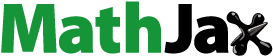
Abstract
In this paper, the problem examined concerns a firm entering a new facility in the market where facilities belonging to the firm and another competitor firm exist. The market’s reverse logistics network that has attracted growing attention with stringent pressures from environmental and social requirements is considered. The firm wants to maximize its profit by finding the best location and the most attractive facility to open. The other firm (the competitor) can counteract this situation and attempt to maximize its own profit by adjusting the attractiveness of its existing facilities. The demand is assumed to be aggregated at certain points in the plane and the facilities of the firm can be located at pre-determined candidate sites. To do this, Huff’s gravity-based rule in modeling the behavior of the customers is employed where the fraction of customers at a demand point that visit a certain facility is proportional to the facility attractiveness and inversely proportional to the distance between the facility site and demand point. A mathematical bi-level mixed-integer nonlinear programming model where the firm entering the market is the leader and the competitor is the follower is delivered. Furthermore, for finding the optimal solution of this model, it is converted into a one-level mixed-integer nonlinear program so that it can be solved by global optimization methods. The computational results on some examples obtained from random generated problems show that the method is able to solve the Bi-Level Programming Problem (BLPP) efficiently in a reasonable time.
Public Interest Statement
Competitive facility location (CFL) problems differ from the classical facility location problems considered in the operations research area because they incorporate the competition among the facilities belonging to different firms. The new facility or facilities to be located by a firm have to compete with the facilities of the other firm(s) that are already (or will be) present in the market in order to capture market share. The nature of the competition is the primary factor determining the class of the CFL problem.
In this paper, the proposed model is applicable for sales and marketing systems which encompass at least two firms as competitors. The solution methodology and the proposed model can be used separately as a basic way for using in the same work in mathematics, engineering science, or even management branches.
1. Introduction
In many competitive location problems, a firm wants to open a set of new facilities to provide goods to customers of a given geographical area where other competing firms offering the same goods are already present. The new facility or facilities to be located by a firm have to compete with the facilities of other firm(s) that are already (or will be) present in the market in order to capture market share. Many factors influence such decisions, including the available budget and expansion strategy of the firm, the location of the firm’s existing facilities and their performance, location of competitors’ facilities, the market size, demographics (e.g. population, age, gender, purchasing power) of the demand-generating population, target customer segments, availability and logistical suitability of candidate locations, and existence of other nearby attractions for generating traffic.
Huff (Citation1964, 1966) proposed one basic formulation for estimating the market share among the competing facilities. He assumes that the probability that a customer patronizes a certain facility is proportional to its floor area and inversely proportional to a certain power of the distance from it. Huff’s model has been improved in (Cooper & Nakanishi, Citation1974) by replacing the floor area by a product of attraction factors. One application of this model has been found in (Jane & Mahajan, Citation1979) and then (Hadgson, Citation1981) which suggested to replace the power of the distance by an exponential function of the distance which accelerates the distance decay. Literature reviews encompass “the probability of consumers patronizing a facility” called attractiveness. It means that a customer patronizing certain facility is proportional to its attraction toward the facility. This paradigm is expressed as a parameter called the facility quality (depends on the characteristics of the facility), divided by a non-negative nondecreasing function of the distance from it. (For more details see (Drezner, Citation1994), (Fernández, Pelegrín, Plastria, & Tóth, Citation2007)) In their models, the market share captured by a facility depends on its quality and its location.
In this paper, it is supposed that the firm as leader wants to locate a single new facility in a given area of the plane. In this chain, already m + s facilities exist which offer the same good or product. It is further supposed that the first m facilities belong to the leader and the next s facilities belong to the competitor firm as follower. This problem includes competitive facility location CFL problem. It was assumed that the demand is inelastic and concentrated at n demand points; these demand points include both demand points for new products (delivered from facilities to customers) and used products (delivered from customers to facilities).
