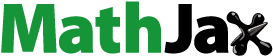
Abstract
This paper presents the nonparametric estimation of first and second infinitesimal moments of the underlying jump-diffusion model with asymmetric kernel functions. In particular, we use asymmetric kernel estimators characterized by the gamma distribution. This approach allows to conciliate the idea of using the asymmetric kernel with jump-diffusion models. We show that the proposed estimators are consistent and asymptotically follow normal distribution under the conditions of recurrence and stationarity.
Public Interest Statement
Nonparametric methods have become increasingly popular research fields e.g. statistics, economics, and probability communities. The main advantage of the nonparametric methods is that they are helpful to estimate the coefficients of underlying model in a flexible way. This study concerned with the nonparametric estimation of infinitesimal moments of jump-diffusion models with asymmetric kernel functions. The author showed that the proposed estimators are consistent and asymptotically follow normal distribution under the conditions of recurrence as well as for stationarity.
1. Introduction
Over the past few decades, nonparametric methods have become increasingly popular research fields e.g. statistics, economics, and probability communities, and such a trend undoubtedly will continue in future. The main advantage of the nonparametric methods is that they are helpful to estimate the coefficients of underlying model in a flexible way. In this paper, we study the nonparametric estimation of infinitesimal moments of jump-diffusion models with asymmetric kernel functions. Our approach allows us to conciliate the idea of using the asymmetric kernel functions and jump-diffusion models. We show that the proposed estimators are consistent and asymptotically follow normal distribution under the conditions of recurrence as well as for stationarity.
In nonparametric estimation, the traditional kernel smoothing has been playing a wide role in estimating continuous-time diffusion processes since many years. Most of the kernels which are used in the literature are symmetric kernels and fixed. Several researchers have used nonparametric methods based on traditional kernel smoothing and studied the diffusion model and jump-diffusion models (cf. Arapis & Gao, Citation2006; Bandi & Phillips, Citation2003; Nicolau, Citation2003; Stanton, Citation1997). Furthermore, the bias of the standard kernel estimator is larger near the boundary than in the interior. Previously, many authors have proposed the methods to remove the boundary bias (see e.g. Fan & Gijbels, Citation1992; Rice, Citation1984; Zhang, Karunamuni, & Jones, Citation1999 and many others).
Asymmetric kernels are useful for the density estimation with a number of advantages such as they are free from boundary bias and are always nonnegative. They also achieve the optimal rate of convergence within the class of nonnegative kernel density estimators. Chen (Citation2000) studied that replacing fixed kernels with asymmetric kernels substantially had increased the precision of density estimation close to boundary. Hanif (Citation2015) studied the nonparametric estimation of drift coefficient and diffusion coefficient in the second-order diffusion equation by using the asymmetric kernel functions. He proved that the proposed estimators of second-order diffusion equation are consistent and asymptotically follow normal distribution. Other successful applications of asymmetric kernels include Bertin and Klutchnikoff (Citation2011), Bouezmarni and Scaillet (Citation2005), Gustafsonn, Hagmann, Nielsen, and Scaillet (Citation2009), Hagmann and Scaillet (Citation2007), Scaillet (Citation2004), and Shunpu (Citation2010); where such kernels are shown to lead desirable properties.
A continuous-time model governed by the stochastic differential equation has the following form:(1.1)
(1.1)
where is a standard Brownian motion. The functions
and
are drift and diffusion functions of the process. The diffusion model defined in Equation (1.1) is widely used to describe the properties of underlying economic variables. Gospodinovy and Hirukawa (Citation2012) studied the gamma kernel for diffusion model and obtained the asymptotic normality for the drift and the diffusion functions. While, we have studied the asymmetric kernel functions for the jump-diffusion models. The purpose of the present paper is to study gamma and beta Nadaraya–Watson estimators of first and second infinitesimal moments of jump-diffusion models. We obtain the consistency and asymptotic normality of estimators under recurrence and stationarity. Furthermore, we study the infinite jump activity case in finite interval. In other words, the jumps parts in this paper can be compensated.
