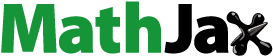
Abstract
A linear stochastic vibration model in state-space form, with output equation
is investigated, where A is the system matrix and b(t) is the white noise excitation. The output equation
can be viewed as a transformation of the state vector x(t) that is mapped by the rectangular matrix S into the output vector x(t). It is known that, under certain conditions, the solution x(t) is a random vector that can be completely described by its mean vector,
, and its covariance matrix,
. If matrix A is asymptotically stable, then
and
, where
is a positive (semi-)definite matrix. Similar results will be derived for some output-related quantities. The obtained results are of special interest to applied mathematicians and engineers.
Public Interest Statement
When a dynamical system with solution vector x of length n describes an engineering problem, only a few components of x are needed, as a rule. But, nevertheless, the whole pertinent initial value problem must be solved. In order to obtain only the components of interest, one defines an output matrix, say S, that selects them from x by defining the new output vector showing its importance. This equation is called output equation. For example, if the engineer wants to apply only the first, second, and nth component, then one defines S as
where
means the ith unit vector for
.
In the present paper, new two-sided estimates on the mean vector and covariance matrix pertinent to the output vector in linear stochastically excited vibration systems are derived that parallel those associated with x obtained recently.
1. Introduction
In order to make the paper more easily readable for a large readership, we first introduce the notions of output vector and output equation common to engineers. When a dynamical system with solution vector x of length n describes an engineering problem, only a few components of x are needed, as a rule. But, nevertheless, the whole pertinent initial value problem must be solved. In order to obtain only the components of interest, one defines an output or transformation matrix, say S, that selects them from x by defining the output . This equation is called output equation. For example, if the engineer wants to use only the first, second, and nth component, then one defines S as
where
means the ith unit vector for
. In other words, by employing the output equation
, a subset of components can be selected from the whole set of degrees of freedom which is usually necessary in practice. Of course, one can also define S such that it forms linear combinations of components of x. Whereas, in the preceding paper Kohaupt (Citation2015b), the whole vector x was analyzed, in the present paper, it is replaced by the output
. The given comments on
show why this is important.
In this paper, a linear stochastic vibration model of the form with output equation
is investigated, where A is a real system matrix, b(t) white noise excitation, and
an initial vector that can be completely characterized by its mean vector
and its covariance matrix
. Likewise, the solution x(t), also called response, is a random vector that can be described by its mean vector
, and its covariance matrix,
. For asymptotically stable matrices A, it is known that
and
, where P is a positive (semi-)definite matrix. Similarly, for the output or transformed quantity
, one has
and
with a positive (semi-)definite matrix
. The asymptotic behavior of
and
was studied in Kohaupt (Citation2015b).
In this paper, we investigate the asymptotic behavior of and
. As appropriate norms for the investigation of this problem, again the Euclidean norm for
and the spectral norm for
is the respective natural choice; both norms are denoted by
.
The main new points of the paper are
the determination of two-sided bounds on
and
,
the derivation of formulas for the right norm derivatives
, and
the application of these results to the computation of the best constants in the two-sided bounds.
Special attention is paid to conditions ensuring the positiveness of the constants in the lower bounds when S is only rectangular and not square regular.
In Section 2, the linear stochastically excited vibration model with output equation is presented. Then, in Section 3, the transformed quantities and
are determined from
and
, respectively, by appropriate use of the output matrix S as transformation matrix. Section 4 derives two-sided bounds on
with
as a preparation to derive two-sided bounds on
in Section 6. Section 5 determines two-sided bounds on
with
as a preparation to derive two-sided bounds on
in Section 7. Section 8 studies the local regularity of
. Then in Section 9, as the main result, formulas for the right norm derivatives
are obtained. Section 10, for the specified data in the stochastically exited model, presents applications, where the differential calculus of norms is employed by computing the best constants in the new two-sided bounds on
and
. In Section , conclusions are drawn. The Appendix A contains sufficient algebraic conditions that ensure the positiveness of the constants in the lower bounds when S is only rectangular and not square regular. Finally, we comment on the References. The author’s papers on the differential calculus of norms are contained in Kohaupt (Citation1999,Citation2001,Citation2002,Citation2003,Citation2004a,Citation2004b,Citation2005,Citation2006,Citation2007a,Citation2007b,Citation2007c,Citation2008a,Citation2008b,Citation2008c,Citation2008d,Citation2009a,Citation2009b,Citation2009c,Citation2010a,Citation2010b,Citation2011,Citation2012,Citation2013,Citation2015a,Citation2015b). The articles Bhatia and Elsner (Citation2003), Benner, Denißen, and Kohaupt (Citation2013,Citation2016), and Whidborne and Amer (Citation2011) refer to some of the author’s works. The publications Coppel (Citation1965), Dahlquist (Citation1959), Desoer and Haneda (Citation1972), Hairer, Nørset, and Wanner (Citation1993), Higueras and García-Celayeta (Citation1999,Citation2000), Hu and Hu (Citation2000), Lozinskiǐ (Citation1958), Pao (Citation1973a,Citation1973b), Söderlind and Mattheij (Citation1985), Ström (Citation1972,Citation1975) contain subjects on the logarithmic norm which was the starting point of the author’s development of the differential calculus of norms. The References Bickley and McNamee (Citation1960), Kučera (Citation1974), and Ma (Citation1966) were important for the author’s article on the equation
in Kohaupt (Citation2008a). The publications Achieser and Glasman (Citation1968), Heuser (Citation1975), Kantorovich and Akilov (Citation1982), Kato (Citation1966), and Taylor (Citation1958) are textbooks on functional analysis useful, for instance, in the proofs of the theorems in Section 5. The books Golub and van Loan (Citation1989), Niemeyer and Wermuth (Citation1987), and Stummel and Hainer (Citation1980) contain chapters on Matrix Theory and Numerical Mathematics valuable in connection with the subject of the present paper. The books Müller (Citation1985), Thomson and Dahleh (Citation1998), and Waller (Citation1975) are on engineering dynamical systems. In paper Guyan (Citation1965), a reduction method for stiffness and mass matrices is discussed, a method that is still in use nowadays. Last, but not least, Kloeden and Platen (Citation1992) is a standard book on the numerical solution of stochastic differential equations.