The primary works comprising of the CFL problem are (Hakimi, Citation1983) and (Revelle, Citation1986). In these problems, the new facilities must be located on a network space to compete with a number of existing (competitor) facilities. The authors use the assumption that a customer always visits the nearest open facility. According to Huff, customers’ patronization of different facilities depends on the attractiveness of each facility and is also inversely proportional to the distance to the facility. This means that customers may not always choose the nearest facility; they may sometimes visit a distant facility because it is more attractive. This idea has been extended a step further by introducing additional attributes for GIS-Based by (Nakanishi & Cooper, Citation1974) (a geographic information system or geographical information system (GIS) is a system designed to capture, store, manipulate, analyze, manage, and present all types of spatial or geographical data).The optimization attitude for Competitive Multi-Facility Location-Routing Problem is calculating facility attractiveness in the form of a multiplicative interaction model. In this work, this rule is applied. Moreover, our considered chain includes forward and reverses logistics operations via three kinds of facilities; forward processing facility, backward processing facility, and hybrid processing facility. New products are delivered to a number of geographically dispersed customers from plants via forward processing facilities. Used products are taken back from the customers and shipped to the plants via backward processing facilities for the purpose of recovery or safe disposal. In this paper, instead of only handling separate forward processing and collection facilities, a type of intermediate depot, namely hybrid processing facility, is also taken into account. Both new products and used products can be transferred via hybrid processing facilities. Advantages of building such hybrid processing facilities might include cost savings and pollution reduction as a result of sharing material handling equipment and infrastructure.
We found how to manipulate the B&B method by reviewing existing B&B methods in (Dempe & Gadhi, Citation2007) for solving Bi-level programming problem (BLPP). However, B&B methods require linear or convex quadratic nonlinear functions in the lower level problem of the bi-level programming (BP) models. In our bi-level model, the objective function of the follower’s problem is nonlinear and non-quadratic. Thus, for applying a solution method, the mixed-integer nonlinear BP formulation is firstly converted into a one-level mixed-integer nonlinear programming (MINLP) problem by using the fact that the follower’s problem is a continuous concave maximization problem. Therefore, the Karush–Kuhn–Tucker optimality conditions make it necessary and efficient. Although the NLP relaxation of resulting equivalent one-level MINLP problem is not a concave programming problem, we transformed it by introducing new variables so that it can be solved for global optimality by applying the General Structure Mixed-Integer Nonlinear a BB (GMIN-aBB) algorithm which is based on parameters and branch and bound method.
The rest of this paper is organized as follows: Section 2 describes the model formulation. In Section 3, an insight into the solution method is provided. In Section 4, concluding remarks and related examples are summarized and finally in Section 5 some conclusions are drawn.
2. Model description
In this section, the problem faced by the firm during the course of entering a single facility into the market is at first described. Then, BP formulation is delivered in which the market entrant firm that attempts to enter (locate) a facility is the leader and the other competing firms (referred to as the competitor) existing in the market are the followers. The firm wishes to determine the optimal location and attractiveness of the new kind of facility to be opened so as to maximize its profit. In addition, there are m existing facilities belonging to the leader and sexisting facility belongs to the competitor. It is assumed that the follower reacts to the market entry of the new facility (by leader) via adjusting the attractiveness of some or all of its existing facilities for maximizing its profit. Attractiveness level of a facility is determined by a function of its attributes related to the facility. For example, when the considered facility is a shopping mall, these factors include the variety of stores, availability of food, court/restaurants, adequate parking, accessibility by public transportation, selling price of products and existence of movie theaters. (Note that decreasing the attractiveness to zero means that the facility is shut down).
Let J denote the set of customer locations and j ∊ J indicate individual customer locations which is considered as aggregate population demand centers in our model. Furthermore, let E, M and S denote the set of potential location, leader and follower facilities, respectively. In our problem setting, there are n1 demand points of new products and there are n2 = n – n1 demand points of used products which are indexed by j.
The other parameters and decision variables of the model are as follows:
hj | = | Annual buying power at point j of both new and used products |
cl | = | Unit attractiveness cost at candidate site l |
= | Unit attractiveness cost or revenue of follower’s facility at site k | |
= | Annualized fixed cost of opening a forward processing facility at site l | |
= | Annualized fixed cost of opening a hybrid processing facility at site l | |
= | Annualized fixed cost of opening a backward processing facility at site l | |
= | Maximum attractiveness level for a forward (hybrid, backward) processing facility to be opened at site l | |
dlj | = | Euclidean distance between candidate site land point j |
= | Euclidean distance between existing facility site k and point j | |
= | Current attractiveness level of competitor’s facility at site k | |
= | Maximum attractiveness level of competitor’s facility at site k | |
Decision variables | = | |
= | Attractiveness of the forward (hybrid, backward) processing facility opened at site l | |
xl | = | Binary variable which is equal to one if a facility is opened at site l, and zero if otherwise |
Ak | = | New attractiveness level of competitor’s facility at site k |
A location solution vector X consists of binary decision variables where l ∊ E and
indicates facility l is open for forward (hybrid, returned) processing facility, i.e. new facility is established in candidate location j.