The rest of the paper is organized as follows: Section 2 introduces the jump-diffusion models and asymmetric kernel functions. This Section defines the conditional moments of the jump-diffusion model and derive the gamma and beta kernel estimators for the conditional moments and Section 3 presents assumptions, some useful preliminary results about the local time and the main results of our paper. Section 4 contains the proofs of the presented results.
2. Jump-diffusion model and asymmetric kernel functions
During the last decade, jump-diffusion models have been considered as a valuable tool in the modeling of stochastic differential equations. The jump-diffusion models have been widely used in a variety of financial applications. Usually, jump-diffusion models consist of two parts, the Brownian motion and the Poisson process. The first attempt to incorporate jumps into diffusion model was made by Merton (Citation1976). Later on, jump-diffusion models have been extended in various directions by specifying different structures for the drift, diffusion, and jump components. Kou (Citation2002) and Ramezani and Zeng (Citation2007) used an asymmetric double exponential distribution for log of jump part and showed that the resulting model could capture asymmetric leptokurtic features and volatility smile features frequently observed in financial data. For other contributions on jump-diffusion processes and their applications, see, AÏt-Sahalia and Jacod (Citation2009), Bandi and Nguyen (Citation2003), Beckers (Citation1981), and the references therein.
Our motivation is to study the nonparametric estimation for jump-diffusion model stated below:(2.1)
(2.1)
where and
are smooth functions,
is a standard Brownian motion,
is a jump process independent of
, and
is the conditional intensity of the jumps. Furthermore, we use
to characterize the impact of jumps, where
is a random variable with range Y and
is the probability distribution function of
. There are several studies on specification of
For example,
where N is a Poisson process with an intensity
and
is the jump size having the time-invariant distribution
. We denote
, and have
(2.2)
(2.2)
The integral form is as follows:(2.3)
(2.3)
where(2.4)
(2.4)
is a compensated Poisson random measure. From Equations (2.2) and (2.4), we have(2.5)
(2.5)
and it represents the variation between t and due to discontinuous jumps. The conditional moments of the jump-diffusion models are defined by the following relations (cf. Bandi & Nguyen, Citation2003):
(2.6)
(2.6)
(2.7)
(2.7)
Many researchers have used nonparametric techniques to study the jump-diffusion models. Schmisser (Citation2014) discussed the nonparametric adaptive estimation of the drift for a jump-diffusion process and provided the bounds for the risks of the two estimators for ergodic and strictly stationary. Lin and Wang (Citation2010) considered the empirical likelihood inference for jump-diffusion model. Hanif, Lin, and Wang (Citation2012) discussed nonparametric estimation of the second infinitesimal moment by using the reweighted Nadaraya–Watson approach of the underlying jump-diffusion model and established the strong consistency and asymptotic normality of the second infinitesimal moment. Hanif (Citation2012) studied the nonparametric estimations of first and second infinitesimal moments based on the local linear estimators for jump-diffusion models.
In fixed symmetric kernel, the allocation of weights is outside the density support when smoothing is made and it causes the boundary bias. To avoid the boundary bias, Chen (Citation2000) proposed a gamma kernel function as the gamma density function that has flexible shapes and lies within [0, . The gamma kernel function is defined as
where is the gamma function, x is the design point, and b the smoothing parameter. The density function of gamma distribution having support
implies that gamma kernel function does not generate the boundary bias. Furthermore, the asymptotic properties of the gamma estimators depend on the position of the design point x.
Beta kernel function can be written as
where is the beta function and b is the smoothing parameter. The density function of beta distribution has support [0, 1]. Beta kernel function has unique features as the shape and the amount of smoothing change according to the position of the design points x. Beta kernel assigns no weight outside the data support and is free from the boundary bias.
Remark 1
In fixed kernel function, one works by using whereas in asymmetric kernel uses
Furthermore,
is not fixed and varies according to the design point x.