2. The linear stochastically excited vibration system with output equation
In order to make the paper as far as possible self-contained, we summarize the known facts on linear stochastically excited systems. In the presentation, we closely follow the line of Müller ((MSch, Sections 9.1 and 9.2).
So, let us depart from the deterministic model in state-space form (1)
(1)
(2)
(2)
with system matrix , the state vector
and the excitation vector
, the output matrix
, and the output vector
. We call (2) output equation. It can be understood as a transformation making of x(t) the transformed quantity
by applying the transformation matrix S to x(t).
Now, we replace the deterministic excitation b(t) by a stochastic excitation in the form of white noise. Thus, b(t) can be completely described by the mean vector and the central correlation matrix
with
(3)
(3)
where is the
intensity matrix of the excitation and
the
-function (more precisely, the
-functional).
From the central correlation matrix, for one obtains the positive semi-definite covariance matrix
(4)
(4)
At this point, we mention that the definition of a real positive semi-definite matrix includes its symmetry.
When the excitation is white noise, the deterministic initial value problem (1) can be formally maintained as the theory of linear stochastic differential equations shows. However, the initial state must be introduced as Gaussian random vector,
(5)
(5)
which is to be independent of the excitation; here, the sign means that the initial state
is completely described by its mean vector
and its covariance matrix
. More precisely:
is a Gaussian random vector whose density function is completely determined by
and
alone.
The stochastic response of the system (1) is formally given by(6)
(6)
where besides the fundamental matrix
and the initial vector
a stochastic integral occurs.
It can be shown that the stochastic response x(t) is a non-stationary Gauss–Markov process that can be described by the mean vector and the correlation matrix
. For
, we get the covariance matrix
.
If the system is asymptotically stable, the properties of first and second order for the stochastic response x(t) we need are given by(7)
(7)
(8)
(8)
where the positive semi-definite matrix P satisfies the Lyapunov matrix equation
This is a special case of the matrix equation , whose solution can be obtained by a method of Ma (Citation1966). For the special case of diagonalizable matrices A and B, this is shortly described in Kohaupt (Citation2015b, Appendix A.1).
For asymptotically stable matrices A, one has and thus by (7) and (8),
(9)
(9)
and(10)
(10)
In Kohaupt (Citation2015b), we have investigated the asymptotic behavior of and
.
In this paper, we want to derive formulas for and
corresponding to those of (7) and (8) and study their asymptotic behavior. This will be done in the next five sections, that is, in Sections 3–7.
3. The output-related quantities 
and 

In this section, we determine the output-related quantities and
from the corresponding quantities
and
by appropriate use of the output matrix S as the transformation matrix.
The results of this section are known to mechanical engineers, but are added for the sake of completeness, especially for mathematicians.
One obtains the following lemma.
Lemma 1
(Formulas for and
)
Let and x(t) the solution vector of (1). Further, let
be given by (2), i.e.
Then, one has(11)
(11)
(12)
(12)
with(13)
(13)
and(14)
(14)
Proof
(i) | One has | ||||
(ii) | Next, we show that, for the central correlation matrices |
Taking into account (8), this leads to (12).
Remark Let the system matrix A be asymptotically stable. Then, and thus from (12) and (13),
as well as
4. Two-sided bounds on 
with 

In this section, we discuss the deterministic case with
as a preparation for Section 6. There, two-sided bounds on
will be given based on those for
here.
For the positiveness of the constants in the lower bounds, we discuss two cases: the special case when matrix A is diagonalizable and the case of a general square matrix A.
Let and
(17)
(17)
We obtain
Theorem 1
(Two-sided bound on by
)
Let ,
, and x(t) be the solution of the initial value problem
. Let
be any vector norm.
Then, there exists a constant and for every
a constant
such that
(18)
(18)
where is the spectral abscissa of A with respect to
.
If A is diagonalizable, then may be chosen, and we write
instead of
.
If S is square and regular, then .