We assume that each population center patronizes an open facility belonging to set E, M, and S proportional to the facility’s attractiveness score and inversely proportional to the distance between the demand center and the facility. In other words, each demand center has a “utility” towards an open facility which is calculated as shown in the following formulation:
Hence the patronage probabilities of a demand center j against all open facilities are formulated as follows:
Under this patronization behavior, customers visit facilities according to probabilities pij and contribute to the overall revenues that are collected by the leader firm and its competitor follower. Consequently, the proportion pij of customers at point j (formulation is the same for both the new demand and used product) who visit a new facility at site l is expressed as:
Now, we can formulate the following bi-level MINLP model P as follows:(1)
(1)
(2)
(2)
(3)
(3)
(4)
(4)
(5)
(5)
(6)
(6)
(7)
(7)
(8)
(8)
(9)
(9)
The objective function (1) of the leader firm has four components. The first one (resp. the second one) represents the revenue of new (resp. used) products which is collected by the new (resp. pervious) facility of leader firm. The third and the forth components represent the fixed cost and attractiveness cost associated with opening the new facility, respectively. Constraints (2)–(4) along with the binary location and restrictions (5) and (6) on the location variables and non-negativity restrictions existing in (6) on attractiveness variables
ensure that if no facility is opened at site l, then the corresponding attractiveness
of the facility is zero and if a facility is opened at site i, then its attractiveness
cannot exceed the maximum level
. The objective function of the competitor (7) contains the summation of three terms: the revenue collected by the competitor’s facilities and the cost or revenue associated with re-adjusting the attractiveness levels. Constraints (8) and (9) ensure that the new attractiveness Ak of an existing facility at site k is between zero and an upper limit Ak.
For the solution of the above MINLP model, the GMIN-aBB algorithm was employed which will be explained in detail in Section 3. This algorithm performs a pre-processing step where all the terms in the objective function as well as in the constraints are grouped into different classes such as linear, fractional, concave, bilinear, univariate convex, and general nonconcave. Then, for each term in all classes, with the exception of the linear and concave ones, a concave over-estimator is generated.
3. Solution methodologies
Global optimization of BLPP is studied in (Gümüş & Floudas, Citation2005). Firstly, they propose a convex relaxation of the inner problem followed by its equivalent representation via necessary and sufficient optimality conditions. The, they introduce a BB global optimal principles presented as branch and bound framework. Their problems involve twice differentiable continuous functions. The first rigorous global optimization approach for the calculation of the flexibility test which is bi-level nonlinear optimization model is introduced by (Floudas et al., Citation1999). They demonstrate the applicability of their model to a heat exchanger network problem, a pump and pip problem, a reactor-cooler system, and a prototype process flow sheet model. (Pistikopoulos, Georgiadis, & Dua, Citation2007) introduce methods based on parametric programming to transform the bi-level problem into a family of single level optimization problems which can be solved for global optimality. The authors present computational results on several small benchmark linear–linear, linear–quadratic, quadratic–linear, and quadratic–quadratic type problems. (Gümüş & Floudas, Citation2005) proposes two new methods for BLPP. The first one is applicable when the outer problem includes mixed integer nonlinear and inner problem encompasses twice differentiable continuous problems; the second method is applied to problems including the inner problem featuring functions which are mixed integer nonlinear in the outer variables, and linear, polynomial or multi-linear in the inner integer variables and linear in the inner continuous variables.
In this work, the aBB global optimal approach is proposed for the efficient solution of the proposed problem.