The Gamma Nadaraya–Watson estimators of and
are denoted by
and
defined by
(2.8)
(2.8)
and(2.9)
(2.9)
respectively. Whereas, ,
and
as
Similarly beta Nadaraya–Watson estimators of
and
are obtained by replacing
with
in Equations (2.8) and (2.9). We obtain the consistency and asymptotic normality of the proposed estimators under recurrence and stationarity.
3. Assumptions and main results
The assumptions we need for the proofs of the main results are listed below for convenient reference. Assume that is the range of the process
Let
be the scale density function and denotes the speed function of the process
(see Bandi & Phillips, Citation2003). Moreover,
is any fixed point belonging to the interval specified above.
A1.
(i) | The coefficients | ||||
(ii) | There exist constants | ||||
(iii) |
|
Remark 2
Assumption A1 assures existence and uniqueness of a strong solution to the stochastic differential Equation (2.1).
A2.
The solution to Equation (2.1) is positive Harris recurrent.
Remark 3
Harris recurrence guarantees the existence and uniqueness of invariant measure s(dx). This assumption is much weaker than the usual assumption.
A3.
,
and
as
such that
(3.4)
(3.4)
where is defined in Lemma 3.
A4.
,
and
as
such that
Now we describe results regarding the local time that will be useful in obtaining the main results of this paper (see, Revuz & Yor, Citation2003).
Lemma 1
Let the process be a semimartingale with quadratic variation
and
be local time at a. Then for every positive bounded Borel measurable function g
Lemma 2
Let X be a semimartingale satisfying a.s.
Then, for any a and t, we have
Lemma 3
Let be the process defined by Equation (2.1) and
as
see, e.g. Bandi and Nguyen (Citation2003). If
(3.5)
(3.5)
then(3.6)
(3.6)
It is well known that for recurrent diffusion process, the local time for a fixed T can be consistently estimated by(3.7)
(3.7)
based on asymmetric kernel (gamma kernel). Similarly, we can obtain results for beta kernel function by replacing with
We now give the main results of our paper.
Theorem 1
Under assumptions A1–A3,
Furthermore, if then for “interior x”
(3.8)
(3.8)
where denotes bias of the estimator
, given by
Similarly for “boundary x”, if then
(3.9)
(3.9)
Theorem 2
Under assumptions A1–A3,
Furthermore, if then for “interior x”
(3.10)
(3.10)
where
Similarly for “boundary x”, if then
(3.11)
(3.11)
In available literature, the asymptotic law of gamma and beta Nadaraya–Watson estimators can also be obtained by assuming the stationarity of the process We assume that the speed function m(x) satisfies
where f(x) is the stationary distribution. Furthermore, it requires that
and
The results are given for such an idea in the following corollaries for “interior x” and “boundary x”, respectively.
Corollary 1
Under assumptions A1 and A4,
Then for “interior x”, if then
(3.12)
(3.12)
where denotes bias of the estimator
, given by
Similarly for “boundary x”, if then
(3.13)
(3.13)
Corollary 2
Under assumptions A1 and A4,
Then for “interior x”, if (3.14)
(3.14)
where
Furthermore, for “boundary x”, if then
(3.15)
(3.15)
An important feature of the gamma kernel estimators is that the variance coefficient decreases as x increases. This property does not hold with any of the fixed symmetric kernels. The large value of x reduces the variance while it increases the bias of gamma kernel function. The shape of gamma kernel varies along with the position of x and the smoothing bandwidth can be changed from one place to another.
The results for the beta kernel are obtained by replacing x with in variance and bias of gamma kernel. For “interior x” design point x satisfies
,
and for “boundary x” it satisfies
,
for some
as
Furthermore, the standard theory on beta distribution has mean
and variance
, respectively.
4. Proofs
Proof of Lemma 3.