Proof
One has(19)
(19)
Further, according to Kohaupt (Citation2006, Theorem 8), there exists a constant and for every
a constant
such that
(20)
(20)
Combining (19) and (20) leads to (18) with .
Further, if S is square and regular, then instead of (19) we get(21)
(21)
Thus, apparently, can be chosen in (18).
An interesting and important question is under what conditions the constant is positive when S is only rectangular, but not necessarily square and regular. To assert that
is positive, additional conditions have to be imposed. We consider two cases.
Case 1: Diagonalizable matrix A In this case, we need the following hypotheses on A from Kohaupt (Citation2011, Section 3.1).
(H1) |
| ||||
(H2) |
| ||||
(H3) |
| ||||
(H4) |
| ||||
(HS) | the eigenvectors |
Representation of the basis
Under the hypotheses (H1), (H2), and (HS), from Kohaupt (Citation2011), we obtain the following real basis functions for the ODE :
(22)
(22)
, where
are the decompositions of
and
into their real and imaginary parts. As in Kohaupt (Citation2011), the indices are chosen such that
.
The spectral abscissa of A with respect to the initial vector Let,
be the eigenvectors of
corresponding to the eigenvalues
. Under (H1), (H2), and (HS), the solution x(t) of (1) has the form
(23)
(23)
with uniquely determined coefficients . Using the relations
(24)
(24) (see Kohaupt, Citation2011, Section 3.1 for the last relation), then according to Kohaupt (Citation2011), the spectral abscissa of A with respect to the initial vector
is given by
(25)
(25) Index sets In the sequel, we need the following index sets:
(26)
(26)
and(27)
(27) Appropriate representation of x(t) We have
(28)
(28)
with(cf. Kohaupt, Citation2011). Thus, due to (28) and (22),
(29)
(29)
with(30)
(30)
.
Appropriate representation of y(t) and (needed in the Appendix) Let
(31)
(31)
with ,
,
. Then, from (29), (30),
(32)
(32)
with(33)
(33)
as well as
(34)
(34)
with(35)
(35)
.
Herewith, one obtains(36)
(36)
for a corresponding estimate on x(t), compare Kohaupt (Citation2011, (10)).
Now, let(37)
(37)
Then, similarly as in Kohaupt (Citation2011, (12)),(38)
(38)
Together with (36), this entails(39)
(39)
with
for sufficiently large . Thus, we obtain
Theorem 2
(Positiveness of the constant in lower bound if A diagonalizable)
Let the hypotheses (H1), (H2), and (HS) for A be fulfilled, ,
, A be diagonalizable as well as condition (37) be satisfied.
Then, there exists a positive constant such that
(40)
(40)
for sufficiently large .
If , then
can be chosen.
Proof
The last statement is proven similarly as in the proof of Kohaupt (Citation2011, Theorem 2).
Remarks
As opposed to (37), the relation
in Kohaupt (Citation2011, (11)) could be proven there and thus needed not be assumed.
We mention that the quantities
depend on the initial vector
through their coefficients
(Kohaupt, Citation2011, (8)). To stress this fact, one can write
or
.
Case 2 General square matrix A In this case, we need the following hypotheses on A from Kohaupt (Citation2011, Section 3.2).
(H1′) |
| ||||
(H2′) |
| ||||
(H3′) |
| ||||
(H4′) |
| ||||
(HS′) |
|
Representation of the basis
Under the hypotheses ,
, and
, from Kohaupt (Citation2011) we obtain the following real basis functions for the ODE
:
(41)
(41)
, where
is the decomposition of into its real and imaginary part.
The spectral abscissa of A with respect to the initial vector Let
be the principal vectors of stage k of
corresponding to the eigenvalue
. Under
,
, and
, the solution x(t) of (1) has the form
(42)
(42)
with uniquely determined coefficients . Using the relations
(43)
(43) (see Kohaupt, Citation2011, Section 3.2 for the last relation), then the spectral abscissa of A with respect to the initial vector
is
(44)
(44) Index sets For the sequel, we need the following index sets:
(45)
(45)
and(46)
(46) Appropriate representation of x(t) We have
(47)
(47)
with(cf. Kohaupt, Citation2011). Thus, due (47),
(48)
(48)
with(49)
(49)
.
Appropriate representation of y(t) and (needed in the Appendix) Set
(50)
(50)
with ,
,
.
Then, from (48), (49)(51)
(51)
with(52)
(52)
as well as(53)
(53)
with(54)
(54)
.
Now, let(55)
(55)
Then, similarly as in Kohaupt (Citation2011, Section 3.2), there exists a constant such that
(56)
(56)
for sufficiently large .
Thus, we obtain
Theorem 3
(Positiveness of the constant in lower bound if A general square)
Let the hypotheses ,
, and
for A be fulfilled,
,
, A be a general square matrix as well as condition (55) be satisfied.
Then, there exists a positive constant such that
(57)
(57)
for sufficiently large .
If , then
can be chosen.
Remark Sufficient algebraic conditions for (37) resp. (55) will be given in the Appendix; they are independent of the initial vector and the time t.