The approach is based on the concept of feasible domain relaxation and the basic principles of the global optimization algorithm a BB. The main feature of the presented approach is that it guarantees convergence to the global optimal solution for problems that are described by general nonconvex constraints. The basic framework of the applied method is described as followed:
(1) | If any of the constraints are nonconvex, the inner level of the feasibility problem is nonconvex. Therefore, the Karush–Kuhn–Tucker (KKT) optimality conditions are necessary but not sufficient to guarantee the global optimum of the inner level. Local or even suboptimal solutions may be obtained. Hence, the first step of the proposed framework involves the convexification of the feasible region defined by the inner constraints of the original problem. The basic underestimation principles of the aBB global optimization approach are utilized to underestimate the constraints nonconvex. For the convexified problem, the KKT optimality conditions are necessary and sufficient, assuming that the linear independence constraint qualification is satisfied. Under these conditions, the convexified inner level optimization problem is equivalent to its KKT optimality conditions. | ||||
(2) | The convexified inner level optimization problem is replaced with its equivalent set of equations that are defined by the KKT optimality conditions; this transforms the bilevel problem into a single level optimization problem. Note that this problem is in general a nonconvex optimization problem due to the complementarity conditions. As a result, further convex underestimation may be needed which takes place according to aBB principles and the solution of the convexified single stage problem provides an upper bound of the original problem P. | ||||
(3) | A lower bound to the problem P is determined through a feasible solution of the original Mixed Integer Nonlinear Programming. MINLP formulation is obtained by substituting the inner problem by the KKT optimality conditions (conditions which are only necessary) as proposed by Grossmann and Floudas. | ||||
(4) | The next step after establishing an upper and a lower bound on the global solution is to refine them. This is accomplished by successfully partitioning the initial region of the variables into smaller ones. The partitioning strategy involves the successive subdivision of a hyperectangle into two sub-rectangles by halving at the middle point of the longest side of the initial rectangle (bisection). In any iteration, the lower bound of the feasibility test and the flexibility index problem is the maximum over the minima found in every sub-rectangle composing of the initial rectangle. Consequently, on-decreasing sequence of lower bounds is generated by halving the sub-rectangle that is responsible for the infimum over the minima obtained at any iteration. A non-increasing sequence of upper bounds is derived by solving the nonconvex MINLP single optimization problem obtained after the substitution of the inner problem by the KKT optimality conditions, and selecting as an upper bound the minimum over all previously determined upper bounds. If at any iteration the solution of the convexified MINLP in any sub-rectangle is found to be greater than the upper bound or the solution is not feasible, this sub-rectangle is fathomed since the global solution cannot be found inside it. |
Thus, as described above, the proposed procedure for solving the problem involves the following steps:
Step 1. Set the lower bound LB = − ∞, upper bound UP = ∞ and select a tolerance ɛ.
Step 2. Substitute the convexified constraints instead of the inner constraints: we will show that the inner problem of P has concave objective function with convex constraints, and therefore it does not need any convexification in this step.
Proposition 1. The objective function
is concave.
The constraints of inner problem are linear and so are convex.
