in Equation 3.4 (Dansie, Citation1983; Plackett, Citation1975).
(3.4)
(3.4)
The parametric distributions of the normal random variable , the gamma random variable
, and the exponential random variable
have been proposed for horserace betting modeling. The normal rank-order model is a class of rank model that is a function of a single independent variable of parametric distribution
with joint pdf
. The permutation probability from the normal rank model is represented by the multivariate integral,
(3.5)
(3.5)
The probability of racer i first for (expected win time) is expressed in Equation 3.6;
(3.6)
(3.6)
Henery (Citation1983) derived the normal rank approximate model from first-degree Taylor expansion to calculate the permutation probability shown in Equation 3.7 which has been modified for associations.(3.7)
(3.7)
The win, place, and trifecta probability approximations are illustrated by the following forms(3.8)
(3.8)
The gamma rank-order model class is a function of bivariate independent variables with gamma distribution and joint pdf
The gamma rank probability permutation by Stern (Citation1990) is in Equation 3.9 which has been modified for associations;
(3.9)
(3.9)
and the racer probability to win by Henery (Citation1983) is expressed in Equation 3.10;(3.10)
(3.10)
The gamma density function is represented by(3.11)
(3.11)
from which a first-degree Taylor expansion approximation for the model in Equation 3.12 is derived by Henery (Citation1983) and modified to allow for associations accordingly:(3.12)
(3.12)
According to Henery (Citation1983) a gamma rank probability approximation for the kth placed racer is expressed below in Equation 3.13;(3.13)
(3.13)
The exponential model with density function denotes the gamma rank-order model with shape parameter r = 1. The exponential model is equivalent to the logistic model (Dansie, Citation1983; Stern, Citation1990); and the derivation of Plackett’s ordered logistic models from the gamma rank-order model (r = 1) using first principles is detailed in Equation 3.14;
(3.14)
(3.14)
A model developed from the both the logistic first-order and gamma models is the discount model; the discount model includes a discount factor to discount the anticipated diminished performance by a racer with a decrease in its placing suggests Lo, Bacon-Shone, and Busche (Citation1995). The log odds ratio assumption that racer i beats racer j for kth place being a discounted function of racer i defeating racer j for the win;
(Lo et al., Citation1995). Both a trifecta probability approximation and permutation probability approximation (that allows for associations) using the discount model technique is illustrated in Equation 3.15.
(3.15)
(3.15)
The discount model is a function of the win probabilities of the n racers in the event, unlike the L-Decomposable model which is a function of the win probabilities of r−1 racers (r ≤ n). These stochastic technical rank-order models use permutation conditional probability to determine expected outcomes for typical horserace betting products, such as win , exacta and quinella
, trifecta
, and first four events.
These permutation probabilities and the odds real vector become arguments to optimize the expected payoff function for an event’s betting products, and to determine the optimal bet vector
: bet on racers “k” to “l” event “i”
.
One approach to determine the optimal win and place bets on r racers (r ≤ n) for a race is the extension of the Kelly criterion (Edelman, Citation2007) of maximizing the expected logarithmic return for the race, as a function of the market bettor odds and probability forecasts for win, exacta, quinella, trifecta, and additional permutation products as outlined in Equation 3.16.(3.16)
(3.16)
When r = n, wagering on the set of partial or complete permutations is analogous to optimal field betting over the entire racer field.
4. Permutation set pooling odds payoff properties and theorem over 

The zero return-to-nil risk pooling property of the gross bettor odds applies to the partial and complete set of rank-order permutations over as illustrated by Equation 4.1 accordingly;
(4.1)
(4.1)
Equation 4.2 extends the pooling property over , and “arbitrage” is achievable from field betting of permutation wager products such as win
, quinella
and first four bet
, with trading the market bettor odds.
subject to: (4.2)
(4.2)
To establish optimal solution from the DBM of Equation 4.2, the multiple system optimization (MSO) theorem over (Hopf & Tularam, Citation2014) is outlined in Equation 4.3, and the proof is provided in Appendix A1.
Theorem 4.1
Multiple system optimization (MSO) over an “n” finite series of complex systems generates a constant real component over each consecutive system.
“n” multiple system complex function,
(4.3)
(4.3)
From the argument of MSO, the optimal solution for field betting on an individual horserace is a constant net payoff across the entire field; and with wagering on multiple, consecutive races, the DBM attempts to lock in arbitrage and a constant optimal payoff over the race series. The working of the DBM is demonstrated in Appendix A2.