By Lemma 1 and 2, we obtain(4.1)
(4.1)
with probability one, around
In order to obtain the main results, it is necessary to show(4.2)
(4.2)
which is equivalent to(4.3)
(4.3)
The left of Equation (4.3) is bounded by(4.4)
(4.4)
where is the some value between
and
Let
It follows from the Lévy continuity of modulus for diffusion process that(4.5)
(4.5)
Using Equation (4.5) and A3, we acquire
The first term on the right-hand side of Equation (4.4)
around . Similarly we can also show the remaining quantities in Equation (4.4). It completes the proof of Lemma 3.
4.1. Proof of Theorem 1
To prove the consistency of we begin by writing
(4.6)
(4.6)
We start with
which implies that around
. Now consider
, the strong law of large number for martingale difference with zero mean and finite variance Hall and Heyde (Citation1986), yields
which can be proved by using Knight’s embedding theorem Revuz and Yor (Citation2003). Since
is martingale, it implies that
. It completes the proof of the consistency of
To derive the asymptotic normality of write
We evaluate these terms separately.
Using A2 and Quotient Limit Theorem for Harris recurrent Markov processes Aźema, Kaplan-Duflo, and Revuz (Citation1967), can be written as
where s(dx) is the finite invariant measure. The standard theory on gamma distribution has mean
and variance
Using the Taylor expansion for
around
, we get
(4.7)
(4.7)
The involvement of in the bias is less desirable, which is due to the fact that x is not the mean of gamma kernel rather it is the mode.
Now we consider and
. To obtain the results, we use the quadratic variation process. We take numerator of
(4.8)
(4.8)
Define
Then (4.8) can be written as
by using Lemma 1. Similarly the numerator of yields
(4.9)
(4.9)
From Chen (Citation2000), we have(4.10)
(4.10)
Hence, for “interior x”
and
Therefore(4.11)
(4.11)
and(4.12)
(4.12)
If we obtain
where denotes bias of the estimator
, given by
The results for “boundary x” can be established similarly. It completes the Proof of Theorem 1.
4.2. Proof of Theorem 2
To prove the consistency of note that
Inserting the four terms into we seprate
into four parts
The first term(4.13)
(4.13)
(4.14)
(4.14)
around .
We now consider . Previous arguments imply that
then(4.15)
(4.15)
Similarly, we can also obtain(4.16)
(4.16)
and(4.17)
(4.17)
Combining Equations (4.14), (4.15), (4.16), and (4.17), we get
It shows the consistency of estimator
For asymptotic normality of we proceed as
First, we consider
where s(dx) is the finite invariant measure. Taylor expansion for
around
gives
(4.18)
(4.18)
The quadratic variation process of provides that
Following Equation (4.8), for “interior x”
and(4.19)
(4.19)
For , it is similar to (4.9). We omit it here.
If then we get
where
Similarly, we can obtain the results for “boundary x”. This leads to the Proof of Theorem 2.
For beta kernel function, Chen (Citation2000) provided that
where d is a nonnegative constant. Furthermore, to get the results, it needs to define
The rest of the procedure is similar to gamma kernel as prescribed above.
Our findings indicate that the corollaries can be proved on the same steps as followed in the case of theorems.
Additional information
Funding
Notes on contributors
Muhammad Hanif
Muhammad Hanif is an associate professor in the Department of Mathematics and Statistics, PMAS-Arid Agriculture University, Rawalpindi, Pakistan. His major research interests are Statistical Inference for Stochastic Processes, Mathematical Statistics, Nonparametric Estimation.
References
- A\’{I}t-Sahalia, Y., & Jacod, J. (2009). Testing for jumps in a discretely observed process. Annals of Statistics, 37, 84–222.
- Arapis, M., & Gao, J. (2006). Empirical comparisons in short-term interest rate models using nonparametric methods. Journal of Financial Econometrics, 4, 310–345.
- A\’{z}ema, J., Kaplan-Duflo, M., & Revuz, D. (1967). Measure invariante sur les classes recurrents des processus de Markov. Zeit Wahrsh Verwand Gebie, 8, 157–181.
- Bandi, F. M., & Nguyen, T. H. (2003). On the functional estimation of jump-diffusion models. Journal of Econometrics, 116, 293–328.