Step 3. Substitute the inner optimization problem of the original problem by the KKT optimality conditions:
where and
are Lagrangean multiplier vectors and s1 = (s11, s12, …, s1s) and s2 = (s21, s22, …, s2s) are slack variables corresponding to constraint sets and , respectively. However, we can remove the nonlinearity caused by the complementarily constraints using the active set strategy suggested by Grossmann and Floudas (Grossmann & Floudas, Citation1987). For example, consider nonlinearity phrase, Grossmann and Floudas condition for this phrase stated as:
where , and M is an upper bound on the slack variables s1k, s2k and y1k, y2k for k = 1, 2, …, s. Consequently, the problem P transformed the following one-level problem:
(10)
(10)
(11)
(11)
(12)
(12)
(13)
(13)
(14)
(14)
(15)
(15)
(16)
(16)
(17)
(17)
(18)
(18)
(19)
(19)
(20)
(20)
(21)
(21)
(22)
(22)
(23)
(23)
Step 4. The resulting formulation P′ is an MINLP model. For its solution, the GMIN-aBB algorithm was employed. To do this, both upper bound and lower bound of the problem P were obtained. First, it was necessary to get rid of nonconvex terms. To do so, new variables v1j,v2j for j = 1, 2, …, n1 and new variables v3j, v4j for j = n1 + 1, 2, …, n were defined as follows:(24)
(24)
(25)
(25)
(26)
(26)
(27)
(27)
(28)
(28)
(29)
(29)
Therefore, by using these new variables P′ is written as below:(30)
(30)
(31)
(31)
(32)
(32)
(33)
(33)
(34)
(34)
(35)
(35)
(36)
(36)
(37)
(37)
(38)
(38)
(39)
(39)
(40)
(40)
(41)
(41)
(42)
(42)
(43)
(43)
(44)
(44)
(45)
(45)
(45)
(45)
(47)
(47)
(48)
(48)
(49)
(49)
(50)
(50)
(51)
(51)
In the above formulation, nonconvex terms are eliminated, but the cost of this work is introducing new bilinear and fractional terms into the formulation. It helps to avoid the computationally intensive calculations used in the convexification procedure of the terms. To obtain the upper bound, the relaxed version of P″ needed to be solved. Firstly, it was necessary to convexify the nonconvex in it except for the linear and convex ones. The terms for which an over-estimator should be constructed are the fractional and bi-linear terms. To do this, the procedure given by ( Floudas, 2000 ) was used:
Let v1jv2j = w1j, v3jv4j = w2j, ,
,
,
, v5jv2j = w7j, and v6jv2j = w8j. Then, to overestimate the fractional terms the following linear constraints are added to P″:
(52)
(52)
(53)
(53)
(54)
(54)
(55)
(55)
(56)
(56)
(57)
(57)
(58)
(58)
(59)
(59)
And the bilinear terms are overestimated using the following linear constraints:(60)
(60)
(61)
(61)
(62)
(62)
(63)
(63)
(64)
(64)
(65)
(65)
(66)
(66)
(67)
(67)
(68)
(68)
(69)
(69)
(70)
(70)
(71)
(71)
(72)
(72)
(73)
(73)
(74)
(74)
(75)
(75)
where and
are the lower and upper bounds of the on
, respectively. Therefore, the upper bound of the problem P″ will be obtained by solving the following convexified problem P‴ at a given node of the B&B tree when binary variables are relaxed.
(76)
(76)
(77)
(77)
And Equations (31)–(35) and (37)–(50) and (52)–(75)
In addition, at the root node of the tree, a local optimal solution is found for the original NLP, which gives a lower bound. In fact a lower bound is obtained by finding a local optimal solution to the problem using, for example, a commercial solver CPLEX that can handle a nonlinear programming problem. To guarantee ɛ-convergence from the global maximum, i.e. the difference between the lower and upper bounds is within ɛ of the optimal objective value, the feasible region of the problem is divided at each node such that the rectangles defined by the lower and upper limits on the decision variables are partitioned into smaller ones. This partitioning constitutes the branching mechanism in the B&B tree only.
Algorithm
(1) | Set the lower bound LB = − ∞, Obtain the relaxed problem P′ by relaxing all integer variables. While there are active nodes in the tree, repeat the following steps: | ||||
(2) | Select an active node according to some branching rule. (Branching at a node is performed by considering the solution at that node and selecting the relaxed binary variable whose value is the closest to 0.5.) | ||||
(3) | Apply the aBB algorithm and obtain an upper bound at the active node from the solution of P′. | ||||
(4) | If a feasible solution is obtained, let LB = UB, where LB indicates a lower bound to the problem. Update ZLB by Report the solution with the objective value ZLB as the optimal solution. |
4. Computational analysis
The performance of the presented approach was verified experimentally. It was implemented in MATLAB software. All tests were conducted for a fixed number of iterations in random test instances for assessment. The instances were classified based on the number of potential facilities and number of customers. The following policy was applied for generating test instances (for each data-set, five different data instances were created):
• | The number of demand points belonging to the {5, 10, 20, 30} where every point encompasses both used and new products | ||||
• | The number of candidate sites s belonging to {2, 5, 7} | ||||
• | The number of existing facility of competitors belonging to {2, 3} | ||||
• | Points representing the facilities and clients are uniformly distributed in [0,100] × [0,100] | ||||