5. Two-sided bounds on 
with 
, 

In this section, we discuss the deterministic case with
,
as a preparation for Section 7. There, two-sided bounds on
will be given based on those for
here.
Moreover, for the positiveness of the constant in the lower bound, we discuss two cases: the special case when matrix A is diagonalizable and the case when A is general square.
We obtain
Theorem 4
(Two-sided bound on based on
)
Let , let
be the associated fundamental matrix with
where E is the identity matrix. Further, let
be the covariance matrices from Section 2.
Then,(58)
(58)
where(59)
(59)
and(60)
(60)
If , then
. If
is regular, then
(61)
(61)
Proof
The proof follows from Kohaupt (Citation2015b, Lemmas 1 and 2) with and
as well as
.
Next, we have to derive two-sided bounds on . For this, we write
(62)
(62)
where are the columns of the fundamental matrix
, i.e.
Now, ,
is equivalent to
(63)
(63)
where is the jth unit column vector.
The two-sided bounds on can be done in any norm. Let the matrix norm
be given by
Then,(64)
(64)
Thus, the two-sided bound on has been reduced to two-sided bounds on
.
Similarly to Theorem 2, we obtain
Theorem 5
(Two-sided bound on by
) Let
and
be the fundamental matrix of A with
, i.e. let
be the solution of the initial value problem
and
.
Then, there exists a constant and for every
a constant
such that
(65)
(65)
where is the spectral abscissa of A.
If A is diagonalizable, then may be chosen, and we write
instead of
.
If S is square and regular, then .
Proof
From (18) and (63), there exist constants and for every
constants
such that
(66)
(66)
Define
and
Then, taking into account (64) and the relation(67)
(67) (cf. Kohaupt, Citation2006, Proof of Theorem 7) as well as the equivalence of norms in finite-dimensional spaces, the two-sided bound (65) follows. The rest is clear from Theorem 1.
Corresponding to Theorems 2 and 3, we obtain the following two theorems.
Theorem 6
(Positiveness of the constant in lower bound if A diagonalizable) Let the hypotheses (H1), (H2), and (HS) for A be fulfilled,
, A be diagonalizable as well as condition (37) be satisfied with
.
Then, there exists a positive constant such that
(68)
(68)
for sufficiently large .
If , then
can be chosen.
Theorem 7
(Positiveness of the constant in lower bound if A general square) Let the hypotheses
,
, and
for A be fulfilled,
, A be a general square matrix as well as condition (55) be satisfied.
Then, there exists a positive constant such that
(69)
(69)
for sufficiently large .
If , then
can be chosen.
6. Two-sided bounds on 

According to Equation (11), we have
Now, in Theorem 1. Assuming
and choosing the Euclidean norm
as well as
instead of
, we therefore obtain from Theorem 1 for every
the two-sided bound
(70)
(70)
for constants and
. Sufficient conditions for
are obtained by Theorems 2 and 3 when replacing there
by
.
7. Two-sided bounds on 

Based on Theorems 4 and 5, we obtain
Corollary 1
(Two-sided bounds on based on
) Let
, let
be the associated fundamental matrix with
, where E is the identity matrix, as well as
and
. Further, let
be the covariance matrices from Section 2.
Then, there exists a constant and for every
a constant
such that
(71)
(71)
If and S are regular, then
.
Remark If is not square regular, under additional conditions stated in Theorems 6 and 7, it can also be asserted that
.
8. Local regularity of the function 

We have the following lemma which states loosely speaking
that for every
, the function
is real analytic in some right neighborhood
.
Lemma 2
(Real analyticity of on
) Let
. Then, there exists a number
and a function
, which is real analytic on
such that
.
Proof
Based on , the proof is similar to that of Kohaupt (Citation2002, Lemma 1). The details are left to the reader.
9. Formulas for the norm derivatives 

Let and
with
. As in Kohaupt (Citation2015b, Section 7), we set
Let and define
Then,
Similarly as in Kohaupt (Citation2015b, Section 7), for ,
with, and thus
with
where the quantities are defined in Kohaupt (Citation2015b, Section 7).
Consequently, one obtains the formulas for
from those for when replacing
by
.
10. Applications
In this section, we apply the new two-sided bounds on obtained in Section 7 as well as the differential calculus of norms developed in Sections 8 and 9 to a linear stochastic vibration model with output equation for asymptotically stable system matrix and white noise excitation vector.
In Section , the stochastic vibration model as well as its state-space form is given, in Subsection 10.2 the transformation matrix S in chosen and in Section 10.3 the data are specified. In Section 10.4, the positiveness of the constants and
in the lower bounds is verified. In Section 10.5, computations with the chosen data are carried out, such as the computation of P and
as well as the computation of the curves
and of the curve
along with its best upper and lower bounds for the two ranges
and
. In Section 10.6, computational aspects are shortly discussed.
10.1. The stochastic vibration model and its state-space form
Consider the multi-mass vibration model in Figure .
The associated initial-value problem is given by
where and
as well as
Here, y is the displacement vector, f(t) the applied force, and M, B, and K are the mass, damping, and stiffness matrices, as the case may be.