Assume the unit investment period for the fund to be the day’s racing, and denotes the generated racer force of interest; i.e. nominal rate of return per annum convertible daily. During the day’s racing, an ETBF may invest on a single race, or invest over consecutive races. In the case of investing on a race series, the value of the fund at day’s end is provided in Equation 4.4 as follows;
(4.4)
(4.4)
Subsequently, the value of the fund at interval end, after the ETBF makes investment over a series of races over consecutive days is calculated by Equation 4.5.(4.5)
(4.5)
Equation 4.6 converts from the force of interest to the required nominal rate of return per annum convertible pthly;(4.6)
(4.6)
5. Pooling betting strategy
Alpha return in industry is defined as the excess investment return over a benchmark (King, Citation2007). Betting strategy alpha is invoked and assumed to be composed of model alpha and ETF alpha. An ETF may be trading at a premium to its benchmark even though the two financial models share identical model alpha (State Street Global Advisors, Citationn.d.). denotes betting strategy alpha to represent the excess return (per race) above the financial benchmark, from exchange traded betting fund with deterministic payoff betting strategy;
= PayoffETBF|DBM − PayoffBenchmark(excess return above benchmark).
The primary hypothesis statement tested at α = 5% is > 0, an exchange traded betting fund with deterministic payoff as betting strategy can achieve abnormal returns above financial benchmarks. The sample mean betting strategy alpha is assumed to be normally distributed, and
represents sample mean betting strategy payoff excess (per race) from the financial benchmark payoff.
The distribution for is derived from the sum of the two assumed normally distributed independent random variables
and
.
and
denote sample means model alpha and fund alpha respectively. Essentially, nested within the primary hypothesis are two sub-hypotheses to be tested, H1a:
> 0 and H2a:
> 0;
represents sample mean DBM payoff excess (per race) from an existing stochastic betting model benchmark, and
represents the sample mean ETBF payoff excess (per race) from the ETF SPDR S&P/ASX 200 fund benchmark.
6. Sample betting strategy alpha hypothesis testing and results
A stratified sample of three-hundred consecutive galloper races, , conveniently commencing from the start of the calendar year on 01 January 2012, from global racetracks of Australasia (Australia (173) and New Zealand (45)), Asia (Singapore (10) and Hong Kong (10)), South Africa (20) and the United Kingdom (42), provides the data for testing the performance of the DBM and ETBF race payoffs, against their respective benchmarks. The online totalizer market prices and race information were taken from the market-makers http://www.sportsbet.com.au/results/horse_racing (day one), and https://tatts.com/tattsbet (days two to six), with exposure predominantly in the Australasian region. Appropriately, horseraces from the Australasian region contributed seventy-three percent of test sample; and the Asian, African, and United Kingdom regions accounted for the remaining twenty-seven percent of the sample. A stratified random sampling technique is employed within these regions (strata) on the assumption that the random sample statistics generated will be a true reflection for the population parameters and is not subject to sampling error. A simple random sampling technique to select a sample size of fifty races from these regions was conducted daily for the six-day interval. The daily analysis of a randomly selected fifty races over six consecutive days simulates the continuous trading on global betting markets from the southern to the northern hemisphere; a premise for financial markets trading comparison.
Initially, regional daily and weekly average payoff (%) per race and accumulative payoff statistics, for “field win wagering”, are calculated using the DBM and SBM benchmark techniques for the sampling period from 01 January 2012 to 06 January 2012 (Hopf, Citation2014). The workings of the DBM and SBM techniques are illustrated in Appendix A2. The regional weekly average payoff (%) per race statistics using the DBM and SBM
techniques are provided in Table . The cross tabulation of Table summarizes the regional daily and weekly accumulative payoffs reported from the DBM technique for sample. Paired payoff results are reported according to two approaches; (a) nil trade strategy where maximum exposure from an individual racer losing is 100%; and (b) trade strategy where the maximum risk of loss from any individual racer is capped at a maximum of 10%.
Table 1. Model regional mean alpha (per race)—daily and weekly averages
Table 2. DBM accumulative payoff cross tabulation
UK race series results for day one report $1 investment from ETBF accumulated to (a) $1.44 (nil trade) and (b) $1.83 (trade strategy). UK race series accumulative results for the sampling period report $1 investment from ETBF diminished to (a) $1.22 × 10−4 (19.3% compounding loss per race from consecutive betting on the 42 race series). However, $1 investment from ETBF accumulated to (b) $4.81 (3.8% compounding profit per race from trading market odds). Similarly, day one’s race series results display $1 investment from ETBF diminished to (a) (22.05% compounding loss per race from consecutive betting on the 50 race series); in comparison, $1 investment from ETBF accumulated to (b) $18.65 (6.03% compounding profit per race from trading market odds using DBM). The sample statistics for DBM and no trade intervention disclosed a constant accumulative investment loss of
from betting consecutively on three-hundred races, and average daily investment loss on each race of
calculated from the five regions and six-day time period. Essentially, results from trade intervention with global market-makers to maximize loss from a race to ten percent of the investment, reported a credible sample compounding race return of
; $1 invested by ETBF accumulated to a prodigious $8180 from consecutive betting on the three-hundred race sample over the six-day period using the DBM method with hedging; to legitimize the hypotheses testings conducted.