• | The annualized buying power hj of customers at point j of new product are uniformly distributed in [10, 10,000] with normal probability distributions in N(50.05, 16. 52) and the annualized buying power hj of customers at point j of used product are uniformly distributed in [5, 5,000] and have normal probability distributions in N(40, 10.52) | ||||
• | The attractiveness cost ci of candidate facility for the leader at point i are real-valued quantities and uniformly distributed in [0.5,5] with normal probability distributions in N(5, 1.52) | ||||
• | The attractiveness cost \tilde{c}_{k} of facility of follower at point k are real-valued quantities and are uniformly distributed in [0.5, 5] with normal probability distributions in N(5, 1.52) | ||||
• | The current attractiveness cost \tilde{A}_{k} of facility at point k are real-valued quantities and are uniformly distributed in [10, 1,000] with normal probability distributions in N(500, 1402) | ||||
• | The fixed costs f_{i}^{f} ,f_{i}^{h}and f_{i}^{r} are set as 800ci,1,100ci and 550ci respectively | ||||
• | Upper bounds u_{l}^{f} ,u_{l}^{h}and u_{l}^{r} are taken as 7,500ci, 8,000ci, and 7,000ci respectively, and upper bound \bar{A}_{k} is assigned a value of 7,500\tilde{c}_{k}. |
As can be seen, GMIN-aBB is computationally expensive especially for very small values of the convergence parameter ɛ. To improve the running time efficiency of GMIN-aBB, instead of terminating the aBB algorithm when the difference ZUB − ZLB ≤ ɛ%, the iterations are stopped as soon as the improvement in the gap ZUB − ZLB between two non-successive iterations becomes less than a user-specified threshold value δ, namely the exploration of BB tree terminated when
where and
are the upper bounds at iterations t and t – k, respectively, while
and
are the best lower bounds at iterations t and t–k, respectively. k is a user-specified parameter that represents the number of iterations during which the improvement in the gap between ZUB and ZLB is measured. In our examples, k = 4 and δ = 0.1.
We present the results on the test instances in the following Tables. In these tables, indicates the best lower bound of the leader obtained by the GMIN-aBB algorithm run with the above-mentioned approximation strategy, and
, stands for the profit realized by the follower as a reaction to the leader.
The computational results in Table show that the GMIN-aBB method solves the proposed model very efficiently and the model is extremely efficient in finding integer solutions. Most of the time, GMIN-aBB method was not invoked to obtain integer solutions, which shows that our model is integer- friendly. It is worth noting that our method for obtaining a lower bound often generated an optimal solution or a high-quality approximate solution with only one or two locations different from the optimal location. Furthermore, for showing the affectedness of attractiveness adjustment of the follower facilities, the advantages of the leader and the disadvantages of the competitor were firstly quantified when the leader takes into consideration the reaction of the competitor (fixed Ak). In the second stage, the aim was to quantify the benefit of the leader and the benefit of the competitor when the leader takes into consideration the reaction of the competitor but when the upper bound of the attractiveness is changed to half . For each instance, the percentage of loss from the competitor was computed.
Table 1. computational results obtained by GMIN-aBB
The conclusion drawn from the results is that the competitor almost takes disadvantage of the leader’s effort when the attractiveness of competitor’s facility is decreased or fixed. In fact, with Ak decreasing, the objective value increases while the market share captured by Firm B shrinks, and the optimal locations tend to be nodes which are closer to the warehouse/plant since the transportation cost becomes the main cost (Table ).
Table 2. The loss of the competitor, in the case of 
and 

5. Conclusion
In this paper, we considered a relatively new discrete CFL problem in a closed chain with two competitors, which at first attempts to add new facility to its facilities so as to maximize own profits. It is assumed that there is a second competitor in the market which reacts to the opening of the new facility by adjusting the new attractiveness levels of its facilities with the objective of maximizing its own profits. As the market includes forward and reverse logistics network, we considered three kinds of facilities in this market: forward, backward, and hybrid processing facilities. A programming model is proposed which is a bi-level model and solves the problem outlined in this paper by using a global optimization method called GMIN-aBB after converting the bi-level model into an equivalent one-level MINLP model. This conversion is possible since the objective function of the competitor, which is the follower in the bi-level programming formulation, is concave in terms of the attractiveness variables when the locations and the attractiveness levels of the leader firm are fixed. We applied this method on the random generated examples and the computation results show the efficiency of the proposed method. For future research, one can consider an extension of the proposed bi-level model where the competitor reacts to the market entrant firm in the case of adjusting the attractiveness levels and also opening new facilities and/or closing existing ones. Furthermore, a combination of available algorithms such as branch and bound and multi-parametric could be helpful.