In the state-space description, one obtains
with , and
, where the initial vector
is characterized by the mean vector
and the covariance matrix
.
The system matrix A and the excitation vector b(t) are given by
respectively. The vector x(t) is called state vector.
The (symmetric positive semi-definite) intensity matrix is obtained from the (symmetric positive semi-definite) intensity matrix
by
See Muller and Schiehlen (Citation1985, (9.65)) and the derivation of this relation in Kohaupt (Citation2015b, Appendix A.5).
10.2. The transformation matrix S and the output equation 

We depart from the equation of motion in vector form, namely , and rewrite it as
Following Müller (Citation1985, (9.56), (9.57)), for a one-mass model with base excitation, we call the absolute acceleration of our vibration system; it can be written as
with the transformation matrix
Our output equation therefore is
where here is a rectangular, but not a square regular matrix.
10.3. Data for the model
As of now, we specify the values as
and
Then,(if n is even), and
We choose n = 5 in this paper so that the state-space vector x(t) has the dimension .
For , we take
with
and
similarly as in Kohaupt (Citation2002) for and
. For the
matrix
, we choose
The white-noise force vector f(t) is specified as
so that its intensity matrix with
has the form
We choose
With , this leads to (see Kohaupt, Citation2015b, Appendix A.5)
10.4. Positiveness of the constants 
and 
(resp. 
)
The eigenvalues of matrix A are given by
and
where the numbering is chosen such that . So,
Thus, matrix A is diagonalizable. Further, conditions (H1)-(H4), and (HS) are fulfilled. Moreover, we have and
Therefore,
are linearly independent. Thus, by Lemma A.1 and Theorem 2 resp. Theorem 6, the constants
and
are positive. Therefore, also the constant
is positive.
10.5. Computations with the specified data
(i) | Bounds on | ||||
(ii) | Computation of P and | ||||
(iii) | Computation of the curves | ||||
(iv) | Bounds on |
Numerical values for range [0;5] First, we consider the range [0;5]. From the initial guess , the computations deliver the values
To compute the lower bound, we have to notice that the curve has kinks like
at
. This is not seen in Figures – in the range [0;5], but in Figure in the range [5;25]. Therefore, the point of contact
between the lower bound
and the curve
cannot be determined by the calculus of norms, but must be computed from
where are the local minima of
. In this way, with the initial guess
, the results are
In Figure , the curve along with the best upper and lower bounds are illustrated with stepsize
. The upper bound is valid for
.
Numerical values for range [5;25] On the rage [5;25], the two-sided bounds can be better adapted to the curve . From the initial guess
, the computations deliver
Further, with the initial guess , we obtain
In Figure , the curve along with the best upper and lower bounds are illustrated with stepsize
. The upper bound is valid for
.
10.6. Computational aspects
In this subsection, we say something about the computer equipment and the computation time for some operations.
(i) | As to the computer equipment, the following hardware was available: an Intel Pentium D(3.20 GHz, 800 MHz Front-Side-Bus, 2x2MB DDR2-SDRAM with 533 MHz high-speed memories). As software package, we used MATLAB, Version 6.5. | ||||
(ii) | The computation time t of an operation was determined by the command sequence |
11. Conclusion
In the present paper, a linear stochastic vibration system of the form with output equation
was investigated, where A is the system matrix and b(t) white noise excitation. The output equation
is viewed as a transformation of the state vector x(t) mapped by the rectangular matrix S into the output vector
. If the system matrix A is asymptotically stable, then the mean vector
and the covariance matrix
both converge with
and
for some symmetric positive (semi-)definite matrix
. This raises the question of the asymptotic behavior of both quantities. The pertinent investigations are made in the Euclidean norm
for
and in the spectral norm, also denoted by
, for
. The main new points are the derivation of two-sided bounds on both quantities, the determination of the right norm derivatives
and, as application, the computation of the best constants in the bounds. In the presentation, the author exhibits the relations between the quantities
,
, and the formulas for
, on the one hand, and the corresponding output-related quantities
,
, and
, on the other hand. As a result, we obtain that there is a close relationship between these quantities. Special attention is paid to the positiveness of the constants in the lower bounds if the transformation matrix is only rectangular and not necessarily square and regular. In the Appendix, a sufficient algebraic condition for the positiveness of the constants in the lower bounds is derived that is independent of the initial vector and the time variable. To make sure that the (new) formulas for
are correct, we have checked them by various difference quotients. They underpin the correctness of the numerical values for the specified data.
The computation time to generate the last figure with a matrix A is about 6 seconds. Of course, in engineering practice, much larger models occur. As in earlier papers, we mention that in this case engineers usually employ a method called condensation to reduce the size of the matrices.
We have shifted the details of the positiveness of the constants in the lower bounds to the Appendix in order to make the paper easier to comprehend.
The numerical values were given in order that the reader can check the results.
Altogether, the results of the paper should be of interest to applied mathematicians and particularly to engineers.
Acknowledgements
The author would like to give thanks to the anonymous referees for evaluating the paper and for comments that led to a better presentation of the paper.