Sample payoff results from the DBM technique are benchmarked against payoff results recorded using the normal rank approximate method. Although dated, both Ali (Citation1998) and Lo et al. (Citation1995) tests showed that the normal rank-order model forecasted horserace rank probabilities more accurately than the gamma rank or L-decomposable models. Uniform unit bets on the field for the normal rank approximate model resulted in the net expected win return per $1 unit bet from a winning racer equaling the negative of the tote track take (t); and the net expected field win payoff is
The sample statistics using the SBM technique in Table recorded a constant, compounding race loss of from consecutive wagering on the three-hundred race sample that equates to an average daily regional loss per race from total sample of
. Both the daily and regional race series recorded consecutive race losses of
.
Table 3. SBM accumulative payoff cross tabulation
The regional weekly average payoff (%) per race and accumulative payoff per race statistics provide the data to determine the alpha central tendency and dispersion measures. Table displays the daily and weekly mean model alpha (per race) measures, and
, for the regions; and Table displays the daily and average weekly fund alpha race returns,
and
. The daily returns for the SPDR S&P/ASX 200 fund benchmark are tabled in Appendix A3. The time interval between successive online races is forty minutes, and appropriately the daily returns reported during sampling period for the SPDR S&P/ASX 200 fund, which trades daily for six hours on the ASX, are converted into equivalent compounding return per forty minutes, to match the ETBF compounding race payoff.
Table 4. Fund alpha (per race)—daily and week average
Collectively, these betting strategy sample statistics (using trade hedging) are provided in Table for testing of the secondary sub-hypotheses and primary hypothesis statements. From Table credible Sharpe and coefficient of variation measures were recorded from sample of = 3.09 and
= 0.32, respectively, for asset benchmark comparison.
Table 5. Betting strategy sample statistics
The secondary hypotheses and primary hypothesis tests and outcomes conducted at ninety-five percent level of confidence are detailed accordingly;
H10: = 0,
H1a: > 0,
∴ Rejection of , and acceptance of alternative sub-hypothesis at
that the DBM achieves abnormal profits from the normal rank approximate model benchmark.
H20: = 0,
H2a: >0,
∴ Rejection of based upon data sample and acceptance of alternative sub-hypothesis at
that the ETBF outperforms the SPDR S&P/ASX 200 fund; statistical evidence for a profitable ETBF product for the risk averse investor.
H0: = 0,
Ha: > 0,
, n1 = n2 = 6, df = min(n1−1,n2−1) = 5, α = 0.05,
The conclusion is rejection of at level of significance
and statistical acceptance for the research primary hypothesis, and endorsement of an exchange-traded horserace betting fund with deterministic payoff for institutional investment consideration.
7. Conclusion and implication
A deterministic betting model for pool wagering is developed from the existing stochastic literature, mathematical pooling principles, and from the development of the MSO theorem over Cn space, to validate the optimal solution generated from the DBM.
The MSO theorem states that optimization over an n finite series of complex systems generates a constant real component over each consecutive system. The MSO theorem has application for all science, and one application is finding optimal solution for deterministic modeling of pool betting. The DBM applied to horse racing optimizes field wagering to determine a feasible, actual payoff—risk trade-off pre-race (or within race). Arbitrage for ETBF is achievable from DBM and market odds trading processes. The structure of the ETBF is a savings fund generating a continuous rate of return.
Testing of betting strategy alpha from race sample invokes a profitable investment strategy of acceptable return—risk for institutional investment, to challenge the existing semi-strong EMH toward horserace betting, and implication for positive benefit to financial markets.
Additional information
Funding
Notes on contributors
Gurudeo Anand Tularam
Craig George Leslie Hopf (BSc, MPhil) is a research associate and Gurudeo Anand Tularam (BSc, PhD) is a senior lecturer in Mathematics and Statistics at Griffith University in Brisbane Australia. Their joint research contribution is toward betting input modeling for legitimate portfolio investment and stock market benefit.
References
- Ali, M. M. (1998). Probability models on horse-race outcomes. Journal of Applied Statistics, 25, 221–229.10.1080/02664769823205
- Bank for International Settlements. (2007). Institutional investors, global savings and asset allocation. Retrieved January 6, 2013, from http://www.bis.org/publ/cgfs27.pdf
- Critchlow, D. E., Fligner, M. A., & Verducci, J. S. (1991). Probability models on rankings. Journal of Mathematical Psychology, 35, 294–318.10.1016/0022-2496(91)90050-4
- Dansie, B. R. (1983). A note on permutation probabilities. Journal of the Royal Statistical Society. Series B (Methodological), 45, 22–24.