Acknowledgments
The author is grateful to the referees for their constructive suggestions for improving this paper.
Additional information
Funding
Notes on contributors
Arsalan Rahmani
He is a faculty member of the Mathematics Department at the University of Kurdistan, Sanandaj, Iran. Other information is outlined below:
Field of Specialty:
Combinatorial Optimization, Mathematical Modeling, Stochastic Programming.
2011–2015
PhD in Applied Mathematics (Operations Research).
Department of Mathematics and Computer Science, Amirkabir University of Technology, Tehran, Iran.
2009–2011
Msc in Applied Mathematics (Operations Research).
Department of Mathematics and Computer Science, Amirkabir University of Technology, Tehran, Iran.
2008
BSc in Pure Mathematics.
University of Kurdistan, Sanandaj, Iran.
Member of Operations Research Society Iran.
References
- Cooper, L. G., & Nakanishi, M. (1974). Parameter estimate for multiplicative interactive choice model: Least squares approach. Journal of Marketing Research, 11, 303–311.
- Dempe, S., & Gadhi, N. (2007). Necessary optimality conditions for bilevel set optimization problems. Journal of Global Optimization, 39, 529–542.10.1007/s10898-007-9154-0
- Drezner, T. (1994). Locating a single new facility among existing unequally attractive facilities. Journal of Regional Science, 34, 237–252.10.1111/jors.1994.34.issue-2
- Fernández, J., Pelegrín, B., Plastria, F., & Tóth, B. (2007). Solving a Huff-like competitive location and design model for profit maximization in the plane. European Journal of Operational Research, 179, 1274–1287.10.1016/j.ejor.2006.02.005
- Floudas, C. A. (2000). Deterministic global optimization: Theory algorithms and applications. Journal of Global Optimization, 18, 103–105.
- Floudas, C. A., Pardalos, P. M., Adjiman, C. S., Esposito, W. R., Gümüş, Z., Harding, S.T, & Schweiger, C. A. (1999). Handbook of test problems for local and global optimization. Kluwer Academic.10.1007/978-1-4757-3040-1
- Grossmann, I. E., & Floudas, C. A. (1987). Active constraint strategy for flexibility analysis in chemical processes. Computers and Chemical Engineering, 11, 675–693.10.1016/0098-1354(87)87011-4
- Gümüş, Z. H., & Floudas, C. A. (2005). Global optimization of mixed-integer bilevel programming problems. Computational Management Science, 2, 181–212.10.1007/s10287-005-0025-1
- Hadgson, M. J. (1981). A location—allocation model maximizing consumers' welfare. Regional Studies, 15, 493–506.10.1080/09595238100185441
- Hakimi, S. L. (1983). On locating new facilities in a competitive environment. European Journal of Operational Research, 12, 29–35.10.1016/0377-2217(83)90180-7
- Huff, D. L. (1964). Defining and estimating a trade area. Journal of Marketing, 28, 34–38.10.2307/1249154
- Huff, D. L. (1966). A programmed solution for approximating an optimum retail location. Land Economics, 42, 293–303.10.2307/3145346
- Jane, A. K., & Mahajan, V. (1979). Evaluating the competitive environment in retailing using multiplicative competitive intreaction model, research in marketing, 2, 217–235.
- Nakanishi, M., & Cooper, L. G. (1974). Parameter estimation for a multiplicative competitive interaction model-least squares approach. Journal of Marketing Research, 11, 303–311.10.2307/3151146
- Pistikopoulos, E. N., Georgiadis, M. C., & Dua, V., (2007). Multi-parametric programming, Weinheim: Wiley–VCH, 1.10.1002/9783527631216
- Revelle, C. (1986). The maximum capture or “sphere of influence” location problem: Hotelling revisited on a network. Journal of Regional Science, 26, 343–358.10.1111/jors.1986.26.issue-2