Additional information
Funding
Notes on contributors
L. Kohaupt
Ludwig Kohaupt received the equivalent to the master’s degree (Diplom-Mathematiker) in Mathematics in 1971 and the equivalent to the PhD (Dr.phil.nat.) in 1973 from the University of Frankfurt/Main. From 1974 until 1979, Kohaupt was a teacher in Mathematics and Physics at a Secondary School. During that time (from 1977 until 1979), he was also an auditor at the Technical University of Darmstadt in Engineering Subjects, such as Mechanics, and especially Dynamics. From 1979 until 1990, he joined the Mercedes-Benz car company in Stuttgart as a Computational Engineer, where he worked in areas such as Dynamics (vibration of car models), Cam Design, Gearing, and Engine Design. Then, in 1990, Dr. Kohaupt combined his preceding experiences by taking over a professorship at the Beuth University of Technology Berlin (formerly known as TFH Berlin). He retired on April 01, 2014.
References
- Achieser, N. I., & Glasman, I. M. (1968). Theorie der linearen Operatoren im Hilbert--Raum [Theory of linear operators in Hilbert space]. Berlin: Akademie-Verlag.
- Bhatia, R., & Elsner, L. (2003). Higher order logarithmic derivatives of matrices in the spectral norm. SIAM Journal on Matrix Analysis and Applications, 25, 662–668.
- Benner, P., Deni{\ss}en, J., & Kohaupt, L. (2013). Bounds on the solution of linear time-periodic systems. Proceedings in Applied Mathematics and Mechanics, 13, 447–448.
- Benner, P., Deni{\ss}en, J., & Kohaupt, L. (2016, January 12). Trigonometric spline and spectral bounds for the solution of linear time-periodic systems ( Preprint MPIMD/16-1). Magdeburg: Max Planck Institute for Dynamics of Complex Technical Systems. Retrieved from https://protect-us.mimecast.com/s/oXA1BRUQvnpoto
- Bickley, W. G., & McNamee, J. (1960). Matrix and other direct methods for the solution of linear difference equations. Philosophical Transactions of the Royal Society of London: Series A, 252, 69–131.
- Coppel, W. A. (1965). Stability and asymptotic behavior of differential equations. Boston, MA: D.C. Heath.
- Dahlquist, G. (1959). Stability and error bounds in the numerical integration of ordinary differential equations. Transactions of the Royal Institute of Technology, Stockholm, No. 130. Uppsala; Almqvist and Wiksells Boktryckeri AB.
- Desoer, Ch. A., & Haneda, H. (1972). The measure of a matrix as a tool to analyse computer algorithms for circuit analysis. IEEE Transaction on Circuit Theory, 19, 480–486.
- Golub, G. H., & van Loan, Ch F (1989). Matrix computations. Baltimore, MD: Johns Hopkins University Press.
- Guyan, R. J. (1965). Reduction of stiffness and mass matrices. AIAA Journal, 3, 380.
- Hairer, E., N{\o}rset, S. P., & Wanner, G. (1993). Solving ordinary differential equations I. Berlin: Springer-Verlag.
- Heuser, H. (1975). Funktionalanalysis [ Functional analysis]. Stuttgart: B.G. Teubner.
- Higueras, I., & García-Celayeta, B. (1999). Logarithmic norms for matrix pencils. SIAM Journal on Matrix Analysis and Applications, 20, 646–666.
- Higueras, I., & Garc\’{\i}a-Celayeta, B. (2000). How close can the logarithmic norm of a matrix pencil come to the spectral abscissa? SIAM Journal on Matrix Analysis and Applications, 22, 472–478.
- Hu, G.-D., & Hu, G.-D. (2000). A relation between the weighted logarithmic norm of a matrix and the Lyapunov equation. BIT, 40, 606–610.
- Kantorovich, L. V., & Akilov, G. P. (1982). Funktional analysis. Oxford: Pergamon Press.
- Kato, T. (1966). Perturbation theory for linear operators. New York, NY: Springer.
- Kloeden, P., & Platen, E. (1992). Numerical solution of stochastic differential equations. Springer-Verlag.
- Kohaupt, L. (1999). Second logarithmic derivative of a complex matrix in the Chebyshev norm. SIAM Journal on Matrix Analysis and Applications, 21, 382–389.
- Kohaupt, L. (2001). Differential calculus for some p-norms of the fundamental matrix with applications. Journal of Computational and Applied Mathematics, 135, 1–21.
- Kohaupt, L. (2002). Differential calculus for p-norms of complex-valued vector functions with applications. Journal of Computational and Applied Mathematics, 145, 425–457.
- Kohaupt, L. (2003). Extension and further development of the differential calculus for matrix norms with applications. Journal of Computational and Applied Mathematics, 156, 433–456.
- Kohaupt, L. (2004a). Differential calculus for the matrix norms and with applications to asymptotic bounds for periodic linear systems. International Journal of Computer Mathematics, 81, 81–101.
- Kohaupt, L. (2004b). New upper bounds for free linear and nonlinear vibration systems with applications of the differential calculus of norms. Applied Mathematical Modelling, 28, 367–388.