- D’Elia, A. (2003). Modelling ranks using the inverse hypergeometric distribution. Statistical Modelling, 3, 65–78.10.1191/1471082X03st047oa
- Edelman, D. (2007). Adapting support vector machine methods for horserace odds prediction. Annals of Operations Research, 151, 325–336.10.1007/s10479-006-0131-7
- Harville, D. A. (1973). Assigning probabilities to the outcomes of multi-entry competitions. Journal of the American Statistical Association, 68, 312–316.10.1080/01621459.1973.10482425
- Henery, R. J. (1983). Permutation probabilities for gamma random variables. Journal of Applied Probability, 20, 822–834.10.2307/3213593
- Hopf, C. G. L. (2014). Exchange traded horserace betting fund with deterministic payoff—a mathematical analysis of a profitable deterministic horserace betting model ( Unpublished master’s thesis, Sup. Tularam, G. A). Griffith University, Brisbane.
- Hopf, C. G. L., & Tularam, G. A. (2014). A mathematical analysis of the inclusion of institutional betting funds into stock market: The case of technical and fundamental payoff models in horserace betting. Journal of Mathematics and Statistics, 10, 390–400.10.3844/jmssp.2014.390.400
- King, D. (2007). Portfolio optimisation and diversification. Journal of Asset Management, 8, 296–307.10.1057/palgrave.jam.2250082
- Koning, R. H., & Van Velzen, B. (2009). Betting exchanges: The future of sports betting? International Journal of Sport Finance, 4, 42–62.
- Lo, V. S., Bacon-Shone, J., & Busche, K. (1995). The application of ranking probability models to racetrack betting. Management Science, 41, 1048–1059.10.1287/mnsc.41.6.1048
- Luce, R. D. (1959). Individual choice behaviour. New York, NY: Wiley.
- Plackett, R. L. (1975). The analysis of permutations. Journal of the Royal Statistical Society Series C, 24, 193–202.
- Roca, E., Wong, V. S. H., & Tularam, G. A. (2010). Are socially responsible investment markets worldwide integrated? Accounting Research Journal, 23, 281–301.
- Snyder, W. W. (1978). Horse racing: Testing the efficient markets model. The Journal of Finance, 33, 1109–1118.10.1111/j.1540-6261.1978.tb02051.x
- State Street Global Advisors. (n.d.). All SPDR ETFS. Retrieved December 1, 2013, from http://www.spdr.com.au/viewall/index.html
- Stern, H. (1990). Models for distributions on permutations. Journal of the American Statistical Association, 85, 558–564.10.1080/01621459.1990.10476235
- Tularam, G. A., Roca, E., & Wong, V. S. H. (2010). Investigation of socially responsible investment markets (SRI) using dynamic conditional correlation (DCC) method: Implications for diversification. Journal of Mathematics and Statistics, 6, 385–394.
- Yang, X. S. (2008). Mathematical modelling for earth sciences. Edinburgh: Dunedin Academic Press. Retrieved from http://www.dunedinacademicpress.co.uk/display.asp?ISB=9781903765920&DS=Mathematical%20Modelling%20for%20Earth%20Sciences
Appendix A1.
Multiple System Optimization Theorem
Multiple System Optimization (MSO) Theorem, Proof by Induction,(A1)
(A1)
(A2)
(A2)
Maximizing or minimizing on the space Cn of n-tuples of complex numbers must satisfy the n-dimensional Cauchy–Riemann equations in order for the complex function to be complex differentiable.(A3)
(A3)
(A4)
(A4)
(A5)
(A5)
(A6)
(A6)
(A7)
(A7)
(A8)
(A8)
Mathematical Induction step,(A 9)
(A 9)
(A10)
(A10)
Appendix A2.
DBM Workings
TrackInvest© Model – Field Win & Place Bet Payoff
Source: 24/02/2012 Riccarton ZS1 (https://tatts.com/racing/racingsearch.aspx).
The payoff function is represented by a complex couple that separates field net payoff from individual racer loss accordingly;
The feasible payoff solution is 30.30% (with nil trade and 100% exposure to shortest priced favorite winning). Trading the market odds reduces the racer exposure (such as hedging to 10% loss), and “arbitrage” optimal payoff solution of 3% is achievable from the bet taking win market odds of 4.7 for the shortest priced racer.
The payoff (%) calculation from SBM is accordingly;
Appendix A3.
ETF and ETBF Daily Payoffs
Source: http://au.finance.yahoo.com/q/hp?s; STW.AX: SPDR S&P/ASX 200 Fund; adj Close: close price adjusted for dividends and splits.