- Bandi, F. M., & Phillips, P. (2003). Fully nonparametric estimation of scalar diffusion models. Econometrica, 71, 241–283.
- Beckers, S. (1981). A note on estimating the parameters of the diffusion jump model of stock returns. Journal of Financial and Quantitative Analysis, 16, 127–140.
- Bertin, K., & Klutchnikoff, N. (2011). Minimax properties of beta kernel estimators. Journal of Statistical Planning and Inferences, 124, 2287–2297.
- Bouezmarni, T., & Scaillet, O. (2005). Consistency of asymmetric kernel density estimators and smoothed histograms with application to income data. Econometric Theory, 21, 390–412.
- Chen, S. X. (2000). Probability density function estimation using gamma kernels. Annals of the Institute of Statistical Mathematics., 52, 471–480.
- Fan, J., & Gijbels, I. (1992). Variable bandwidth and local linear regression smoothers. Annals of Statistics, 20, 2008–2036.
- Gospodinovy, N., & Hirukawa, M. (2012). Nonparametric estimation of scalar diffusion processes of interest rates using asymmetric kernels. Journal of Empirical Finance, 19, 595–609.
- Gustafsonn, J., Hagmann, M., Nielsen, J. P., & Scaillet, O. (2009). Local transformation kernel density estimation of loss distributions. Journal of Business and Economic Statistics, 27, 161–175.
- Hall, P., & Heyde, C. C. (1986). Martingale limit theory and its applications. New York, NY: Academic Press.
- Hagmann, M., & Scaillet, O. (2007). Local multiplicative bias correction for asymmetric kernel density estimators. Journal of Econometrics, 141, 213–249.
- Hanif, M. (2012). Local linear estimation of recurrent jump-diffusion models. Communications in Statistics: Theory and Methods, 41, 4142–4163.
- Hanif, M., Lin, Z. Y., & Wang, H. C. (2012). Reweighted Nadaraya--Watson estimator of jump-diffusion models. Science China Mathematics, 55, 1005–1016.
- Hanif, M. (2015). Nonparametric estimation of second order diffusion models by using asymmetric kernels. Communications in Statistics: Theory and Methods, 44, 1896–1910.
- Kou, S. G. (2002). A jump-diffusion model for option pricing. Management Science, 48, 1086–1101.
- Lin, Z. Y., & Wang, H. C. (2010). Empirical likelihood inference for diffusion processes with jumps. Science China Mathematics, 53, 1805–1816.
- Merton, R. C. (1976). Option pricing when underlying stock returns are discontinuous. Journal of Financial Economics, 3, 125–144.
- Nicolau, J. (2003). Bias reduction in nonparametric diffusion coefficient estimation. Econometric Theory, 19, 754–777.
- Ramezani, C. A., & Zeng, Y. (2007). Maximum likelihood estimation of the double exponential jump-diffusion process. Annals of Finance, 3, 487–507.
- Revuz, D., & Yor, M. (2003). Continuous Martingales and Brownian motion. New York, NY: Springer.
- Rice, J. (1984). Boundary modification for kernel regression. Communications in Statistics: Theory and Methods, 13, 893–900.
- Scaillet, O. (2004). Density estimation using inverse and reciprocal inverse Gaussian kernels. Journal of Nonparametric Statistics., 16, 217–226.
- Schmisser, \’{E}. (2014). Non-parametric adaptive estimation of the drift for a jump diffusion process. Stochastic Processes and Their Applications, 124, 883–914.
- Shunpu, Z. (2010). A note on the performance of the gamma kernel estimators at the boundary. Statistics and Probability Letters, 80, 548–557.
- Stanton, R. (1997). A nonparametric model of term structure dynamics and the market price of interest rate risk. Journal of Finance, 52, 1973–2002.
- Zhang, S., Karunamuni, R. J., & Jones, M. C. (1999). An improved estimator of the density function at the boundary. Journal of the American Statistical Association, 94, 1231–1241.