- Kohaupt, L. (2005). Illustration of the logarithmic derivatives by examples suitable for classroom teaching. Rocky Mountain Journal of Mathematics, 35, 1595–1629.
- Kohaupt, L. (2006). Computation of optimal two-sided bounds for the asymptotic behavior of free linear dynamical systems with application of the differential calculus of norms. Journal of Computational Mathematics and Optimization, 2, 127–173.
- Kohaupt, L. (2007a). New upper bounds for excited vibration systems with applications of the differential calculus of norms. International Journal of Computer Mathematics, 84, 1035–1057.
- Kohaupt, L. (2007b). Short overview on the development of a differential calculus of norms with applications to vibration problems (in Russian). Information Science and Control Systems, 13, 21–32. ISSN 1814-2400.
- Kohaupt, L. (2007c). Construction of a biorthogonal system of principal vectors of the matrices A and A* with applications to the initial value problem. Journal of Computational Mathematics and Optimization, 3, 163–192.
- Kohaupt, L. (2008a). Solution of the matrix eigenvalue problem with applications to the study of free linear systems. Journal of Computational and Applied Mathematics, 213, 142–165.
- Kohaupt, L. (2008b). Biorthogonalization of the principal vectors for the matrices and with application to the computation of the explicit representation of the solution of. Applied Mathematical Sciences, 2, 961–974.
- Kohaupt, L. (2008c). Solution of the vibration problem without the hypothesis or. Applied Mathematical Sciences, 2, 1989–2024.
- Kohaupt, L. (2008d). Two-sided bounds on the difference between the continuous and discrete evolution as well as on with application of the differential calculus of norms. In M. P. {\’A}lvarez (Ed.), Leading-edge applied mathematical modeling research (pp. 319–340). Nova Science. ISBN 978-1-60021-977-1.
- Kohaupt, L. (2009a, July 10--13). A short overview on the development of a differential calculus for norms with applications to vibration problems. In The 2nd International Multi-Conference on Engineering and Technological Innovation (Vol. II). Orlando, FL. ISBN-10: 1–934272-69-8, ISBN13: 978-1-934272-69-5
- Kohaupt, L. (2009b). On an invariance property of the spectral abscissa with respect to a vector. Journal of Computational Mathematics and Optimization, 5, 175–180.
- Kohaupt, L. (2009c). Contributions to the determination of optimal bounds on the solution of ordinary differential equations with vibration behavior (Habilitation Thesis, TU Freiberg, 91 pp.). Aachen: Shaker-Verlag.
- Kohaupt, L. (2010). Two-sided bounds for the asymptotic behavior of free nonlinear vibration systems with application of the differential calculus of norms. International Journal of Computer Mathematics, 87, 653–667.
- Kohaupt, L. (2010b, January). Phase diagram for norms of the solution vector of dynamical multi-degree-of-freedom systems. Lagakos, et al. (Eds.), Recent advances in applied mathematics (pp. 69–74, 27--29). American Conference on Applied Mathematics (American Math10), WSEAS Conference in Cambridge/USA at Harvard University. WSEAS Press. ISBN 978-960-474-150-2, ISSN 1790-2769.
- Kohaupt, L. (2011). Two-sided bounds on the displacement and the velocity of the vibration problem with application of the differential calculus of norms. The Open Applied Mathematics Journal, 5, 1–18.
- Kohaupt, L. (2012). Further investigations on phase diagrams for norms of the solution vector of multi-degree-of-freedom systems. Applied Mathematical Sciences, 6, 5453–5482. ISSN: 1312–885X.
- Kohaupt, L. (2013). On the vibration-suppression property and monotonicity behavior of a special weighted norm for dynamical systems. Applied Mathematics and Computation, 222, 307–330.
- Kohaupt, L. (2015a). On norm equivalence between the displacement and velocity vectors for free linear dynamical systems. Cogent Mathematics, 2, 1095699 (33 p.).
- Kohaupt, L. (2015b). Two-sided bounds on the mean vector and covariance matrix in linear stochastically excited vibration systems with application of the differential calculus of norms. Cogent Mathematics, 2, 1021603 ( 26 p).
- Kučera, V. (1974). The matrix equation A X+X B = C. SIAM Journal on Applied Mathematics, 26, 15–25.
- Lozinski\v{i}, S. M. (1958). Error estimates for the numerical integration of ordinary differential equations I (in Russian). Izv. Vys\v{s}. U\v{c}ebn. Zaved Matematika, 5, 52–90.
- Ma, E.-Ch. (1966). A finite series solution of the matrix equation A X - X B = C. SIAM Journal of Applied Mathematics, 14, 490–495.
- Müller, P. C., & Schiehlen, W. O. (1985). Linear vibrations. Dordrecht: Martinus Nijhoff.
- Niemeyer, H., & Wermuth, E. (1987). Lineare algebra [Linear algebra]. Braunschweig: Vieweg.
- Pao, C. V. (1973a). Logarithmic derivatives of a square matrix. Linear Algebra and its Applications, 6, 159–164.
- Pao, C. V. (1973b). A further remark on the logarithmic derivatives of a square matrix. Linear Algebra and its Applications, 7, 275–278.
- S{\"o}derlind, G., & Mattheij, R. M. M. (1985). Stability and asymptotic estimates in nonautonomous linear differential systems. SIAM Journal on Mathematical Analysis, 16, 69–92.
- Str{\"o}m, T. (1972). Minimization of norms and logarithmic norms by diagonal similarities. Computing, 10, 1–7.
- Str{\"o}m, T. (1975). On logarithmic norms. SIAM Journal on Numerical Analysis, 10, 741–753.
- Stummel, F., & Hainer, K. (1980). Introduction to numerical analysis. Edinburgh: Scottish Academic Press.
- Taylor, A. E. (1958). Introduction to functional analysis. New York, NY: Wiley.
- Thomson, W. T., & Dahleh, M. D. (1998). Theory of vibration with applications. Upper Saddle River, NJ: Prentice-Hall.
- Waller, H., & Krings, W. (1975). Matrizenmethoden in der Maschinen- und Bauwerksdynamik [Matrix methods in machine and building dynamics]. Mannheim: Bibliographisches Institut.
- Whidborne, J. F., & Amer, N. (2011). Computing the maximum transient energy growth. BIT Numerical Mathematics, 51, 447–457.
Appendix 1
Algebraic conditions ensuring the positiveness of 
resp. 
for 
.
We discuss two cases, namely the case of a diagonalizable matrix A and the case of a general square matrix A.
The corresponding Lemmas A.1 and A.2 will deliver sufficient algebraic criteria for the positiveness of and
, as the case may be, that are independent of the initial condition and the time, which is the important point.
The results are of interest on their own.
Case 1: Diagonalizable matrix A
Let the hypotheses (H1), (H2), and (HS) be fulfilled. Further, according to (37), we assume(A1)
(A1)
with
so that
Thus, (37) resp. (72) is equivalent to(A2)
(A2)
We have the following
Sufficient condition for :
(A3)
(A3) (see Kohaupt, Citation2011, Section 5.1 for a similar case).
Lemma 3
(Some equivalences of the sufficient algebraic condition)
Let the conditions (H1), (H2), (H3), and (HS) be fulfilled. Further, let and M be regular.
Then, the following equivalences are valid:(A4)
(A4)
(A5)
(A5)
(A6)
(A6)
(A7)
(A7)
Proof
The equivalences (75) (76) and (77)
(78) are clear. Further, since
(A8)
(A8) (76) is equivalent to
(A9)
(A9)
Since(80) is equivalent to
(A10)
(A10)
Since due to (H3) and M is regular by assumption, (81) is equivalent to (77).
Case 2: General square matrix A Let the hypotheses ,
,
, and
be fulfilled. Further, according to (55), we assume
(A11)
(A11)
with
so that. Thus, (55) resp. (82) is equivalent to
(A12)
(A12)
We have the following
Sufficient condition for :
(A13)
(A13) (see Kohaupt, Citation2011, Section 5.2 for a similar case).
Lemma 4
(Some equivalences of the sufficient algebraic condition) Let the hypotheses ,
,
, and
be fulfilled. Further, let
, and M be regular.
Then, the following equivalences are valid:(A14)
(A14)
(A15)
(A15)
(A16)
(A16)
(A17)
(A17)
Proof
The equivalences (85) (86) and (87)
(88) are clear.
Now we prove the equivalence of (86) and (87). From the relations(A18)
(A18)
with(A19)
(A19)
, in a first step, we derive associated relations for
and
,
. Now, (89) means
that is,
(A20)
(A20)
or(A21)
(A21)
. Based on (93) and the assumed regularity of M, we see that (86) is equivalent to
(A22)
(A22) In the second step, we show the equivalence of (94) with the following condition:
(A23)
(A23)
and then in the third step, the equivalence of (95) with (87).
Equivalence of (94) and (95):
(94) (95): So, let (94) be fulfilled. We write
in the form
(A24)
(A24)
, where the
are also principal vectors of stage k corresponding to the eigenvalue
. This is done as follows:
where is a principal vector of stage 1 (or eigenvector) and
. Similarly,
where is a principal vector of stage 2 corresponding to the eigenvalue
.
Proceeding in this way, using induction, (96) is proven.
Therefore, apart from (94), also the following property must hold:(A25)
(A25)
which proves (95).
(95) (94): Let (95) be fulfilled and
Fully written, we obtain with ,
or regrouping
By assumption, from the last line, we get
or
further,
leading to
Continuing in this way, we ultimately obtain
so that (94) is proven.
Further, because of the representation (92), then (95) is equivalent to(A26)
(A26)
Similarly as above, this is equivalent to (87).
On the whole, Lemma A.2 is proven.
Alternative proof for the positiveness of resp.
when
In the special case of , there is an alternative proof for the positiveness of
. This alternative proof is simpler, but the foregoing one is applicable to more general forms of S.
We employ the vector resp. matrix norm to obtain
with
so that
Due to the equivalence of norms in finite-dimensional spaces, this entails that for every vector norm one has
with a positive constant .
A similar proof for the positiveness of is possible. This is left to the reader.